- 1Department of Biology, University of Rome Tor Vergata, Rome, Italy
- 2Department of Mathematics and Statistics, American University of Sharjah, Sharjah, United Arab Emirates
- 3Institute for Applied Computing, National Research Council of Italy, Rome, Italy
- 4Data Science Program, Sapienza University of Rome, Rome, Italy
- 5Fondazione Santa Lucia Istituto di Ricovero e Cura a Carattere Scientifico (IRCCS), Rome, Italy
Macrophages derived from monocyte precursors undergo specific polarization processes which are influenced by the local tissue environment: classically activated (M1) macrophages, with a pro-inflammatory activity and a role of effector cells in Th1 cellular immune responses, and alternatively activated (M2) macrophages, with anti-inflammatory functions and involved in immunosuppression and tissue repair. At least three different subsets of M2 macrophages, namely, M2a, M2b, and M2c, are characterized in the literature based on their eliciting signals. The activation and polarization of macrophages is achieved through many, often intertwined, signaling pathways. To describe the logical relationships among the genes involved in macrophage polarization, we used a computational modeling methodology, namely, logical (Boolean) modeling of gene regulation. We integrated experimental data and knowledge available in the literature to construct a logical network model for the gene regulation driving macrophage polarization to the M1, M2a, M2b, and M2c phenotypes. Using the software GINsim and BoolNet, we analyzed the network dynamics under different conditions and perturbations to understand how they affect cell polarization. Dynamic simulations of the network model, enacting the most relevant biological conditions, showed coherence with the observed behavior of in vivo macrophages. The model could correctly reproduce the polarization toward the four main phenotypes as well as to several hybrid phenotypes, which are known to be experimentally associated to physiological and pathological conditions. We surmise that shifts among different phenotypes in the model mimic the hypothetical continuum of macrophage polarization, with M1 and M2 being the extremes of an uninterrupted sequence of states. Furthermore, model simulations suggest that anti-inflammatory macrophages are resilient to shift back to the pro-inflammatory phenotype.
Author Summary
Macrophages are key players in the elicitation of an efficient immune response. Latest classification of macrophage functional types comprises the classically activated (M1) macrophages with a pro-inflammatory activity and the alternatively activated (M2) macrophages, with anti-inflammatory functions. The latter is further subdivided into at least three different subsets, namely, M2a, M2b, and M2c, which are characterized on the basis of distinct eliciting signals.
Accounting for the gene-related mechanisms of macrophage differentiation is a challenging task. We have used the methodology known as gene regulation network modeling on a newly constructed network of gene regulation originated from published experimental data. We have used computer simulations to explore the dynamical behavior of this network and derived conclusions about the hypothetical continuum of macrophage polarization with M1 and M2 being the extremes of an uninterrupted sequences of states. Our simulations also suggest that anti-inflammatory macrophages are resilient to shift to the pro-inflammatory phenotype.
Introduction
Macrophages and neutrophils of the innate immune system represent the first line of defense against most common microorganisms. Indeed, macrophages can recognize and respond to a wide range of stimuli, expressing a great variety of surface and intracellular receptors that activate several signal transduction pathways and complex gene expression patterns. Macrophages respond to extracellular stimuli upon contact with different cell types via endocytic, phagocytic, and secretory functions (Figure 1). Their activity is modulated by contact synapsis established with proximal cellular and molecular entities, including microorganisms, chemical mediators, and other macrophages (Gordon et al., 2014).
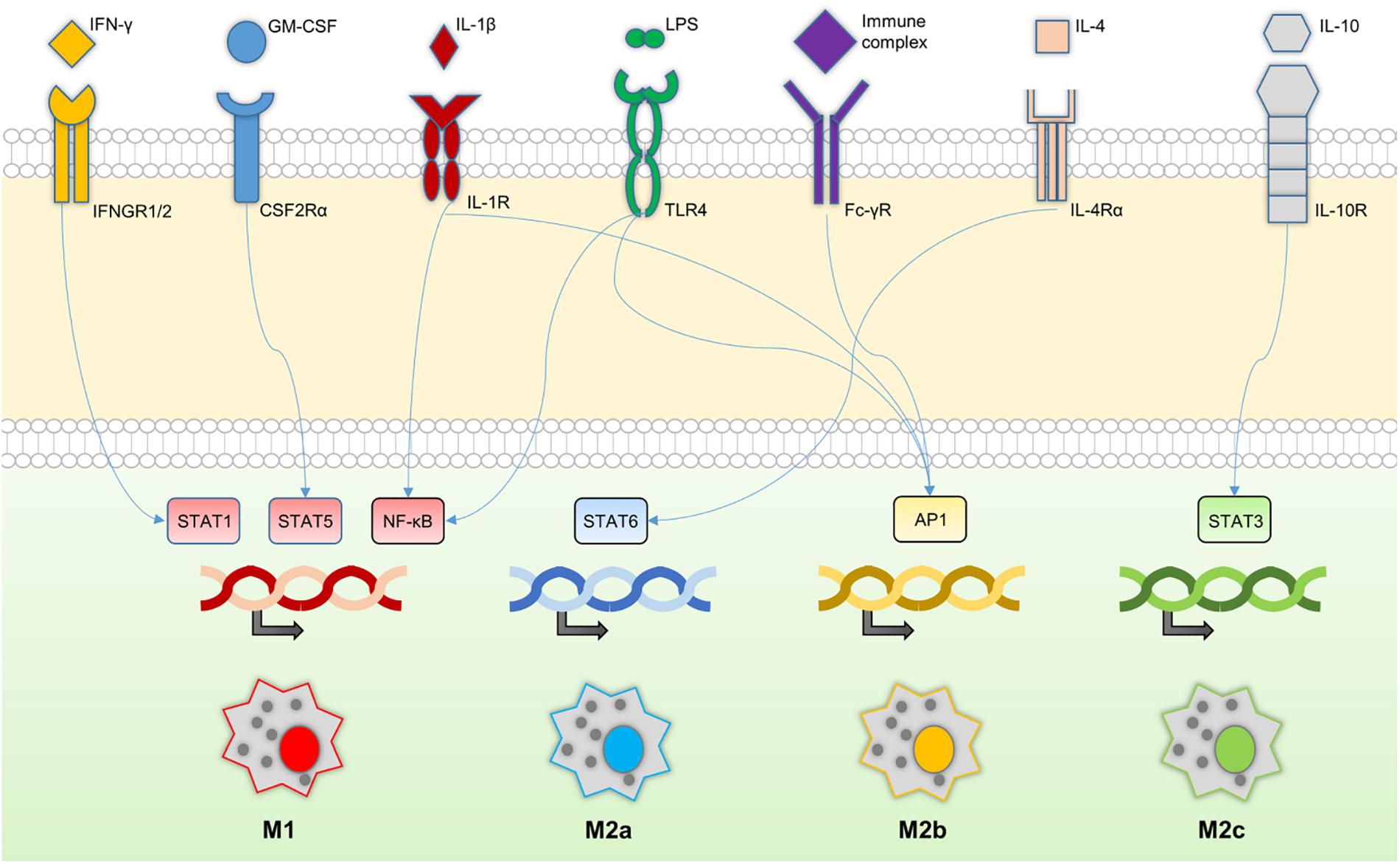
FIGURE 1. Macrophage signaling cascade. Macrophage receptors and their relationships with key transcription factors downstream of the signaling cascade. The transcriptions of different sets of genes lead to distinctive macrophage phenotypes; M1, M2a, M2b, and M2c.
The monocyte–macrophage differentiation pathway is known to exhibit plasticity and diversity (Mantovani et al., 2002; Bowdish et al., 2007; Gordon, 2008; Mantovani, 2008). Similar to the polarization process of helper T type 1 and 2 cells (Th1–Th2), two distinct polarized forms of macrophages have been recognized in the past: the classically activated (M1) macrophage phenotype and the alternatively activated (M2) macrophage phenotype (Biswas and Mantovani, 2010). Moreover, macrophages have also been observed in “M2-like” states, which share some features of both M1 and M2. Indeed, recent studies support the view that fully polarized macrophages (M1 and M2) are the extremes of a continuum of macrophage polarization (Mantovani, 2008). For example, various stimuli, such as immune complexes (IC) together with LPS or interleukin-1 beta (IL-1β), glucocorticoids, transforming growth factor-β (TGF-β), and interleukin-10 (IL-10), give rise to M2-like functional phenotypes that share properties with IL-4- or IL-13-activated macrophages [such as high expression of mannose receptor (MR) and IL-10, as well as TNFα, IL-1β, and IL-6] (Mantovani et al., 2004). Variations of the gene expression patterns corresponding to M1 or M2 are also found in vivo (e.g., in the placenta and embryo, and during helminthic infection, Listeria infection, obesity, and cancer) (Raes et al., 2005; Biswas et al., 2006; Kraakman et al., 2014).
The M1 and M2 phenotypes Kraakman et al., 2014 correspond to cell activation states driven by cytokines, which are typically secreted by Th1, Th2, and T-regulatory cells, but also basophils, mast cells, B lymphocytes, and eosinophils. The M1 phenotype is polarized by single or a combination of Th1 cytokines and pro-inflammatory mediators, including granulocyte-macrophage colony-stimulating factor (GM-CSF), tumor necrosis factor (TNF)-α, IL-6, IL-1β, IL-12, and various pathogen-associated molecules, such as lipopolysaccharide (LPS). By contrast, the M2 polarization is induced by macrophage colony-stimulating factor (M-CSF), IL-4 and IL-13, IC, IL-10, as well as glucocorticoid, TGFβ, and serotonin (Sang et al., 2015) (see Table 1).
Although there is a wealth of information about the different macrophage subsets in vitro, features such as plasticity, heterogeneity, and adaptability make them very difficult to study using conventional experimental tools. Furthermore, as many of the studies are done in different settings or for different goals, some literature reports are not conclusive and sometimes contradictory. It is not clear how robust the different macrophage subsets are to environmental changes. In particular, how does a modification of the cytokine environment affect the phenotype of macrophages? Which polarization state is most stable? Which possible gene knockouts can lead to a phenotypic change?
Macrophages polarization is essential in orchestrating the immune system response both in infectious and sterile immune settings. To shed light on this complex molecular process and address the questions above, we employed computational modeling of gene regulatory networks (GRNs) (Karlebach and Shamir, 2008).
Computational and mathematical modeling provide a means to assemble the known relevant molecules and their interactions into a network of pathways, with cross-talk between them. This allows, for examples, the test of whether the assimilated knowledge is sufficient to reproduce experimental results, and, furthermore, introduce cell-specific perturbations into the network to generate and test hypotheses in silico. For recent reviews, see Eftimie et al. (2016), Chakraborty (2017).
Computational models of GRNs have been shown to be a good approach to study how cells integrate several signals driving the cell phenotypic changes, especially for their ability to quantitatively and qualitatively describe a great variety of poorly characterized biological situations (Méndez and Mendoza, 2016). Computational models are used to describe immunological phenomena, to provide a better understanding of aspects of the immune response, and to produce outcomes coherent with available data, thus unraveling basic mechanisms of immunology and possibly leading to new hypotheses that can be tested experimentally in vivo or in vitro (Castiglione and Celada, 2015).
Discrete logical (Boolean or multi-state) models are usually the method of choice especially when the biological questions are of qualitative nature or when the available data (and knowledge) are mainly qualitative. Boolean networks and logical models have been used extensively to model many biological systems including immunological systems such as T-cell signaling and T helper cell differentiation (Naldi et al., 2010; Abou-Jaoudé et al., 2016; Méndez and Mendoza, 2016).
There are several computational models of some pathways that are involved in the pro and anti-inflammatory immune response, such as the NF-κB, TNF-α, IL-1, and IL-10 signaling pathways. Furthermore, there are computational models of T helper cell differentiation including continuous (Carbo et al., 2014), Boolean (Martinez-Sanchez et al., 2015), multistate logical (Naldi et al., 2010), and multi-scale (Santoni et al., 2008; Tieri et al., 2014). However, we are not aware of any GRN models of the molecular network describing macrophage differentiation. We have recently developed a multiscale model (Castiglione et al., 2016) of the immune response incorporating a minimalistic Boolean model of macrophages differentiation accounting for M1 and M2 polarization, but not for the subsets of M2. Maiti et al. (2014) presented an ODE model to describe the pro- and anti-inflammatory signaling in macrophages toward understanding immune homeostasis.
In this paper, we present a novel logical model of the gene regulation underlying macrophage differentiation and polarization, where the regulatory interactions and logical rules are inferred from the literature. We then used the model to study the dynamical behavior of the network. The model not only was able to reproduce known experimental data but also provides the first computational evidence of the continuum hypothesis of phenotypes which was suggested by Sica and Mantovani (2012).
Materials and Methods
Logical Models of Regulatory Networks
Gene regulatory network modeling aims at describing the way cells integrate extracellular stimuli to run cellular programs consisting of activations and inhibitions of genes (Kestler et al., 2008).
Logical network modeling was introduced by the geneticist R. Thomas (Thomas and D’Ari, 1990; Thomas and Kaufman, 2001) for the study of GRNs. Since then, they have been developed further, and have been used extensively to model many biological systems including cell-fate determination in A. thaliana (Espinosa-Soto et al., 2004; Benítez and Hejátko, 2013), E. coli metabolism (Samal and Jain, 2008), and the differentiation and plasticity of T helper cells (Naldi et al., 2010; Abou-Jaoudé et al., 2014), to name a few.
Gene regulatory networks are typically drawn from a mixture of literature, data mining and experimental data. Signal transducers, transcription factors and target genes in the activation of specific cellular programs (e.g., cell maturation or differentiation) are identified, as well as their relationships coded in terms of inhibition/activation. This data mining step produces a network (N, E) in which the nodes N are the molecules and the edges E = E- ∪E+ are the activations (edges in the set E+) and inhibitions (edges in E-) relationships. Gene activation levels (states) or molecular concentrations are represented either by a discrete and usually very small set of values (two levels, i.e., active/inactive, represents the most used one, called Boolean) or by a continuous range of activity levels. In this paper, we have used the discrete Boolean formulation.
Each node nk of the network N has a function Fk specifying how the state of that node may change in response to changes in the states of its neighbors (the nodes nj for which there exists an edge ejk ∈E) in the network. The synchronous or asynchronous calculation of the functions F1,…,Fn, at each discrete step makes the network evolve from one macro-state to another. In the synchronous mode, all node states are updated at the same time, while in an asynchronous case, nodes are randomly updated at different time steps.
The Boolean model of a GRN is therefore defined as a discrete dynamical system which can then be studied for its dynamical properties. Since the space of all possible macro-states is finite, starting from any configuration, the repeated application of the functions F1,…,Fn, will lead the system to be in states that it has reached before. These states correspond to stable patterns of gene expression that can be reasonably regarded as real biological states characterizing a specific cellular function. Starting from any configuration and after a certain transient period, the network dynamics will either reach a state and stay there (such a state is called a steady state), or can keep cycling forever among the same set of states (such a set of states is called a limit cycle) (Guevara, 2003; Ortiz-Gutiérrez et al., 2015). The transient period before the network dynamics reaches a certain steady state or limit cycle is called the basin of attraction of that state or cycle.
The dynamics of the system is encoded by a graph, whose vertices are all configurations (states) of the network and directed edges where each such edge indicates the transition of the system from one state to the next.
We used the software GINsim (Naldi et al., 2009) for the development of the model and the analysis of the network, including the identification of all steady states (Karlebach and Shamir, 2008; Méndez and Mendoza, 2016), and the BooleanNet Python library (Albert et al., 2008) and BoolNet R library (Müssel et al., 2010) for the study of the dynamics of the system.
Results
Molecular Basis of the Macrophage Polarization
During the inflammation process, several immune cells are involved in initiating and maintaining the inflammatory state. Macrophages, together with leukocytes, are the first cells recruited to the inflammation site. They start releasing pro-inflammatory cytokines (mostly IFN-γ and IL-1β), creating an inflammatory environment. The binding of those molecules to their specific receptors triggers a signal transduction cascade resulting in the release of other inflammatory molecules. This positive feedback mechanism allows the maintenance of the inflammatory state and reinforce the M1 polarized state.
The resolution of inflammation occurs by different mechanisms, such as the downregulation of pro-inflammatory molecules, the short half-life of the inflammatory mediators, and the production of anti-inflammatory molecules. In this context, macrophages are expected to switch to M2, and, consequently, produce anti-inflammatory mediators, such as IL-10, inhibiting M1-related transcriptional regulators, while a positive feedback loop provides the means to maintain their anti-inflammatory phenotype.
Interferon (IFN) receptors have multi-chain structures and interact with members of the Janus-activated kinase (JAK) family (Darnell et al., 1994). When IFN-γ binds to its cognate receptor, the activation of the receptor-associated JAKs occurs in response to rearrangement and dimerization of the receptor subunits, followed by auto-phosphorylation and activation of the associated JAKs. This process determines the activation of classical JAK–STAT (signal transducer and activator of transcription) signaling pathways, resulting in the transcription of target genes (Platanias, 2005; Mosser and Edwards, 2008; McLaren and Ramji, 2009). Among the STATs, a pivotal role is played by STAT1, which undergoes dimerization after its JAK-mediated tyrosine phosphorylation. Hence, STAT1–STAT1 homodimer binds to cis elements known as “gamma-activated sequences” (GAS) in the promoters of the genes encoding NOS2, the MHC class II transactivator (CIITA) and IL-12, among others (Darnell et al., 1994; Sadler and Williams, 2008; Lawrence and Natoli, 2011). The IFN-associated JAK/STAT pathway exerts its function in the regulation of several immune cells, including macrophages, with a great increase of IFN production, the synthesis of several cytokines, such as interleukins IL-1β, IL-6, IL-12, IL-18, IL-23, and TNF-α, and nitric oxide (NO), as well as reactive oxide intermediates (ROI) and enzymes required for tissue remodeling.
Toll-like receptors (TLRs) mediate the immune response to a great variety of infectious agents and facilitate transcription of many pro-inflammatory genes (Sheikh et al., 2014). LPS is a component of the Gram-negative bacteria cell wall and induces expression of a wide variety of genes that constitute the innate immune response to bacterial infections. LPS signals through TLR4 on the cell surface of many cell types, including macrophages (Kawai and Akira, 2010, 2011). Signaling through TLR4 induces rapid activation of two distinct intracellular signaling pathways: one is the MyD88-dependent pathway, which leads the cascade through interferon regulatory factor (IRF)-3, and the other is the MyD88-independent signaling pathway, which acts through TIR-domain-containing adapter-inducing interferon β (TRIF). These pathways converge to activate the transcription of NOS2; the inducible NO synthase (Kawai et al., 2001; Doyle et al., 2002).
The M1 phenotype can also result from differentiation in the presence of GM-CSF, with increased expression of IL-12 and pro-inflammatory cytokines, the ability to activate Th1 cell immune responses and decreased expression of IL-10 (Krausgruber et al., 2011).
M2 macrophages exhibit a functionally distinct phenotype to that of M1s, originally via the ability of IL-4 to induce MR expression, followed by IL-13, which is another Th2 cytokine. IL-4/IL-13 and TGFβ/IL-10 have been described to be associated with priming M2 macrophage subsets (M2a and M2c, respectively). The role of IL-4- and IL-13-mediated signaling in M2 macrophage polarization has been well established both in vitro and in vivo (Gordon, 2003; Martinez et al., 2009; Gordon and Martinez, 2010). Mice with a myeloid cell-specific knockout of IL-4 receptor-α (IL4Rα) were found to lack M2 macrophage development in mouse models of helminth infection and in Th2 cell-mediated inflammation, where IL-4 has a major role (Lawrence and Natoli, 2011). It is well established that IL-4 and IL-13 are associated with Th2-type responses, which have well-defined effects on macrophages, other cells and immune functions. IL-4 and IL-13 are produced particularly in allergic, cellular, and humoral responses to parasitic and extracellular pathogens. IL-4 and IL-13 upregulate expression of the MR and MHC class II molecules by macrophages, which stimulates endocytosis and antigen presentation, and they induce the expression of selective chemokines (Gordon, 2003; Gordon and Martinez, 2010). IL-4 and IL-13 act through a common receptor chain – IL-4Rα – through signal transducer and activator of transcription 6 (STAT6).
Interleukin-1 beta and IC, together with TLR4-signaling inducers (i.e., LPS), drive the macrophage to an M2b phenotype. IL-1β not only plays a pivotal role in the initiation and maintenance of the inflammatory response but also modulates immunosuppressive mechanisms through the process of macrophages endotoxin tolerance. IL-1β is also produced in response to LPS, emphasizing a collaborative interplay between M1 and M2b macrophages in eliciting and maintaining the inflammatory response (Sato et al., 2012).
Interleukin-10 acts on a distinct plasma membrane receptor to those for IL-4 and IL-13 (Riley et al., 1999; Moore et al., 2001; Deng et al., 2012), and its effects on macrophage gene expression are different, involving a more profound inhibition of a range of antigen-presenting and effector functions, together with the activation of selected genes or functions. T cells themselves are more heterogeneous than was thought originally, including not only Th0-, Th1-, and Th2-type cells but also regulatory and possibly Th3-type cells, some of which secrete TGF-β and IL-10 (Gordon, 2003). TGFβ and IL-10 have been described to be associated with priming M2-like macrophages subset polarization. TGFβ and IL-10 modulate macrophage polarization and functional plasticity to that of an M2c subset which exhibits a characteristic cytokine phenotype of IL-10hi, IL-12lo, IL-23lo, and TGFβ+ which is associated with anti-inflammatory responses, scavenging, immune regulation, tissue repair, and tumor promotion. Both TGFβ and IL-10 directly suppress immune activation via the down-regulation of the expression of MHC II and pro-inflammatory cytokine production, with an indirect effect through cross-regulation of M1-derived cytokines and functionality (Gordon and Martinez, 2010; Lawrence and Natoli, 2011; Sica and Mantovani, 2012). IL-10 is a potent STAT3-dependent inhibitor of pro-inflammatory cytokine production and NO release, after challenge with LPS. IL-10-deficient mice develop widespread inflammatory cell infiltrates, including in the bowel, and transgenic animals that constitutively overexpress IL-metricconverterProductID10 in10 in macrophages suffer from septic shock and over-activity of pro-inflammatory cytokines (Lang et al., 2002b). The upregulation of expression of IL-4Rα by IL-10 correlates with increased IL-4-dependent expression of arginase-1. IL-10 also synergizes with LPS to increase the expression of arginase-2. Therefore, IL-10 increases the total level of arginases in macrophages in many ways (Lang et al., 2002a,b).
Phenotypes depending on complex regulatory logic can be effectively studied by using mathematical and computational approaches, such as GRN models.
A Logical Network Model of Macrophage Differentiation
We have constructed a logical regulatory network model (Figure 2 and Supplementary File S1) that describes macrophage polarization using experimental data and knowledge derived from literature (see Table 2) and a curated database of causal relationships between biological entities (Perfetto et al., 2016). The network comprises 30 nodes and 49 interactions among them. Interactions can be either positive (activations) or negative (inhibitions) (Figure 2). Table 2 shows a list of the molecules, interactions, and references from the literature supporting each interaction, while Table 3 shows logical rules for each molecule.
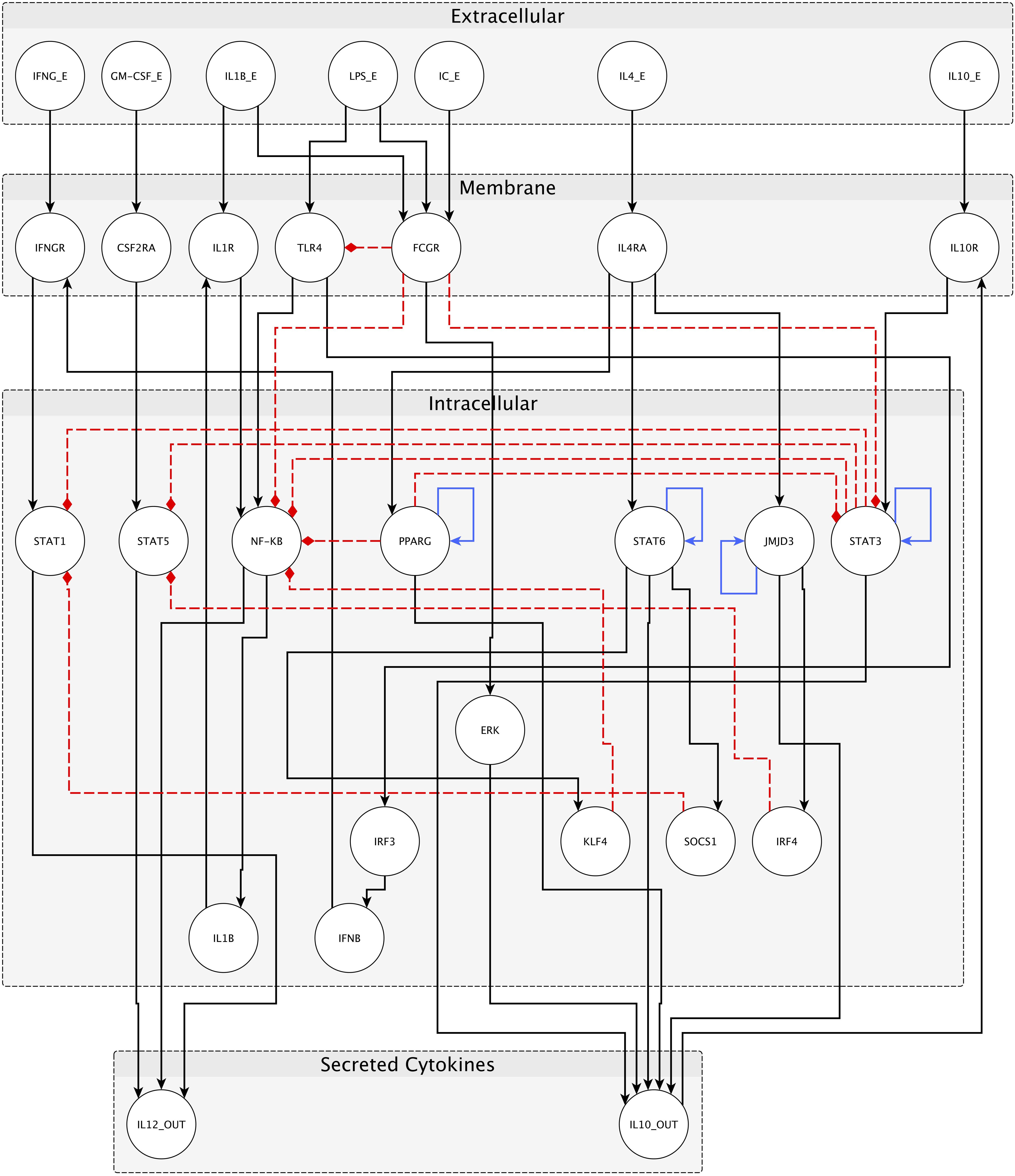
FIGURE 2. Network for macrophage polarization. External stimuli are reported in the extracellular space, receptors inside membrane space, and internal transducers/transcription factors in the intracellular space. Secreted cytokines (IL-10 and IL12) are also reported. Black arrows represent positive interactions (activations), red dashed arrows are negative interactions (inhibitions), and blue arrows are transcriptional auto-regulatory loops. Nodes represent both genes and proteins; edges represent both protein–protein interactions and transcriptional regulations.
Nodes are of four kinds, depending on cellular location and function (Figure 2): seven input nodes, which represent the extracellular stimuli (IFNγ, GM-CSF, IL-1β, LPS, IC, IL-4, and IL-10), seven receptors (IFNγR, CSF2Ra, IL-1R, TLR4, FcγR, IL-4R, and IL-10R), 14 internal regulators (STAT1, STAT5, NF-κB, PPARγ, STAT6, JMJD3, STAT3, IRF3, ERK, KLF4, SOCS1, IRF4, IL1β, and IFN-β), and two main products of each distinct type of macrophage (IL-12 and IL-10). The input nodes represent the main intercellular molecular stimuli that drive macrophage polarization, as reported in the literature. Each external molecule (input) is connected to its specific receptor, and this binding elicits a signaling cascade, involving intracellular transducers and transcription factors (mostly STAT factors). Each specific transcription factor binds the promoter of a target gene, resulting in the production of IL12 or IL10 depending on the macrophage polarized form.
Interactions among nodes are derived from experimental data available in the literature as shown in Table 2. All interactions have been deposited in SIGNOR (Perfetto et al., 2016), a public database of causal interactions between biological entities. Each node is associated to a logical function which determines the activation level of the node based on the activation levels reached by its source nodes in the previous time step. The logical function of each node is inferred from the available literature (see Table 3).
The network encompasses several pathways. Different cell fates, i.e., macrophage phenotypes, are defined by steady or stable states (also called fixed point attractors) of gene expression, and described in this dynamic model as multiple, specific, and stable configurations of activated/deactivated nodes. In other words, stable states are configurations toward which the system tends to evolve, for a wide range of starting conditions. Thus, according to the network, its starting configuration, and the initial external stimuli, the pathways lead to a configuration that resembles a specific cell state in terms of the given gene expression pattern. In this regard, we assumed that the sum of the sizes of the basins of attraction of the steady states characterizes the likelihood of finding the cell in a specific differentiation state. In other words, the probability that the cell, stimulated by cytokines, will switch to the certain differentiation state is proportional to the size of the subspace of all network configurations eventually reached by the network dynamics.
Inhibitory pathways among M1 and M2 phenotype-related transcription factors are particularly interesting, because they allow a mutual exclusivity of transcription factors and, therefore, of the macrophage phenotypes, as reported in literature (Lawrence and Natoli, 2011). Notably, among the interactions describing the network and reported in tables above, the inhibition of TLR4 and NF-κB signaling by FcγR activation were added. These relationships allow the inhibition of M1 polarization in the presence of IC, that together with LPS and IL-1β, drives the otherwise absent M2b polarization.
To analyze the dynamics of the network under different conditions we used GINsim [Gene Interaction Network simulation1; (Chaouiya et al., 2012)], a software tool for modeling and simulation of genetic regulatory networks (Chaouiya et al., 2012). In some cases, for further confirmation or additional details, we used the BooleanNet Python (Albert et al., 2008) as well as the BoolNet R library (Müssel et al., 2010).
The fate of a macrophage strongly depends on the local biochemical microenvironment. To reproduce these different microenvironments that influence the cells, we defined a set of inputs to run the simulations. Hence, we could discriminate among steady states with a real biological meaning.
The starting expression state of the network corresponds to the naïve macrophage M0 (unstimulated/not-activated) phenotype, in which the state of each node in the network is set to “0” (i.e., low expression).
In our simulations, we found that our model has five sets of steady states fitting the following five specific macrophage phenotypes markers according to literature (Figure 3):
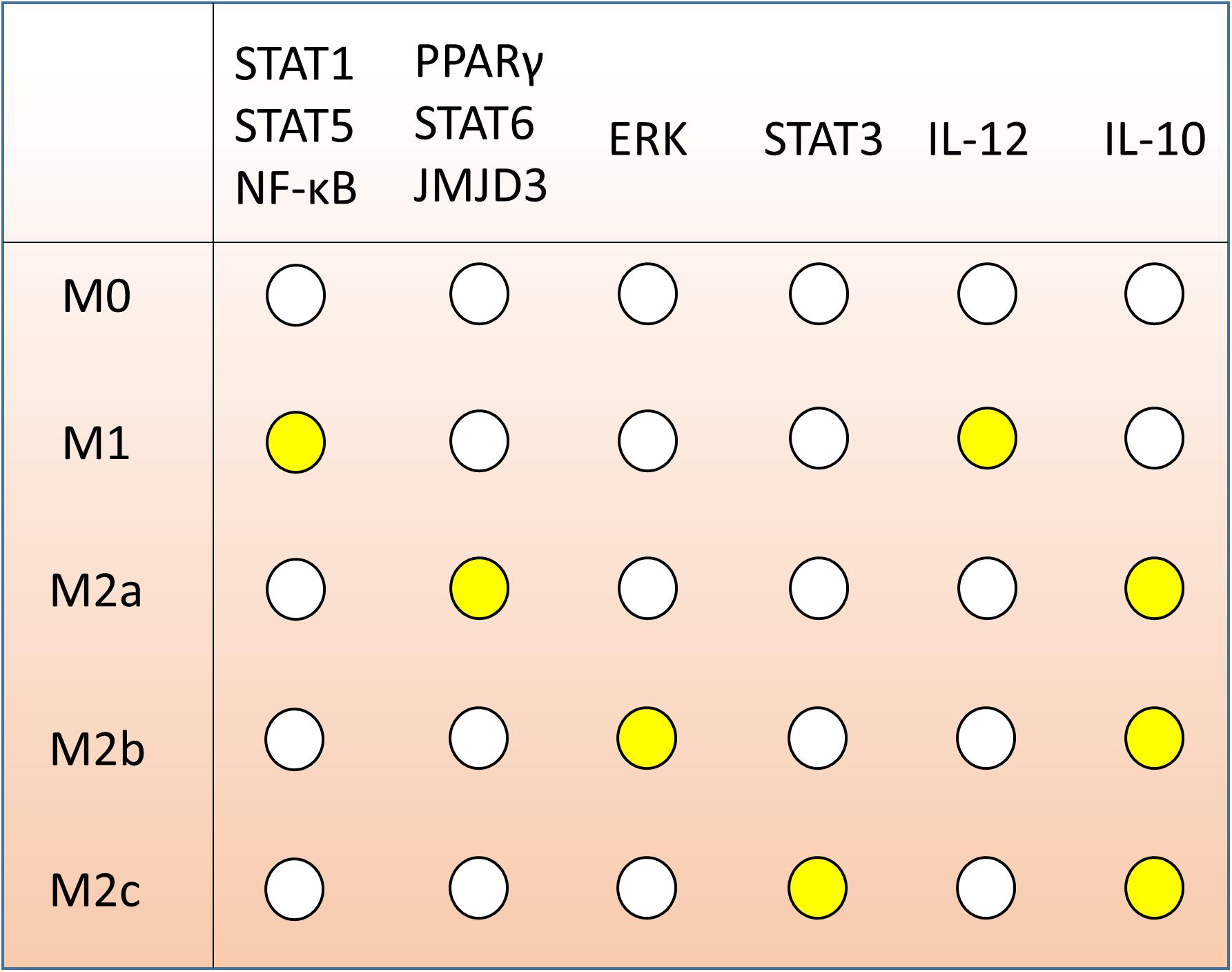
FIGURE 3. Gene expression markers of macrophage polarization according to literature. Each row, associated to one of M0, M1, M2a, M2b, and M2c, indicates the expression of the 10 marker genes determining the polarization fate. White dots represent inactive genes; yellow dots indicate expressed genes.
1. M0: no nodes active;
2. M1: IL-12 and at least one among STAT1, STAT5 or NF-κB are active;
3. M2a: all of PPARγ, STAT6, JMJD3 and IL-10 are active;
4. M2b: ERK and IL-10 are active; and
5. M2c: STAT3 and IL-10 are active.
We computed the steady states of macrophage polarization network using a synchronous update. The system reached 1056 states, 1040 of which are steady states and 16 are cycles made of three different states. Among the 1040 unique steady states (Supplementary Table S1), 228 can be mapped to the five canonical macrophage phenotypes reported via experimental studies in the literature. The frequencies of these 228 steady states are reported in Figure 4. The remaining steady states do not characterize the macrophage in any of the known canonical phenotypes. These states, for which there is a lack of experimental knowledge, could correspond to input conditions not existing among in vivo inflammation settings or even be artefacts of the modeling approach. Alternatively, they could correspond to hybrid phenotypes (O’Carroll et al., 2013) resembling gene expression patterns of two or more canonical phenotypes (discussed below). It is worth to note that a higher number of steady states does not imply a corresponding higher probability of polarization, since the final outcome depends on the combination of external stimuli. In other words, the number of steady states indicates the propensity of the network logic to lead the cell to the specific phenotypes yet driven by environmental cues.
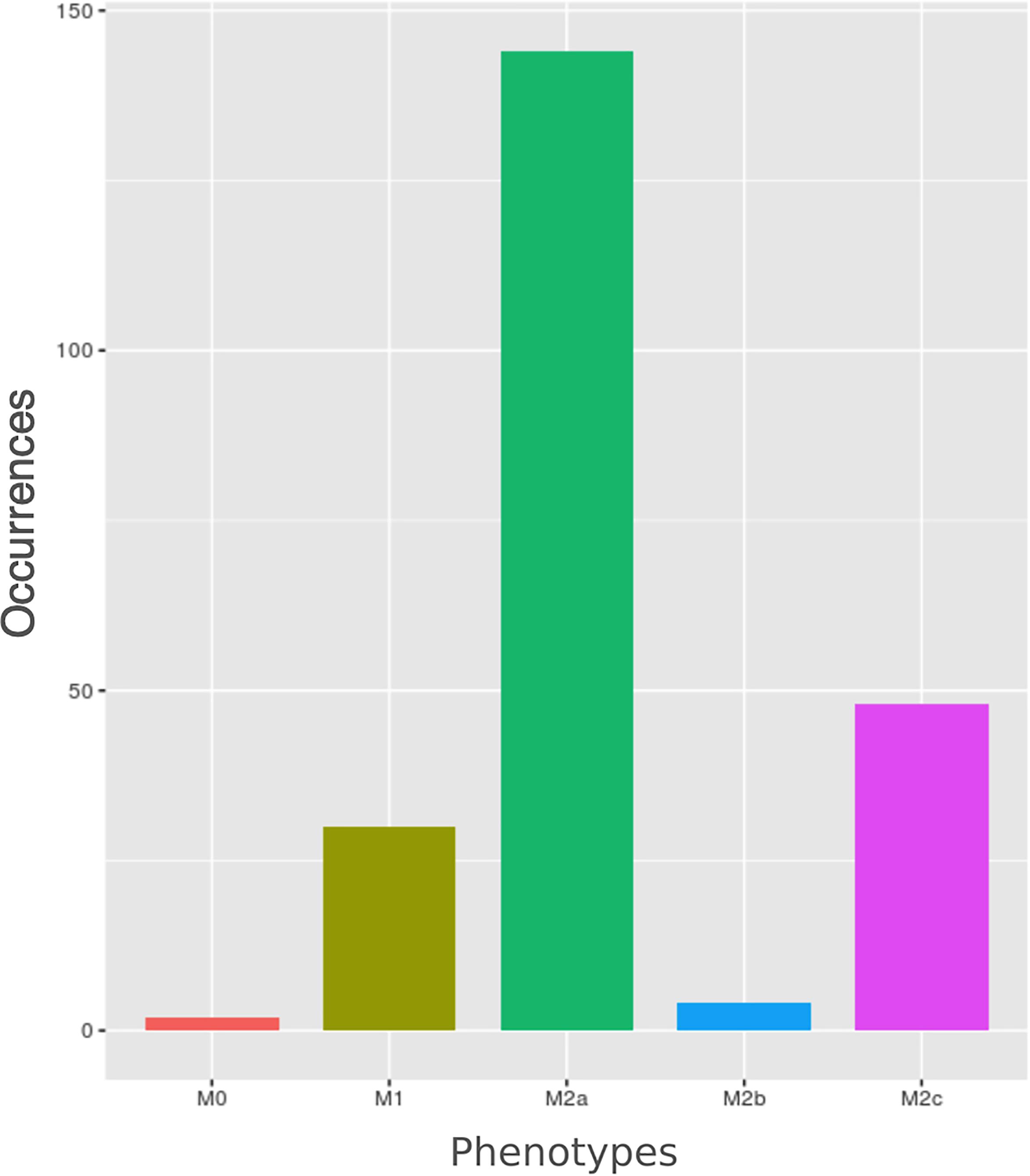
FIGURE 4. Barplot of macrophages’ phenotypes occurrences. Each bar represents the number of steady states (total number = 228) related to a specific polarized form.
The most frequent polarized state is the M2a followed by M2c and then M1. This is consistent with the pivotal role of macrophages in inflammation (M1), and in the resolution of inflammation (M2a and M2c). On the other hand, according to our analysis, M2b is the least frequent state, which might be consistent with the lack of knowledge of M2b-related pathways which is reflected in the network. This behavior of the model is consistent with observed data (Sica and Mantovani, 2012).
A closer look at the dynamics of the model (Figure 5) is obtained by performing several rounds of asynchronous simulations by using the BooleanNet Python library. We observed that any combination of stimuli among IFN-γ, IL-1β, LPS, and GM-CSF keep the polarization of the M1 macrophage. Once macrophages have polarized into an M1 form, the steady states are taken as initial conditions to polarize macrophages into the three different forms of M2 macrophage. IL-4 input is activated (i.e., IL-4 binding by IL-4RA) to polarize M2a macrophage, IL-10 is activated to polarize the M2c macrophages, and IC in combination with either IL-1β or LPS is activated to polarize M2b macrophages, according to the available literature on macrophage polarization stimuli (Gordon and Martinez, 2010).
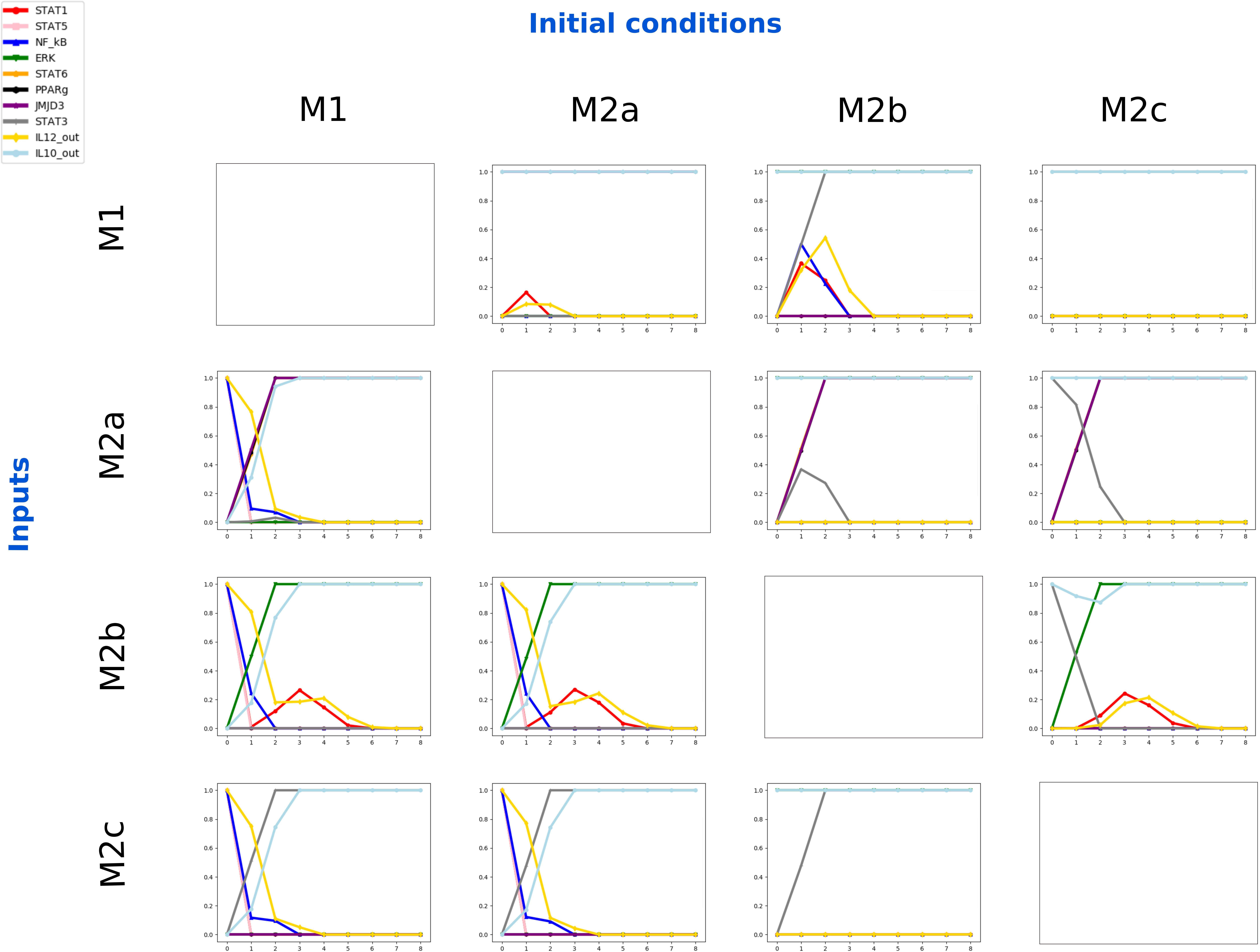
FIGURE 5. Dynamics of the gene activation levels obtained for all combinations of initial polarization state and polarizing stimuli. The average activation values are computed over 104 asynchronous simulations of the activation level of the genes. For each subplot, the horizontal axis represents eight time steps and the vertical axis the average activity of a molecule from 0 to 1.
The M1 polarization is simulated starting from an M0 (i.e., all non-input signal nodes set to zero) cellular environment and switching on all input nodes, as reported in literature. Following the typical cellular response to inflammation, starting from an M1-like configuration, and M2-related external stimuli (i.e., IL-4 for M2a, IL-10 for M2c and IC in combination with LPS or IL-1β for M2b macrophages), the dynamics of transcription factors and secreted molecules (i.e., IL-12 and IL-10) show the macrophage moves from pro- to anti-inflammatory states, as reported in literature. The M2-related polarizations from an M0 initial state have been also performed to check the ability of the system to simulate the situation in which new monocyte-derived macrophage populations are recruited to the inflammation site during the resolution of inflammation, in addition to M2 macrophages polarized from the pro-inflammatory M1 state (see Supplementary File S2).
We also tested in silico the “plasticity” of the polarized phenotypes, i.e., the capability to revert the state from inflammatory to anti-inflammatory and vice versa. In order to proceed, we run a set of numerical experiments in which macrophages, starting from the four polarized states M1, M2a, M2b, and M2c, were challenged with the four characteristic stimuli (i.e., pro-M1, -M2a, -M2b, and -M2c) resulting in 16 possible couples “initial condition/stimuli.” Each of those simulation settings was repeated 104 times using the asynchronous updating scheme and averages were computed. After that, we used the steady states obtained as initial states for other simulations, giving each input from the input set (see Figure 5).
We focused on M1-related initial states, since a normal immune response begins with an inflammation state, followed by anti-inflammatory environment settings.
With an M0 steady state as initial condition, several stimuli were applied for each simulation. To represent the M1 polarization we gave a combination of random M1-related stimuli (LPS, GM-CSF, IFN-γ, and IL-1β). The initial state for each node of the network are those related to the M0 steady state (no active nodes at all).
We then performed M2a, M2b, and M2c polarizations with IL-4, a combination of IC and IL-1β or LPS, and IL-10 as inputs, respectively. In other words, we started with M1 macrophages, changed their environment and stimulated them with different types of stimuli. Thus, we performed all the combinations for the simulations and analyzed the dynamics and the differences (see Figure 5 for details). We also investigated the possibility of transforming an M2-like phenotype to an M1 macrophage by changing the environment using a variety of external stimuli. However, all considered combinations resulted in states that do not characterize the macrophage M1 canonical phenotype.
Robustness Evaluation of the Macrophage Network
Biological networks are considered to be robust when compared to random networks, if a single perturbation does not influence the behavior of the entire system. We analyzed the robustness of macrophage polarization network as follows. First, we evaluated the transition robustness by perturbing states of the network with random bit flips (Müssel et al., 2010). When the successor states of the original and the perturbed states are computed, the distance between then is calculated as the Hamming distance (HD, that is, the difference between strings of equal length is the number of positions at which the corresponding symbols are different). The HD, normalized by the number of genes in the network, shows how robust the network is to small mutations: the lower the normalized HD, the more robust is the network.
A hundred of these tests were repeated for 100 randomly generated networks and the results plotted in Figure 6. Results show that the macrophage model is statistically more robust (p = 0.01) in comparison to the randomly generated networks. The resulting mean normalized HD equal to 0.03 can be interpreted as if, on average in the mutated networks, 3% of the gene states are different.
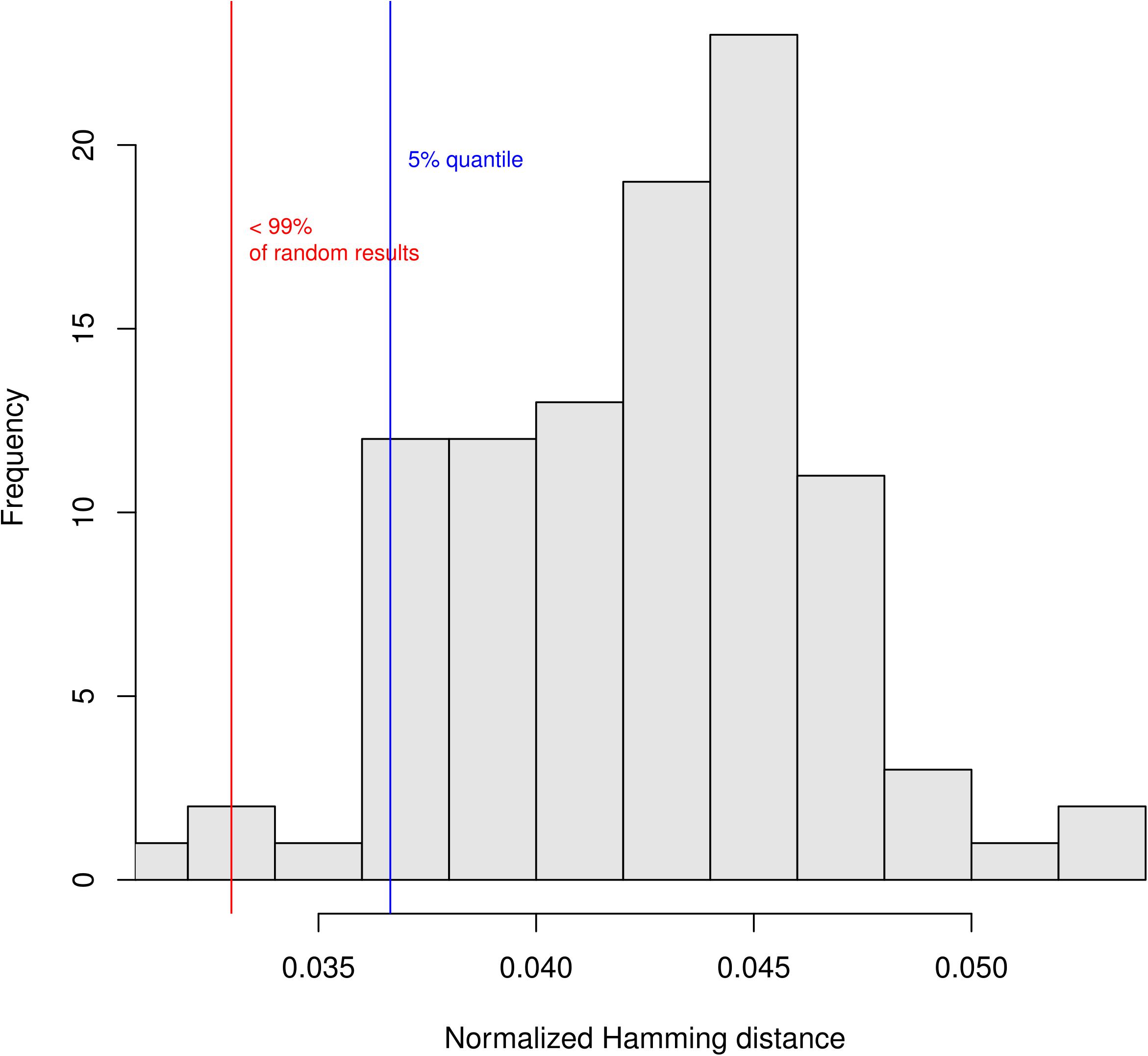
FIGURE 6. Test of the robustness of the macrophage network. Histogram of the normalized Hamming distance (HD) of randomly generated networks (RGN) in comparison to the HD of the perturbed macrophage network (PMN). The red line shows the mean of the PMN-HD histogram (not shown) which is smaller than the 5% quantile of the RGN-HD distribution (blue line). The test shows that the noise influences the randomly generated networks significantly more than the macrophage network (Müssel et al., 2010).
Effects of Knockouts in the Simulations
To analyze the dynamics and investigate the role of each component in the polarization process, we performed knockout (components’ value set to “0”) and ectopic expression (components’ value set to “1”) in silico experiments. These constraints allowed us to see how perturbations of the system affect the network functionality with respect to the macrophage behavior. At a biological level, this analysis may have potential impact in in-silico pharmaceutical target prioritization.
In our network, gene knockout is interpreted as a deactivation of one or more components, just like the deactivation of a protein that is a target of a drug.
We performed systematic knockouts on every internal node of the network (internal transducers/transcription factors), to see how they affect the dynamics of the network by calculating the fold change of the number of steady states reached by the system (see Figure 7 and Supplementary Files S3, S4 for details). The idea is that a knockout modifies the network characteristics so that also its dynamics is modified and the number of steady states, for example, a higher number of pro-inflammatory steady states is interpreted as a greater probability to induce, via that specific knockout, a pro-inflammatory polarization of the macrophages.
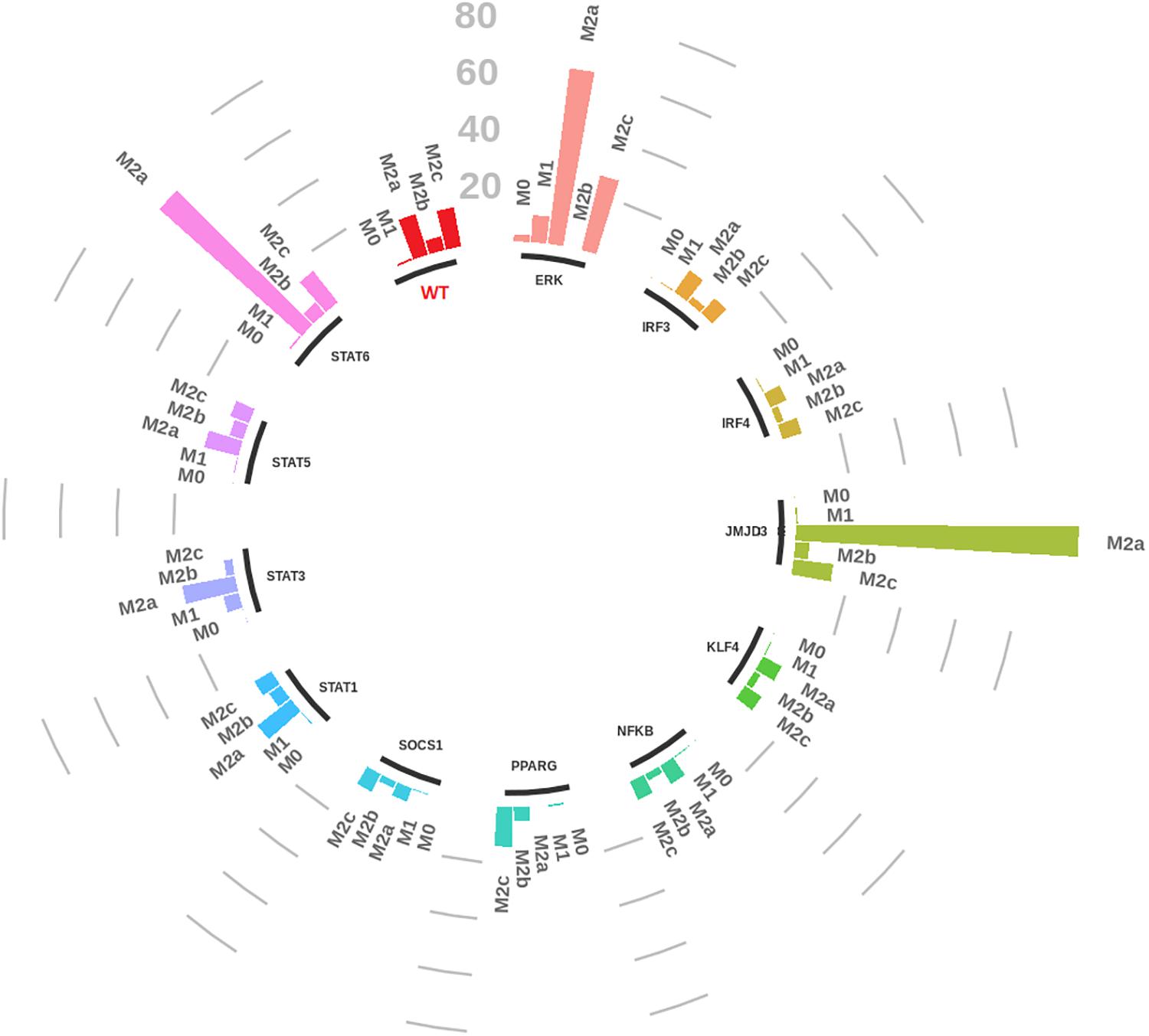
FIGURE 7. Circular bar plot of macrophage gene knockouts. Each group represents the knockout of a specific transcription factor of the network. Bar heights represent the number of steady states for each macrophage canonical phenotype with respect to the wild type (WT in red).
Discussion
Pro-inflammatory macrophages are those polarized by cytokines like IFN-γ or LPS (among other molecules). They are produced during cell-mediated immune responses, interacting with chemical mediators produced by other cells, such as the IFN-γ secreted by natural killer (NK) cells (Mosser and Edwards, 2008). Resting macrophages are primed by IFN-γ to produce pro-inflammatory cytokines, according to our simulations of an unstimulated macrophage which undergoes an M1 polarization when stimulated by IFN-γ (see Figure 5). TLR ligands, such as the well-known LPS can also polarize macrophage into an M1 form, via NF-κB signaling, producing pro-inflammatory mediators, other stimuli such as GM-CSF and IL-1β gave similar results (Mosser and Edwards, 2008; Lawrence and Natoli, 2011; Sica and Mantovani, 2012). Macrophages respond to micro-environmental cues, showing a distinct transcriptional profile depending on the stimulus. Starting from M0, that is assumed to be a cell with no typical constitutive gene expression profile, an M1 stimulus (i.e., IFN-γ, LPS, IL-1β, and GM-CSF) leads to a M1 phenotype, IL4 to a M2a phenotype, IC together with LPS and/or IL-1β to an M2b phenotype, and IL-10 to a M2c phenotype, the network can represent the polarization process (see Figure 8 for a visual representation of macrophage switch pathways).
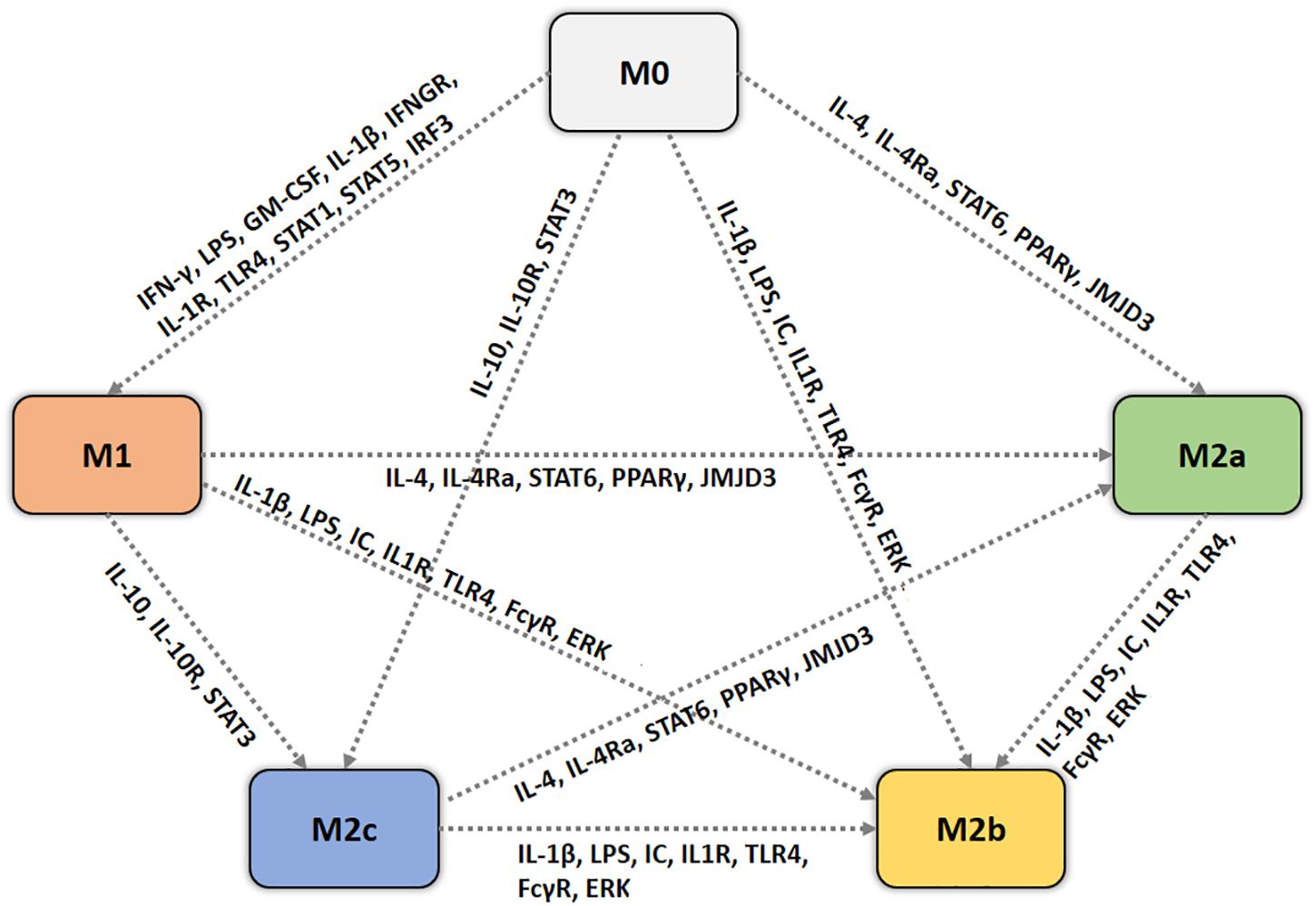
FIGURE 8. Cell fate map for macrophages. Each dotted arrow represents the switch of macrophage from a phenotype to another, annotated with the gene expression patterns, based on simulation dynamics and results.
Transcription factor NF-κB is among the most important regulators of M1 polarization of macrophages (Wang et al., 2014). Its expression is stable and maintained during macrophage polarization after stimulation with M1-related inputs. If no inputs are given to an M1-polarized system, NF-κB seems to maintain the M1 polarization (see Figure 5), while STAT1 and STAT5 decrease their expression (if not stimulated by IFN-γ and GM-CSF), until an M2-related stimulus (IL-4, IL-10 or IC) is present, which result in the resolution of the inflammation phase, and in the increase of the expression of M2 master regulators.
In the presence of IL-4 (i.e., activation of input node IL4), we noticed rapid expression of M2a master regulators (i.e., STAT6, PPARγ, and JMJD3) and the production of IL-10, with a slow decrease in the production of IL-12, indicating that M2a-related stimuli can immediately suppress the pro-inflammatory function of macrophage, as already evidenced in literature (Sica and Mantovani, 2012). In M2b polarization, despite the slow decrease of the expression of pro-inflammatory transcription factors and secreted molecules, IL-10 is finally produced by this type of macrophage, and its master regulator, ERK. M2c polarization is reached when IL-10 is given as input, with IL-10 production and STAT3 expression.
In the absence of external stimuli, a polarized M2 macrophage maintained its state with no alteration on the molecules expression, highlighting the stability of this phenotype.
M1 stimuli do not affect M2-like macrophage, apart from M2b in which we can assist to a slower decrease of IL12, reaching its stable state at the seventh time step, at variance with M2a and M2c simulations in which the anti-inflammatory stimuli lead to the absence of IL12 at the fourth time step. For any input given to an M2b-polarized macrophage, a phenotype change related to the given stimulus seems to be a common feature, except for M1 stimuli, which appear to polarize macrophage to a form corresponding to the production of both output cytokines (IL12 and IL10) and the repression of ERK. This behavior has not been reported in literature, but could explain the existence of this not-well characterized type of macrophage that share common features between pro- and anti-inflammatory macrophages (Sica and Mantovani, 2012).
A similar behavior can be observed when M2c macrophage are polarized with M1-related cytokines, even though M2a and M2b stimulations can subvert M2c polarization, indicating that M2c macrophages are more likely to be polarized from an M0 phenotype or switch from an already M1-polarized macrophage. Indeed, in some physiological and pathological conditions, such as muscle regeneration, the co-existence of different populations of M2 macrophages can be found at later stages, comprising M2a and M2c macrophage (Novak et al., 2014; Rigamonti et al., 2014). Hence, they can be thought of as distinct populations of macrophage polarized independently, since this regulatory network is characterized by well-known interactions between molecules involved in the polarization pathway (Novak et al., 2014; Rigamonti et al., 2014).
Conclusion
Transforming acute diseases into chronic ones is a realistic strategy for those pathologies for which no definitive cure is known, such as in the case of HIV (Scandlyn, 2000). A better understanding of the pathways involved in the transition from acute to chronic states and a more comprehensive knowledge of the cellular and molecular mechanisms are in need. Understanding how the immune response is regulated, and how immune cells integrate information from the multitude of molecular signals could certainly lead to improvements of existing therapies and make suggestions on the way forward.
In this work, we presented a dynamic logical model of the GRN of macrophage polarization, which is coherent to the expected behavior, under different experimental conditions. The model identified mechanisms driving a pro- into an anti-inflammatory setting, and hence maybe useful in transforming, fully or in part, an acute inflammation into a chronic one.
One example of network dynamics that could be affected by providing different types of stimuli is reported in Figure 8. We examined the different dynamics of this process to study how macrophages switch their phenotype during ineffective and sterile immune responses, focusing on M2-like polarization from a pro-inflammatory micro-environment.
A first result regards the importance of two inhibitions, namely, of TLR4 and NF-κB signaling by FcγR, that turned out essential to obtain the M2b phenotype. In fact, a preliminary version of the network, not accounting for these two inhibitions, was not able to reach the M2b polarized state.
The repolarization from M2 to M1 has been experimentally observed, yet occasionally in specific environments (Davis et al., 2013; Zheng et al., 2013; Zhang et al., 2017; Gao et al., 2018). Simulation results suggest that such polarization reversion seems to show a higher inertia. In fact, as shown in Figure 5 panels a, b, and c, the average values of pro-inflammatory genes starting from an anti-inflammatory phenotype only reach the value of 30% of the activation level. Furthermore, our in silico knockout experiments evidenced how some regulator plays a role by downregulating genes that are known for their inhibition activity. For instance, in M2-related knockouts in silico experiments, such regulators, as for example PPARG, are responsible for the resolution of inflammation and the maintenance of an anti-inflammatory environment by enabling the production of IL-10 and other important anti-inflammatory mediators. Similar studies could focus on networks that are specific to some pathogen or some physiological mechanism, to get a better comprehension in terms of the logic of the regulatory machinery.
This modeling study yielded another important observation, which is related to the environmental-dependent expression of mixed markers identifying one of the four canonical macrophage polarizations. Indeed, recent studies support the view that fully polarized macrophages (M1 and M2) as being the extremes of a continuum of macrophages polarization (Mantovani, 2008). This could for example be obtained by mixing various stimuli, such as IC together with LPS or IL-1β and IL-10, which give rise to M2-like functional phenotypes, yet sharing properties with IL-4-activated macrophages (Mantovani et al., 2004). This continuum of macrophages phenotypes parallels a continuum in CD4+ T cell states, recently observed, as opposed to a limited number of discrete phenotypes (Eizenberg-Magar et al., 2017). Indeed, while T helper cell induction requires the participation of macrophages, several signal feedback mechanisms are implemented for the activation and differentiation of macrophages. Even if this intertwinement may vary in both quantitative and qualitative aspects, the continuum of states detected in T helper and macrophage cells may be more linked than observed up to now.
We surmise that shifts among different phenotypes in our model mimic the hypothetical continuum of macrophage polarization, being M1 and the three subtypes of M2 the extremes of such uninterrupted sequences of states. Figure 9 conceptualizes this continuum in the progression of gene activations leading from one form of polarization to another driven by various stimuli. For instance, an M1+M2 successive stimuli can lead to an M2a stable configuration while passing through an M1 state (see Figure 9).
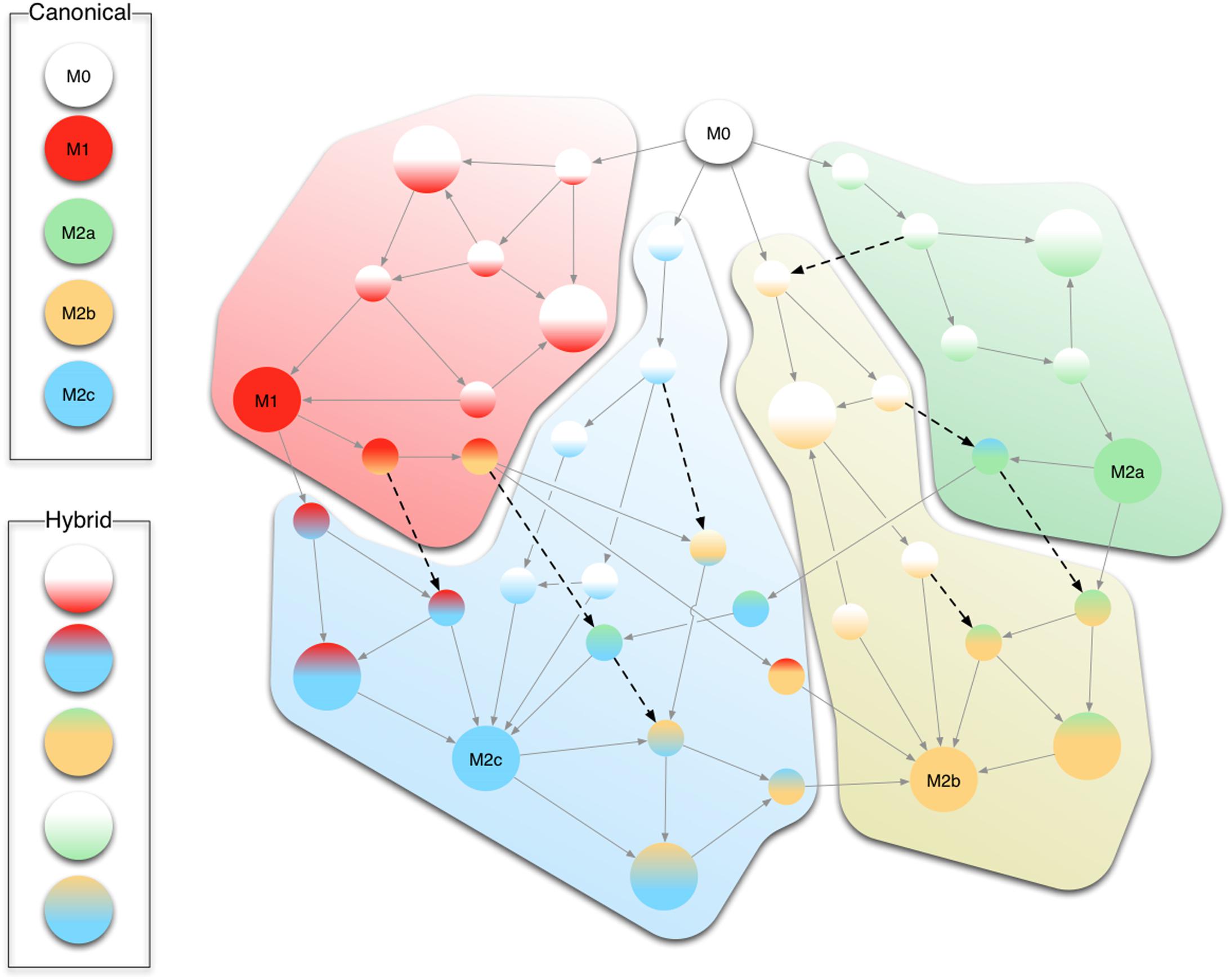
FIGURE 9. Conceptual representation of the continuum of differentiation states. Circles show intermediate stable states (smaller circles) between the five canonical M0, M1, and M2a/b/c (larger monochromatic circles). Stable states whose correspondent phenotype is not uniquely determined are indicated as larger circles with more than one color. Gray arrows indicate state changes the cell undergoes upon reception of extracellular stimuli. Black dashed arrows show jumps from one differentiation pathway to another. For instance, just by changing the extracellular stimuli (e.g., IL10) a macrophage which started the differentiation from M1 to M2b can divert toward the M2c phenotype.
The presented approach, although promising and general, is not free of pitfalls. Even if little mathematical knowledge is needed to build a Boolean network, the information gained from its analysis is strongly affected by the accuracy of the relationships among genes encoded in the Boolean rules characterizing the overall dynamics. Manually curated networks optimally convey the biological information but cannot ensure completeness. The usefulness of Boolean networks therefore is found while dealing with poorly characterized systems, especially when quantitative experimental data is missing. In some cases, alternative approaches should be considered such as introducing uncertainty with probabilistic networks or using continuous models that describe the kinetic with greater accuracy than Boolean networks.
To conclude, although there is a wealth of information about the different macrophage subsets in vitro, features such as plasticity, heterogeneity, and adaptability make them very difficult to study using conventional experimental tools. In this paper, we have shown that relatively simple logical description of the gene regulation machinery can support the analysis of the emerging complexity of the phenomena of mammalian cell differentiation and can be used to provide testable predictions as, for instance, which combination of stimuli leads to hybrid phenotypes.
The network provided here is manually curated and has been built based on the available information derived from literature to date. This should be considered as-is, that is, limited to the current knowledge which, regarding the less characterized pathways and molecular interactions leading to M2b macrophages, is admittedly lacking.
Author Contributions
All authors conceived the study. AP and FC performed the experiments. All authors carried out the analysis and contributed to writing the paper.
Conflict of Interest Statement
The authors declare that the research was conducted in the absence of any commercial or financial relationships that could be construed as a potential conflict of interest.
Acknowledgments
GC acknowledges partial support from the European Research Council (grant DEPTH, grant agreement no. 322749) and from the Italian Association for Cancer Research AIRC (grant IG 2017, StateplaceId. 20322). FC and PT acknowledge partial support from the European Commission under the Seventh Framework Programme (MISSION-T2D project, contract no. 600803), and from COST Action CA15120 Open Multiscale Systems Medicine (OpenMultiMed).
Supplementary Material
The Supplementary Material for this article can be found online at: https://www.frontiersin.org/articles/10.3389/fphys.2018.01659/full#supplementary-material
Footnotes
References
Abou-Jaoudé, W., Monteiro, P. T., Naldi, A., Grandclaudon, M., Soumelis, V., Chaouiya, C., et al. (2014). Model checking to assess T-helper cell plasticity. Front. Bioeng. Biotechnol. 2:86. doi: 10.3389/fbioe.2014.00086
Abou-Jaoudé, W., Traynard, P., Monteiro, P. T., Saez-Rodriguez, J., Helikar, T., Thieffry, D., et al. (2016). Logical modeling and dynamical analysis of cellular networks. Front. Genet. 7:94. doi: 10.3389/fgene.2016.00094
Abrahams, V. M., Cambridge, G., Lydyard, P. M., and Edwards, J. C. (2000). Induction of tumor necrosis factor alpha production by adhered human monocytes: a key role for Fcgamma receptor type IIIa in rheumatoid arthritis. Arthritis Rheum. 43, 608–616. doi: 10.1002/1529-0131(200003)43:3<608::AID-ANR18>3.0.CO;2-G
Albert, I., Thakar, J., Li, S., Zhang, R., and Albert, R. (2008). Boolean network simulations for life scientists. Source Code Biol. Med. 3:16. doi: 10.1186/1751-0473-3-16
Arnold, C. E., Whyte, C. S., Gordon, P., Barker, R. N., Rees, A. J., and Wilson, H. M. (2014). A critical role for suppressor of cytokine signalling 3 in promoting M1 macrophage activation and function in vitro and in vivo. Immunology 141, 96–110. doi: 10.1111/imm.12173
Baker, B. J., Akhtar, L. N., and Benveniste, E. N. (2009). SOCS1 and SOCS3 in the control of CNS immunity. Trends Immunol. 30, 392–400. doi: 10.1016/j.it.2009.07.001
Bally, A. P. R., Lu, P., Tang, Y., Austin, J. W., Scharer, C. D., Ahmed, R., et al. (2015). NF-κB regulates PD-1 expression in macrophages. J. Immunol. 194, 4545–4554. doi: 10.4049/jimmunol.1402550
Barahmand-Pour, F., Meinke, A., Groner, B., and Decker, T. (1998). Jak2-Stat5 interactions analyzed in yeast. J. Biol. Chem. 273, 12567–12575. doi: 10.1074/jbc.273.20.12567
Benítez, M., and Hejátko, J. (2013). Dynamics of cell-fate determination and patterning in the vascular bundles of Arabidopsis thaliana, Candela H, editor. PLoS One 8:e63108. doi: 10.1371/journal.pone.0063108
Biswas, S. K., Gangi, L., Paul, S., Schioppa, T., Saccani, A., Sironi, M., et al. (2006). A distinct and unique transcriptional program expressed by A distinct and unique transcriptional program expressed by tumor-associated macrophages (defective NF- B and enhanced IRF-3/STAT1 activation). Blood 107, 2112–2122. doi: 10.1182/blood-2005-01-0428
Biswas, S. K., and Mantovani, A. (2010). Macrophage plasticity and interaction with lymphocyte subsets: cancer as a paradigm. Nat. Immunol. 11, 889–896. doi: 10.1038/ni.1937
Bouhlel, M. A., Derudas, B., Rigamonti, E., Dièvart, R., Brozek, J., Haulon, S., et al. (2007). PPARγ activation primes human monocytes into alternative M2 macrophages with anti-inflammatory properties. Cell Metab. 6, 137–143. doi: 10.1016/j.cmet.2007.06.010
Bowdish, D. M. E., Loffredo, M. S., Mukhopadhyay, S., Mantovani, A., and Gordon, S. (2007). Macrophage receptors implicated in the “adaptive” form of innate immunity. Microbes Infect. 9, 1680–1687. doi: 10.1016/j.micinf.09.002
Carbo, A., Hontecillas, R., Andrew, T., Eden, K., Mei, Y., Hoops, S., et al. (2014). Computational modeling of heterogeneity and function of CD4+ T cells. Front. Cell Dev. Biol. 2:31. doi: 10.3389/fcell.2014.00031
Castiglione, F., and Celada, F. (2015). Immune System Modelling and Simulation. Boca Raton, FL: CRC Press. doi: 10.1201/b18274
Castiglione, F., Tieri, P., Palma, A., and Jarrah, A. S. (2016). Statistical ensemble of gene regulatory networks of macrophage differentiation. BMC Bioinform. 17:506. doi: 10.1186/s12859-016-1363-4
Chakraborty, A. K. (2017). A perspective on the role of computational models in immunology. Annu. Rev. Immunol. 35, 403–439. doi: 10.1146/annurev-immunol-041015-055325
Chaouiya, C., Naldi, A., and Thieffry, D. (2012). Logical modelling of gene regulatory networks with GINsim. Methods Mol. Biol. 804, 463–479. doi: 10.1007/978-1-61779-361-5_23
Chawla, A. (2010). Control of macrophage activation and function by PPARs. Circ. Res. 106, 1559–1569. doi: 10.1161/CIRCRESAHA.110.216523
Clatworthy, M. R., Harford, S. K., Mathews, R. J., and Smith, K. G. C. (2014). FcγRIIb inhibits immune complex-induced VEGF-A production and intranodal lymphangiogenesis. Proc. Natl. Acad. Sci. U.S.A. 111, 17971–17976. doi: 10.1073/pnas.1413915111
Darnell, J. E., Kerr, I. M., and Stark, G. R. (1994). Jak-STAT pathways and transcriptional activation in response to IFNs and other extracellular signaling proteins. Science 264, 1415–1421. doi: 10.1126/science.8197455
Davis, M. J., Tsang, T. M., Qiu, Y., Dayrit, J. K., Freij, J. B., Huffnagle, G. B., et al. (2013). Macrophage M1/M2 polarization dynamically adapts to changes in cytokine microenvironments in Cryptococcus neoformans Infection. MBio. 4:e264-13. doi: 10.1128/mBio.00264-13
Deng, B., Wehling-Henricks, M., Villalta, A. A., Wang, Y., and Tidball, J. G. (2012). Interleukin-10 triggers changes in macrophage phenotype that promote muscle growth and regeneration. J. Immunol. 189, 53669–53680. doi: 10.1038/nmeth.2250.Digestion
Dickensheets, H., Vazquez, N., Sheikh, F., Gingras, S., Murray, P. J., Ryan, J. J., et al. (2007). Suppressor of cytokine signaling-1 is an IL-4-inducible gene in macrophages and feedback inhibits IL-4 signaling. Genes Immun. 8, 21–27. doi: 10.1038/sj.gene.6364352
Doyle, S., Vaidya, S., O’Connell, R., Dadgostar, H., Dempsey, P., Wu, T., et al. (2002). IRF3 mediates a TLR3/TLR4-specific antiviral gene program. Immunity 17, 251–63. doi: 10.1016/S1074-7613(02)00390-4
Eftimie, R., Gillard, J. J., and Cantrell, D. A. (2016). Mathematical models for immunology: current state of the art and future research directions. Bull. Math. Biol. 78, 2091–2134. doi: 10.1007/s11538-016-0214-9
Eizenberg-Magar, I., Rimer, J., Zaretsky, I., Lara-Astiaso, D., Reich-Zeliger, S., and Friedman, N. (2017). Diverse continuum of CD4 + T-cell states is determined by hierarchical additive integration of cytokine signals. Proc. Natl. Acad. Sci. U.S.A. 114, E6447–E6456. doi: 10.1073/pnas.1615590114
Espinosa-Soto, C., Padilla-Longoria, P., and Alvarez-Buylla, E. R. (2004). A Gene regulatory network model for cell-fate determination during Arabidopsis thaliana flower development that is robust and recovers experimental gene expression profiles. Plant Cell. 16, 2923–2939. doi: 10.1105/tpc.104.021725
Foey, A. D. (2014). Macrophages—Masters of Immune Activation, Suppression and Deviation, Immune Response Activation, Guy Huynh Thien Duc, Chap. 5. London: IntechOpen, 121–149. doi: 10.5772/57541
Gao, C.-H., Dong, H.-L., Tai, L., and Gao, X.-M. (2018). Lactoferrin-containing immunocomplexes drive the conversion of human macrophages from M2- into M1-like phenotype. Front. Immunol. 9:37. doi: 10.3389/fimmu.2018.00037
Gong, D., Shi, W., Yi, S., Chen, H., Groffen, J., and Heisterkamp, N. (2012). TGFβ signaling plays a critical role in promoting alternative macrophage activation. BMC Immunol. 13:31. doi: 10.1186/1471-2172-13-31
Gordon, S. (2003). Alternative activation of macrophages. Nat. Rev. Immunol. 3, 23–35. doi: 10.1038/nri978
Gordon, S. (2008). Elie metchnikoff: father of natural immunity. Eur. J. Immunol. 38, 3257–3264. doi: 10.1002/eji.200838855
Gordon, S., and Martinez, F. O. (2010). Alternative activation of macrophages: mechanism and functions. Immunity 32, 593–604. doi: 10.1016/j.immuni.2010.05.007
Gordon, S., Plüddemann, A., and Martinez Estrada, F. (2014). Macrophage heterogeneity in tissues: phenotypic diversity and functions. Immunol. Rev. 262, 36–55. doi: 10.1111/imr.12223
Guevara, M. R. (2003). Bifurcations Involving Fixed Points and Limit Cycles in Biological Systems. New York, NY: Springer, 41–85. doi: 10.1007/978-0-387-21640-9_3
Guilliams, M., Bruhns, P., Saeys, Y., Hammad, H., and Lambrecht, B. N. (2014). The function of Fcγ receptors in dendritic cells and macrophages. Nat. Rev. Immunol. Nat. 14, 94–108. doi: 10.1038/nri3582
Hamilton, J. A. (2008). Colony-stimulating factors in inflammation and autoimmunity. Nat. Rev. Immunol. 8, 533–545. doi: 10.1016/S1471-4906(02)02260-3
Hirano, M., Davis, R. S., Fine, W. D., Nakamura, S., Shimizu, K., Yagi, H., et al. (2007). IgEb immune complexes activate macrophages through FcgammaRIV binding. Nat. Immunol. 8, 762–771. doi: 10.1038/ni1477
Honda, K., Yanai, H., Takaoka, A., and Taniguchi, T. (2005). Regulation of the type I IFN induction: a current view. Int. Immunol. 17, 1367–1378. doi: 10.1093/intimm/dxh318
Hutchins, A. P., Diez, D., and Miranda-Saavedra, D. (2013). The IL-10/STAT3-mediated anti-inflammatory response: recent developments and future challenges. Brief Funct. Genomics 12, 489–498. doi: 10.1093/bfgp/elt028
Ishii, M., Wen, H., Corsa, C. A. S., Liu, T., Coelho, A. L., Allen, R. M., et al. (2009). Epigenetic regulation of the alternatively activated macrophage phenotype. Blood 114, 3244–3254. doi: 10.1182/blood-2009-04-217620
Ito, S., Ansari, P., Sakatsume, M., Dickensheets, H., Vazquez, N., Donnelly, R. P., et al. (1999). Interleukin-10 inhibits expression of both interferon alpha- and interferon gamma- induced genes by suppressing tyrosine phosphorylation of STAT1. Blood 93, 1456–1463.
Ji, J.-D., Tassiulas, I., Park-Min, K.-H., Aydin, A., Mecklenbrauker, I., Tarakhovsky, A., et al. (2003). Inhibition of interleukin 10 signaling after Fc receptor ligation and during rheumatoid arthritis. J. Exp. Med. 197, 1573–1583. doi: 10.1084/jem.20021820
Karlebach, G., and Shamir, R. (2008). Modelling and analysis of gene regulatory networks. Nat. Rev. Mol. Cell. Biol. 9, 770–780. doi: 10.1038/nrm2503
Kawai, T., and Akira, S. (2010). The role of pattern-recognition receptors in innate immunity: update on Toll-like receptors. Nat. Immunol. 11, 373–384. doi: 10.1038/ni.1863
Kawai, T., and Akira, S. (2011). Toll-like receptors and their crosstalk with other innate receptors in infection and immunity. Immunity 34, 637–650. doi: 10.1016/j.immuni.2011.05.006
Kawai, T., Takeuchi, O., Fujita, T., Inoue, J., Mühlradt, P. F., Sato, S., et al. (2001). Lipopolysaccharide stimulates the MyD88-independent pathway and results in activation of IFN-regulatory factor 3 and the expression of a subset of lipopolysaccharide-inducible genes. J. Immunol. 167, 5887–5894. doi: 10.4049/jimmunol.167.10.5887
Kestler, H. A., Wawra, C., Kracher, B., and Kühl, M. (2008). Network modeling of signal transduction: establishing the global view. BioEssays 30, 1110–1125. doi: 10.1002/bies.20834
Kotenko, S.V., Izotova, L. S., Pollack, B. P., Mariano, T. M., Donnelly, R. J., Muthukumaran, G., et al. (1995). Interaction between the components of the interferon gamma receptor complex. J. Biol. Chem. 270, 20915–20921. doi: 10.1074/jbc.270.36.20915
Kraakman, M. J., Murphy, A. J., Jandeleit-Dahm, K., and Kammoun, H. L. (2014). Macrophage polarization in obesity and type 2 diabetes: weighing down our understanding of macrophage function? Front. Immunol. 5:470. doi: 10.3389/fimmu.2014.00470
Krausgruber, T., Blazek, K., Smallie, T., Alzabin, S., Lockstone, H., Sahgal, N., et al. (2011). IRF5 promotes inflammatory macrophage polarization and TH1-TH17 responses. Nat. Immunol. 12, 231–238. doi: 10.1038/ni.1990
Lang, R., Patel, D., Morris, J. J., Rutschman, R. L., and Murray, P. J. (2002a). Shaping gene expression in activated and resting primary macrophages by IL-10. J. Immunol. 169, 2253–2263. doi: 10.4049/jimmunol.169.5.2253
Lang, R., Rutschman, R. L., Greaves, D. R., and Murray, P. J. (2002b). Autocrine deactivation of macrophages in transgenic mice constitutively overexpressing IL-10 under control of the human CD68 promoter. J. Immunol. Am. Assoc. Immunol. 168, 3402–3411. doi: 10.4049/JIMMUNOL.168.7.3402
Lawrence, T., and Natoli, G. (2011). Transcriptional regulation of macrophage polarization: enabling diversity with identity. Nat. Rev. Immunol. 11, 750–761. doi: 10.1038/nri3088
Lehtonen, A., Matikainen, S., Miettinen, M., and Julkunen, I. (2002). Granulocyte-macrophage colony-stimulating factor (GM-CSF)-induced STAT5 activation and target-gene expression during human monocyte/macrophage differentiation. J. Leukoc Biol. 71, 511–519.
Liu, W., Ouyang, X., Yang, J., Liu, J., Li, Q., Gu, Y., et al. (2009). AP-1 activated by toll-like receptors regulates expression of IL-23 p19. J. Biol. Chem. 284, 24006–24016. doi: 10.1074/jbc.M109.025528
Lucas, M., Zhang, X., Prasanna, V., and Mosser, D. M. (2005). ERK activation following macrophage FcgammaR ligation leads to chromatin modifications at the IL-10 locus. J. Immunol. 175, 469–477. doi: 10.4049/jimmunol.175.1.469
Luo, Y., Pollard, J. W., and Casadevall, A. (2010). FC Receptor cross-linking stimulates cell proliferation of macrophages via the ERK pathway. J. Biol. Chem. 285, 4232–4242. doi: 10.1074/jbc.M109.037168
Maiti, S., Dai, W., Alaniz, R., Hahn, J., and Jayaraman, A. (2014). Mathematical modeling of pro- and anti-inflammatory signaling in macrophages. Process. Multidiscipl. Digital Publish. Inst. 3, 1–18. doi: 10.3390/pr3010001
Mantovani, A. (2008). From phagocyte diversity and activation to probiotics: back to Metchnikoff. Eur. J. Immunol. 38, 3269–3273. doi: 10.1002/eji.200838918
Mantovani, A., Sica, A., Sozzani, S., Allavena, P., Vecchi, A., and Locati, M. (2004). The chemokine system in diverse forms of macrophage activation and polarization. Trends Immunol. 25, 677–686. doi: 10.1016/j.it.2004.09.015
Mantovani, A., Sozzani, S., Locati, M., Allavena, P., and Sica, A. M. (2002). Macrophage polarization: tumor-associated macrophages as a paradigm for polarized M2 mononuclear phagocytes. Trends Immunol. 23, 549–555. doi: 10.1016/S1471-4906(02)02302-5
Mao, A.-P., Shen, J., and Zuo, Z. (2015). Expression and regulation of long noncoding RNAs in TLR4 signaling in mouse macrophages. BMC Genomics 16:45. doi: 10.1186/s12864-015-1270-5
Martinez, F. O., Helming, L., and Gordon, S. (2009). Alternative activation of macrophages: an immunologic functional perspective. Annu. Rev. Immunol. 27, 451–483. doi: 10.1146/annurev.immunol.021908.132532
Martinez-Sanchez, M. E., Mendoza, L., Villarreal, C., and Alvarez-Buylla, E. R. (2015). A minimal regulatory network of extrinsic and intrinsic factors recovers observed patterns of CD4+ T cell differentiation and plasticity. PLoS Comput. Biol. Public Libr. Sci. 11:e1004324. doi: 10.1371/journal.pcbi.1004324
McLaren, J. E., and Ramji, D. P. (2009). Interferon gamma: a master regulator of atherosclerosis. Cytokine Growth Fact. Rev. 20, 125–135. doi: 10.1016/j.cytogfr.2008.11.003
Méndez, A., and Mendoza, L. (2016). A network model to describe the terminal differentiation of B cells. PLoS Comput. Biol. 12:e1004696. doi: 10.1371/journal.pcbi.1004696
Moore, K. W., de Waal Malefyt, R., Coffman, R. L., and O’Garra, A. (2001). Interleukin-10 and the interleukin-10 receptor. Annu. Rev. Immunol. 19, 683–765. doi: 10.1146/annurev.immunol.19.1.683
Mosser, D. M., and Edwards, J. P. (2008). Exploring the full spectrum of macrophage activation. Nat. Rev. Immunol. 8, 958–969. doi: 10.1038/nri2448
Müssel, C., Hopfensitz, M., and Kestler, H. A. (2010). BoolNet—an R package for generation, reconstruction and analysis of Boolean networks. Bioinformatics 26, 1378–1380. doi: 10.1093/bioinformatics/btq124
Nakamura, R., Sene, A., Santeford, A., Gdoura, A., Kubota, S., Zapata, N., et al. (2015). IL10-driven STAT3 signalling in senescent macrophages promotes pathological eye angiogenesis. Nat. Commun. 6:7847. doi: 10.1038/ncomms8847
Naldi, A., Berenguier, D., Fauré, A., Lopez, F., Thieffry, D., and Chaouiya, C. (2009). Logical modelling of regulatory networks with GINsim 2.3. BioSystems 97, 134–139. doi: 10.1016/j.biosystems.2009.04.008
Naldi, A., Carneiro, J., Chaouiya, C., and Thieffry, D. (2010). Diversity and plasticity of Th cell types predicted from regulatory network modelling, Bonneau R, editor. PLoS Comput. Biol. 6:e1000912. doi: 10.1371/journal.pcbi.1000912
Nimmerjahn, F., and Ravetch, J. V. (2008). Fcgamma receptors as regulators of immune responses. Nat. Rev. Immunol. 8, 34–47. doi: 10.1038/nri2206
Novak, M., Weinheimer-Haus, E., and Koh, T. (2014). Macrophage activation and skeletal muscle healing following traumatic injury. J. Pathol. 232, 344–355. doi: 10.1038/nmeth.2250.Digestion
O’Carroll, C., Fagan, A., Shanahan, F., and Carmody, R. J. (2013). Identification of a unique hybrid macrophage-polarization state following recovery from lipopolysaccharide tolerance. J. Immunol. 192, 427–436. doi: 10.4049/jimmunol.1301722
Ortiz-Gutiérrez, E., García-Cruz, K., Azpeitia, E., Castillo, A., Sánchez Mde la, P., and Álvarez-Buylla, E. R. (2015). A dynamic gene regulatory network model that recovers the cyclic behavior of Arabidopsis thaliana cell cycle, Albert R, editor. PLoS Comput. Biol. 11:e1004486. doi: 10.1371/journal.pcbi.1004486
Park, B. S., Song, D. H., Kim, H. M., Choi, B.-S., Lee, H., and Lee, J.-O. (2009). The structural basis of lipopolysaccharide recognition by the TLR4-MD-2 complex. Nature 458, 1191–1195. doi: 10.1038/nature07830
Perfetto, L., Briganti, L., Calderone, A., Perpetuini, A. C., Iannuccelli, M., Langone, F., et al. (2016). SIGNOR: a database of causal relationships between biological entities. Nucleic Acids Res. 44, D548–D554. doi: 10.1093/nar/gkv1048
Platanias, L. C. (2005). Mechanisms of type-I- and type-II-interferon-mediated signalling. Nat. Rev. Immunol. 5, 375–386. doi: 10.1038/nri1604
Raes, G., Brys, L., Dahal, B. K., Brandt, J., Grooten, J., Brombacher, F., et al. (2005). Macrophage galactose-type C-type lectins as novel markers for alternatively activated macrophages elicited by parasitic infections and allergic airway inflammation. J. Leukoc Biol. 77, 321–327. doi: 10.1189/jlb.0304212
Rauch, I., Müller, M., and Decker, T. (2013). The regulation of inflammation by interferons and their STATs. JAKSTAT 2:e23820. doi: 10.4161/jkst.23820
Ricote, M., Li, A. C., Willson, T. M., Kelly, C. J., and Glass, C. K. (1998). The peroxisome proliferator-activated receptor-gamma is a negative regulator of macrophage activation. Nature 391, 79–82. doi: 10.1038/34178
Rigamonti, E., Zordan, P., Sciorati, C., Rovere-querini, P., and Brunelli, S. (2014). Macrophage plasticity in skeletal muscle repair. Biomed. Res. Int. 2014:560629. doi: 10.1155/2014/560629
Riley, J. K., Takeda, K., Akira, S., and Schreiber, R. D. (1999). Interleukin-10 receptor signaling through the JAK-STAT pathway. J. Biol. Chem. 274, 16513–16521. doi: 10.1074/jbc.274.23.16513
Ritter, M., Buechler, C., Langmann, T., Orso, E., Klucken, J., and Schmitz, G. (1999). The scavenger receptor CD 163: regulation, promoter structure and genomic organization. Pathobiology 67, 257–261. doi: 10.1159/000028105
Sadler, A. J., and Williams, B. R. G. (2008). Interferon-inducible antiviral effectors. Nat. Rev. Immunol. 8, 559–568. doi: 10.1038/nri2314
Samal, A., and Jain, S. (2008). The regulatory network of E. coli metabolism as a Boolean dynamical system exhibits both homeostasis and flexibility of response. BMC Syst. Biol. 2:21. doi: 10.1186/1752-0509-2-21
Sánchez-Mejorada, G., and Rosales, C. (1998). Signal transduction by immunoglobulin Fc receptors. J. Leukoc Biol. 63, 521–533. doi: 10.1002/jlb.63.5.521
Sang, Y., Miller, L. C., and Blecha, F. (2015). Macrophage polarization in virus-host interactions. J. Clin. Cell Immunol. 6:311. doi: 10.4172/2155-9899.1000311
Sanin, D. E., Prendergast, C. T., and Mountford, A. P. (2015). IL-10 production in macrophages is regulated by a TLR-Driven CREB-mediated mechanism that is linked to genes involved in cell metabolism. J. Immunol. 195, 1218–1232. doi: 10.4049/jimmunol.1500146
Santoni, D., Pedicini, M., and Castiglione, F. (2008). Implementation of a regulatory gene network to simulate the TH1/2 differentiation in an agent-based model of hypersensitivity reactions. Bioinformatics 24, 1374–1380. doi: 10.1093/bioinformatics/btn135
Sato, A., Ohtaki, H., Tsumuraya, T., Song, D., Ohara, K., Asano, M., et al. (2012). Interleukin-1 participates in the classical and alternative activation of microglia/macrophages after spinal cord injury. J. Neuroinflamm. 9:553. doi: 10.1186/1742-2094-9-65
Satoh, T., Takeuchi, O., Vandenbon, A., Yasuda, K., Tanaka, Y., Kumagai, Y., et al. (2010). The Jmjd3-Irf4 axis regulates M2 macrophage polarization and host responses against helminth infection. Nat. Immunol. 11, 936–944. doi: 10.1038/ni.1920
Scandlyn, J. (2000). When AIDS became a chronic disease. West J Med. 172, 130–133. doi: 10.1136/ewjm.172.2.130
Sheikh, F., Dickensheets, H., Gamero, A. M., Vogel, S. N., and Donnelly, R. P. (2014). An essential role for IFN-β in the induction of IFN-stimulated gene expression by LPS in macrophages. J. Leukoc Biol. 96, 591–600. doi: 10.1189/jlb.2A0414-191R
Sica, A., and Mantovani, A. (2012). Macrophage plasticity and polarization: in vivo veritas. J. Clin. Invest. 122, 787–795. doi: 10.1172/JCI59643
Sutterwala, F. S., Noel, G. J., Salgame, P., and Mosser, D. M. (1998). Reversal of proinflammatory responses by ligating the macrophage Fcgamma receptor type I. J. Exp. Med. 188, 217–222. doi: 10.1084/jem.188.1.217
Thomas, R., and Kaufman, M. (2001). Multistationarity, the basis of cell differentiation and memory. I. Structural conditions of multistationarity and other nontrivial behavior. Chaos 11:170. doi: 10.1063/1.1350439
Tieri, P., Prana, V., Colombo, T., Santoni, D., and Castiglione, F. (2014). Multi-scale simulation of T helper lymphocyte differentiation. Adv. Bioinform. Comput. Biol. 8826, 123–134. doi: 10.1007/978-3-319-12418-6_16
Tran-Thi, T. A., Decker, K., and Baeuerle, P. A. (1995). Differential activation of transcription factors NF-kappa B and AP-1 in rat liver macrophages. Hepatology 22, 613–619. doi: 10.1002/hep.1840220235
Vogelpoel, L. T. C., Baeten, D. L. P., de Jong, E. C., and den Dunnen, J. (2015). Control of cytokine production by human Fc gamma receptors: implications for pathogen defense and autoimmunity. Front. Immunol. 6:79. doi: 10.3389/fimmu.2015.00079
Vogelpoel, L. T. C., Hansen, I. S., Rispens, T., Muller, F. J. M., van Capel, T. M. M., Turina, M. C., et al. (2014). Fc gamma receptor-TLR cross-talk elicits pro-inflammatory cytokine production by human M2 macrophages. Nat. Commun. 5:5444. doi: 10.1038/ncomms6444
Wang, N., Liang, H., and Zen, K. (2014). Molecular mechanisms that influence the macrophage M1-M2 polarization balance. Front. Immunol. 5:614. doi: 10.3389/fimmu.2014.00614
Weber, A., Wasiliew, P., and Kracht, M. (2010). Interleukin-1 (IL-1) pathway. Sci. Signal. 3:cm1. doi: 10.1126/scisignal.3105cm1
Whyte, C. S., Bishop, E. T., Ruckerl, D., Gaspar-Pereira, S., Barker, R. N., Allen, J. E., et al. (2011). Suppressor of cytokine signaling (SOCS)1 is a key determinant of differential macrophage activation and function. J. Leukoc Biol. 90, 845–854. doi: 10.1189/jlb.1110644
Yamaoka, K., Otsuka, T., Niiro, H., Arinobu, Y., Niho, Y., Hamasaki, N., et al. (1998). Activation of STAT5 by lipopolysaccharide through granulocyte-macrophage colony-stimulating factor production in human monocytes. J. Immunol. 160, 838–845.
Zhang, Y.-H., He, M., Wang, Y., and Liao, A.-H. (2017). Modulators of the Balance between M1 and M2 macrophages during pregnancy. Front. Immunol. 8:120. doi: 10.3389/fimmu.2017.00120
Zhang, Y., Liu, S., Liu, J., Zhang, T., Shen, Q., Yu, Y., et al. (2009). Immune complex/Ig negatively regulate TLR4-triggered inflammatory response in macrophages through Fc gamma RIIb-dependent PGE2 production. J. Immunol. 182, 554–562. doi: 10.4049/jimmunol.182.1.554
Zheng, X.-F., Hong, Y.-X., Feng, G.-J., Zhang, G.-F., Rogers, H., Lewis, M. A. O., et al. (2013). Lipopolysaccharide-Induced M2 to M1 macrophage transformation for IL-12p70 production is blocked by candida albicans mediated up-regulation of EBI3 expression, Tran DQ, editor. PLoS One 8:e63967. doi: 10.1371/journal.pone.0063967
Keywords: macrophage, differentiation, phenotype, model, gene regulating network, polarization, immune system
Citation: Palma A, Jarrah AS, Tieri P, Cesareni G and Castiglione F (2018) Gene Regulatory Network Modeling of Macrophage Differentiation Corroborates the Continuum Hypothesis of Polarization States. Front. Physiol. 9:1659. doi: 10.3389/fphys.2018.01659
Received: 31 July 2018; Accepted: 02 November 2018;
Published: 27 November 2018.
Edited by:
Matteo Barberis, University of Amsterdam, NetherlandsReviewed by:
Carlos Villarreal, Universidad Nacional Autónoma de México, MexicoNathan Weinstein, Universidad Autónoma de Mexico, Mexico
Copyright © 2018 Palma, Jarrah, Tieri, Cesareni and Castiglione. This is an open-access article distributed under the terms of the Creative Commons Attribution License (CC BY). The use, distribution or reproduction in other forums is permitted, provided the original author(s) and the copyright owner(s) are credited and that the original publication in this journal is cited, in accordance with accepted academic practice. No use, distribution or reproduction is permitted which does not comply with these terms.
*Correspondence: Filippo Castiglione, Zi5jYXN0aWdsaW9uZUBpYWMuY25yLml0