- Jet Propulsion Laboratory, California Institute of Technology, Pasadena, CA, United States
How long should we self-isolate at home to reduce the chances of a second wave of COVID-19? This is a question that billions of people are wondering early 2020 due to the outbreak of the novel coronavirus SARS-CoV-2. This virus can produce a severe pneumonia that has killed over 230,000 people so far, was detected for the first time late 2019 in Wuhan (China), and has spread all over the world due, in part, to the difficulty of detecting and isolating asymptomatic or mild-symptomatic cases. In this paper, we explore how long suppression strategies (i.e., home confinement and social distancing) must be put into practice in highly populated cities to reduce the chances that a quick rebound of COVID-19 infections occur again over the next months. This is explored, using New York City (USA), San Francisco (USA), and Madrid (Spain) as case studies, through a simple but realistic Monte Carlo stochastic model that takes into account that part of the undetected infected individuals remain in circulation propagating the virus. Our simulations reflect that, if suppression strategies are not properly applied, they can be counterproductive because there are high chances that the confinement time has to be lengthened without reducing the total number of infections. We also estimate that, in the most conservative scenario and under the model assumptions, home confinement is effective if applied at least ~110 days in New York City, ~80 days in San Francisco, and ~70 days in Madrid, i.e., until mid-July 2020, early June 2020, and late May 2020, respectively.
Introduction
COVID-19 pneumonia, produced by the novel coronavirus SARS-CoV-2, has become a global public threat a few months after several cases were reported late 2019 in Wuhan, China [1–6]. Preliminary studies suggest that COVID-19 has a mortality rate as high as ~2.5% [2], although actual rates are probably lower because virus carriers that are asymptomatic or present mild symptoms go unnoticed, thus facilitating the rapid dissemination of the virus [7]. COVID-19 is highly contagious, having produced >3,000,000 confirmed infections and >230,000 fatalities in >200 countries/areas/territories as of 30 April 2020, accompanied by a sharp decrease in economic and societal activity all over the world [8]. This caused the World Health Organization to classify COVID-19 as a pandemic on 11 March 2020 [9], and several international leaders to describe this disease as the main challenge facing humanity since World War II.
The preferred strategy to reduce the impact of COVID-19 is suppression [10], consisting of a combination of policies to reverse epidemic growth and keep the total number of infections at low levels until a vaccine becomes available. Suppression is being applied in many countries by encouraging social distancing and decreeing different degrees of home confinement, in some cases by law. For example, as of 30 April 2020, confinement is compulsory, to a greater or lesser extent, in many countries of the European Union (e.g., Italy, Spain, France, Germany), America (e.g., USA, Mexico, Costa Rica, Chile, Argentina), Africa (e.g., South Africa), Asia (e.g., India), and Oceania (e.g., Australia), representing around a third of the world population that cannot freely leave their homes except for essential reasons. Suppression strategies seem to be efficient in China and South Korea to minimize local transmissions of the virus [10–14], although it is unclear whether other areas should apply the same restrictions or there is some degree of flexibility. How long should suppression strategies last to be effective, i.e., to avoid quick rebounds in the transmission once interventions are relaxed? How does the effective intervention time depend on the mobility restrictions imposed to the population and social interaction? Addressing these questions is fundamental to minimize healthcare and societal stress during a pandemic crisis; in addition, estimating effective confinement times will help policy makers to forecast the impact of COVID-19 on economy, and thus make timely decisions. Below, we address the aforementioned questions using a Monte Carlo stochastic framework.
Method
We have developed a Monte Carlo stochastic framework to model local viral transmission (Figure 1). This framework reproduces the typical epidemic bell-shaped curves (Figure 1A); and is used to explore how many days confinement should apply in highly populated cities to be effective, taking into account that individuals with severe symptoms are removed from circulation and individuals with mild or no symptoms can remain undetected, and thus circulating and disseminating the virus [7]. The fundamental rules of our stochastic model are detailed below:
(a) A synthetic population of N individuals is randomly distributed on a 1 km2 domain, two of which are assumed to be initially infected with SARS-CoV-2.
(b) Healthy individuals become infected if they are within the radius of influence (rinf) of virus carriers and if P1 < Pinf, where P1 is a uniform-distribution-generated random number and Pinf is the probability of infection, which decreases when reducing social interactions (e.g., less social gatherings, hand shaking, hugging, or kissing). Note that the larger the radius of influence and the probability of infection, the faster the disease can spread in the population.
(c) Infected individuals are removed from the domain if P2 > Pund (Figure 1B), where P2 is a uniform-distribution-generated random number and Pund is the probability that a person infected with the virus is not detected and thus not removed from circulation (detected virus carriers are assumed to be quarantined and not infect other individuals). Note that Pund decreases with more severe symptoms and with the availability of accurate tests for early case detection.
(d) If a virus carrier is infected during τshed days without being detected, it becomes immune and does not have the ability to continue infecting. Note that τshed represents the period of viral shedding of an infected individual.
(e) The healthy, immune, and undetected infected individuals remaining after applying the previous rules are distributed randomly in the domain to start a new time step (we use 1 day time step).
(f) Rules b-e are repeated until time step τfree, when a certain amount of individuals (chosen randomly among healthy, immune, and infected) are removed from the domain (Figure 1C). This simulates the application of suppression policies, and thus the beginning of confinement of part of the inhabitants. Healthy, immune, and undetected infected individuals remaining after applying mobility restrictions are distributed randomly in the domain. Note that τfree represents the duration of free spread of the outbreak, i.e., when no suppression policies are applied.
(g) The system keeps evolving with time, repeating rules b-e, as long as there are virus carriers undetected in the domain (Figure 1C). No virus carriers is the condition used to ensure that quick rebounds of the disease do not occur once suppression strategies are alleviated. Hence, confinement is considered to be effective if it lasts, at least, the time elapsed between the onset of suppression policies and the day in which the density of infected individuals reduces below one per square kilometer.
We use this model to explore three different scenarios, mimicking the evolution of the COVID-19 epidemic in New York City (USA), San Francisco (USA), and Madrid (Spain). Each simulation is repeated 1,000 times, and we export the mean effective confinement time and the mean ratio of total infections (detected and undetected) in terms of the mobility reduction (i.e., the ratio of individuals confined) and the reduction of social interactions. The values used for the different parameters of the model are provided in Table 1.
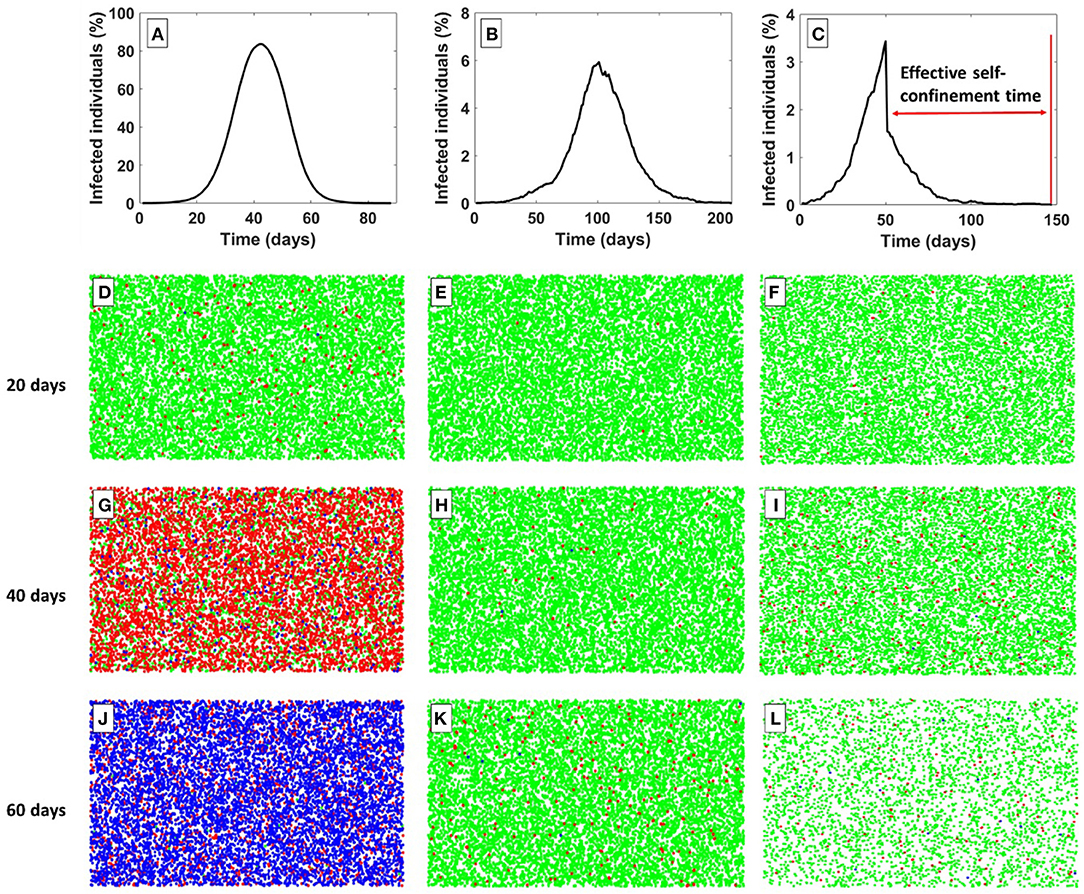
Figure 1. Examples of different simulations performed with our Monte Carlo stochastic model. (A) Variation of infected individuals with time for a scenario in which: (i) virus carriers are never detected (all of them are assumed asymptomatic) nor removed from the domain (probability of not detecting infected individuals, Pund = 100%), and (ii) no part of the population is confined (duration of free spread of the outbreak, τfree → ∞). (B) Variation of infected individuals with time for a scenario in which: (i) there is a Pund = 85% chance that virus carriers are not detected, (ii) infected individuals detected are removed from the domain, and (iii) no confinement of part of the population is imposed (τfree → ∞). (C) Variation of infected individuals with time for a scenario in which: (i) there is a Pund = 85% chance that virus carriers are not detected, (ii) infected individuals detected are removed from the domain, and (iii) confinement of 60% of the population is imposed after τfree = 50 days of free spread of the outbreak. (D–L) Healthy (green), infected (red), and immune (blue) individuals, as predicted with our model, after 20, 40, and 60 days since the onset of the outbreak and for each of the scenarios above. For the rest of the parameters of the model, we use: number of individuals, N = 11, 000; duration of viral shedding, τshed = 20 days; radius of influence, rinf = 4 m; and probability of infection, Pinf = 50%. We use 1 day time step. A Matlab script with the model can be found in Supplementary Material.
Results
The main predictions of our Monte Carlo computational experiments are described below, using New York City as example (Figure 2). First, the confinement time required to avoid quick rebounds of COVID-19 does not necessarily decrease monotonically with mobility reduction, as expected. For example, if social interactions remain as usual (i.e., no social distancing policies apply), the effective confinement time increases on average from ~75 days (no suppression policies) to a maximum of ~125 days, when mobility reduces around 70% with respect to typical values (Figure 2A). Only if mobility reduces beyond 70%, the effective confinement time decreases monotonically with the ratio of individuals confined. For example, with a mobility reduction of 80%, there is a 68% chance (1-sigma confidence level) that the effective isolation time lies in the range ~49–154 days, whereas it is in the range ~23–240 days with a 95% chance (2-sigma confidence level). In contrast, with a mobility reduction of 95%, there is a 68% chance that the effective self-isolation time is in the range ~20–62 days, whereas it is in the range ~6–83 days with a 95% chance. Only with a very strict mobility reduction of 99.5%, the effective isolation time is ≲40 days at a 2-sigma confidence level. A second prediction of the model is that the ratio of people infected (detected and undetected) by the virus decreases from ~97%, if no suppression policies are applied, to a minimum of ~15–20% on average (determined by the infections produced during the free spread of the outbreak) for mobility reductions over ~80% (Figure 2B). Interestingly, whereas the effective isolation time decreases by ~82% if mobility reduction is 99.5% instead of 80%, the ratio of people infected decreases by ~22% only. However, it is important to highlight the high level of uncertainty in the estimation of people infected. For example, with 50% of the individuals confined, 34–67% of the population is predicted to be infected at 1-sigma confidence level, whereas the 2-sigma confidence interval is ~12–84%. In contrast, with 90% of the individuals confined, between ~3 and 37% of the population is expected to be infected at 1-sigma confidence level, whereas the 2-sigma confidence interval is ~1–65%. Even with a strict mobility reduction of 99.5%, there are high chances that up to ~65–70% of the population can become infected during the outbreak in New York City.
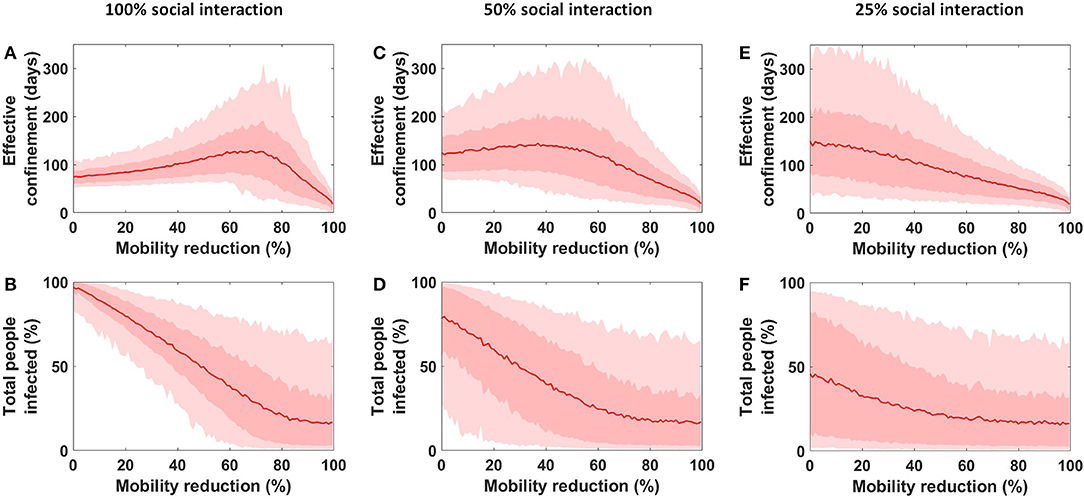
Figure 2. Effective days of confinement and total (detected and undetected) people infected as a function of the mobility reduction (i.e., ratio of individuals confined). (A,B) Scenario in which social interactions do not decrease once confinement is decreed (i.e., no social distancing is applied). (C,D) Scenario in which social interactions (i.e., the probability of infection Pinf) reduce by 50% once confinement is decreed. (E,F) Scenario in which social interactions reduce by 75% once confinement is decreed. The red line is the mean value obtain from 1,000 Monte Carlo simulations, the dark red area represents the 1-sigma confidence interval, and the light red area represents the 2-sigma confidence interval. For the parameters of the model, we use: number of individuals, N = 11, 000; radius of influence, rinf = 4 m; and duration of free spread of the outbreak, τfree = 21 days. The duration of viral shedding is chosen randomly in the range τshed = 8−37 days; and initial probability of infection, Pinf, and probability of not detecting infected individuals, Pund, are chosen randomly between 50 and 100%. Only combinations of the parameters providing exponential growth rates at the beginning of the outbreak in the range 0.2–0.4 day−1 are accepted. These simulations are for the case of New York City.
It is worth highlighting that the results described in the previous paragraph correspond to the end-member scenario in which only confinement is imposed/encouraged, i.e., with no other suppression policies. The other major suppression strategy consists of reducing social interaction through social distancing (e.g., by keeping distance with others, no hand-shaking, no kissing, no hugging, etc.), which this model can account for by assuming that the probability of infection Pinf decreases once confinement begins at time τfree. In such a case, the model yields three major predictions. First, the maximum of effective isolation time moves toward lower confinement ratios (Figures 2A,C,E). For example, the maximum effective isolation time is reached at ~70% confinement if no social distancing is implemented, but it is reached at ~40–45% confinement when the probability of infection reduces to 50% with respect to the beginning of the outbreak. With better implementation of social distancing, the peak eventually disappears and the effective confinement time decreases monotonically when decreasing mobility (Figure 2E). A second prediction is that the ratio of infected individuals is very little sensitive to the confinement ratio if social distancing is strictly implemented; however, the probability that a large amount of the population becomes infected remains very high (Figures 2D,F). Third, reducing the level of social interaction can be harmful if there are no many individuals confined; in such a case, there are high probabilities for the effective confinement time to be longer than with usual social interaction, whereas the number of infections may not diminish significantly. For example, for 20% of the population confined, the effective confinement time is in the range ~31–350 days (2-sigma confidence level) if social interaction reduces to 25% of usual, whereas it is expected to be in the range ~56–127 (2-sigma confidence level) if social interaction is not reduced. Overall results are similar for all the case studies.
Finally, the confinement time that should be applied in the cities of interest (New York, San Francisco, and Madrid) to minimize the chances of a quick second wave of COVID-19 can be inferred if the reduction of mobility (i.e., the ratio of individuals confined) can be constrained. Mobility reduction can be estimated using the Citymapper Mobility Index, as calculated from the trips planned with the Citymapper application [23] (Table 1; Supplementary Figures 1–3). Using this estimate, we explore the effective confinement time as a function of social interaction, i.e., as a function of the probability of infection Pinf (Figure 3). Our Monte Carlo simulations predict that reducing social interaction to 5% of usual can reduce the effective confinement time by up to ~50–60%. For example, reducing social interaction to 5% of usual yields effective confinement times that are ≤ 50 days in New York City and ≤ 37 days in San Francisco and Madrid (95% confidence). In contrast, reducing social interaction to 60% of usual yields effective confinement times that are ≤ 85 days in New York City, ≲65 days in San Francisco, and ≲60 days in Madrid (95% confidence). In the most conservative scenario (i.e., no social distancing), confinement would need to be kept, since it was enforced/encouraged, for ~110 days in New York City (i.e., until mid-July 2020), ~80 days in San Francisco (i.e., until early June 2020), and ~70 days in Madrid (i.e., until late May 2020). This would minimize the possibility of a quick rebound of the disease. Note that there are high chances that New York City, with about double of the population density of Madrid, requires almost double isolation time.
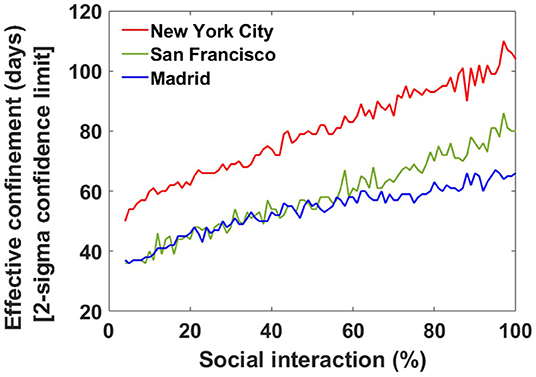
Figure 3. Effective confinement days for the three cities explored as a function of social interaction (if lockdown conditions are not relaxed). The lines represent the 2-sigma upper limit, i.e., there is, at least, a 95% chance that the confinement time required to reduce the density of infected individuals below one per square kilometer is below the lines depicted. Social interaction is expressed in terms of the percentage of the probability of infection at the beginning of the outbreak (i.e., before suppression strategies apply; note that more social interaction implies higher probability of infection). The values of the parameters of the model are provided in Table 1.
Discussion
The Importance of Suppression Strategies
Our model highlights the importance of applying in combination both home confinement and social distancing to reduce the duration that these strategies need to be applied to minimize the chances of a quick second wave of COVID-19. An important prediction is that, if suppression strategies are not properly applied, they not only are ineffective but they can be indeed counterproductive. In other words, a mild application of the suppression strategies can be worse than no applying suppression strategies at all because there are high chances that they lengthen the effective confinement time without reducing the total number of infections (see, for example, Figures 2C,D). This outcome can be interpreted in terms of the average period (T) elapsed between a close interaction of individuals, which in turn depends on the amount of individuals remaining in circulation. No application of the suppression strategies implies that T is much lower than the time that asymptomatic virus carriers can infect (i.e., the shedding time, τshed); in such a case, the virus can propagate very quickly among the population, thus producing a large number of infections in a very short time period. A mild application of the suppression strategies implies that T and the shedding time are on the same order magnitude; in such a case, it is highly likely that many individuals become infected but when virus carriers are close to the end of their contagious period, thus producing a large number of infections in a long time. Mild suppression strategies can therefore diminish healthcare stress but without necessarily decreasing the number of infections and fatalities. However, a strict application of the suppression strategies implies that T is much larger than the shedding time; in such a case, the chances for asymptomatic virus carriers to infect other individuals reduce drastically, thus producing a low number of infections and the prompt elimination of contagious agents.
Approximations of the Model
Several stochastic modeling approaches have been proposed recently to simulate different aspects of the COVID-19 epidemic [10, 13, 14, 24]. This paper aims to simulate the dynamics of virus propagation when symptomatic individuals are quarantined, and through a simple stochastic model that minimizes the number of tuning parameters while being realistic. Our model is based on a set of assumptions and simplifications that are summarized below:
(a) Scaling. We assume that results obtained on a 1 km2 domain are realistic as long as the number of individuals used in the simulations reproduces the average population density of the cities under study. This approach also implies that confinement is considered to be effective when the average density of virus carriers reduces below one per square kilometer.
(b) Closed system. We assume that, in the cities or areas studied, there is no flow of individuals moving in or out from the domain (only those infected individuals that are detected and therefore quarantined and removed from circulation). In such a case, the ratio of infected/healthy individuals is only a function of the interactions in previous time steps. If new individuals (healthy or asymptomatic virus carriers) were imported with time, the effective confinement time would tend to increase.
(c) Person-to-person transmission. We consider that infection occurs predominantly through close contact with virus carriers, which is thought to be the main transmission method [1–4]. Other transmission ways (e.g., through contact with contaminated surfaces) are not taken into account because they are thought to be a second-order source of infection.
(d) Population distribution. We assume for simplicity that encounters between different individuals are controlled by a uniform random distribution. More complex random distributions could be incorporated in the model to account for non-uniform population density and for different confinement conditions in distinct neighborhoods; for example, confinement is probably stricter in richer areas because more people is expected to be able to work remotely. However, more complex random distributions would lead to new tuning parameters that are difficult to constrain because it is impossible to know the actual mobility of free individuals. An outcome from our simulations is therefore that suppression strategies may need to be applied longer in poorer, highly populated, neighborhoods with lower mobility restrictions.
(e) Constant mobility restrictions and social interactions. We assume that, once suppression strategies are put into practice, the degree of mobility reduction and social distancing does not change with time. Variations in the degree of applicability of the suppression strategies might lengthen or shorten the effective confinement time, although that effect may not be significant due to its intrinsic uncertainty.
(f) Interaction with confined individuals. We assume that confined individuals no longer interact with the rest of the population and therefore cannot infect nor be infected, i.e., they are considered a second-order factor in the spreading of the disease. This implies that they are assumed to apply extreme social distancing and cleaning habits (e.g., when they go to the supermarket).
(g) Tuning parameters. Our model contains six different parameters, most of which can be constrained based on previous studies (see Table 1). The duration of viral shedding (τshed) is chosen randomly (assuming uniform distribution) for each individual in the range 8–37 days (range reported from a sample of 191 patients [15]), whereas the probability of infection (Pinf) and the probability that a virus carrier is not detected (Pund) are randomly assigned between 50 and 100%. The actual values of these three parameters are not well-known, but we assume that realistic values are those that produce an exponential growth rate at the beginning of the outbreak in the range 0.2–0.4 day−1 [21]. We do not use the specific exponential growth rates reported for each of the cities explored because data of the nascent phase of epidemics are not typically reliable [25].
Conclusions
The epidemic of COVID-19 spreads quickly due, in part, to the difficulty of detecting and isolating asymptomatic or mild-symptomatic cases, a factor that must be taken into account to forecast the evolution of the outbreak. This is accounted for in this work, focused on estimating how long suppression strategies (i.e., home confinement and social distancing) must be put into practice in highly populated cities in order to reduce the chances that a quick second wave of COVID-19 cases emerge over the next months. In particular, the questions addressed in this work are: How long should suppression strategies last to be effective, i.e., to avoid quick rebounds in the transmission once interventions are relaxed? How does the effective intervention time depend on the mobility restrictions imposed to the population and social interaction? These questions are addressed through a set of Monte Carlo stochastic simulations, using New York City (USA), San Francisco (USA), and Madrid (Spain) as case studies. Our main conclusions are: (1) If suppression strategies are not properly applied, they not only are ineffective but they can be indeed counterproductive because there are high chances that they lengthen the effective confinement time without reducing the total number of infections. This results from a non-linear interplay between degree of confinement, confinement time, and social distancing. (2) Confinement is effective, beyond the 95% confidence level and under the model assumptions, if it is applied ~110 days in New York City, ~80 days in San Francisco, and ~70 days in Madrid. As a general guide, we conclude that these cities should keep >90% of mobility reduction until, at least, mid-July 2020, early June 2020, and late May 2020, respectively; this would minimize the chances of an uncontrolled resurgence of the disease right after restrictions are alleviated.
Data Availability Statement
The original contributions presented in the study are included in the article/Supplementary Material. Further inquiries can be directed to the author.
Author Contributions
TG conceived the idea and led the modeling, analysis, and writing of the manuscript.
Funding
TG was supported by an appointment to the NASA Postdoctoral Program at the Jet Propulsion Laboratory, California Institute of Technology, administered by Universities Space Research Association under contract with the National Aeronautics and Space Administration.
Conflict of Interest
The author declares that the research was conducted in the absence of any commercial or financial relationships that could be construed as a potential conflict of interest.
Acknowledgments
California Institute of Technology Government sponsorship acknowledged. The research was carried out at the Jet Propulsion Laboratory, California Institute of Technology, under a contract with the National Aeronautics and Space Administration (80NM0018D0004). The author appreciate discussions on the matters of this paper with R. Zinke, A. Probst, and E. Havazli.
Supplementary Material
The Supplementary Material for this article can be found online at: https://www.frontiersin.org/articles/10.3389/fphy.2020.00186/full#supplementary-material
References
1. Wang L, Wang Y, Ye D, Liu Q. A review of the 2019 Novel Coronavirus (COVID-19) based on current evidence. Int J Antimicrob Agents. (2020). doi: 10.1016/j.ijantimicag.2020.105948
2. Lai CC, Shih TP, Ko WC, Tang HJ, Hsueh PR. Severe acute respiratory syndrome coronavirus 2 (SARS-CoV-2) and coronavirus disease-2019 (COVID-19): the epidemic and the challenges. Int J Antimicrob Agents. (2020) 55:105924. doi: 10.1016/j.ijantimicag.2020.105924
3. Sahin AR. 2019 Novel Coronavirus (COVID-19) outbreak: a review of the current literature. Eurasian J Med Investig. (2020) 4:1–7. doi: 10.14744/ejmo.2020.12220
4. Sohrabi C, Alsafi Z, O'Neill N, Khan M, Kerwan A, Al-Jabir A, et al. World Health Organization declares global emergency: a review of the 2019 novel coronavirus (COVID-19). Int J Surg. (2020) 76:71–6. doi: 10.1016/j.ijsu.2020.02.034
5. Van Bavel JJ, Baicker K, Boggio PS, Capraro V, Cichocka A, Cikara M, et al. Using Social and Behavioural Science to Support COVID-19 Pandemic Response. Available online at: https://psyarxiv.com/y38m9
6. Perc M, Gorišek Miksić N, Slavinec M, StoŽer A. Forecasting COVID-19. Front Phys. (2020) 8:127. doi: 10.3389/fphy.2020.00127
7. Li R, Pei S, Chen B, Song Y, Zhang T, Yang W, et al. Substantial undocumented infection facilitates the rapid dissemination of novel coronavirus (SARS-CoV2). Science. (2020) 3221:1–9. doi: 10.1126/science.abb3221
8. World Health Organization W. Coronavirus Disease 2019 (COVID-2019). Situation Report - 66. Available online at: https://www.who.int/docs/default-source/coronaviruse/situation-reports/20200326-sitrep-66-covid-19.pdf?sfvrsn=9e5b8b48_2. Situat Rep - 66 (2020).
9. World Health Organization W. Coronavirus Disease 2019 (COVID-2019). Situation Report - 51. Available online at: https://www.who.int/docs/default-source/coronaviruse/situation-reports/20200311-sitrep-51-covid-19.pdf?sfvrsn=1ba62e57_10. World Heal Organization (2020).
10. Ferguson NM, Laydon D, Nedjati-Gilani G, Imai N, Ainslie K, Baguelin M, et al. Impact of Non-pharmaceutical Interventions (NPIs) to Reduce COVID-19 Mortality and Healthcare Demand. Imperial College COVID−19 Response Team (2020).
11. Lau H, Khosrawipour V, Kocbach P, Mikolajczyk A, Schubert J, Bania J, et al. The positive impact of lockdown in Wuhan on containing the COVID-19 outbreak in China. J Travel Med. (2020) 1:taaa037. doi: 10.1093/jtm/taaa037
12. Salzberger B, Glück T, Ehrenstein B. Successful containment of COVID-19: the WHO-Report on the COVID-19 outbreak in China. Infection. (2020) 48:1–3. doi: 10.1007/s15010-020-01409-4
13. Prem K, Liu Y, Russell T, Kucharski AJ, Eggo RM, Davies N, et al. The effect of control strategies that reduce social mixing on outcomes of the COVID-19 epidemic in Wuhan, China. Lancet Public Health. (2020). doi: 10.1016/S2468-2667(20)30073-6
14. Kraemer MUG, Yang C-H, Gutierrez B, Wu C-H, Klein B, Pigott DM, et al. The effect of human mobility and control measures on the COVID-19 epidemic in China. Science. (2020) 21:1–9. doi: 10.1126/science.abb4218
15. Zhou F, Yu T, Du R, Fan G, Liu Y, Liu Z, et al. Clinical course and risk factors for mortality of adult inpatients with COVID-19 in Wuhan, China: a retrospective cohort study. Lancet. (2020) 395:1054–62. doi: 10.1016/S0140-6736(20)30566-3
16. Coronavirus Disease 2019 (COVID-19). NYC Health. Available online at: https://www1.nyc.gov/site/doh/covid/covid-19-main.page
17. City and County of San Francisco. Department of Public Health. Order of the Health Officer No. C19-07. Available online at: https://sf.gov/stay-home-except-essential-needs
18. Department of Public Health. Coronvirus Press Releases and Statements. Available online at: https://www.sfdph.org/dph/alerts/coronavirus-pressreleases.asp
19. Situación de COVID-19 en España. Available online at: https://covid19.isciii.es/
20. Ministerio de Sanidad. Consumo y Bienestar Social - Profesionales - Situación Actual Coronavirus. Available online at: https://www.mscbs.gob.es/profesionales/saludPublica/ccayes/alertasActual/nCov-China/situacionActual.htm
21. Remuzzi A, Remuzzi G. COVID-19 and Italy: what next? Lancet. (2020) 2:10–3. doi: 10.1016/S0140-6736(20)30627-9
22. Loh N-HW, Tan Y, Taculod J, Gorospe B, Teope AS, Somani J, et al. The impact of high-flow nasal cannula (HFNC) on coughing distance: implications on its use during the novel coronavirus disease outbreak. Can J. Anesth. (2020). doi: 10.1007/s12630-020-01634-3
23. Citymapper Mobility Index. Citymapper. Available online at: https://citymapper.com/cmi
24. Kretzschmar ME, Rozhnova G, van Boven ME. Effectiveness of isolation and contact tracing for containment and slowing down a COVID-19 epidemic: a modelling study. medRxiv. (2020). doi: 10.2139/ssrn.3551343
Keywords: COVID-19, coronavirus, confinement time, stochastic model, quick rebound
Citation: Girona T (2020) Confinement Time Required to Avoid a Quick Rebound of COVID-19: Predictions From a Monte Carlo Stochastic Model. Front. Phys. 8:186. doi: 10.3389/fphy.2020.00186
Received: 06 April 2020; Accepted: 28 April 2020;
Published: 08 May 2020.
Edited by:
Matjaž Perc, University of Maribor, SloveniaReviewed by:
Valerio Capraro, Middlesex University, United KingdomJose A. Cuesta, Universidad Carlos III de Madrid, Spain
Copyright © 2020 California Institute of Technology. This is an open-access article distributed under the terms of the Creative Commons Attribution License (CC BY). The use, distribution or reproduction in other forums is permitted, provided the original author(s) and the copyright owner(s) are credited and that the original publication in this journal is cited, in accordance with accepted academic practice. No use, distribution or reproduction is permitted which does not comply with these terms.
*Correspondence: Társilo Girona, dGFyc2lsby5naXJvbmEmI3gwMDA0MDtqcGwubmFzYS5nb3Y=