- 1Turku PET Centre, University of Turku and Turku University Hospital, Turku, Finland
- 2Department of Medical Physics, Turku University Hospital, Turku, Finland
- 3Department of Investigative Radiology, National Cerebral and Cardiovascular Center Research Institute, Osaka, Japan
- 4Athinoula A. Martinos Center for Biomedical Imaging, Department of Radiology, Massachusetts General Hospital and Harvard Medical School, Charlestown, MA, United States
- 5Medical Image Analysis and Biometry Lab, Universidad Rey Juan Carlos, Madrid, Spain
- 6Institute of Neuroscience and Medicine 4, Forschungszentrum, Jülich, Germany
- 7Lawson Health Research Institute, London, ON, Canada
- 8Graduate School of Information Science and Data Science Center, Nara Institute of Science and Technology, Nara, Japan
- 9Institute of Biomedicine, University of Turku, Turku, Finland
In this review, we will summarize the past and current state-of-the-art developments in attenuation and scatter correction approaches for hybrid positron emission tomography (PET) and magnetic resonance (MR) imaging. The current status of the methodological advances for producing accurate attenuation and scatter corrections on PET/MR systems are described, in addition to emerging clinical and research applications. Future prospects and potential applications that benefit from accurate data corrections to improve the quantitative accuracy and clinical applicability of PET/MR are also discussed. Novel clinical and research applications where improved attenuation and scatter correction methods are beneficial are highlighted.
Introduction
Hybrid positron emission tomography/magnetic resonance (PET/MR) systems for clinical imaging have been first introduced nearly a decade ago, with systems capable of either sequential or simultaneous image acquisition. The first concept studies for successful combined PET/MR measurements were initially performed in 1996 [1]. After solving several technical challenges due to the complexity of integration of PET and MR, the first commercial whole-body PET/MR systems were installed in 2010 [2, 3]. PET/MR instrumentation has been an active field and has been discussed in several review articles [4–9]. The introduction of simultaneous PET/MR systems for clinical use has been suggested to mark a paradigm shift for neuroimaging, and the combination of both systems offers a multitude of advantages [10–12]. As can be seen in several clinical and research scenarios, PET/MR offers many advantages over positron emission tomography/computed tomography (PET/CT) or standalone MR.
Fusion information of MR and PET provides advantages over PET and computed tomography (CT) in neurological applications, as overlaying MR and PET image supplies improved diagnostic information in characterization of many conditions [13]. Beyond image fusion, MR offers a library of multiparametric imaging information from morphology, function to even metabolism that cannot be obtained from CT. MR has also an excellent sensitivity in capturing the small changes in brain structure and function [14]. PET on the other hand, offers a high specificity and a wide quality of radiotracers applicable for investigation of numerous molecular targets [14]. Potential applications include metabolism, receptor function, neurotransmitter distribution, inflammation, antigen targeting, and tissue perfusion. Thus, PET/MR unlocks a multitude of novel research and diagnostic applications. To improve data synergy between PET and MR, the focus from hardware integration has shifted to development of integrated data processing and analyses [14]. It is clear that both the research and the diagnostic capabilities of hybrid PET/MR could be extended from that of PET/CT, once fusion information from PET and MR is realized in a more complementary fashion.
However, as PET imaging is severely affected by both photon attenuation and scatter, effective data corrections for both physical phenomena are needed to produce quantitative images reflecting the true spatial distribution of the radiotracer. The physical basis of both scatter and attenuation is explained in detail in review papers of Zaidi and Hasegawa [15], Zaidi and Montandon [16], and Martinez-Möller and Nekolla [17]. Thus, a prerequisite to these corrections is the availability of an accurate attenuation map containing the attenuation coefficients for 511-keV photons at each voxel [17], which makes attenuation correction fundamental to produce visually and quantitatively accurate PET images. Shortly after the introduction of the first commercial PET/MR systems, this became a fundamental limitation of the modality, until the methodological challenges were addressed and effective methods were introduced.
Rotating transmission sources and a bilinear scaling procedure with CT can be applied for attenuation correction on standalone PET and PET/CT systems, respectively [18]. On PET/MR, there are two basic methodological challenges related to attenuation correction. First, MR-based attenuation correction (MRAC) is a challenge due to the very basic idea of what MR images represent—proton density and relaxation time properties of biological tissues [17]. However, for attenuation correction purposes, tissue electron density information needs to be resolved. Therefore, there is no standardized transformation procedure that can translate the MR tissue intensities to tissue attenuation properties as in CT-based attenuation correction (CTAC). An equally important second challenge is the short T2* relaxation time of bone, which makes it hard to delineate bone, unless MR sequences based on ultrashort echo time (UTE) or zero echo time (ZTE) sequences are used [19].
Thus, the main challenges in MRAC originated from deriving bone density information on an individual basis and differentiating bone in MR images. These two challenges led to the first clinically implemented MRAC methods ignoring bone entirely in the body and brain region and replacing bone with soft tissue. However, neglecting bone in attenuation map in the head region was shown to cause large, spatially varying bias in regions close to bone, such as in the cortical regions of the gray matter with errors of magnitude across the brain ranging from −10 to −25% [20, 21]. These errors could also be visually regarded as cortical hypometabolism, representing a potential factor for misdiagnosis, thus impairing the diagnostic quality of the PET/MR images [20, 21]. This led to a large and active field of methodological development in MRAC.
To account for these challenges, a multitude of innovative methods for MRAC were developed over the course of years following the introduction of the first simultaneous PET/MR systems. These methods are well-summarized in the excellent review papers of Martinez-Möller and Nekolla [22], Hofmann et al. [23], Berker and Li [24], Bezrukov et al. [18], Chen and An [25], Izquierdo-Garcia and Catana [26], Keereman et al. [17], Wagenknecht et al. [27], and Mehranian et al. [28] and partially in Teuho [29]. These methods have been proven to be fairly accurate, as a recent multicenter study with 11 MRAC methods and three radiotracers including [18F]-fluorodeoxyglucose ([18F]-FDG), [11C]-Pittsburgh compound B ([11C]-PiB), and [18F]-Florbetabir showed. The methods included have an average global performance within ±5% of CT-based reference [30]. To illustrate this, Figure 1 shows the reported global bias and standard deviation of the methods reported in the MR-Based Attenuation Correction for PET/MR Neuroimaging section, where applicable.
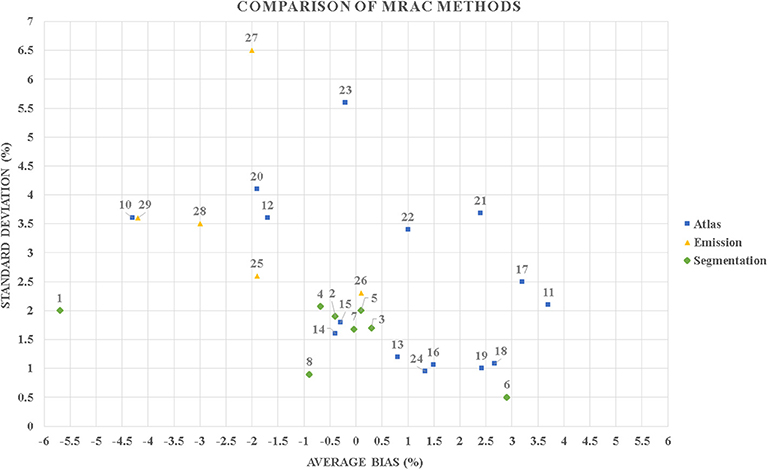
Figure 1. A scatter plot diagram that contains the reported global average bias and standard deviation in percentage of the methods included in this review. It can be seen that majority of the published methods perform with a mean bias up to ±5% in positron emission tomography (PET) evaluation. Most of the methods also have a mean bias in the range of the difference (−6 to +4%) to what was previously reported in a phantom study between different positron emission tomography/computed tomography (PET/CT) and positron emission tomography/magnetic resonance (PET/MR) systems [31]. Segmentation-based methods: 1–4 [30] MR-ACDIXON, MR-ACUTE, MR-ACCAR−RiDR, MR-ACRESOLUTE; 5 [32] 6-class discrete; 6 [33] ZTE; 7 [34] ZTE; 8 [35] ZTE; 9 [36] ZTE. Atlas-based methods: 10–15 [30] MR-ACONTARIO, MR-ACMUNICH, MR-ACSEGBONE, MR-ACUCL, MR-ACMAXPROB, MR-ACBOSTON; 16 [37] Single-atlas; 17 [38] Pattern recognition; 18–19 [39] Mean atlas, PASSR; 20 [40] GMM regression; 21 [41] UTE; 22 [42] Atlas; 23 [43] U-net AC; 24 [44] Single-template. Emission-based methods: 25 [30] MR-ACMLAA, 26 [45] MR-MLAA, 27-29 [46] MLAA, P-MLAA+, P-MLAA++.
Thus, while the challenge of improving accuracy of MRAC with adult brains with normal anatomy can be considered to be solved [30, 47], future methodological advances are of interest. It seems that the focus of methodological research in MRAC is now shifting on improving the accuracy of the existing methods and applying them to novel clinical and research applications. While the accuracy of MRAC is no longer the major methodological problem, increasing accuracy is always desirable [36] in combination of the assessment and application of MRAC methods for more challenging clinical and research applications. Therefore, any application that involves detection of subtle changes in the brain, such as dementia trajectory or epilepsy lesion detection, will benefit from increased accuracy of MRAC and subsequently from accurate scatter correction.
In addition to attenuation correction, scatter correction is one of the major quantitative corrections performed in PET, as the fraction of scattered photons in three-dimensional (3D) PET acquisitions in the brain region can be increased to over 30% [48]. Both attenuation and scatter correction are related, as the attenuation sinogram is used in the calculation of the scattered photons when performing single scatter simulation (SSS). A few reports have investigated the effects of the accuracy of the attenuation map on scatter correction. These investigations unanimously concluded that the errors in the attenuation map introduced to calculated scatter sinograms are much smaller than errors produced by incorrect attenuation correction [49–52]). While scatter correction has not been a major issue to be addressed for neurological PET/MR imaging, developments in scatter correction are also beneficial for increasing the quantitative accuracy of PET images. Consequently, methodological developments that increase the accuracy of attenuation correction will also result in minimization of errors in scatter correction.
MR-Based Attenuation Correction for PET/MR Neuroimaging
A multitude of MRAC methods have emerged, and significant methodological advances have been made in the PET/MR field of neuroimaging, since the introduction of the first PET/MR systems. Attenuation correction for PET/MR can be performed based on anatomical MR images, PET data-driven approaches, or a combination of both. The attenuation correction strategies can be roughly divided into (a) methods based on image segmentation, (b) atlas or database approaches including machine learning methods, and (c) emission data-driven approaches, which use PET data alone or in synergy with existing magnetic resonance imaging (MRI) data. A strict division between methods is challenging, as some of the methodology can be used in combination, e.g., segmentation and template. Table 1 contains a generalized summary of the methodology between different MRAC methods, with concerns, solutions, and future directions.
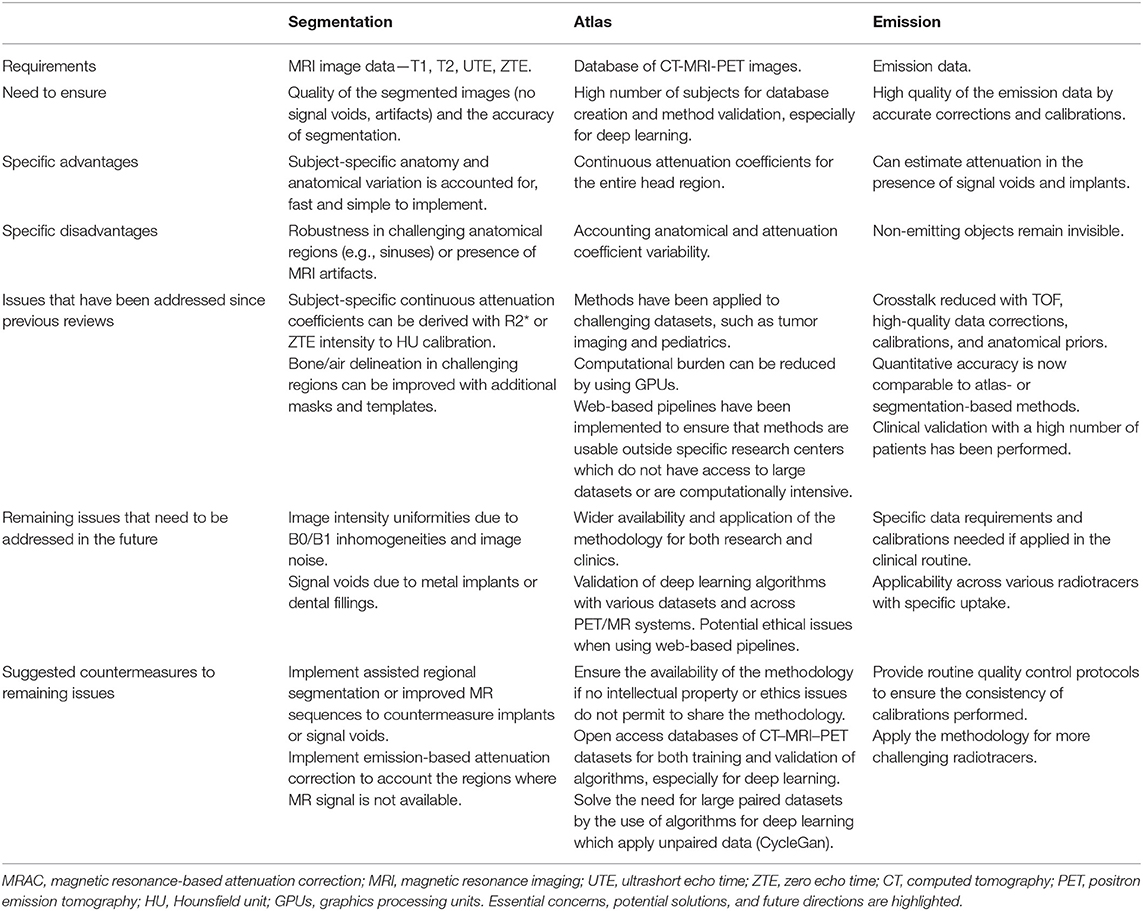
Table 1. Generalized summary of the methodology between (a) segmentation-, (b) template-, and (c) emission-based MRAC methods. Essential concerns, potential solutions, and future directions are highlighted.
For a complete list of methods, we refer to the excellent review papers of Hofmann et al. [22], Berker and Li [23], Bezrukov et al. [53], Izquierdo-Garcia and Catana [18], Keereman et al. [25], Wagenknecht et al. [27], Chen et al. [26], and Mehranian et al. [28]. The physical basis of attenuation correction concerning PET/MR is addressed in Martinez-Möller and Nekolla [17]. In this section, we will focus on methods that allow for bone delineation for brain imaging applications, as methods that ignore bone should be avoided.
MRAC Methods Based on Image Segmentation
The aims of any segmentation-based MRAC method are to divide the tissues from MR images into specific classes and to assign the attenuation coefficients either based on predefined value or determining that value individually. The number of classes used depends on the method, where three tissue classes (bone, soft tissue, air) are considered the minimum, while additional classes might improve the accuracy. The first methodological challenge is to ensure that tissues are segmented and classified in an accurate and reproducible manner, so that the overlap between tissues that have large differences of attenuation coefficients is minimized, such as bone (0.151 cm−1) and air (0.0 cm−1). Challenging regions to segment are those that include, e.g., tissue interfaces such as the sinus region. Challenges for image segmentation for UTE and ZTE can be found, e.g., in Delso et al. [54, 55] and Aasheim et al. [56].
The tissues in the head region might be roughly divided into the following classes: soft tissue (0.094–0.100 cm−1), adipose tissue (0.086–0.093 cm−1), air cavities, and bone. A further division can be made between the nasal cavities (sinus region), different classes of bone (spongy and cortical: 0.130–0.172 cm−1), and different brain tissues (gray and white matter: 0.099 cm−1, cerebrospinal fluid: 0.096 cm−1). Each of these tissues has a different attenuation coefficient, which might vary on an inter- or intrapatient basis. Thus, the most preferable way would be to account and assign the attenuation coefficients on an individual basis. This would reduce the variation caused by the differences in individual anatomy and different patient groups. Thus, the second methodological challenge is to account for the variation of attenuation coefficients in tissues and in patients.
Segmentation-based methods are popular due to ease of implementation and low computational cost. Multiple methods based on segmentation of T1-weighted images have been proposed. Zaidi and Fei proposed using T1-weighted MRI images, which are co-registered to PET data and segmented by fuzzy C-means clustering to air, scalp, skull, gray matter, white matter, and nasal sinuses [57, 58]. Statistical parametric mapping version 8 (SPM8) has also been applied to extract the bone component from T1-weighted images, which is added to the Dixon-based attenuation map [59, 60] or by deriving a three- to six-class attenuation map from T1-images alone [32, 61]. The advantage of these methods is that they are straightforward to apply across multitude of datasets, as the only requirement is access to T1 data, which is collected routinely. In addition to T1-weighted MR images, methods based on segmentation of [18F]-sodium fluoride ([18F]-NaF) PET [62] or on a combination of segmentation and a fixed point source have been applied [63].
Methods based on bone delineation from short echo time (STE) sequences such as UTE or ZTE have become one of the most popular fields in segmentation-based MRAC. To visualize and account for bone, UTE-based methods were introduced in Keereman et al. [64], Catana et al. [65], and Berker et al. [66]. While these methods showed improvements in the visual and quantitative accuracy of PET images, inconsistencies in bone delineation were reported with UTE [21, 56, 67, 68], which later resulted in improved segmentation methods and MRI sequences. After the introduction of UTE, methods based on ZTE emerged [55, 69, 70]. With both UTE and ZTE, the development of more advanced segmentation techniques and new MRI sequences has been an active field of research, to increase the accuracy of bone delineation and segmentation.
To improve the quality of segmentation, several techniques have been applied. One is to use regional masks [71] or anatomical templates [36] to assist in the delineation of different tissues. Specific masks for challenging regions such as the sinus cavities have been also proposed [72, 73]. Delineation of tissues based on tissue clusters from dual echo UTE has also been applied [54]. Recently, improved segmentation with UTE was achieved by using custom templates and tissue probability maps with statistical parametric mapping version 12 (SPM12) segmentation engine to improve the delineation of both air and bone [74]. Machine learning techniques have also been used to refine the quality of the attenuation maps derived with UTE and could potentially be applied in any segmentation-based MRAC method [75].
Improved MRI sequences for UTE include STE/Dixon and fuzzy clustering [76], improved UTE using point-wise encoding time reduction with radial acquisition (PETRA) [77] or likewise a fast dual-echo ramped hybrid encoding (dRHE) [78], and reduction of eddy current artifacts [79]. Sequences based on triple-echo UTE have also been applied [66, 79, 80]. These methods have shown improvements in terms of accuracy of the attenuation map, PET image quality, and quantitative accuracy, compared to UTE-based methods introduced previously. Recent developments include also the use of 3D radial ZTE imaging [81], which is primarily a proton density-weighted sequence. Several studies on successful use of ZTE-based methods on MRAC have been published recently [55, 69, 70, 72] in addition to assessment of repeatability [82].
Nevertheless, all previously described methods assign discrete linear attenuation coefficients to each class. Methods that apply continuous attenuation coefficients for bone have been introduced to address the limitations with discrete attenuation coefficients. A common factor for these methods is to apply a calibration curve (sigmoid, polynomial, or linear function) between the relationship of Hounsfield unit (HU) values vs. R2* values or ZTE intensities. This allows for mapping of the MR intensities in the bone region to HU values on a voxel basis. HU to R2* mapping has been successfully applied in Navalpakkam et al.; [41], Ladefoged et al. [71], Baran et al. [74], and Juttukonda et al. [83], while HU to ZTE intensity transformation has been applied in Khalifé et al. [84] and Yang et al. [72]. The performance of methods using continuous attenuation values is generally considered superior over discrete-tissue methods.
In summary, it can be seen that methods based on UTEs, such as UTE and ZTE, have become a very popular option for segmentation-based MRAC as they allow us to visualize and segment bone with fairly good accuracy, addressing the first challenge, which is the delineation of skull bones. Previously, segmentation-based methods were limited by assigning fixed attenuation coefficients to bone, but lately, this problem has been circumvented by implementing calibration curves between HU and R2* or HU and ZTE intensities, addressing the second methodological challenge. However, there are several regions, such as air–tissue interfaces in the sinus cavity, which are still challenging for segmentation-based methods. To account for this challenge, a multitude of techniques from improved MR acquisition to masks and templates to assist segmentation have been applied, helping to improve the accuracy of the available methods.
Methods Based on Atlas or Database Approaches Including Machine Learning
Atlas- or template-based methods are typically based on using a co-registered database or atlas of CT and MR images. Methods can be further divided into approaches applying a single probabilistic atlas or a multiple atlas. To create a substitute CT, the subject MR image is matched to an MR image in the database or a predefined template. The best match is determined using a predefined similarity metric. Thereafter, the purpose is to create a CT substitute corresponding to subject anatomy with continuous attenuation coefficients for the whole image volume, usually referred to as pseudo-CT. The substitute pseudo-CT is usually created in a volume-by-volume, slice-by-slice, or voxel-by-voxel basis, using a trained classifier, image intensity mapping, or registration techniques. Recently, methods based on machine learning or deep learning techniques have also become increasingly popular.
The most straightforward implementation is to use a single atlas in combination of a predefined template. Templates can be created by taking an average of multiple co-registered CTAC or transmission-based attenuation correction (TXAC) images to represent mean attenuation coefficients and anatomical variability in a given population [85]. Template-based approaches using registration and nonlinear wrapping of a predefined TXAC or CTAC template to the individual subject anatomy using SPM have been proposed [44, 86]. SPM8 has also been used to perform segmentation and state-of-the-art registration with a probabilistic template to derive a pseudo-CT [87]. Similar approaches have been implemented by either wrapping of a CT atlas to the patient MR image using a two-step registration [88] or registering a subject MR image to an MR template and mask pair, followed by a two-step registration to delineate bony regions with the mask [89]. Limitations of the single-atlas approaches include ignoring the intersubject variation of attenuation coefficients and that the anatomical transformation might be prone to errors in registration between the template and subject images, especially with non-conventional anatomy or in the presence of disease.
Several methods based on a multiatlas approach have been introduced, where an atlas of multiple pairs of CT and anatomical MR images is used to derive patient-specific substitute pseudo-CT either by intensity mapping or by registration. Several approaches to derive the pseudo-CT substitute exist, such as using patches to match CT and MRI intensities [90, 91] or image registration techniques [50, 92–94]. The substitute pseudo-CT can be also derived by linking the intensities between CT and MR images with a trained model, such as using a Gaussian mixture regression model with probabilistic measures [40, 95, 96] or a Gaussian mixture model based with patches [97]. While methods using multiple atlases are inherently more complex and computationally intensive, they are able to overcome the limitations of single-atlas approaches [98].
Probabilistic measurers in combination of atlases have been also applied for pseudo-CT creation, which can be used to improve the quality of segmentation or to generate continuous-valued attenuation maps [39, 53, 99]. In addition, pattern recognition [38] or machine learning techniques [41] have been successfully applied. Machine learning applying a random forest regression with patch-based anatomical signatures was used to generate pseudo-CT from T1-weighted images in Yang et al. [100]. Recently, several methods based on deep learning have also become increasingly popular in the creation of a suitable attenuation maps for MRAC.
Santos Ribeiro et al. [101] proposed a feed-forward neural network to directly output a continuous-valued head attenuation map by nonlinear regression of several UTE images and a template-based MRAC map. Gong et al. [102] used a convolutional neural network with Dixon images only or in a combination of Dixon and ZTE images to generate a continuous valued attenuation map. Similarly, a deep convolutional neural network that derived attenuation maps based on ZTE images was shown to outperform both ZTE and atlas-based method in Blanc-Durand et al. [43]. Interestingly, while most evaluations have been performed with adults with normal anatomy, Ladefoged et al. [103] evaluated deep learning methods in pediatric brain tumor patients, with robust performance. The preliminary results obtained with these methods are encouraging.
However, it would be advantageous if conventional MR images collected routinely or PET data could be used to obtain an attenuation map. The use of deep convolutional neural networks with T1 images only has been reported in Han [104] and Liu et al. [105], and they have been applied also to synthetize a pseudo-CT from patient-specific transmission data [106]. Recently, Liu et al. [107] proposed to use only uncorrected PET images with a deep convolutional encoder-decoder network to generate a pseudo-CT. Very recently, a multiparametric MRI model was also suggested to generate pseudo-CT maps based only on Dixon MRI images and was evaluated on head and pelvic images [108]. Among other benefits, these methods also show a great potential for whole-body applications. Finally, any deep learning methodology conventionally requires a large dataset of paired CT and MRI images. Recently, methods based on unpaired image-to-image translation using Cycle-Consistent Generative Adversarial Networks (CycleGan) [109] have been applied to pseudo-CT generation [110, 111], which might circumvent this requirement.
In summary, the atlas or database approaches range from simple single-template methods to complex approaches, allowing us to derive continuous attenuation coefficients not just in bone but also in a range of tissues. There is also a multitude of good methods available. Most of these methods, however, have been solely applied in research context. Until recently, a few promising studies in using atlas-based approaches on non-normal populations such as pediatric patients and in brain tumors have emerged. Naturally, pediatric patients require that an atlas fit for different anatomy will be created. In this regard, the research questions on the applicability of the atlas-based methods for more challenging clinical and research applications is being addressed.
Recently, several machine learning approaches have emerged, which can be applied in a flexible manner. They can be applied in conjunction with segmentation- or emission-based methods, to refine the quality of the segmentation or the resulting attenuation maps. A very promising aspect is that machine learning methods might be able to create accurate attenuation maps solely on conventional T1 and T2 data or from non-attenuation-corrected PET images alone, without requiring specific sequences such as UTE or ZTE. While these methods have initially shown promising results, they need to undergo further validation studies to investigate their applicability in larger patient groups, different datasets, and a variety of radiotracers, in case PET images are used to derive an attenuation map.
Emission-Based Attenuation Correction Approaches for PET/MR
Emission-driven methods allow for estimating attenuation maps (a) based on reconstruction of emission data alone, (b) based on a combination of jointly reconstructed emission and transmission data, and (c) by the use of information from scattered coincidences, background radiation, or radiating sources. An extensive and thorough review of the methods that apply emission data for attenuation correction in PET and single-photon emission computed tomography (SPECT) was conducted by Berker and Li [22]. In this section, we will focus mainly on emerging PET/MR-specific approaches applied for clinical imaging of the head region.
A popular approach for emission-based attenuation correction is the maximum likelihood reconstruction of attenuation and activity (MLAA), which was originally proposed by Nuyts et al. [112], based on a concept introduced by Censor et al. [113]. The MLAA method is based on simultaneous reconstruction of both attenuation and activity using maximum likelihood expectation maximization (MLEM) algorithm and enables deriving an attenuation sinogram up to a constant using measured emission data only. The accuracy of the method is improved if high quality of the emission data can be guaranteed.
To reduce the cross-talk and dependence on count statistics in MLAA, the use of time-of-flight (TOF) information with MLAA has been shown to be beneficial [114]. In addition, incorporating spatial constraints or prior information, e.g., from MR data, can be used to improve the MLAA estimate [115]. MR-guided MLAA imposes MR spatial and CT statistical constraints with a Gaussian mixture model and Markov random field smoothness prior to improving the quality of the attenuation map [116]. Another approach is to jointly estimate the emission distribution and the attenuation correction factors, avoiding the reconstruction of the attenuation map. Rezaei et al. [117] proposed a maximum likelihood algorithm to jointly estimate the activity distribution and attenuation correction factors (MLACF), up to a scaling constant.
Advanced MLAA methods with additional penalty functions might offer better performance in brain imaging as shown in Ahn et al. [118] and Mehranian et al. [46], which could overcome the limitations of earlier MLAA methods for brain imaging when compared to, e.g., atlas-based methods [42]. Recent developments in the methodology have allowed for reaching <5% of error in brain PET quantification with TOF-based MLAA using an MRI prior [46]. Deep learning has been also applied to improve the quality of the MLAA for attenuation correction in the brain using nonconventional tracers, such as 18F-radiolabeled N-(3-fluoropropyl)-2β-carboxymethoxy-3β-(4-iodophenyl) nortropane ([18F]-FP-CIT) used for brain dopamine transporter imaging [119]. Thus, it would seem that most of the limitations in the emission-based methods are being addressed.
Methods that use either transmission sources or background radiation have been also introduced. Improvement of the accuracy of the attenuation maps with an external transmission source was shown in Mollet et al. [120] and Mollet et al. [121], with supplemental transmission sources [122], and using different source geometries [123]. External radiation, such as 176Lu emitted by lutetium oxyorthosilicate/lutetium-yttrium oxyorthosilicate (LSO/LYSO) scintillation crystals, could also be used to acquire transmission data [124], or the scattered background radiation could be used to derived an attenuation map [125]. However, these methods have been mostly applied solely in research context. Recently, an integrated approach that includes a moving point source on a helical path around a 24-channel MR-receiver coil to perform a transmission scan was introduced in Navarro de Lara et al. [126] and Renner et al. [127], which might offer a clinically feasible approach for transmission imaging on brain PET/MR. Finally, several authors have highlighted the benefits of TOF in reducing the errors in the PET images reconstructed with MRAC [84, 128, 129]. This is due to TOF reconstruction being less sensitive to inconsistencies in emission data and data corrections such as attenuation, normalization, and scatter [130].
It can be seen that emission-based attenuation correction is an active field of research, where large clinical validation studies have begun to emerge. A scale-corrected MLACF has recently been shown to provide images that quantitatively and visually correspond to CTAC-reconstructed PET images in 57 patients [131]. Benoit et al. [132] studied a modified non-TOF MLAA algorithm with a relatively large group of patients, i.e., with 204 [18F]-FDG patients, 35 [11C]-PiB patients, and 1 O-(2-[18F]-fluoroethyl)-l-tyrosine ([18F]-FET) patient. Moreover, in a study consisting of 34 dementia patients imaged with [18F]-FDG, an MLAA method was compared with state-of-the-art MRAC and CTAC, producing errors within a few percent [45]. The authors suggested that MLAA might be useful in patients where metal implants or other imaging challenges hinder the use of traditional segmentation-based methods.
In summary, emission-based attenuation correction has been an active field of research, and the methods proposed have begun to show both increases in quantitative accuracy (errors below 5%) and advantages compared to segmentation- or atlas-based methods. For example, emission-based methods might be able to estimate the attenuation coefficients in the presence of metal implants, which would pose a problem for segmentation- or atlas-based methods due to large signal voids. Thus, they would be able to complement both segmentation- or atlas-based methods in challenging cases where they fall short.
There have been traditionally two challenges in emission-based MRAC, which have been addressed in recent methodological studies. Originally, most methods were validated using [18F]-FDG only and a small group of patients. Very recently, however, the applicability of the emission-based methods to a variety of radiotracers has been shown. Moreover, the sensitivity of MLAA to the quality of emission data has been addressed in the following manners. For example, by carefully improving and ensuring the quality of both TOF calibrations and accuracy of data corrections and by the implementation of anatomical priors from MRI, the accuracy of the attenuation map estimation can be improved. Deep learning methods can be also applied to improve the quality of the emission estimate in non-conventional radiotracers.
Status of PET/MR Attenuation Correction for Neuroimaging for Research and Clinics
As can be seen from above, a multitude of MRAC methods are available for research settings, where most of the presented methods perform with good accuracy [30, 133]. While the problem concerning the availability of accurate attenuation correction in research settings has been solved, investigation of new attenuation correction methods still is of interest to improve the accuracy of the available methods for more challenging research applications. Most of the methods presented in the previous chapters might be also applied on PET/MR systems of different vendors, unless a specialized, vendor-dependent sequence or software is required.
However, it seems that there is still an ongoing discussion whether reasonably accurate methods have become commercially available, especially in challenging clinical applications [134], while the accuracy of MRAC can be considered adequate for the majority of routine clinical situations [47]. Furthermore, future efforts for standardization and quality control are important for accurate and robust results in both research and clinics and are needed for PET/MR as well [135]. In addition to technical efforts, knowledge sharing in terms of new guidelines and procedures for PET interpretation and reading will help to improve clinical confidence [28, 136]. Finally, promising methods available for research use might be eventually translated to commercial platforms and thus result in further benefit for clinical applications.
Perhaps the greatest challenge so far has been to present the knowledge gained in the evaluation of different MRAC methods in terms of clinically interpretable information. Impact of MRAC on clinical reading is generally determined by visual analysis, presence of visible artifacts in MRAC or PET, lesion detectability, and standardized uptake value (SUV) quantification accuracy in PET [28]. Recent studies have proposed that standardized, clinical metrics need to be taken into use for MRAC method evaluation, to make direct comparison of method performance less challenging [30, 137]. Implementing more advanced vendor-based attenuation correction methods will certainly result in an increase in the clinical evaluations performed with MRAC.
Concerning the availability of accurate MRAC methods outside the research setting, there are currently two state-of-the-art vendor-based attenuation correction methods for imaging of the brain region on the Siemens mMR and General Electric (GE) Signa PET/MR system. The Philips Ingenuity TF is the only PET/MR system that does not account for bone, as it uses the method described in Schulz et al. [138]. In this regard, while it seems that there is an overflow of MRAC methods to be selected in the research setting, there are also vendor-based methods available that have been successfully applied in a multitude of evaluations in clinical setting. Increasing the amount of clinical evaluations will undoubtedly result in increased clinical confidence in applying the vendor-based methods in clinical routine imaging of, e.g., dementia, epilepsy, and other diagnostic applications.
The Siemens mMR system implements a UTE-based method with fixed attenuation coefficients (bone: 0.151 cm−1, soft tissue: 0.100 cm−1, air: 0 cm−1) for delineating bone for the head region, described in the paper of Aasheim et al. [56] from the internal software version of VB20P and above. Another method for delineating bone with continuous attenuation coefficients using a Dixon sequence and superimposed model-based bone compartment is presented in the paper of Koesters et al. [89]. Similarly, the GE Signa system has two methods routinely available. The first method is based on atlas registration to delineate bone with continuous attenuation coefficients for the head region [88]. The second method is based on a ZTE sequence, which derives bone in the head region by segmentation of ZTE images and assigns continuous attenuation coefficients using a ZTE-intensity vs. HU calibration curve [69], available from the internal software version of MP26 and above.
Clinical evaluations of the impact on MRAC with the vendor-based methods have been performed on the Siemens mMR using the Dixon, UTE, and model-based attenuation correction. In the study of Werner et al., 13 patients suspected of having dementia were imaged with [18F]-FDG. Both UTE and Dixon-based attenuation correction were assessed for differentiating hypometabolism [139]. In a similar study consisting of 16 patients, both model-based and Dixon attenuation corrections were assessed for the visual interpretation of regional hypometabolism [140]. Both studies concluded that the typical patterns of hypometabolism were not significantly changed when even the most inaccurate MRAC was used. Furthermore, several research and clinical methods were recently assessed in terms of z-scores, with 27 patients with suspected dementia [141]. The study concluded that while the research methods proved superior, the model-based attenuation correction should be preferred for diagnostic assessment in the clinical routine.
Similar methods have also been applied in the diagnostic evaluations in brain tumors and amyloid imaging. A study from Rausch et al. evaluated Dixon-based attenuation correction and the model-based method described in Koesters et al. [89], where the authors showed no significant change in diagnosis even when an attenuation map without bone was used [137] in brain tumors. Su et al. [80] showed that an attenuation map without bone is also sufficient for visual interpretation and clinical diagnosis when using [18F]-Florbetapir to determine either amyloid positive or negative status. In a very recent study, Rausch et al. [142] assessed three vendor-based attenuation correction methods, where the authors found no significant changes in time–activity curve (TAC) pattern categorization in [18F]-FET PET and that tumor grading seems to be feasible, regardless of the choice of the MR-AC method. Thus, the accuracy of the vendor-based attenuation correction has been shown to be feasible for both amyloid and tumor imaging.
Evaluations of the template-based method described in the paper of Wollenweber et al. [88] have been performed against CTAC [37, 143], a multiatlas method [98], and ZTE-based attenuation correction [70]. The ZTE method has also been evaluated against Ge-68 transmission sources [34], in dynamic PET [33], and in MR-based radiotherapy of the head [35]. In these evaluations, the ZTE-based method proved superior to the template-based method, especially if the intent is to perform kinetic analysis. These studies have shown that the ZTE-based methods can be considered to be feasible in clinical PET/MR imaging applications. Undoubtedly, more evaluations on the vendor-based methods presented above will follow in the near future.
As can be seen, there are a wide range of MRAC options from the first-generation of segmentation-, atlas-, and emission-based methods to second-generation machine learning and deep learning methods. However, the current application of these methods for PET/MRI in multicenter clinical trials is a challenge contrary to PET/CT in population multisite studies such as Alzheimer's Disease Neuroimaging Initiative (ADNI) or Open Access Series of Imaging Studies (OASIS). Since the methods developed for research used perform, to some extent, differently in terms of different magnitude of residual bias and regional accuracy, the community still needs to work on the standardization of MRAC methods in PET/MR neuroimaging. These challenges could be potentially overcome by using either a commonly available and acceptable library of well-established MRAC methods or by using one comparable, integrated method from either of the PET/MR vendors.
Scatter Correction for PET/MR Neuroimaging
A 30–35% scatter fraction in brain studies can be expected [16], which makes scatter correction one of the fundamental data corrections in addition to attenuation correction. The majority of the scattered events originate from the patient, whereas the physical hardware components contribute from 5 to 15% of the scatter [144]. The physical basis for scatter correction in PET/MR is addressed in the review paper of Martinez-Möller and Nekolla [17]. In this section, we will summarize the current approaches and highlight some emerging applications for scatter correction in brain PET/MR imaging.
While scatter correction has not been a major issue to be addressed for neurological PET/MR imaging, developments in accuracy of scatter correction are considered beneficial especially in regard to quantitative PET imaging. Furthermore, advanced scatter correction methods would be beneficial also in whole-body imaging applications. The current approaches for scatter correction can be divided into (a) historical approaches; (b) simulation or model-based methods, which estimate the single Compton scatter events; and (c) emerging Monte-Carlo-based scatter correction methods and methods based on machine learning. In Table 2, a flowchart describing the main steps in both SSS and Monte-Carlo scatter correction is described.
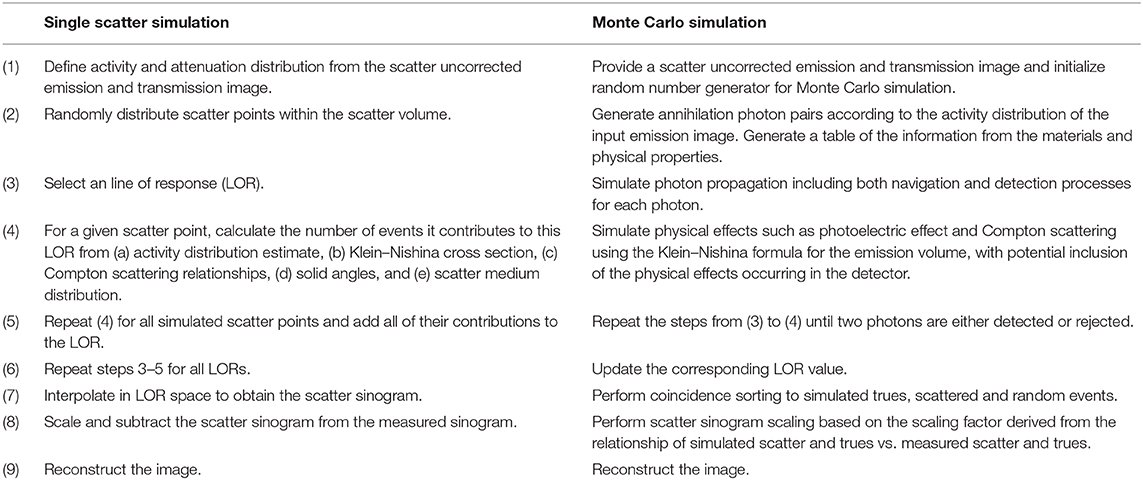
Table 2. Flowchart of single scatter simulation following the methodology in Accorsi et al. [145], Watson et al. [146], and Ollinger [147] in comparison to Monte Carlo simulation-based scatter correction shown in Kim et al. [148], Magota et al. [149], and Ma et al. [150].
Scatter Correction, Historical Approaches
Historically, a multitude of methods were introduced for PET scatter correction, which are summarized in the following books and reviews: [16, 48, 85, 151–154]. These methods were based on either using (1) multiple energy windows to acquire PET data, e.g., Grootoonk et al. [155]; (2) scatter modeling based on convolution and subtraction, e.g., Bergstrom et al. [156] and Bailey and Meikle [157]; and (3) performing Monte Carlo (MC) simulation, e.g., Levin et al. [158]. In addition to software-based methods, hardware approaches such as coarse septa or beam stoppers made from lead were suggested [16]. These methods are no longer in use, with the exception of emerging MC approaches discussed later in this paper.
Much effort was also put into the development of approximate scatter correction techniques for PET, when shifting from two-dimensional (2D) to 3D PET imaging. These techniques are summarized in the following articles: Bailey and Meikle [157], Adam et al. [159], Barney et al. [160], Chen et al. [161], Cherry [162] and Zaidi [163]. The use of full MC methods to derive a “gold standard” approach for scatter modeling was also investigated, although they were considered computationally too intensive to be implemented in clinical routine at the time [158, 164, 165]. Interestingly, MC-based methods have recently reemerged due to the availability of parallel graphics processing units (GPUs), which offer a boost in computing power at a reasonable cost and availability [166].
In the gradual shift from 2D imaging to 3D imaging in PET, most methods were superseded by mainly two approaches based on direct calculation of scatter distribution, which we will refer to as simulation-based approaches.
Scatter Correction, Simulation- and Model-Based Methods
Simulation-based approaches proved most successful for calculation of scatter in 3D PET. Two methods based on direct estimation of scatter distribution became most widely adopted, generally denoted as the SSS algorithm of Watson et al. [146] and the model-based single scatter approach of Ollinger [147], which are incorporated as part of the iterative reconstruction loop in clinical PET/CT and PET/MR systems. The algorithm implementations vary to some extent across different PET system vendors, e.g., comparing the approaches of Siemens Healthcare [167], Philips Healthcare [145], and GE Healthcare [168].
Both the simulation-based [146] and the model-based approaches [147] are essentially based on the direct calculation of the single scatter distribution, which considers emission and attenuation sinograms as input data and is scaled to match the emission distribution. While there are differences in implementation of how single Compton scattered events are modeled between these two approaches, some common principles can be identified. These are as follows: (1) scatter is due to single Compton scatter events, (2) single scatter distribution can be calculated by application of the Klein–Nishina formula using the known emitter density and attenuation coefficients from emission and attenuation sinogram data, and (3) the derived scatter estimate can be scaled to the emission data tails for subtraction (tail fitting) and to account for multiple scattered photons. For discussion in differences in scatter correction approaches and potential relation of scatter correction to MRAC, we refer the interested reader to Teuho [29].
Both simulation- and model-based methods have been extended and modified since the original publications. The details of the modifications in the model-based method are described in detail in the following references: Ollinger [147], Wollenweber et al. [168], Iatrou et al. [169], and Iatrou et al. [170]. Extensions and modifications to the original work in the simulation-based method are described in the following references: Watson et al. [167, 171–174]. A major extension of the original work was to include the calculation of scatter for TOF PET, described in the works of Werner et al. [175], Watson [173], and Iatrou et al. [170], by inclusion of double scatter in the model, which also excludes the need for tail fitting [174, 176].
While the currently available scatter correction methods for PET neuroimaging in general do not pose problems for the majority of neuroimaging studies on PET/MR, there might be specific applications where more accurate methods for scatter correction would be beneficial. As in the case of MRAC, increasing accuracy of the available methods is always seen beneficial.
Emerging Methods Based on MC Simulation and Machine Learning
Methods based on MC simulation and machine learning have recently gained interest. MC methods offer potential improvements in increasing the accuracy of scatter estimation or tail fitting in cases of challenging acquisition conditions. Machine learning methods allow potentially new approaches to be implemented in scatter correction, where the computationally intensive process of scatter calculation could be ignored entirely. In addition to these, several groups have investigated how current scatter correction methods could be improved in terms of tail scaling [177], out-field of view scatter compensation [178], multiple scatters [179], or speed [180].
Improved scatter correction methodology might prove useful in several applications. Data-driven methodologies such as MLAA benefit from accurate data corrections. Recently, it was shown that improved scatter correction is helpful for increasing the visual and quantitative accuracy of PET and could also result in improved attenuation correction with data-driven methodologies using PET and MRI [45]. Furthermore, accurate scatter correction methods could be useful in improving the quantitative accuracy of dynamic PET data with low count statistics [150], which is often the case in neuroimaging research. While studies in the head region do not suffer from the same effects from, e.g., truncation or large bladder-to-background ratio as PET/MR studies in the body region, accurate methodologies developed for the head region might eventually become useful for whole-body PET/MR as well.
With the introduction of fast GPUs that could be implemented in parallel, promising methods based on MC simulation have been proposed. Doing a full MC simulation combined with a GPU implementation could offer a feasible way to implement a very accurate method for scatter correction [166]. Recently, a method based on the paper of Gaens et al. [166] was further refined and applied in a phantom and patient study using a brain PET/MR imaging system [150], with promising initial results. MC simulation has been also applied to implement more robust scaling of the scatter sinograms in the presence of high activity in [15O]-inhalation studies [149]. Combining MC simulation in both scatter calculation and scaling would be beneficial for neurological applications as well as whole-body studies, and the availability of GPU-based methods might enable just that.
Finally, several groups have investigated deep learning methods for scatter estimation to calculate both scatter and attenuation estimates without requiring conventional attenuation map generation and time-consuming scatter correction. The works of Qian et al. [181] and Berker et al. [182] have shown promising early results in using deep learning to calculate scatter estimates for PET, which could offer increases in both accuracy and computational speed. Interestingly, both papers discussed that a deep learning network trained with MC-simulated data would offer further improvements in accuracy [181, 182]. Finally, a very recent work proposed performing both scatter and attenuation corrections in image space using non-corrected PET [183]. Undoubtedly, it is expected that more deep learning-based methods for both attenuation and scatter correction will emerge in the upcoming years, given the popularity of the research topic. An intriguing possibility might be to combine the best of both worlds—by the use of fast GPU-based MC for accuracy and deep learning for computational speed.
Finally, concerning PET/MR neuroimaging, the current status for vendor-based, clinically available scatter correction methods could be summarized very shortly. The SSS algorithm and the model-based SSS with current extensions form the basis of vendor-based scatter correction in PET/MR systems, which offer a well-validated and reasonable accurate solution for a multitude of neurologic applications. Although the challenges in traditional approaches, e.g., related to tail fitting, are well-known in whole-body applications, these generally do not pose a problem, with the exception of very specific neuroimaging applications, such as [15O]-inhalation studies.
It could also be argued, as with MRAC, that the increase of accuracy in scatter correction is beneficial in both research and clinical settings, resulting in improved visual quality and quantitative accuracy. With the increasing appeal of using either a GPU-based MC or a deep learning-based approach for scatter correction, it remains to be seen whether these methods will be implemented as an alternative approaches in future clinical PET/MR systems.
Emerging Clinical and Research Applications
Finally, there are several clinical and research applications where accurate attenuation and scatter correction methods will prove to be beneficial. Eventually, the success of PET/MR depends on the level of confidence revealed by current and future reports on clinical and research applications where combined PET/MR provides useful additional information. This might lead to more widespread acceptance of PET/MR in clinical setting, particularly in neurology and oncology [28]. However, at the same time, it should be emphasized that PET/MR is much more than mere attenuation and scatter correction.
For emerging applications in neurological PET/MR, we wish to highlight recent reports where accurate data corrections are beneficial. These include, e.g., PET measurements of cerebral blood flow (CBF) using [15O]-labeled water ([15O]-H2O) PET [184] and receptor binding studies with [18F]-(E)-N-(3-iodoprop-2-enyl)-2β-carbofluoroethoxy-3β-(4-methyl-phenyl) nortropane ([18F]-PE2I) [33]. When PET is used to derive the arterial input function for quantification and kinetic modeling, it should be ensured that the method delivers consistent performance in terms of regional bias, accuracy, and precision. The impact of accuracy of attenuation correction on dynamic PET studies in PET/MR has been studied for 3,4-dihydroxy-6-[18F] fluoro-L-phenylalanine ([18F]-FDOPA) [185], [11C]-cimbi-36 [186], 4-(2-methoxyphenyl)-1-[2-(N-2-pyridinyl)-p-[18F]fluorobenzamido]ethylpiperazine ([18F]-MPPF) [50], (R)-[11C]-verapamil [187], and carbonyl-11C]N-(2-(1-(4-(2-methoxyphenyl)-piperazinyl)ethyl)-N-pyridinyl) cyclohexanecarboxamide ([11C]-WAY-100635) with [11C] N, N-dimethyl-2-(2-amino-4-cyanophenylthio)benzylamine ([11C]-DASB) [106]. The methods evaluated in these studies have proven to perform with sufficient accuracy for both dynamic and static PET studies. Furthermore, they have highlighted the importance that bias between the reference region (e.g., cerebellum) and the analyzed region should be kept minimal to ensure accurate quantification in kinetic analysis.
Using an image-derived input function (IDIF) for kinetic modeling on PET/MRI will certainly benefit from accurate data corrections and will be further improved by the complementary nature of both modalities. MRI data could be used to assist and complement PET data in several ways [188]. Using TOF MR angiography can improve the delineation of the arterial volume in PET [184, 189–191]. The IDIF could be implemented in an automated pipeline to provide absolute values of cerebral glucose metabolism in a clinically feasible manner [192]. The IDIF measured from either MRI and PET could also be used interchangeably [193]. The need to measure IDIF could also be circumvented entirely by the measurement of global CBF by phase-contrast MRI [194]. Another approach is to incorporate information from arterial spin labeling (ASL) into PET pharmacokinetic modeling [195]. Thus, methods using complementary information from MR with PET for defining IDIF might be beneficial for kinetic modeling in PET, as MR-driven or MR-assisted approaches might be less dependent on accuracy of data corrections.
In regard to emerging applications and new opportunities that complement the neuroimaging field in PET/MR, we wish to briefly highlight the following review papers. A review of the neurologic applications where the complementary natures of PET and MRI are beneficial in both research and clinics has been provided in Chen et al. [14], Chen et al. [196], Catana et al. [197], Hope et al. [198], Miller-Thomas and Benzinger [199]. The clinical applications where PET/MR might clinically excel are brain tumor imaging, epilepsy, stroke, and a number of neurodegenerative conditions, to name a few. Another new clinical field where PET/MR might excel is the study of movement disorders [200]. There are also numerous research applications related to neuroreceptor studies, cerebral metabolism, and blood flow, which are not yet explored extensively. From a technical viewpoint, deep learning has several potential applications, although it has been used mainly for attenuation correction. A very recent review of potential applications of artificial intelligence for PET/MR neuroimaging was given in Zaharchuk [201]. Although out of the scope of this review, synergistic PET and MRI reconstruction might be also be applied to improve PET image quality in terms of noise or resolution.
In addition, we would like to specially mention pediatric PET/MRI imaging, which presents a number of specific challenges [202, 203] but also has the utmost benefits [204, 205]. Clinical applications for PET/MR in pediatrics have been highlighted in Gatidis et al. [206], Kwatra et al. [207], Lee et al. [208]. Accurate PET quantification is crucial in this population, and the use of combined PET/MR with accurate attenuation and scatter corrections could lead to reduced injected doses, as well as reduced exposure to CT radiation burden. This aspect has significance for pediatric cancer patients, who may need to undergo repeated diagnostic imaging sessions. In regard to novel applications, a recent study showed the benefit of PET/MR in low activity imaging (14 MBq) of [15O]-H2O PET for quantitative relative cerebral blood flow (rCBF) assessment in unsedated healthy newborn infants [209]. In this regard, applying and modifying existing MRAC methods to pediatric cohorts such as in Ladefoged et al. [103] are encouraged.
Finally, MR-based radiotherapy treatment (MR-RT) and PET/MR have a lot in common in terms of methodological applications. PET/MR could be even used to improve radiotherapy treatment planning, after the challenges related to patient positioning for radiotherapy planning have been sufficiently addressed [210, 211]. This requires designing MR compatible and PET transparent radiotherapy (RT) equipment [212] and accounting the attenuation of immobilization devices and flat table tops [213]. The information from MR and generated pseudo-CT images could give a better insight for particle RT where the beam range depends strongly on chemical composition [28]. Numerous accurate MRAC methods exist, which could be used for MR-RT, with careful investigation and application of the methodology for more challenging populations undergoing radiotherapy. Similarly, there are a multitude of methods that are used for MR-RT and could be applied for attenuation correction in PET/MR [214, 215]. The methodological advances in both MR-RT and PET/MR could be applied to derive pseudo-CT images on both platforms to improve the PET quantification or accuracy of RT treatment planning.
Discussion—Concluding Remarks and Outlook
In research settings, the accuracy of the attenuation correction is no longer the major methodological factor to be solved, and promising state-of-the-art methods are in the process of being implemented in vendor-based attenuation correction as well. The remaining issues in research settings are being slowly overcome in all major fields of (a) segmentation-based, (b) template-based, and (c) emission-based attenuation corrections. In this regard, the state of MRAC is very positive, with the major focus on improving the accuracy of the existing methods. This would indicate that in the future, MRAC will no longer be considered as an issue, at least in terms of its impact on the clinical interpretation of the images.
In clinical setting, the next challenge to overcome is for the PET/MR community to work on standardization of the use of different MRAC methods in neuroimaging applications. This concerns especially the application of MRAC in PET/MRI in multicenter clinical trials, as there are a wide range of MRAC options available with varying accuracy and regional bias. These challenges could be potentially overcome by using either a commonly available and acceptable library of well-established MRAC methods with similar accuracy or one comparable, integrated method from either of the PET/MR vendors.
While the currently available scatter correction methods for PET neuroimaging in general do not pose problems for the majority of neuroimaging studies on PET/MR, it could be argued that increasing accuracy for scatter correction would be beneficial in a range of clinical and research studies. Methods based on MC calculation might become clinically feasible for accurate scatter scaling and estimation. GPU-based approaches might eventually be implemented in vendor-based methods for scatter calculation.
Deep learning is an emerging trend in medical imaging in general, where both attenuation and scatter corrections are no exception, and where several methods applying deep learning in both attenuation and scatter estimation have emerged. Undoubtedly, more methods will emerge in the near future. However, for the introduced methods to become eventually popular, the applicability of the methods between different PET/MR systems, different MR sequences, PET tracers, and patient populations should be carefully investigated.
Currently available clinical and research methods for attenuation and scatter corrections will be useful in numerous emerging applications in neurological PET/MR, such as dynamic PET studies with different radiotracers, IDIF used for kinetic modeling, clinical applications for neurology, pediatric imaging, and MR-based radiation therapy. Thus, development, application, and refinement of advanced methods for attenuation and scatter correction methods in these fields are further encouraged, with the focus on taking into account fully the simultaneous acquisition of both PET and MRI.
Author Contributions
JT contributed to the conception and design of the content, conducted the review, and wrote the manuscript. AT-C, HH, and UA contributed to the content design, conducting the review, and writing and revising the manuscript. RK, HI, and MT supervised the work and participated in writing and revising the manuscript. All authors contributed to manuscript revision, read, and approved the submitted version.
Funding
This study was financially supported by the Alfred Kordelin Foundation and the Finnish Cultural Foundation, Varsinais-Suomi Regional fund. Financial support was also received from the Hospital District of Southwest Finland (ERVA-project, number 13236) and the funding from the University of Turku Graduate School in the Doctoral Programme in Clinical Research. Finally, the Academy of Finland is acknowledged for the strategic Japanese–Finnish research cooperation program on Application of Medical ICT Devices supported by the Academy of Finland (project number 269977), University of Turku, Turku University Hospital, and the Åbo Akademi University. This project was also partially supported by Real Colegio Complutense at Harvard University Research Fellowships.
Conflict of Interest
The authors declare that the research was conducted in the absence of any commercial or financial relationships that could be construed as a potential conflict of interest.
Acknowledgments
JT acknowledges Prof. Ronald Boellaard for the review and feedback received during the pre-examination phase of his thesis, which eventually evolved into this review paper. In addition, M. D. Terhi Tuokkola was acknowledged for providing her clinical expertise, comments, and viewpoint for the manuscript.
This study was conducted within the Finnish Center of Excellence in Molecular Imaging in Cardiovascular and Metabolic Research supported by the Academy of Finland, University of Turku, Turku University Hospital, and Åbo Akademi University.
References
1. Shao Y, Cherry SR, Farahani K, Meadors K, Siegel S, Silverman RW, et al. Simultaneous PET and MR imaging. Phys Med Biol. (1997) 42:1965–70. doi: 10.1088/0031-9155/42/10/010
2. Ratib O, Willi JP, Wissmeyer M, Steiner C, Allaoua M, Garibotto V, et al. Clinical application of whole body hybrid PET-MR scanner in oncology. Eur J Nucl Med Mol Imaging. (2010) 37:S220.
3. Delso G, Fürst S, Jakoby B, Ladebeck R, Ganter C, Nekolla SG, et al. Performance measurements of the Siemens mMR integrated wholebody PET/MR scanner. J Nucl Med. (2011) 52:1914–22. doi: 10.2967/jnumed.111.092726
4. Vandenberghe S, Marsden PK. PET-MRI: a review of challenges and solutions in the development of integrated multimodality imaging. Phys Med Biol. (2015) 60:R115–54. doi: 10.1088/0031-9155/60/4/R115
5. Herzog H, Lerche C. Advances in clinical PET/MRI Instrumentation. PET Clin. (2016) 11:95–103. doi: 10.1016/j.cpet.2015.09.001
6. Cabello J, Ziegler SI. Advances in PET/MR instrumentation and image reconstruction. Br J Radiol. (2018) 91:20160363. doi: 10.1259/bjr.20160363
7. Disselhorst JA, Bezrukov I, Kolb A, Parl C, Pichler BJ. Principles of PET/MR imaging. J Nucl Med. (2014) 55:2S−10S. doi: 10.2967/jnumed.113.129098
8. Torigian DA, Zaidi H, Kwee TC, Saboury B, Udupa JK, Cho Z-H, et al. PET/MR imaging: technical aspects and potential clinical applications. Radiology. (2013) 267:26–44. doi: 10.1148/radiol.13121038
9. Rausch I, Quick HH, Cal-Gonzalez J, Sattler B, Boellaard R, Beyer T. Technical and instrumentational foundations of PET/MRI. Eur J Radiol. (2017) 94:A3–13. doi: 10.1016/j.ejrad.2017.04.004
10. Herzog H, Pietrzyk U, Shah NJ, Ziemons K. The current state, challenges and perspectives of MR-PET. Neuroimage. (2010) 49:2072–82. doi: 10.1016/j.neuroimage.2009.10.036
11. Zaidi H, Ojha N, Morich M, Griesmer J, Hu Z, Maniawski P, et al. Design and performance evaluation of a whole-body ingenuity TF PET–MRI system. Phys Med Biol. (2011) 56:3091–106. doi: 10.1088/0031-9155/56/10/013
12. Barthel H, Schroeter ML, Hoffmann K-T, Sabri O. PET/MR in dementia and other neurodegenerative diseases. Semin Nucl Med. (2015) 45:224–33. doi: 10.1053/j.semnuclmed.2014.12.003
13. Ehman EC, Johnson GB, Villanueva-Meyer JE, Cha S, Leynes AP, Larson PEZ, et al. PET/MRI: where might it replace PET/CT? J Magn Reson Imaging. (2017) 46:1247–62. doi: 10.1002/jmri.25711
14. Chen Z, Jamadar SD, Li S, Sforazzini F, Baran J, Ferris N, et al. From simultaneous to synergistic MR-PET brain imaging: a review of hybrid MR-PET imaging methodologies. Hum Brain Mapp. (2018) 39:5126–44. doi: 10.1002/hbm.24314
15. Zaidi H, Hasegawa B. Determination of the attenuation map in emission tomography. J Nucl Med. (2003) 44:291–315.
16. Zaidi H, Montandon M-L. Scatter compensation techniques in PET. PET Clin. (2007) 2:219–34. doi: 10.1016/j.cpet.2007.10.003
17. Martinez-Möller A, Nekolla SG. Attenuation correction for PET/MR: problems, novel approaches and practical solutions. Z Med Phys. (2012) 22:299–310. doi: 10.1016/j.zemedi.2012.08.003
18. Hofmann M, Pichler B, Schölkopf B, Beyer T. Towards quantitative PET/MRI: a review of MR-based attenuation correction techniques. Eur J Nucl Med Mol Imaging. (2009) 36:S93–104. doi: 10.1007/s00259-008-1007-7
19. Robson M, Gatehouse P, Bydder M, Bydder G. Magnetic resonance: an introduction to ultrashort TE (UTE) imaging. J Comput Assist Tomogr. (2003) 27:825–46. doi: 10.1097/00004728-200311000-00001
20. Andersen FL, Ladefoged CN, Beyer T, Keller SH, Hansen AE, Højgaard L, et al. Combined PET/MR imaging in neurology: MR-based attenuation correction implies a strong spatial bias when ignoring bone. Neuroimage. (2014) 84:206–16. doi: 10.1016/j.neuroimage.2013.08.042
21. Dickson JC, O'Meara C, Barnes A. A comparison of CT- and MR-based attenuation correction in neurological PET. Eur J Nucl Med Mol Imaging. (2014) 41:1176–89. doi: 10.1007/s00259-013-2652-z
22. Berker Y, Li Y. Attenuation correction in emission tomography using the emission data—a review. Med Phys. (2016) 43:807–32. doi: 10.1118/1.4938264
23. Bezrukov I, Mantlik F, Schmidt H, Schölkopf B, Pichler BJ. MR-Based PET attenuation correction for PET/MR imaging. Semin Nucl Med. (2013) 43:45–59. doi: 10.1053/j.semnuclmed.2012.08.002
24. Chen Y, An H. Attenuation correction of PET/MR imaging. Magn Reson Imaging Clin N Am. (2017) 25:245–55. doi: 10.1016/j.mric.2016.12.001
25. Izquierdo-Garcia D, Catana C. MR imaging–guided attenuation correction of PET data in PET/MR imaging. PET Clin. (2016) 11:129–49. doi: 10.1016/j.cpet.2015.10.002
26. Keereman V, Mollet P, Berker Y, Schulz V, Vandenberghe S. Challenges and current methods for attenuation correction in PET/MR. Magn Reson Mater Phys. (2013) 26:81–98. doi: 10.1007/s10334-012-0334-7
27. Wagenknecht G, Kaiser H-J, Mottaghy FM, Herzog H. MRI for attenuation correction in PET: methods and challenges. Magn Reson Mater Phys. (2013) 26:99–113. doi: 10.1007/s10334-012-0353-4
28. Mehranian A, Arabi H, Zaidi H. Vision 20/20: magnetic resonance imaging-guided attenuation correction in PET/MRI: challenges, solutions, and opportunities. Med Phys. (2016) 43:1130–55. doi: 10.1118/1.4941014
29. Teuho J. MR-based attenuation correction and scatter correction in neurological PET/MR imaging with 18F-FDG (Ph.D. dissertation), University of Turku, Turku, Finland (2018). Available online at: http://urn.fi/URN:ISBN:978-951-29-7138-1
30. Ladefoged CN, Law I, Anazodo U, St. Lawrence K, Izquierdo-Garcia D, Catana C, et al. A multi-centre evaluation of eleven clinically feasible brain PET/MRI attenuation correction techniques using a large cohort of patients. Neuroimage. (2017) 147:346–59. doi: 10.1016/j.neuroimage.2016.12.010
31. Teuho J, Johansson J, Linden J, Hansen AE, Holm S, Keller SH, et al. Effect of attenuation correction on regional quantification between PET/MR and PET/CT: a multicenter study using a 3-dimensional brain phantom. J Nucl Med. (2016) 57:818–24. doi: 10.2967/jnumed.115.166165
32. Teuho J, Tuisku J, Karlsson A, Linden J, Teräs M. Effect of brain tissue and continuous template-based skull in MR-based attenuation correction for brain PET/MR. IEEE Trans Radiat Plasma Med Sci. (2017) 1:246–61. doi: 10.1109/TNS.2017.2692306
33. Schramm G, Koole M, Willekens SMA, Rezaei A, Van Weehaeghe D, Delso G, et al. Regional accuracy of ZTE-based attenuation correction in static and dynamic brain PET/MR. arXiv. (2018) 180603481. Available online at: http://arxiv.org/abs/1806.03481
34. Sousa JM, Appel L, Engström M, Papadimitriou S, Nyholm D, Larsson E-M, et al. Evaluation of zero-echo-time attenuation correction for integrated PET/MR brain imaging-comparison to head atlas and 68Ge-transmission-based attenuation correction. EJNMMI Phys. (2018) 5:20. doi: 10.1186/s40658-018-0220-0
35. Wiesinger F, Bylund M, Yang J, Kaushik S, Shanbhag D, Ahn S, et al. Zero TE-based pseudo-CT image conversion in the head and its application in PET/MR attenuation correction and MR-guided radiation therapy planning. Magn Reson Med. (2018) 80:1440–51. doi: 10.1002/mrm.27134
36. Delso G, Kemp B, Kaushik S, Wiesinger F, Sekine T. Improving PET/MR brain quantitation with template-enhanced ZTE. Neuroimage. (2018) 181:403–13. doi: 10.1016/j.neuroimage.2018.07.029
37. Sekine T, Buck A, Delso G, Ter Voert EEGW, Huellner M, Veit-Haibach P, et al. Evaluation of atlas-based attenuation correction for integrated PET/MR in human brain: application of a head atlas and comparison to true CT-based attenuation correction. J Nucl Med. (2016) 57:215–20. doi: 10.2967/jnumed.115.159228
38. Hofmann M, Steinke F, Scheel V, Charpiat G, Farquhar J, Aschoff P, et al. MRI-based attenuation correction for PET/MRI: a novel approach combining pattern recognition and atlas registration. J Nucl Med. (2008) 49:1875–83. doi: 10.2967/jnumed.107.049353
39. Chen Y, Juttukonda M, Su Y, Benzinger T, Rubin BG, Lee YZ, et al. Probabilistic air segmentation and sparse regression estimated pseudo CT for PET/MR attenuation correction. Radiology. (2015) 275:562–9. doi: 10.1148/radiol.14140810
40. Larsson A, Johansson A, Axelsson J, Nyholm T, Asklund T, Riklund K, et al. Evaluation of an attenuation correction method for PET/MR imaging of the head based on substitute CT images. MAGMA. (2013) 26:127–36. doi: 10.1007/s10334-012-0339-2
41. Navalpakkam BK, Braun H, Kuwert T, Quick HH. Magnetic resonance-based attenuation correction for PET/MR hybrid imaging using continuous valued attenuation maps. Invest Radiol. (2013) 48:323–32. doi: 10.1097/RLI.0b013e318283292f
42. Mehranian A, Arabi H, Zaidi H. Quantitative analysis of MRI-guided attenuation correction techniques in time-of-flight brain PET/MRI. Neuroimage. (2016) 130:123–33. doi: 10.1016/j.neuroimage.2016.01.060
43. Blanc-Durand P, Khalife M, Sgard B, Kaushik S, Soret M, Tiss A, et al. Attenuation correction using 3D deep convolutional neural network for brain 18F-FDG PET/MR: comparison with Atlas ZTE and CT based attenuation correction. PLoS ONE. (2019) 14:e0223141. doi: 10.1371/journal.pone.0223141
44. Rota Kops E, Hautzel H, Herzog H, Antoch G, Shah NJ. Comparison of template-based versus CT-based attenuation correction for hybrid MR/PET scanners. IEEE Trans Nucl Sci. (2015) 62:2115–21. doi: 10.1109/TNS.2015.2452574
45. Rezaei A, Schramm G, Willekens SM, Delso G, Laere KV, Nuyts J. A quantitative evaluation of joint activity and attenuation reconstruction in TOF-PET/MR brain imaging. J Nucl Med. (2019) 60:1649–55. doi: 10.2967/jnumed.118.220871
46. Mehranian A, Zaidi H, Reader AJ. MR-guided joint reconstruction of activity and attenuation in brain PET-MR. Neuroimage. (2017) 162:276–88. doi: 10.1016/j.neuroimage.2017.09.006
47. Bailey DL, Pichler BJ, Gückel B, Antoch G, Barthel H, Bhujwalla ZM, et al. Combined PET/MRI: global warming-summary report of the 6th international workshop on PET/MRI, March 27–29, 2017, Tübingen, Germany. Mol Imaging Biol. (2018) 20:4–20. doi: 10.1007/s11307-017-1123-5
48. Zaidi H, Koral KF. Scatter modelling and compensation in emission tomography. Eur J Nucl Med Mol Imaging. (2004) 31:761–82. doi: 10.1007/s00259-004-1495-z
49. Burgos N, Thielemans K, Cardoso MJ, Markiewicz P, Jiao J, Dickson J, et al. Effect of scatter correction when comparing attenuation maps: application to brain PET/MR. In: 2014 IEEE Nuclear Science Symposium and Medical Imaging Conference (NSS/MIC). Seattle, WA (2014). p. 1–5. doi: 10.1109/NSSMIC.2014.7430775
50. Mérida I, Reilhac A, Redouté J, Heckemann RA, Costes N, Hammers A. Multi-atlas attenuation correction supports full quantification of static and dynamic brain PET data in PET-MR. Phys Med Biol. (2017) 62:2834–58. doi: 10.1088/1361-6560/aa5f6c
51. Son Y-D, Kim H-K, Kim S-T, Kim N-B, Kim Y-B, Cho Z-H. Analysis of biased PET images caused by inaccurate attenuation coefficients. J Nucl Med. (2010) 51:753–60. doi: 10.2967/jnumed.109.070326
52. Teuho J, Saunavaara V, Tolvanen T, Tuokkola T, Karlsson A, Tuisku J, et al. Quantitative evaluation of 2 scatter-correction techniques for 18F-FDG brain PET/MRI in regard to MR-based attenuation correction. J Nucl Med. (2017) 58:1691–8. doi: 10.2967/jnumed.117.190231
53. Chen KT, Izquierdo-Garcia D, Poynton CB, Chonde DB, Catana C. On the accuracy and reproducibility of a novel probabilistic atlas-based generation for calculation of head attenuation maps on integrated PET/MR scanners. Eur J Nucl Med Mol Imaging. (2017) 44:398–407. doi: 10.1007/s00259-016-3489-z
54. Delso G, Zeimpekis K, Carl M, Wiesinger F, Hüllner M, Veit-Haibach P. Cluster-based segmentation of dual-echo ultra-short echo time images for PET/MR bone localization. EJNMMI Phys. (2014) 1:7. doi: 10.1186/2197-7364-1-7
55. Delso G, Wiesinger F, Sacolick LI, Kaushik SS, Shanbhag DD, Hüllner M, et al. Clinical evaluation of zero-echo-time MR imaging for the segmentation of the skull. J Nucl Med. (2015) 56:417–22. doi: 10.2967/jnumed.114.149997
56. Aasheim LB, Karlberg A, Goa PE, Håberg A, Sørhaug S, Fagerli U-M, et al. PET/MR brain imaging: evaluation of clinical UTE-based attenuation correction. Eur J Nucl Med Mol Imaging. (2015) 42:1439–46. doi: 10.1007/s00259-015-3060-3
57. Zaidi H, Montandon M-L, Slosman DO. Magnetic resonance imaging-guided attenuation and scatter corrections in three-dimensional brain positron emission tomography. Med Phys. (2003) 30:937–48. doi: 10.1118/1.1569270
58. Fei B, Yang X, Nye JA, Aarsvold JN, Raghunath N, Cervo M, et al. MR/PET quantification tools: Registration, segmentation, classification, and MR-based attenuation correction. Med Phys. (2012) 39:6443–54. doi: 10.1118/1.4754796
59. Anazodo UC, Thiessen JD, Ssali T, Mandel J, Günther M, Butler J, et al. Feasibility of simultaneous whole-brain imaging on an integrated PET-MRI system using an enhanced 2-point Dixon attenuation correction method. Front Neurosci. (2015) 8:434. doi: 10.3389/fnins.2014.00434
60. Izquierdo-Garcia D, Eldaief MC, Vangel MG, Catana C. Intrascanner reproducibility of an SPM-based head MR-based attenuation correction method. IEEE Trans Radiat Plasma Med Sci. (2019) 3:327–33. doi: 10.1109/TRPMS.2018.2868946
61. Teuho J, Linden J, Johansson J, Tuisku J, Tuokkola T, Teräs M. Tissue probability-based attenuation correction for brain PET/MR by using SPM8. IEEE Trans Nucl Sci. (2016) 63:2452–63. doi: 10.1109/TNS.2015.2513064
62. Schramm G, Maus J, Hofheinz F, Petr J, Lougovski A, Beuthien-Baumann B, et al. Correction of quantification errors in pelvic and spinal lesions caused by ignoring higher photon attenuation of bone in [18F]NaF PET/MR. Med Phys. (2015) 42:6468–76. doi: 10.1118/1.4932367
63. Kawaguchi H, Hirano Y, Yoshida E, Kershaw J, Shiraishi T, Suga M, et al. A proposal for PET/MRI attenuation correction with μ-values measured using a fixed-position radiation source and MRI segmentation. Nucl Instr Methods Phys Res Sect A Acceler Spectrom Detect Assoc Equip. (2014) 734:156–61. doi: 10.1016/j.nima.2013.09.015
64. Keereman V, Fierens Y, Broux T, Deene YD, Lonneux M, Vandenberghe S. MRI-based attenuation correction for PET/MRI using ultrashort echo time sequences. J Nucl Med. (2010) 51:812–8. doi: 10.2967/jnumed.109.065425
65. Catana C, van der Kouwe A, Benner T, Michel CJ, Hamm M, Fenchel M, et al. Toward implementing an MRI-based PET attenuation-correction method for neurologic studies on the MR-PET brain prototype. J Nucl Med. (2010) 51:1431–8. doi: 10.2967/jnumed.109.069112
66. Berker Y, Franke J, Salomon A, Palmowski M, Donker HCW, Temur Y, et al. MRI-based attenuation correction for hybrid PET/MRI systems: a 4-class tissue segmentation technique using a combined ultrashort-echo-time/Dixon MRI sequence. J Nucl Med. (2012) 53:796–804. doi: 10.2967/jnumed.111.092577
67. Choi H, Cheon GJ, Kim H-J, Choi SH, Lee JS, Kim Y, et al. Segmentation-based MR attenuation correction including bones also affects quantitation in brain studies: an initial result of 18F-FP-CIT PET/MR for patients with Parkinsonism. J Nucl Med. (2014) 55:1617–22. doi: 10.2967/jnumed.114.138636
68. Delso G, Carl M, Wiesinger F, Sacolick L, Porto M, Hüllner M, et al. Anatomic evaluation of 3-dimensional ultrashort-echo-time bone maps for PET/MR attenuation correction. J Nucl Med. (2014) 55:780–5. doi: 10.2967/jnumed.113.130880
69. Wiesinger F, Sacolick LI, Menini A, Kaushik SS, Ahn S, Veit-Haibach P, et al. Zero TEMR bone imaging in the head. Magn Reson Med. (2016) 75:107–14. doi: 10.1002/mrm.26094
70. Sekine T, Ter Voert EEGW, Warnock G, Buck A, Huellner M, Veit-Haibach P, et al. Clinical evaluation of zero-echo-time attenuation correction for brain 18F-FDG PET/MRI: comparison with atlas attenuation correction. J Nucl Med. (2016) 57:1927–32. doi: 10.2967/jnumed.116.175398
71. Ladefoged CN, Benoit D, Law I, Holm S, Kjær A, Højgaard L, et al. Region specific optimization of continuous linear attenuation coefficients based on UTE (RESOLUTE): application to PET/MR brain imaging. Phys Med Biol. (2015) 60:8047–65. doi: 10.1088/0031-9155/60/20/8047
72. Yang J, Wiesinger F, Kaushik S, Shanbhag D, Hope TA, Larson PEZ, et al. Evaluation of sinus/edge-corrected zero-echo-time-based attenuation correction in brain PET/MRI. J Nucl Med. (2017) 58:1873–9. doi: 10.2967/jnumed.116.188268
73. Teuho J, Tuisku J, Linden J, Teräs M. Effect of sinus attenuation in MR-based attenuation correction in 18F-FDG brain PET/MR. In: EMBEC & NBC 2017. IFMBE Proceedings. Singapore: Springer (2017). p. 266–9. doi: 10.1007/978-981-10-5122-7_67
74. Baran J, Chen Z, Sforazzini F, Ferris N, Jamadar S, Schmitt B, et al. Accurate hybrid template-based and MR-based attenuation correction using UTE images for simultaneous PET/MR brain imaging applications. BMC Med Imaging. (2018) 18:41. doi: 10.1186/s12880-018-0283-3
75. Shi K, Fürst S, Sun L, Lukas M, Navab N, Förster S, et al. Individual refinement of attenuation correction maps for hybrid PET/MR based on multi-resolution regional learning. Comput Med Imaging Graph. (2017) 60:50–7. doi: 10.1016/j.compmedimag.2016.11.005
76. Khateri P, Saligheh Rad H, Jafari AH, Fathi Kazerooni A, Akbarzadeh A, Shojae Moghadam M, et al. Generation of a four-class attenuation map for MRI-based attenuation correction of PET data in the head area using a novel combination of STE/Dixon-MRI and FCM clustering. Mol Imaging Biol. (2015) 17:884–92. doi: 10.1007/s11307-015-0849-1
77. Grodzki DM, Jakob PM, Heismann B. Ultrashort echo time imaging using pointwise encoding time reduction with radial acquisition (PETRA). Magn Reson Med. (2012) 67:510–8. doi: 10.1002/mrm.23017
78. Jang H, Liu F, Bradshaw T, McMillan AB. Rapid dual-echo ramped hybrid encoding MR-based attenuation correction (dRHE-MRAC) for PET/MR. Magn Reson Med. (2018) 79:2912–22. doi: 10.1002/mrm.26953
79. Aitken AP, Giese D, Tsoumpas C, Schleyer P, Kozerke S, Prieto C, et al. Improved UTE-based attenuation correction for cranial PET-MR using dynamic magnetic field monitoring. Med Phys. (2014) 41:012302. doi: 10.1118/1.4837315
80. Su K-H, Hu L, Stehning C, Helle M, Qian P, Thompson CL, et al. Generation of brain pseudo-CTs using an undersampled, single-acquisition UTE-mDixon pulse sequence and unsupervised clustering. Med Phys. (2015) 42:4974–86. doi: 10.1118/1.4926756
81. Madio DP, Lowe IJ. Ultra-fast imaging using low flip angles and FIDs. Magn Reson Med. (1995) 34:525–9. doi: 10.1002/mrm.1910340407
82. Delso G, Fernandez B, Wiesinger F, Jian Y, Bobb C, Jansen F. Repeatability of ZTE bone maps of the head. IEEE Trans Radiat Plasma Med Sci. (2018) 2:244–9. doi: 10.1109/TRPMS.2017.2772329
83. Juttukonda MR, Mersereau BG, Chen Y, Su Y, Rubin BG, Benzinger TLS, et al. MR-based attenuation correction for PET/MRI neurological studies with continuous-valued attenuation coefficients for bone through a conversion from R2* to CT-Hounsfield units. Neuroimage. (2015) 112:160–8. doi: 10.1016/j.neuroimage.2015.03.009
84. Khalifé M, Fernandez B, Jaubert O, Soussan M, Brulon V, Buvat I, et al. Subject-specific bone attenuation correction for brain PET/MR: can ZTE-MRI substitute CT scan accurately? Phys Med Biol. (2017) 62:7814–32. doi: 10.1088/1361-6560/aa8851
85. Zaidi H, Montandon M-L, Meikle S. Strategies for attenuation compensation in neurological PET studies. Neuroimage. (2007) 34:518–41. doi: 10.1016/j.neuroimage.2006.10.002
86. Rota Kops E, Herzog H. Template based attenuation correction for PET in MR-PET scanners. In: IEEE Nuclear Science Symposium Conference Record, 2008 NSS '08. Honolulu, HI (2008) p. 3786–9. doi: 10.1109/NSSMIC.2007.4437073
87. Izquierdo-Garcia D, Hansen AE, Förster S, Benoit D, Schachoff S, Fürst S, et al. An SPM8-based approach for attenuation correction combining segmentation and nonrigid template formation: application to simultaneous PET/MR brain imaging. J Nucl Med. (2014) 55:1825–30. doi: 10.2967/jnumed.113.136341
88. Wollenweber SD, Ambwani S, Delso G, Lonn AHR, Mullick R, Wiesinger F, et al. Evaluation of an atlas-based PET head attenuation correction using PET/CT & MR patient data. IEEE Trans Nucl Sci. (2013) 60:3383–90. doi: 10.1109/TNS.2013.2273417
89. Koesters T, Friedman KP, Fenchel M, Zhan Y, Hermosillo G, Babb J, et al. Dixon sequence with superimposed model-based bone compartment provides highly accurate PET/MR attenuation correction of the brain. J Nucl Med. (2016) 57:918–24. doi: 10.2967/jnumed.115.166967
90. Andreasen D, Van Leemput K, Hansen RH, Andersen JAL, Edmund JM. Patch-based generation of a pseudo CT from conventional MRI sequences for MRI-only radiotherapy of the brain. Med Phys. (2015) 42:1596–605. doi: 10.1118/1.4914158
91. Torrado-Carvajal A, Herraiz JL, Alcain E, Montemayor AS, Garcia-Cañamaque L, Hernandez-Tamames JA, et al. Fast patch-based pseudo-CT synthesis from T1-weighted MR images for PET/MR attenuation correction in brain studies. J Nucl Med. (2016) 57:136–43. doi: 10.2967/jnumed.115.156299
92. Burgos N, Cardoso MJ, Thielemans K, Modat M, Pedemonte S, Dickson J, et al. Attenuation correction synthesis for hybrid PET-MR scanners: application to brain studies. IEEE Trans Med Imaging. (2014) 33:2332–41. doi: 10.1109/TMI.2014.2340135
93. Burgos N, Cardoso MJ, Thielemans K, Modat M, Dickson J, Schott JM, et al. Multi-contrast attenuation map synthesis for PET/MR scanners: assessment on FDG and florbetapir PET tracers. Eur J Nucl Med Mol Imaging. (2015) 42:1447–58. doi: 10.1007/s00259-015-3082-x
94. Schreibmann E, Nye JA, Schuster DM, Martin DR, Votaw J, Fox T. MR-based attenuation correction for hybrid PET-MR brain imaging systems using deformable image registration. Med Phys. (2010) 37:2101–9. doi: 10.1118/1.3377774
95. Johansson A, Karlsson M, Nyholm T. CT substitute derived from MRI sequences with ultrashort echo time. Med Phys. (2011) 38:2708–14. doi: 10.1118/1.3578928
96. Johansson A, Garpebring A, Asklund T, Nyholm T. CT substitutes derived from MR images reconstructed with parallel imaging. Med Phys. (2014) 41:082302. doi: 10.1118/1.4886766
97. Roy S, Wang W-T, Carass A, Prince JL, Butman JA, Pham DL. PET attenuation correction using synthetic CT from ultrashort echo-time MRI. J Nucl Med. (2014) 55:2071–7. doi: 10.2967/jnumed.114.143958
98. Sekine T, Burgos N, Warnock G, Huellner M, Buck A, Ter Voert EEGW, et al. Multi-atlas-based attenuation correction for brain 18F-FDG PET imaging using a time-of-flight PET/MR scanner: comparison with clinical single-atlas- and CT-based attenuation correction. J Nucl Med. (2016) 57:1258–64. doi: 10.2967/jnumed.115.169045
99. Poynton CB, Chen KT, Chonde DB, Izquierdo-Garcia D, Gollub RL, Gerstner ER, et al. Probabilistic atlas-based segmentation of combined T1-weighted and DUTE MRI for calculation of head attenuation maps in integrated PET/MRI scanners. Am J Nucl Med Mol Imaging. (2014) 4:160–71.
100. Yang X, Wang T, Lei Y, Higgins K, Liu T, Shim H, et al. MRI-based attenuation correction for brain PET/MRI based on anatomic signature and machine learning. Phys Med Biol. (2019) 64:025001. doi: 10.1088/1361-6560/aaf5e0
101. Santos Ribeiro A, Rota Kops E, Herzog H, Almeida P. Hybrid approach for attenuation correction in PET/MR scanners. Nucl Instr Methods Phys Res Sect A Acceler Spectrom Detect Assoc Equip. (2014) 734:166–70. doi: 10.1016/j.nima.2013.09.034
102. Gong K, Yang J, Kim K, Fakhri GE, Seo Y, Li Q. Attenuation correction for brain PET imaging using deep neural network based on Dixon and ZTE MR images. Phys Med Biol. (2018) 63:125011. doi: 10.1088/1361-6560/aac763
103. Ladefoged CN, Marner L, Hindsholm A, Law I, Højgaard L, Andersen FL. Deep learning based attenuation correction of PET/MRI in pediatric brain tumor patients: evaluation in a clinical setting. Front Neurosci. (2019) 12:1005. doi: 10.3389/fnins.2018.01005
104. Han X. MR-based synthetic CT generation using a deep convolutional neural network method. Med Phys. (2017) 44:1408–19. doi: 10.1002/mp.12155
105. Liu F, Jang H, Kijowski R, Bradshaw T, McMillan AB. Deep learning MR imaging-based attenuation correction for PET/MR imaging. Radiology. (2018) 286:676–84. doi: 10.1148/radiol.2017170700
106. Spuhler KD, Gardus J, Gao Y, DeLorenzo C, Parsey R, Huang C. Synthesis of patient-specific transmission data for PET attenuation correction for PET/MRI neuroimaging using a convolutional neural network. J Nucl Med. (2019) 60:555–60. doi: 10.2967/jnumed.118.214320
107. Liu F, Jang H, Kijowski R, Zhao G, Bradshaw T, McMillan AB. A deep learning approach for 18F-FDG PET attenuation correction. EJNMMI Phys. (2018) 5:24. doi: 10.1186/s40658-018-0225-8
108. Torrado-Carvajal A, Vera-Olmos J, Izquierdo-Garcia D, Catalano OA, Morales MA, Margolin J, et al. Dixon-VIBE deep learning (DIVIDE) pseudo-CT synthesis for pelvis PET/MR attenuation correction. J Nucl Med. (2019) 60:429–35. doi: 10.2967/jnumed.118.209288
109. Zhu J-Y, Park T, Isola P, Efros AA. Unpaired image-to-image translation using cycle-consistent adversarial networks. In: 2017 IEEE International Conference on Computer Vision (ICCV). Venice (2017). p. 2242–51. doi: 10.1109/ICCV.2017.244
110. Yang H, Sun J, Carass A, Zhao C, Lee J, Xu Z, et al. Unpaired brain MR-to-CT synthesis using a structure-constrained CycleGAN. In: Stoyanov D, Taylor Z, Carneiro G, Syeda-Mahmood T, Martel A, Maier-Hein L, et al., editors. Deep Learning in Medical Image Analysis and Multimodal Learning for Clinical Decision Support. Lecture Notes in Computer Science. Cham: Springer International Publishing (2018). p. 174–82. doi: 10.1007/978-3-030-00889-5_20
111. Wolterink JM, Dinkla AM, Savenije MHF, Seevinck PR, van den Berg CAT, Išgum I. Deep MR to CT synthesis using unpaired data. In: Tsaftaris SA, Gooya A, Frangi AF, Prince JL, editors. Simulation and Synthesis in Medical Imaging. Lecture Notes in Computer Science. Cham: Springer International Publishing (2017). p. 14–23. doi: 10.1007/978-3-319-68127-6_2
112. Nuyts J, Dupont P, Stroobants S, Benninck R, Mortelmans L, Suetens P. Simultaneous maximum a posteriori reconstruction of attenuation and activity distributions from emission sinograms. IEEE Trans Med Imaging. (1999) 18:393–403. doi: 10.1109/42.774167
113. Censor Y, Gustafson DE, Lent A, Tuy H. A new approach to the emission computerized tomography problem: simultaneous calculation of attenuation and activity coefficients. IEEE Trans Nucl Sci. (1979) 26:2775–9. doi: 10.1109/TNS.1979.4330535
114. Rezaei A, Defrise M, Bal G, Michel C, Conti M, Watson C, et al. Simultaneous reconstruction of activity and attenuation in time-of-flight PET. IEEE Trans Med Imaging. (2012) 31:2224–33. doi: 10.1109/TMI.2012.2212719
115. Salomon A, Goedicke A, Schweizer B, Aach T, Schulz V. Simultaneous reconstruction of activity and attenuation for PET/MR. IEEE Trans Med Imaging. (2011) 30:804–13. doi: 10.1109/TMI.2010.2095464
116. Mehranian A, Zaidi H. Joint estimation of activity and attenuation in whole-body TOF PET/MRI using constrained gaussian mixture models. IEEE Trans Med Imaging. (2015) 34:1808–21. doi: 10.1109/TMI.2015.2409157
117. Rezaei A, Defrise M, Nuyts J. ML-reconstruction for TOF-PET with simultaneous estimation of the attenuation factors. IEEE Trans Med Imaging. (2014) 33:1563–72. doi: 10.1109/TMI.2014.2318175
118. Ahn S, Cheng L, Shanbhag DD, Qian H, Kaushik SS, Jansen FP, et al. Joint estimation of activity and attenuation for PET using pragmatic MR-based prior: application to clinical TOF PET/MR whole-body data for FDG and non-FDG tracers. Phys Med Biol. (2018) 63:045006. doi: 10.1088/1361-6560/aaa8a6
119. Hwang D, Kim KY, Kang SK, Seo S, Paeng JC, Lee DS, et al. Improving the accuracy of simultaneously reconstructed activity and attenuation maps using deep learning. J Nucl Med. (2018) 59:1624–9. doi: 10.2967/jnumed.117.202317
120. Mollet P, Keereman V, Clementel E, Vandenberghe S. Simultaneous MR-compatible emission and transmission imaging for PET using time-of-flight information. IEEE Trans Med Imaging. (2012) 31:1734–42. doi: 10.1109/TMI.2012.2198831
121. Mollet P, Keereman V, Bini J, Izquierdo-Garcia D, Fayad ZA, Vandenberghe S. Improvement of attenuation correction in time-of-flight PET/MR imaging with a positron-emitting source. J Nucl Med. (2014) 55:329–36. doi: 10.2967/jnumed.113.125989
122. Watson CC. Supplemental transmission method for improved PET attenuation correction on an integrated MR/PET. Nucl Instr Methods Phys Res Sect A Acceler Spectrom Detect Assoc Equip. (2014) 734:191–5. doi: 10.1016/j.nima.2013.08.068
123. Bowen SL, Fuin N, Levine MA, Catana C. Transmission imaging for integrated PET-MR systems. Phys Med Biol. (2016) 61:5547–68. doi: 10.1088/0031-9155/61/15/5547
124. Rothfuss H, Panin V, Moor A, Young J, Hong I, Michel C, et al. LSO background radiation as a transmission source using time of flight. Phys Med Biol. (2014) 59:5483–500. doi: 10.1088/0031-9155/59/18/5483
125. Berker Y, Kiessling F, Schulz V. Scattered PET data for attenuation-map reconstruction in PET/MRI. Med Phys. (2014) 41:102502. doi: 10.1118/1.4894818
126. Navarro de Lara LI, Frass-Kriegl R, Renner A, Sieg J, Pichler M, Bogner T, et al. Design, implementation, and evaluation of a head and neck MRI RF array integrated with a 511 keV transmission source for attenuation correction in PET/MR. Sensors (Basel). (2019) 19:E3297. doi: 10.3390/s19153297
127. Renner A, Rausch I, Cal Gonzalez J, Frass-Kriegl R, de Lara LN, Sieg J, et al. A head coil system with an integrated orbiting transmission point source mechanism for attenuation correction in PET/MRI. Phys Med Biol. (2018) 63:225014. doi: 10.1088/1361-6560/aae9a9
128. Boellaard R, Hofman MBM, Hoekstra OS, Lammertsma AA. Accurate PET/MR quantification using time of flight MLAA image reconstruction. Mol Imaging Biol. (2014) 16:469–77. doi: 10.1007/s11307-013-0716-x
129. Mehranian A, Zaidi H. Impact of time-of-flight PET on quantification errors in MR imaging-based attenuation correction. J Nucl Med. (2015) 56:635–41. doi: 10.2967/jnumed.114.148817
130. Conti M. Why is TOF PET reconstruction a more robust method in the presence of inconsistent data? Phys Med Biol. (2011) 56:155–68. doi: 10.1088/0031-9155/56/1/010
131. Bal H, Panin VY, Platsch G, Defrise M, Hayden C, Hutton C, et al. Evaluation of MLACF based calculated attenuation brain PET imaging for FDG patient studies. Phys Med Biol. (2017) 62:2542–58. doi: 10.1088/1361-6560/aa5e99
132. Benoit D, Ladefoged CN, Rezaei A, Keller SH, Andersen FL, Højgaard L, et al. Optimized MLAA for quantitative non-TOF PET/MR of the brain. Phys Med Biol. (2016) 61:8854–74. doi: 10.1088/1361-6560/61/24/8854
133. Cabello J, Lukas M, Rota Kops E, Ribeiro A, Shah NJ, Yakushev I, et al. Comparison between MRI-based attenuation correction methods for brain PET in dementia patients. Eur J Nucl Med Mol Imaging. (2016) 43:2190–200. doi: 10.1007/s00259-016-3394-5
134. Catana C, Quick HH, Zaidi H. Current commercial techniques for MRI-guided attenuation correction are insufficient and will limit the wider acceptance of PET/MRI technology in the clinic. Med Phys. (2018) 45:4007–10. doi: 10.1002/mp.12963
135. Boellaard R, Rausch I, Beyer T, Delso G, Yaqub M, Quick HH, et al. Quality control for quantitative multicenter whole-body PET/MR studies: a NEMA image quality phantom study with three current PET/MR systems. Med Phys. (2015) 42:5961–9. doi: 10.1118/1.4930962
136. Brendle C, Schmidt H, Oergel A, Bezrukov I, Mueller M, Schraml C, et al. Segmentation-based attenuation correction in positron emission tomography/magnetic resonance: erroneous tissue identification and its impact on positron emission tomography interpretation. Invest Radiol. (2015) 50:339–46. doi: 10.1097/RLI.0000000000000131
137. Rausch I, Rischka L, Ladefoged CN, Furtner J, Fenchel M, Hahn A, et al. PET/MRI for oncologic brain imaging: a comparison of standard MR-based attenuation corrections with a model-based approach for the Siemens mMR PET/MR system. J Nucl Med. (2017) 58:1519–25. doi: 10.2967/jnumed.116.186148
138. Schulz V, Torres-Espallardo I, Renisch S, Hu Z, Ojha N, Börnert P, et al. Automatic, three-segment, MR-based attenuation correction for whole-body PET/MR data. Eur J Nucl Med Mol Imaging. (2011) 38:138–52. doi: 10.1007/s00259-010-1603-1
139. Werner P, Rullmann M, Bresch A, Tiepolt S, Jochimsen T, Lobsien D, et al. Impact of attenuation correction on clinical [(18)F]FDG brain PET in combined PET/MRI. EJNMMI Res. (2016) 6:47. doi: 10.1186/s13550-016-0200-0
140. Franceschi AM, Abballe V, Raad RA, Nelson A, Jackson K, Babb J, et al. Visual detection of regional brain hypometabolism in cognitively impaired patients is independent of positron emission tomography-magnetic resonance attenuation correction method. World J Nucl Med. (2018) 17:188. doi: 10.4103/wjnm.WJNM_61_17
141. Øen SK, Keil TM, Berntsen EM, Aanerud JF, Schwarzlmüller T, Ladefoged CN, et al. Quantitative and clinical impact of MRI-based attenuation correction methods in [18F]FDG evaluation of dementia. EJNMMI Res. (2019) 9:83. doi: 10.1186/s13550-019-0553-2
142. Rausch I, Zitterl A, Berroterán-Infante N, Rischka L, Prayer D, Fenchel M, et al. Dynamic [18F]FET-PET/MRI using standard MRI-based attenuation correction methods. Eur Radiol. (2019) 29:4276–85. doi: 10.1007/s00330-018-5942-9
143. Yang J, Jian Y, Jenkins N, Behr SC, Hope TA, Larson PEZ, et al. Quantitative evaluation of atlas-based attenuation correction for brain PET in an integrated time-of-flight PET/MR imaging system. Radiology. (2017) 284:169–79. doi: 10.1148/radiol.2017161603
144. Hirano Y, Koshino K, Iida H. Influences of 3D PET scanner components on increased scatter evaluated by a Monte Carlo simulation. Phys Med Biol. (2017) 62:4017–30. doi: 10.1088/1361-6560/aa6644
145. Accorsi R, Adam L-E, Werner ME, Karp JS. Optimization of a fully 3D single scatter simulation algorithm for 3D PET. Phys Med Biol. (2004) 49:2577–98. doi: 10.1088/0031-9155/49/12/008
146. Watson CC, Newport D, Casey ME. A single scatter simulation technique for scatter correction in 3D PET. In: Three-Dimensional Image Reconstruction in Radiology and Nuclear Medicine. (1996). p. 255–68. doi: 10.1007/978-94-015-8749-5_18
147. Ollinger JM. Model-based scatter correction for fully 3D PET. Phys Med Biol. (1996) 41:153–76. doi: 10.1088/0031-9155/41/1/012
148. Kim KS, Son YD, Cho ZH, Ra JB, Ye JC. Ultra-fast hybrid CPU–GPU multiple scatter simulation for 3-D PET. IEEE J Biomed Health Inform. (2014) 18:148–56. doi: 10.1109/JBHI.2013.2267016
149. Magota K, Shiga T, Asano Y, Shinyama D, Ye J, Perkins AE, et al. Scatter correction with combined single-scatter simulation and Monte Carlo simulation scaling improved the visual artifacts and quantification in 3-dimensional brain PET/CT imaging with 15O-gas inhalation. J Nucl Med. (2017) 58:2020–5. doi: 10.2967/jnumed.117.193060
150. Ma B, Gaens M, Caldeira L, Bert J, Lohmann P, Tellmann L, et al. Scatter correction based on GPU-accelerated Full Monte Carlo simulation for Brain PET/MRI. IEEE Trans Med Imaging. (2019) 39:140–51. doi: 10.1109/TMI.2019.2921872
151. Zaidi H. Quantitative Analysis of Nuclear Medicine Images. New York, NY: Springer (2007). p. 205–35. doi: 10.1007/b107410
152. Bendriem B, Townsend DW. The Theory and Practice of 3D PET. Kluwer Academic Publishers (1998). doi: 10.1007/978-94-017-3475-2
153. Valk PE, Bailey DL, Townsend DW, Maisey MN editors. Positron Emission Tomography: Basic Science and Clinical Practice. London: Springer (2003). p. 115–46.
154. Zaidi H. Scatter modelling and correction strategies in fully 3-D PET. Nucl Med Commun. (2001) 22:1181–4. doi: 10.1097/00006231-200111000-00003
155. Grootoonk S, Spinks TJ, Sashin D, Spyrou NM, Jones T. Correction for scatter in 3D brain PET using a dual energy window method. Phys Med Biol. (1996) 41:2757–74. doi: 10.1088/0031-9155/41/12/013
156. Bergstrom M, Eriksson L, Bohm C, Blomqvist G, Litton J. Correction for scattered radiation in a ring detector positron camera by integral transformation of the projections. J Comput Assist Tomogr. (1983) 7:42–50. doi: 10.1097/00004728-198302000-00008
157. Bailey DL, Meikle SR. A convolution-subtraction scatter correction method for 3D PET. Phys Med Biol. (1994) 39:411–24. doi: 10.1088/0031-9155/39/3/009
158. Levin CS, Dahlbom M, Hoffman EJ. A Monte Carlo correction for the effect of Compton scattering in 3-D PET brain imaging. IEEE Trans Nucl Sci. (1995) 42:1181–8. doi: 10.1109/23.467880
159. Adam LE, Karp JS, Freifelder R. Scatter correction using a dual energy window technique for 3D PET with NaI(Tl) detectors. In: 1998 IEEE Nuclear Science Symposium Conference Record 1998 IEEE Nuclear Science Symposium and Medical Imaging Conference. Toronto, ON (1998). p. 2011–8.
160. Barney JS, Rogers JG, Harrop R, Hoverath H. Object shape dependent scatter simulations for PET. IEEE Trans Nucl Sci. (1991) 38:719–25. doi: 10.1109/23.289380
161. Chen CH, Muzic RF Jr., Nelson AD, Adler LP. A non-linear spatially variant object-dependent system model for prediction of partial volume effects and scatter in PET. IEEE Trans Med Imaging. (1998) 17:214–27. doi: 10.1109/42.700733
162. Cherry SR, Huang S-C. Effects of scatter on model parameter estimates in 3D PET studies of the human brain. IEEE Trans Nucl Sci. (1995) 42:1174–9. doi: 10.1109/23.467730
163. Zaidi H. Comparative evaluation of scatter correction techniques in 3D positron emission tomography. Eur J Nucl Med. (2000) 27:1813–26. doi: 10.1007/s002590000385
164. Adam LE, Karp JS, Brix G. Investigation of scattered radiation in 3D whole-body positron emission tomography using Monte Carlo simulations. Phys Med Biol. (1999) 44:2879–95. doi: 10.1088/0031-9155/44/12/302
165. Holdsworth CH, Levin CS, Janecek M, Dahlbom M, Hoffman EJ. Performance analysis of an improved 3-D PET Monte Carlo simulation and scatter correction. IEEE Trans Nucl Sci. (2002) 49:83–9. doi: 10.1109/TNS.2002.998686
166. Gaens M, Bert J, Pietrzyk U, Autret A, Shah NJ, Visvikis D. GPU-accelerated Monte Carlo based scatter correction in brain PET/MR. EJNMMI Phys. (2014) 1:A32. doi: 10.1186/2197-7364-1-S1-A32
167. Watson CC, Casey ME, Michel C, Bendriem B. Advances in scatter correction for 3D PET/CT. In: IEEE Symposium Conference Record Nuclear Science. Rome (2004). p. 3008–12.
168. Wollenweber SD. Parameterization of a model-based 3-D PET scatter correction. IEEE Trans Nucl Sci. (2002) 49:722–7. doi: 10.1109/TNS.2002.1039554
169. Iatrou M, Manjeshwar RM, Ross SG, Thielemans K, Stearns CW. 3D implementation of scatter estimation in 3D PET. In: 2006 IEEE Nuclear Science Symposium Conference Record. San Diego, CA (2006). p. 2142–5. doi: 10.1109/NSSMIC.2006.354338
170. Iatrou M, Manjeshwar RM, Stearns CW. Comparison of two 3D implementations of TOF scatter estimation in 3D PET. In: 2007 IEEE Nuclear Science Symposium Conference Record. Honolulu, HI (2007). p. 3474–7. doi: 10.1109/NSSMIC.2007.4436878
171. Watson CC, Newport D, Casey ME, deKemp RA, Beanlands RS, Schmand M. Evaluation of simulation-based scatter correction for 3-D PET cardiac imaging. IEEE Trans Nucl Sci. (1997) 44:90–7. doi: 10.1109/23.554831
172. Watson CC. New, faster, image-based scatter correction for 3D PET. In: 1999 IEEE Nuclear Science Symposium Conference Record 1999 Nuclear Science Symposium and Medical Imaging Conference (Cat No99CH37019). Seattle, WA (1999). p. 1637–41.
173. Watson CC. Extension of single scatter simulation to scatter correction of time-of-flight PET. IEEE Trans Nucl Sci. (2007) 54:1679–86. doi: 10.1109/TNS.2007.901227
174. Watson CC, Hu J, Zhou C. Extension of the SSS PET scatter correction algorithm to include double scatter. In: 2018 IEEE Nuclear Science Symposium and Medical Imaging Conference Proceedings (NSS/MIC). Sydney, NSW (2018). p. 1–4. doi: 10.1109/NSSMIC.2018.8824475
175. Werner ME, Surti S, Karp JS. Implementation and evaluation of a 3D PET single scatter simulation with TOF modeling. In: IEEE Nuclear Science Symposium Conference Record. San Diego, CA (2006). p. 1768–73. doi: 10.1109/NSSMIC.2006.354238
176. Tsoumpas C, Aguiar P, Ros D, Dikaios N, Thielemans K. Scatter simulation including double scatter. In: IEEE Nuclear Science Symposium Conference Record. Fajardo (2005). p. 5–1619.
177. Rezaei A, Salvo K, Vahle T, Panin V, Casey M, Boada F, et al. Plane-dependent ML scatter scaling: 3D extension of the 2D simulated single scatter (SSS) estimate. Phys Med Biol. (2017) 62:6515–31. doi: 10.1088/1361-6560/aa7a8c
178. Hori Y, Hirano Y, Koshino K, Moriguchi T, Iguchi S, Yamamoto A, et al. Validity of using a 3-dimensional PET scanner during inhalation of (15)O-labeled oxygen for quantitative assessment of regional metabolic rate of oxygen in man. Phys Med Biol. (2014) 59:5593–609. doi: 10.1088/0031-9155/59/18/5593
179. Polycarpou I, Thielemans K, Manjeshwar R, Aguiar P, Marsden PK, Tsoumpas C. Comparative evaluation of scatter correction in 3D PET using different scatter-level approximations. Ann Nucl Med. (2011) 25:643–9. doi: 10.1007/s12149-011-0514-y
180. Nikulin P, Maus J, Hofheinz F, Lougovski A, van den Hoff J. Time efficient scatter correction for time-of-flight PET: the immediate scatter approximation. Phys Med Biol. (2019) 64:075005. doi: 10.1088/1361-6560/ab0e9b
181. Qian H, Rui X, Ahn S. Deep learning models for PET scatter estimations. In: 2017 IEEE Nuclear Science Symposium and Medical Imaging Conference (NSS/MIC). Atlanta, GA (2017). p. 1–5. doi: 10.1109/NSSMIC.2017.8533103
182. Berker Y, Maier J, Kachelrieß M. Deep scatter estimation in PET: fast scatter correction using a convolutional neural network. In: 2018 IEEE Nuclear Science Symposium and Medical Imaging Conference Proceedings (NSS/MIC). (2018). p. 1–5. doi: 10.1109/NSSMIC.2018.8824594
183. Yang J, Park D, Gullberg GT, Seo Y. Joint correction of attenuation and scatter in image space using deep convolutional neural networks for dedicated brain 18F-FDG PET. Phys Med Biol. (2019) 64:075019. doi: 10.1088/1361-6560/ab0606
184. Okazawa H, Tsujikawa T, Higashino Y, Kikuta K-I, Mori T, Makino A, et al. No significant difference found in PET/MRI CBF values reconstructed with CT-atlas-based and ZTE MR attenuation correction. EJNMMI Res. (2019) 9:26. doi: 10.1186/s13550-019-0494-9
185. Cabello J, Avram M, Brandl F, Mustafa M, Scherr M, Leucht C, et al. Impact of non-uniform attenuation correction in a dynamic [18F]-FDOPA brain PET/MRI study. EJNMMI Res. (2019) 9:77. doi: 10.1186/s13550-019-0547-0
186. Mansur A, Newbould R, Searle GE, Redstone C, Gunn RN, Hallett WA. PET- MR attenuation correction in dynamic brain pet using [11C]cimbi-36: a direct comparison with PET-CT. IEEE Trans Rad Plasma Med Sci. (2018) 2:483–9. doi: 10.1109/TRPMS.2018.2852558
187. Lassen ML, Muzik O, Beyer T, Hacker M, Ladefoged CN, Cal-González J, et al. Reproducibility of quantitative brain imaging using a PET-only and a combined PET/MR system. Front Neurosci. (2017) 11:396. doi: 10.3389/fnins.2017.00396
188. Zhu Y, Zhu X. MRI-driven PET image optimization for neurological applications. Front Neurosci. (2019) 13:782. doi: 10.3389/fnins.2019.00782
189. Khalighi MM, Deller TW, Fan AP, Gulaka PK, Shen B, Singh P, et al. Image-derived input function estimation on a TOF-enabled PET/MR for cerebral blood flow mapping. J Cereb Blood Flow Metab. (2018) 38:126–35. doi: 10.1177/0271678X17691784
190. Jochimsen TH, Zeisig V, Schulz J, Werner P, Patt M, Patt J, et al. Fully automated calculation of image-derived input function in simultaneous PET/MRI in a sheep model. EJNMMI Phys. (2016) 3:2. doi: 10.1186/s40658-016-0139-2
191. Sundar LK, Muzik O, Rischka L, Hahn A, Rausch I, Lanzenberger R, et al. Towards quantitative [18F]FDG-PET/MRI of the brain: automated MR-driven calculation of an image-derived input function for the non-invasive determination of cerebral glucose metabolic rates. J Cereb Blood Flow Metab. (2019) 39:1516–30. doi: 10.1177/0271678X18776820
192. Shiyam Sundar LK, Muzik O, Rischka L, Hahn A, Lanzenberger R, Hienert M, et al. The promise of fully-integrated PET/MR imaging: non-invasive clinical quantification of cerebral glucose metabolism. J Nucl Med. (2019). doi: 10.2967/jnumed.119.229567. [Epub ahead of print].
193. Poulin E, Lebel R, Croteau E, Blanchette M, Tremblay L, Lecomte R, et al. Conversion of arterial input functions for dual pharmacokinetic modeling using Gd-DTPA/MRI and 18F-FDG/PET. Magn Reson Med. (2013) 69:781–92. doi: 10.1002/mrm.24318
194. Ssali T, Anazodo UC, Thiessen JD, Prato FS, Lawrence KS. A noninvasive method for quantifying cerebral blood flow by hybrid PET/MRI. J Nucl Med. (2018) 59:1329–34. doi: 10.2967/jnumed.117.203414
195. Scott CJ, Jiao J, Melbourne A, Burgos N, Cash DM, De Vita E, et al. Reduced acquisition time PET pharmacokinetic modelling using simultaneous ASL–MRI: proof of concept. J Cereb Blood Flow Metab. (2019) 39:2419–32. doi: 10.1177/0271678X18797343
196. Catana C, Drzezga A, Heiss W-D, Rosen BR. PET/MRI for neurologic applications. J Nucl Med. (2012) 53:1916–25. doi: 10.2967/jnumed.112.105346
197. Cecchin D, Palombit A, Castellaro M, Silvestri E, Bui F, Barthel H, et al. Brain PET and functional MRI: why simultaneously using hybrid PET/MR systems? Q J Nucl Med Mol Imaging. (2017) 61:345–59. doi: 10.23736/S1824-4785.17.03008-4
198. Hope TA, Fayad ZA, Fowler KJ, Holley D, Iagaru A, McMillan AB, et al. Summary of the first ISMRM–SNMMI workshop on PET/MRI: applications and limitations. J Nucl Med. (2019) 60:1340–6. doi: 10.2967/jnumed.119.227231
199. Miller-Thomas MM, Benzinger TLS. Neurologic applications of PET/MR imaging. Magn Reson Imaging Clin N Am. (2017) 25:297–313. doi: 10.1016/j.mric.2016.12.003
200. Tondo G, Esposito M, Dervenoulas G, Wilson H, Politis M, Pagano G. Hybrid PET-MRI applications in movement disorders. Int Rev Neurobiol. (2019) 144:211–57. doi: 10.1016/bs.irn.2018.10.003
201. Zaharchuk G. Next generation research applications for hybrid PET/MR and PET/CT imaging using deep learning. Eur J Nucl Med Mol Imaging. (2019) 46:2700–7. doi: 10.1007/s00259-019-04374-9
202. Purz S, Sabri O, Viehweger A, Barthel H, Kluge R, Sorge I, et al. Potential pediatric applications of PET/MR. J Nucl Med. (2014) 55:32S−9S. doi: 10.2967/jnumed.113.129304
203. Bezrukov I, Schmidt H, Gatidis S, Mantlik F, Schäfer JF, Schwenzer N, et al. Quantitative evaluation of segmentation- and atlas-based attenuation correction for pet/MR on pediatric patients. J Nucl Med. (2015) 56:1067–74. doi: 10.2967/jnumed.114.149476
204. Marner L, Henriksen OM, Lundemann M, Larsen VA, Law I. Clinical PET/MRI in neuro-oncology: opportunities and challenges from a single-institution perspective. Clin Transl Imaging. (2017) 5:135–49. doi: 10.1007/s40336-016-0213-8
205. Marner L, Nysom K, Sehested A, Borgwardt L, Mathiasen R, Henriksen OM, et al. Early postoperative 18F-FET PET/MRI for pediatric brain and spinal cord tumors. J Nucl Med. (2019) 60:1053–8. doi: 10.2967/jnumed.118.220293
206. Gatidis S, Bender B, Reimold M, Schäfer JF. PET/MRI in children. Eur J Radiol. (2017) 94:A64–70. doi: 10.1016/j.ejrad.2017.01.018
207. Kwatra NS, Lim R, Gee MS, States LJ, Vossough A, Lee EY. PET/MR imaging: current updates on pediatric applications. Magn Reson Imaging Clin N Am. (2019) 27:387–407. doi: 10.1016/j.mric.2019.01.012
208. Lee YZ, Oldan JD, Fordham LA. Pediatric applications of hybrid PET/MR imaging. Magn Reson Imaging Clin N Am. (2017) 25:367–75. doi: 10.1016/j.mric.2016.12.005
209. Andersen JB, Lindberg U, Olesen OV, Benoit D, Ladefoged CN, Larsson HB, et al. Hybrid PET/MRI imaging in healthy unsedated newborn infants with quantitative rCBF measurements using 15O-water PET. J Cereb Blood Flow Metab. (2019) 39:782–93. doi: 10.1177/0271678X17751835
210. Thorwarth D, Leibfarth S, Mönnich D. Potential role of PET/MRI in radiotherapy treatment planning. Clin Transl Imaging. (2013) 1:45–51. doi: 10.1007/s40336-013-0006-2
211. Winter RM, Leibfarth S, Schmidt H, Zwirner K, Mönnich D, Welz S, et al. Assessment of image quality of a radiotherapy-specific hardware solution for PET/MRI in head and neck cancer patients. Radiother Oncol. (2018) 128:485–91. doi: 10.1016/j.radonc.2018.04.018
212. Paulus DH, Quick HH. Hybrid positron emission tomography/magnetic resonance imaging: challenges, methods, and state of the art of hardware component attenuation correction. Invest Radiol. (2016) 51:624–34. doi: 10.1097/RLI.0000000000000289
213. Witoszynskyj S, Andrzejewski P, Georg D, Hacker M, Nyholm T, Rausch I, et al. Attenuation correction of a flat table top for radiation therapy in hybrid PET/MR using CT- and 68Ge/68Ga transmission scan-based μ-maps. Phys Med. (2019) 65:76–83. doi: 10.1016/j.ejmp.2019.08.005
214. Edmund JM, Nyholm T. A review of substitute CT generation for MRI-only radiation therapy. Radiat Oncol. (2017) 12:28. doi: 10.1186/s13014-016-0747-y
215. Johnstone E, Wyatt JJ, Henry AM, Short SC, Sebag-Montefiore D, Murray L, et al. Systematic review of synthetic computed tomography generation methodologies for use in magnetic resonance imaging-only radiation therapy. Int J Radiat Oncol Biol Phys. (2018) 100:199–217. doi: 10.1016/j.ijrobp.2017.08.043
Keywords: positron emission tomography/magnetic resonance imaging, attenuation correction, scatter correction, positron emission tomography image quantification, magnetic resonance-based attenuation correction
Citation: Teuho J, Torrado-Carvajal A, Herzog H, Anazodo U, Klén R, Iida H and Teräs M (2020) Magnetic Resonance-Based Attenuation Correction and Scatter Correction in Neurological Positron Emission Tomography/Magnetic Resonance Imaging—Current Status With Emerging Applications. Front. Phys. 7:243. doi: 10.3389/fphy.2019.00243
Received: 25 October 2019; Accepted: 19 December 2019;
Published: 29 January 2020.
Edited by:
Ivo Rausch, Medical University of Vienna, AustriaReviewed by:
Roberta Frass-Kriegl, Medical University of Vienna, AustriaDavid B. Stout, Independent Researcher, Culver City, United States
Copyright © 2020 Teuho, Torrado-Carvajal, Herzog, Anazodo, Klén, Iida and Teräs. This is an open-access article distributed under the terms of the Creative Commons Attribution License (CC BY). The use, distribution or reproduction in other forums is permitted, provided the original author(s) and the copyright owner(s) are credited and that the original publication in this journal is cited, in accordance with accepted academic practice. No use, distribution or reproduction is permitted which does not comply with these terms.
*Correspondence: Jarmo Teuho, amFybW8udGV1aG8mI3gwMDA0MDt0eWtzLmZp