- 1Tianjin Key Laboratory of Intelligence Computing and Novel Software Technology, Tianjin University of Technology, Tianjin, China
- 2Key Laboratory of Computer Vision and System, Ministry of Education, Tianjin University of Technology, Tianjin, China
- 3Faculty of Natural Sciences and Mathematics, University of Maribor, Maribor, Slovenia
- 4Center for Applied Mathematics and Theoretical Physics, University of Maribor, Maribor, Slovenia
- 5Complexity Science Hub Vienna, Vienna, Austria
Voluntary participation, as an effective mechanism to promote cooperation in game theory, has been widely concerned. In the meanwhile, reciprocal rewarding plays an important role in motivating individual initiative. Inspired by this phenomenon, we investigate the effect of reciprocal rewarding on the evolutionary cooperation in spatial social dilemmas, including prisoner's dilemma game and the snowdrift game with voluntary participation. In our model, a cooperative individual fitness will be redefined if one could obtain additional incentive bonus which is proportional to the number of cooperative neighbors. Moreover, each individual is a pure strategist in the spatial structured population and could only choose one of three strategies—cooperation, defection and being a loner. Through numerical simulations, we have confirmed that, compared with the traditional situation, reciprocal rewarding and the payoff of loner can significantly promote the cooperative behavior among the population, and the greater the contribution of reciprocal rewarding/payoff of loner, the more obvious the promoting effect on cooperation. In addition, we also find that there is a condition for loner to make the system fall into the three-strategy cyclic dominance, that is, the payoff of loner can not be too small or too large, which will destroy the situation of cyclic dominance. With regard to these results, it is strongly unveiled that reciprocal rewarding has a positive role to resolve the social dilemmas in the evolution of cooperation.
1. Introduction
Cooperation, as a ubiquitous phenomenon in nature and human society, is the internal driving force of species evolution and social development [1–3], and considered as another evolutionary criterion after natural selection and gene mutation, which is obviously against Darwin's theory of evolution and natural selection [4]. Thus, it is of great significance to explain the maintenance and emergence of cooperative behaviors among selfish and unrelated individuals, which has attracted extensive attention from scholars in the field of natural and social science [5–12].
Over the last few decades, evolutionary game theory [13], combining game theory with dynamical analysis, has provided a simple and forceful mathematical framework to describe and analyze the conflict of interest among selfish and unrelated individuals as social conflict is similar to the competition of individuals for limited resources. In particular, the prisoner's dilemma game (PDG) [14, 15] and snowdrift game (SDG) [16, 17], as the simplest models, represent different social dilemmas and mode of conflicts, which endow typical paradigms to explain the persistence and emergence of cooperation among selfish individuals, and have achieved a series of fruitful results [18–24] [see references [25, 26] for more recent information]. In the traditional PDG and SDG, it is known that two involved individuals must simultaneously decide either to cooperate or to defect without knowing the choice of the opponent in the processes of the game. They will both gain reward R for mutual cooperation and punishment P for mutual defection. However, if they choose different strategies, the cooperator gets the sucker's payoff S, while the defector obtains the temptation T. As a standard practice, these payoffs satisfy the ranking T > R > P > S and 2R > T + S for PDG. It means that defection always represents the optimal strategy regardless of the opponent's decision, which leads to the tragedy of the commons [27], because private interest and collective welfare are inconsistent.While in the SDG, the payoff ranking must be ordered as T > R > S > P. The slight variation of the payoff ranking results in a significant change of the game dynamics so that the best action for the individual strongly depends on the strategy of his/her opponent.
In the traditional case, all individuals interact equally with each other in an infinitely large, completely unstructured and well-mixed population, where all individuals inevitably fall into mutual defection under the social dynamics [28–30]. However, infinitely large, completely unstructured and well-mixed population could not accurately and truthfully reflect the real-world population structure as it is often not well-mixed [31]. In practice, many individuals hold not only local connections but also long-range links, which has been confirmed by many complex networks in real life and thus inspired the rapid development of network science. Based on this discovery, the combination of evolutionary graph theory and evolutionary game theory opens the way for investigating the emergence and maintenance of cooperative phenomena in biological and social systems [32–34]. In the structured population, a node can only represent one agent and the edges indicate the interactions among individuals. Thus, the individuals located on the vertices are limited to play with their nearest directly neighbors. In consideration of these simplified settings, Nowak and May [35] seminally introduced the PDG into the spatial structured population, which demonstrated that the cooperative individuals, locating on the square lattices, resisted the invasion of defectors by forming tight clusters, so that the cooperation can be greatly promoted. This very important rule of kinetics is referred to as spatiality or network reciprocity, which has attracted the attention of more and more scholars and been extensively and deeply studied in various types of spatial topologies, such as square lattice network [36, 37], small-world network [38, 39], BA scale-free network [40, 41], ER random network [42, 43], multilayer coupling network [44–48], to name but a few. Network topology has been found as a key to the success of evolution of cooperation. Along this line of research, a series of mechanisms from the real world has been introduced into the spatial game to explore the evolution of cooperation, including reputation [49, 50], memory [51], social diversity [52], punishment [53], aspiration [54], and so on. All of these mechanisms promote the emergence and maintenance of cooperation to some extent.
In recent years, an important factor reward, as a novel means to promote the cooperation, has aroused extensive attention [55–58]. Reward, as a means of motivation in real life, is a measure of coruscating people's sense of honor and enterprise, and a management to mobilize the enthusiasm of administrative personal and management counterparts and to explore the potential capacity to the maximum extent. It means that reward has the guiding function of mobilizing individual positive contribution. For instance, when a person makes a contribution to others or a group, in order to encourage more people to follow his/her example, we tend to reward him/her for his/her efforts. Inspired by the phenomenon of self-reflection in real life, Ding [59] explored the effect of self-interaction in which the cooperative individual will gain an additional benefit through self-interaction. It is found that the self-interaction has a positive role in the evolution of cooperation. While Wu et al. [60] further believed that it was not complete to only focus on the cooperative subjects but ignore their opponent's attribute in the rewarding mechanism. In their opinion, the reward must be based on mutual benefit, that is, the additional benefit is the reciprocal rewarding, which showed the model could also greatly promote the evolution of cooperation in the spatial structured population.
However, it is sometimes difficult for two involved individuals to simultaneously decide either to cooperate or defect. For instance, when an individual is in an unfavorable situation, to cooperate will damage his/her own interest while to defect will injure the collective benefit, which makes him/her get into the hot water. Actually, the best way is to let it alone in this scenario. In many cases, in order to avoid risks, some individuals may choose not to participate in the game. In contrast, they begin to pursue the tiny but at least stable earnings according to their own efforts [61]. Thus, we define the risk-averse individuals as the loners (L), who are inclined to voluntarily participate in the social dilemmas when they trap in a disadvantageous situation for themselves, which has been proved to be an effective way to promote and maintain the cooperation in the spatial structured population [62]. In its basic form, individuals may adopt three optional strategies consisting of the cooperation, defection, and going it alone in spatial structured population. Szabó and Hauert [63, 64] firstly introduced the voluntary game into the spatial structured population, and found that the system was trapped in a rock-scissor-paper cyclic dominance due to the risk-averse loners. The cooperative behavior could be maintained. Reference [65] focused on probing into the effect of iterated prisoner's dilemma game with voluntary players on interdependent networks, which showed voluntary participation could remarkably improve the frequency of cooperation. Reference [66] further took the self-interaction into account in the voluntary prisoner's dilemma game and observed the cooperation was significantly enhanced with the increment of additional reward. It is no doubt that voluntary participant has played an important role in promoting the cooperative behavior.
Based on the above discussions, in this paper, we focus on the effect of reciprocal rewarding on iterated PDG and SDG with voluntary participation on the square lattice network, which is different from the previous work [60]. The results indicate the cooperation level can be drastically enhanced if it is compared with the traditional spatial PDG or SDG model. The remainder of the paper is organized as follows. At first, we present the mathematical method and model in section 2. Subsequently, the main simulation results and discussions are shown at great length in section 3. Finally, we summarize our conclusions in section 4.
2. Evolving Game Model
In this section, we will present the improved reciprocal rewarding spatial game model in detail, including iterated PDG and SDG with voluntary participation, which represent different social dilemmas and model conflict and competition.
In order to highlight the effect of cooperative belief and avoid the influence of degree heterogeneity on the game dynamics, we assume a regular L × L square lattice with periodic boundary conditions and von Neumann neighborhood as the topology of the whole game system, where each player can occupy only one lattice site, and has four fixed neighbors to interact and obtain payoff. Initially, each player can randomly choose to be a cooperator (sx = C), a defector (sx = D), or a loner (sx = L) with the equal probability in each game round. The strategy attributes of a player can be expressed by the following vector,
In addition, the fundamental form of payoff matrix of spatial voluntary PDG and voluntary SDG is based on the payoff matrix of traditional PDG and SDG, since the third strategy of loner (L) is appended to the classic PDG and SDG. The risk-averse loners and their opponents always receive a tiny but fixed benefit σ in the voluntary game, where σ ∈ (0, 1). For simplicity but without loss of generality, we consider the weak PDG and simple SDG in our model. Like previous work, the elements of PDG payoff matrix are set as T = b, R = 1 and P = S = 0, so the payoff matrix of spatial voluntary PDG can be described as,
where b ∈ (1, 2) ensures a proper payoff ranking, i.e., T > R > P ≥ S. While for the SDG, the elements of payoff matrix are set as T = 1 + r, R = 1, S = 1 − r and P = 0, so the payoff matrix of spatial voluntary SDG can be simplified as,
where r ∈ (0, 1) denotes the cost-to-benefit ratio of mutual cooperation. Following the common practice [63], we set σ = 0.3 through all this paper both in the PDG and SDG with voluntary participation if not directly stated. It is worth noting that although we choose weak voluntary PDG and simplified voluntary SDG, the corresponding conclusions can be drawn in the strict PDG and SDG with voluntary participation.
In the spatial structured population, each player x could only interact with its four nearest neighbors and acquire cumulative benefits, which can be indicated as,
where Ωx represents the set of nearest neighbors of focal player x.
Under the reciprocal rewarding mechanism of three strategy in iterated PDG and SDG with voluntary participant, whether an individual obtains additional incentive benefit depends not only on his/her own strategy, but also on his/her opponent's strategy. Only when the focal individual and one's neighbors simultaneously adopt cooperative strategy, the former can have a chance to obtain additional incentive income. It is worth emphasizing that each cooperative neighbor corresponds to an extra incentive benefit β for the focal player. The more cooperative neighbors of the focal cooperative individual has, the more additional incentive benefit the focal individual receives. That is to say, the additional incentive income for the focal cooperator is proportional to the number of cooperative neighbors. However, if the focal player adopts other strategies, he/she will get none of additional inventive income no matter what strategies the opponent adopts. Thus, the fitness Fx of the focal player x can be calculated in the following expression,
where n represents the number of cooperative neighbors of the focal cooperator, and β denotes the additional incentive benefit when he/she plays with one of his/her cooperative neighbors. When β = 0, the model is reduced to the traditional form, which means that there is no reciprocal rewarding in the system. In our model, considering the additional reward is tiny, we follow the previous work [60]. Here, we assume the value of β ranging from 0 to 0.5 to investigate how the reciprocal rewarding affects the evolution of cooperation in spatial voluntary PDG and voluntary SDG.
The game is iterated by using Monte Carlo simulations (MCS) procedure composed of the following elementary steps. First, at each time step, player x and his/her neighbor player y are stochastically selected and their fitness accumulated according to Equation (5). Then, we will asynchronously update the strategy of focal player x and decide whether to adopt the strategy from the randomly selected player y with a probability in accordance with Fermi updating rule [67],
where K quantifies the uncertainty during the process of strategy transition, including irrationality and errors. Under the normal circumstance, the strategy of the better-performing player will be adopted. However, there is also the rare exception to adopt the worse-performing strategy. Without loss of generality, we set K = 0.1 through all this paper if not directly stated.
It needs to be pointed out that each player has on average once to update his/her strategy during a full MCS step, which will be finished if the aforementioned two elementary steps have been completed. All numerical simulation results are conducted on a square lattice network with L = 100. We also investigate some larger lattice size (e.g., L = 200 or L = 400) to avoid finite-size effects during our preliminary analysis, and confirm the qualitatively same results can be obtained. To analyze simulation results and further increase the accuracy of the key quantity, the frequency of three strategies are determined by the average values of the last 5 × 103 independent steps after the system reaches a stationary state within total 5 × 104 steps. Moreover, to avoid additional disturbances, the final results are averaged over 30 independent realizations for each set of parameter values to ensure the accuracy.
3. Numerical Simulation Results
In this section, we will discuss the effect of reciprocal rewarding on the evolution of cooperation in spatial voluntary PDG and voluntary SDG from the macroscopic and microscopic level through the results of Monte Carlo simulation.
3.1. Frequency of Three Strategies
We first explore the effects of reciprocal rewarding on the evolution of cooperation behavior by the frequency of three strategies after the system reaches a stable state. Figure 1 shows the stationary frequency of cooperators ρC, frequency of defectors ρD, and frequency of loners ρL as a function of the temptation to defect b in the spatial voluntary PDG or the cost-to-benefit ratio r in the spatial voluntary SDG for different reciprocal rewarding β, in which the first and the second rows indicate the results in spatial voluntary PDG and voluntary SDG, respectively. As we have defined previously, on a regular L × L square lattice with periodic boundary conditions, the cooperator will receive an additional incentive income β as a reward if there is a cooperative neighbor around him/her. Since the additional incentive benefit the cooperator has obtained is in direct proportion to the number of the cooperative neighbors around him/her,for example, the cooperator could gain additional inventive benefits nβ if there are n cooperative neighbors around him/her. In our model, we set the value of β range from 0 to 0.5 to investigate the effect of reciprocal rewarding mechanism on the evolution of cooperation in spatial voluntary PDG and voluntary SDG. Compared with reference [60], we obtain entirely different results no matter what value of the additional reciprocal rewarding β is, i.e., cooperation cannot disappear completely even with the larger defection temptation b or cost-to-benefit ratio r since there are risk-averse loners in the spatial structured population. For β = 0, the system returns the traditional voluntary participation game, in which the frequency of cooperation decreases with the increase of temptation b in voluntary PDG or cost-to-benefit ratio r in voluntary SDG. But thanks to the emergency of risk-averse loners, cooperators could survive in the population, which leads to the co-existence case of cooperators (C), defectors (D), and loners (L). However, when we take the reciprocal rewarding into account, the frequency of cooperation is dramatically enhanced, which means cooperators effectively resist the exploitation of defectors. In particular, as the increment of β, the cooperative level monotonously increases, which indicates that the cooperative behavior is highly promoted by the reciprocal rewarding mechanism. Here, we define two thresholds Cd and Le, which denote cooperators dominate the whole spatial grid and loners emerge, respectively. Furthermore, we can observe from the Figure 1 that the thresholds Cd and Le increase as β grows. Therefore, the reciprocal rewarding mechanism promotes the evolution of cooperation. The larger the contribution of the reciprocal rewarding, the more obvious the promoting effect.
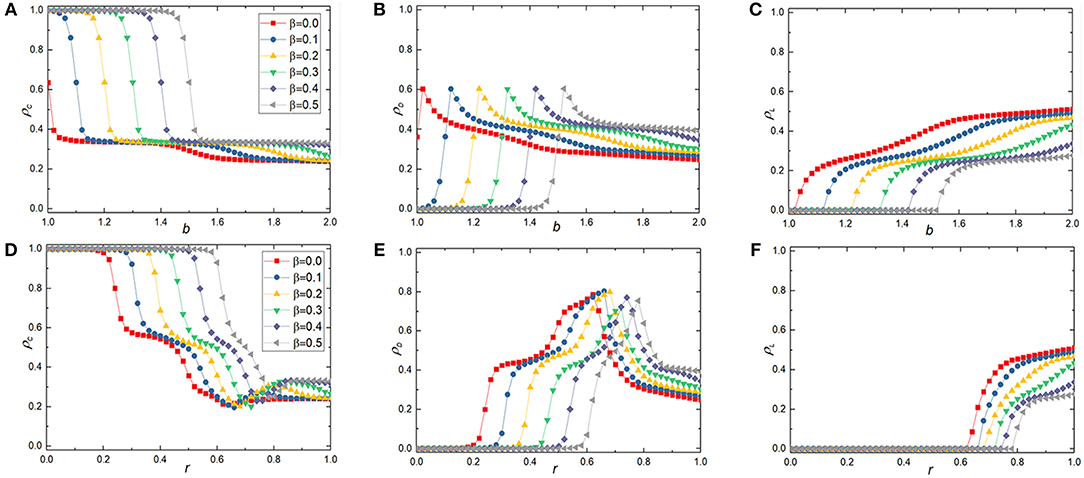
Figure 1. Average frequency of cooperators(ρC), defectors(ρD), loners(ρL) at the stationary sate in dependence on b in the voluntary PDG or r in the voluntary SDG at different reciprocal rewarding strength β on the square lattice. In the top panels [from (A–C)], the simulation results are acquired in the voluntary PDG, while for the bottom panels [from (D–F)], results are obtained in voluntary SDG. In all simulations, other parameters are set to be L = 100, MCS = 5 × 104, σ = 0.3, K = 0.1.
Considering the differences among individuals in the spatial structured population, cooperators may not gain the same additional incentive benefit for interacting with their cooperative neighbors. Therefore, we extend the conditions of reciprocal rewarding to further study the effect of the proposed mechanism on the evolution of cooperation in voluntary game, including in PDG and SDG. In this situation, when a cooperator has a cooperative neighbor around him/her, he/she no longer gains a certain extra incentive benefit but a random number within a uniform distribution range. Moreover, when there are more than one cooperative neighbor around him/her, the cooperator may receive different extra incentive income by interacting with cooperative neighbors. Figure 2 depicts the frequency of cooperators, defectors and loners as a function of the temptation to defect b in spatial voluntary PDG and the cost-to-benefit ratio r in spatial voluntary SDG for different range reciprocal rewarding [0, β], in which the top and the bottom panels indicate the results in spatial voluntary PDG and voluntary SDG, respectively. Although we could get the same qualitative trends as in Figure 1 after the introduction of reciprocal rewarding mechanism. However, the promotion level of cooperation is weakened when compared with the corresponding reciprocal rewarding β. The most intuitive finding is that the thresholds Cd and Le become smaller than those under the situation of certain extra incentive income. It is not difficult to understand this phenomenon if we conduct an in-depth analysis. Under a uniformly distributed interval of reciprocal rewarding mechanism, the extra incentive income to a cooperator may be smaller than the certain reciprocal rewarding if there are cooperative neighbors around him/her in the corresponding case. These results show that the reciprocal rewarding can promote the evolution of cooperation from another aspect, that is, the larger the contribution of the reciprocal rewarding, the more obvious the promoting effect on the evolution of cooperation. In any way, the present model further enriches the reciprocal rewarding mechanism proposed in reference [60].
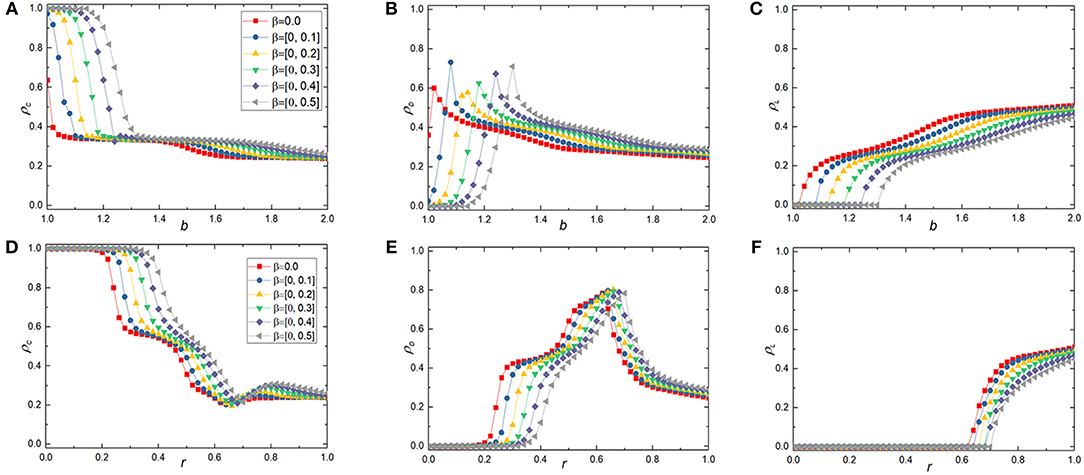
Figure 2. Average frequency of cooperators(ρC), defectors(ρD), loners(ρL) at the stationary sate in dependence on b in the voluntary PDG or r in the voluntary SDG at different random reciprocal rewarding strength [0, β] on square lattice. In the top panels [from (A–C)], the simulation results are acquired in the voluntary PDG, while for the bottom panels [from (D–F)], results are acquired in voluntary SDG. In all simulations, other parameters are set to be L = 100, MCS = 5 × 104, σ = 0.3, K = 0.1.
Actually, it is not hard to clarify the underlying cause that the reciprocal rewarding promotes the evolution of cooperation in spatial voluntary PDG and voluntary SDG. The additional incentive benefits among cooperators improve the payoff of cooperator to a certain degree, which enhances the advantages of cooperators in the process of strategy communication. Thus, under the influence of evolutionary dynamics, cooperative strategy will be easily spread in the spatial structured population. Under the effect of network reciprocity, cooperators can resist the invasion of defectors by forming tight clusters. It needs to be pointed out that in the voluntary SDG, after the frequency of cooperators drops to the lowest state, it will be a slight rise and then gradually decline until it tends to be stable whether it is in the case of certain additional incentive income or an uncertain interval extra incentive income regardless of the value of β. The possible reason is that SDG is not the pure altruistic game and has two different Nash-equilibria which lead to a bistable state. Therefore, we can get different results from the voluntary PDG.
In order to make a comprehensive understanding for the effect of reciprocal rewarding on the evolutionary processes of three strategies in spatial voluntary PDG, we depict the color map encoding of their frequency on the b − β panel in Figure 3. We can clearly observe the fraction of cooperators increases with the augmentation of reciprocal rewarding factor β, but decreases with the increase of temptation b. Individuals gradually change from full cooperators to co-existence of cooperators and defectors and then to cyclic dominance of cooperators, defectors, and loners with the increase of temptation b when the reciprocal rewarding reaches a certain value, which is in accordance with the result of our previous studies, i.e., reciprocal rewarding could dramatically promote the evolution of cooperation. In particular, the greater the reciprocal rewarding, the more obvious the promotion of cooperation.
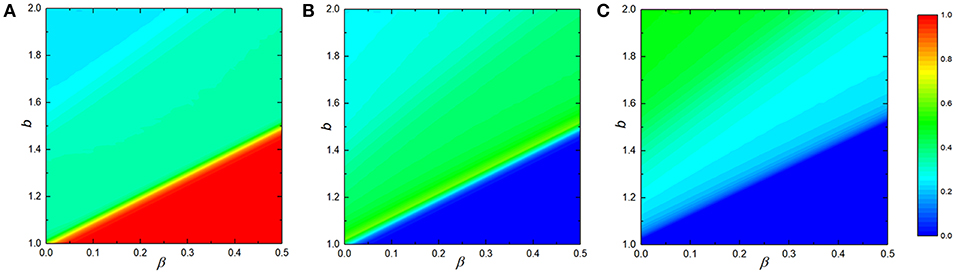
Figure 3. Average frequency of three strategies evolves with the temptation b and reciprocal rewarding strength β at the stationary sate in the voluntary PDG. From (A–C), it represents cooperators, defectors and loners, respectively. In all simulations, other parameters are set to be L = 100, MCS = 5 × 104, σ = 0.3, K = 0.1.
To sum up, through the aforementioned results, we could preliminary prove from a macroscopic perspective that reciprocal rewarding mechanism can ensure the advantage of cooperator in the competition of strategic evolution and significantly improve the cooperative level of spatial structured population.
3.2. Analysis of Strategies Evolution and Strategies Distribution
In order to further understand the reason that the reciprocal rewarding mechanism promotes the evolution of cooperation, we record the time series of three strategies frequency under the given value of temptation b and reciprocal rewarding strength β in Figure 4. In all panels, the temptation b is fixed to be 1.28, while the reciprocal rewarding strength β is set to be 0, 0.2, 0.3, and 0.5, respectively. Taken as a whole, the frequency of defectors always rises at the early stage regardless of any situation, because the defectors' inherent advantage in payoff ensures their expansion. For the traditional case (Figure 4A), the fraction of defectors will firstly reach the highest point because of its best performance, while the other strategies are quite abject. As time step evolves, the increase of defectors provides opportunities for loners to break out, while the decrease of cooperators weakens the advantage of defectors in invasion at the same time. Thus, the frequency of loners starts to rise and the frequency of defectors drops gradually. However, as loner can only obtain tiny but fixed benefits, the strategy of cooperating is superior to the strategy of being a loner. Thus, some loners are ultimately assimilated by cooperators. Then, the fraction of cooperators begins to rise after touching the trough, while the frequency of loners declines after peaking. With abundance of cooperators being exploited, the fraction of defectors rises again. There is no doubt that the system is trapped into what is known as a so-called rock-scissor-paper game, which exhibits the cyclic dominance and lasts a period of time, and then three strategies coexist in a relatively stable state. Although the frequency of defectors is larger than that of cooperators and loners, it ensures the existence of cooperative behavior. Cooperative behavior has been dramatically improved after the reciprocal rewarding is introduced into the system. As β increases, the cyclic dominance of three strategies becomes less obvious until it disappears and three strategies co-exist in the system at last. In regard to β = 0.5, cooperators would even overcome the intrusion of the defectors and dominate the whole spatial structured population while the defectors and the loners have no opportunity to survive. From the microscopic perspective of time series evolution, we prove that the reciprocal rewarding mechanism can significantly promote the cooperative behavior of voluntary PDG. The larger contribution of reciprocal rewarding, the more obvious the promotion of cooperation level.
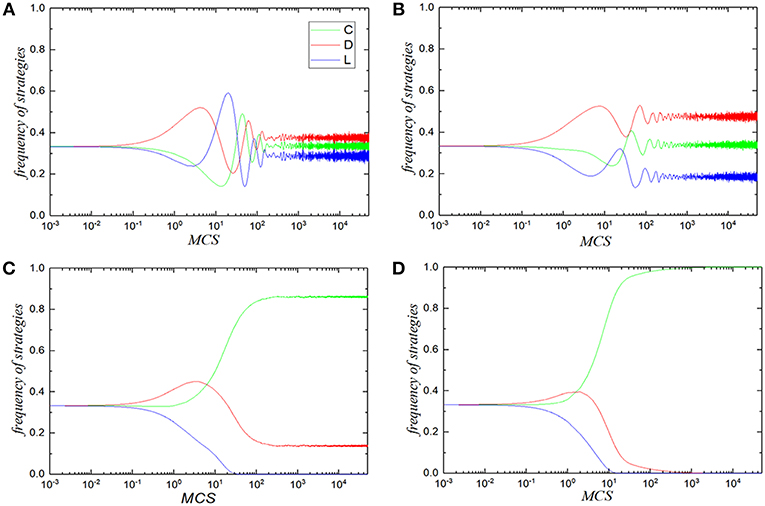
Figure 4. Frequency of three strategies with the spacial structured population at each time step under the specified reciprocal rewarding strength β in the voluntary PDG. Panels (A–D) correspond to β = 0.0 (traditional situation), β = 0.2, β = 0.3, β = 0.5, respectively. In all panels, green, red and blue curves correspond to cooperators, defectors, and loners, respectively. In all simulations, Other parameters are set to be L = 100, MCS = 5 × 104, σ = 0.3, b = 1.28, K = 0.1.
Exploring the spatial distribution and organizational form the three strategies is great significance for us to deeply understand the impact of reciprocal rewarding mechanism on the evolutionary cooperation with the spatial structured population from a microscopic perspective. Figure 5 presents the spatial distribution of three strategies evolving over time under diverse additional incentive income β in spatial voluntary PDG. From top to bottom, the contributions of reciprocal rewarding β to fitness is 0, 0.3, 0.4, and 0.5, while the time step from left to right is equal to 0, 5, 20, and 50,000, respectively. In the initial state, the three strategies—cooperation(C), defection(D), and loner(L)—are randomly distributed in spatial structured population regardless of β. For β = 0 (the first row), it degenerates into the traditional voluntary PDG, in which cooperators could not gain additional incentive income no matter there are cooperative neighbors around them. Thus, the defectors have an unparalleled advantage over the cooperative player so that the defectors invade the cooperators on the edge of the cooperative clusters. It is clear that the clusters of defectors expand while the number of cooperative clusters decreases at the time step 5. To avoid risk and pursue tiny but stable returns, some individuals within the clusters of defection turn to be loners and gather in them. In the meanwhile, the number of cooperators in the system continues to shrink, which further weakens the advantage of defectors. As time goes on, the rapid rise of the loners as powerful clusters deal a heavy blow to the proud hearted defectors, which protects the cluster of cooperators from further being attacked by defectors. So we can observe there are lots of largest clusters of the loners at time step 20. Loners' strategy is at a disadvantage in terms of payoff compared with cooperative individuals, so it is unavoidable that the loners who are on the edge of cooperators gradually become cooperative players. This observation reinforces that defectors are superior to cooperators, and cooperators are better than loners, and loners outperform defectors. Therefore, we can see that three strategies ultimately co-exist in the spatial structured population.
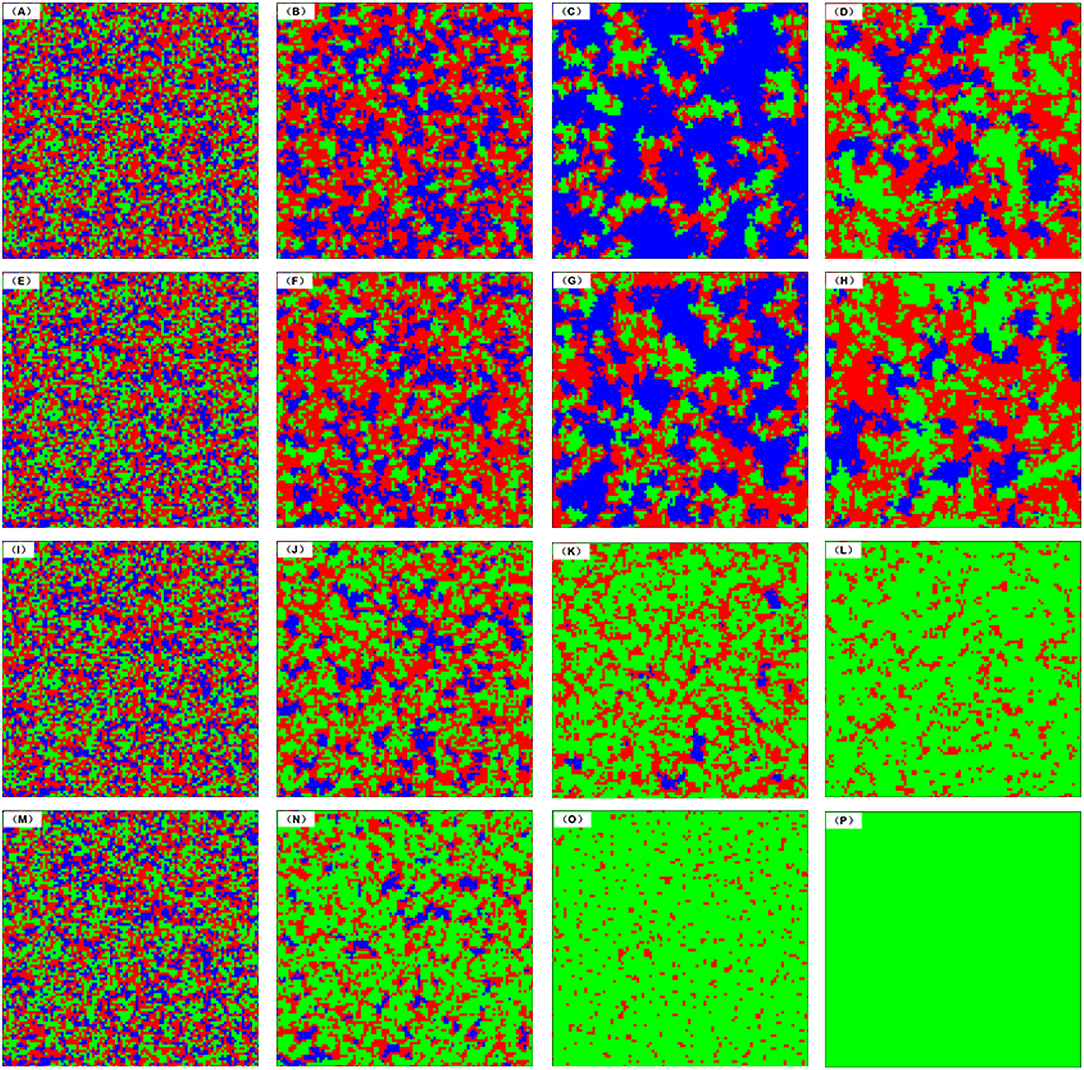
Figure 5. Characteristic snapshots of the spatial distribution of three strategies for different reciprocal rewarding strength β and time step in the voluntary PDG. The row from top to bottom, β takes the value to be 0.0, 0.2, 0.3, and 0.5, respectively. The column from left to right, the snapshots are acquired at MCS steps 0, 5, 20, and 5 × 104, respectively. In all panels, the red dots represent defectors, the green ones express cooperators and the blue ones stand for loners. In all simulations, other parameters are set to be L = 100, MCS = 5 × 104, σ = 0.3, b = 1.28, K = 0.1.
However, if β ≠ 0, it means the reciprocal rewarding is introduced into the system, that is, cooperators can receive addition incentive income if there are some cooperative neighbors around them, which fundamentally changes the ability of cooperative clusters to defend themselves against the invasion of defectors, and the greater contribution of reciprocal rewarding, the stronger their ability. Compared with the traditional situation, when β = 0.2 (the second row), although the cooperative clusters are still attacked by defectors, the case is significantly improved for the number and size of cooperative clusters are obviously better. When β = 0.3 (the third row), the clusters of loner could not get rid of the fate of annihilation by the cluster of cooperation while the defector spreads into the sea of the huge cooperative cluster in the form of scattered little clusters. While β = 0.5 (the last row), cooperators could resist the attack of the defector and dominate the whole spatial structured population at last. These results further demonstrate the significance of reciprocal rewarding in promoting the evolution of voluntary PDG cooperation from a microscopic perspective.
3.3. The Effect of Loner's Benefit σ on the Evolution of Cooperation
As we already argued that loner's benefit can radically change the sate of evolutionary cooperation shaping the famous cyclic dominance of rock-scissor-paper state no matter what value of the additional reciprocal rewarding β is. To gain deeper insights into the effect of loner's payoff σ on the evolution of cooperation, Figure 6 depicts the heat maps of the average of the three strategies in the full b − σ panel for different reciprocal rewarding β. In all situation, the frequency of cooperators monotonously rises with the increase of the loner's benefit for the same temptation to defect b (see the first row of Figure 6). The only difference is that there are scenarios of full cooperation in the spatial structured population after the reciprocal rewarding is introduced, i.e., β ≠ 0. Meanwhile, the greater reciprocal rewarding, the larger of full cooperation area, which is consistent with what we have been talking about, that is, reciprocal rewarding can significantly promote the evolution of cooperation. What has drawn our attention is that the reciprocal rewarding and the payoff of loner do not promote the evolution of cooperation at the same time. However, we also can observe a very interesting phenomenon, that is, the famous cyclic dominance of rock-scissor-paper state is broken when the loner's payoff are at the minimum or maximum. In detail, the spatial structured population traps into the frozen state of full loners at the minimum of loner's payoff and frozen state of full cooperators at the maximum of loner's payoff. This seems to contradict our previous discussion.
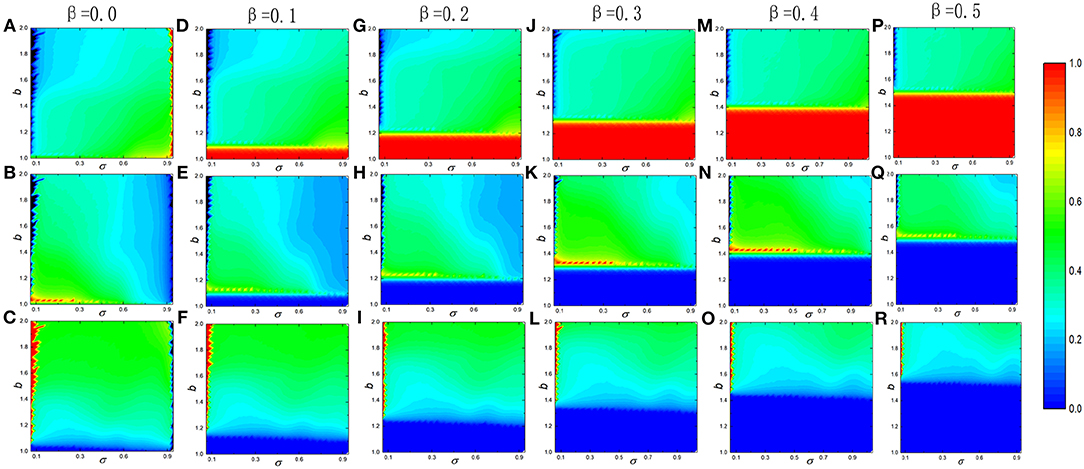
Figure 6. Average frequency of cooperators (top row),defectors (middle row) and loners (bottom row) evolves with the temptation b and loners' payoff σ for different reciprocal rewarding strength β at the stationary state in the voluntary PDG. From left to right, the strength of the reciprocal rewarding is 0.0, 0.1, 0.2, 0.3, 0.4, and 0.5, respectively. In all simulations, other parameters are set to be L = 100, MCS = 5 × 104, K = 0.1.
Considering the discrepancy observed in Figure 6, we now hope to find the deep reasons through the time evolution of the three strategies. As the frozen state of full pure strategies emergences in all situation of reciprocal rewarding β, we only talk about the special case where the typical reciprocal rewarding β = 0. Figure 7 features the time course of the average frequency of each pure strategy, i.e., cooperators, defectors and loners, for loner's payoff σ = 0.02 (panel A) and σ = 0.99 (panel B). From the panel A of Figure 7, we can see that an initial drop follows by a quick recovery of the frequency of loners while the frequency of defectors rises to the peak then quickly declines, but the frequency of cooperators continues to decrease overall although there is a slight increase over the time course of evolution. On the one hand, the strong ability to attack of defectors makes the cooperators cannot form tight clusters. On the other hand, the payoff of loner is too small to assist the cooperator in effectively resisting the invasion of the defector. So the cooperators first vanish from sight in the spacial structured population. After the cooperator disappears, there are only defectors and loners in the system. However, as the strategy of loners is superior to the strategy of defectors, thus, defectors cannot escape the fate of being destroyed and further absorbed by loners. Eventually the loners dominant the whole system when the benefit of loner is at the minimum value. On the contrary, we can get the opposite result when the payoff of loner is enough large, i.e., the cooperators coverage the whole spacial structured population while the loners and defectors completely vanish at the situation of σ = 0.99. From the panel B of Figure 7, we can observe that the frequency of cooperators and defectors declines while the frequency of loner increases at the initial stage. Then the frequency of cooperators begins to boost under the help of loners but the frequency of defectors continue to decrease until disappears first. After that, there are only cooperators and loners in the system. As the cooperators can beat the loners, thus, the cooperators occupy the full spacial structured population at last.
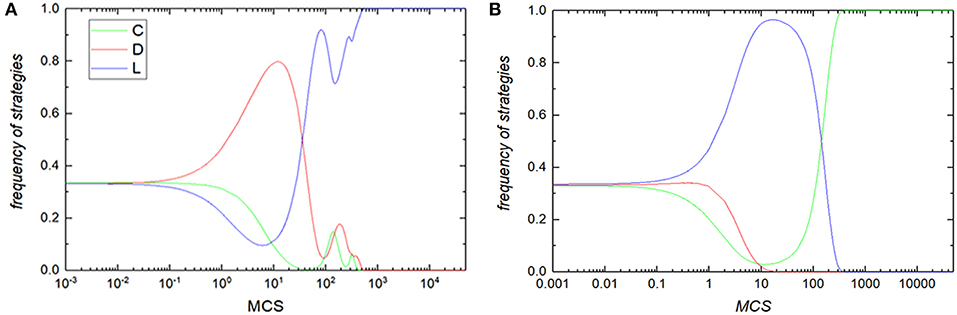
Figure 7. Frequency of three strategies with the spacial structured population at each time step under the small tiny benefit σ = 0.02 (A) and the large tiny benefit σ = 0.99 (B) under the reciprocal rewarding strength β = 0.0 in the voluntary PDG. In all panels, green, red, and blue curves correspond to cooperators, defectors, and loners, respectively. In all simulations, other parameters are set to be L = 100, MCS = 5 × 104, b = 1.4, K = 0.1.
After analysis, we find that the loners are conditioned to maintain the cyclic dominance of rock-scissor-paper state, that is, the payoff of loner can not be too small or too large. Meanwhile, there is a key factor to enhance the evolution of cooperation between reciprocal rewarding β and payoff of loner σ at different stage in our model, i.e., they can not promote the cooperation of evolution at the same time although they can serve the same purpose.
3.4. The Influence of Uncertainty Factor K on the Evolution of Cooperation
Finally, it is instructive to investigate the phase transition process to understand the behavior of cooperation on different levels of uncertainty K by strategy adoptions. If K → ∞ means that all information is lost so that strategies are chosen randomly. In contrast, when K → 0 enables players adopt their neighbor's strategy with the full certainly [68]. Figure 8 shows the full b − K phase diagram for different value of β = 0, 0.3, and 0.5 from left to right on a square lattice. Blue curve corresponds to the boundary of defectors' emergence, while the other curve is the boundary of extinction of loners. It is worth noting that we relax the range of the temptation b, i.e., allow b < 1. Mainly, the required values of temptation b that cause emergence of loners are always larger than that of defectors regardless of K in all given case, and all graphs feature bell shape separating the pure cooperators and mixed cooperators, defectors and loners phases, indicating that there is an optimal level of K that can promote the evolution of cooperation at best. Similarly, it corresponds to the traditional situation when β = 0, and the result is consistent with the previous work [69]. When β > 0, the quantitative properties of phase diagrams are significantly modified although the shape of phase diagrams in qualitatively kept unchanged compared with the traditional case, because the cyclic dominance phase C + D + L is substantially compressed while the C phase is widely enlarged. In particular, as the value of β increases, the C area expands. Taking together, it proves that the introduction of reciprocal rewarding could greatly encourage cooperator to form compact clusters against the adverse situation and maintain cooperative behaviors from another aspect.
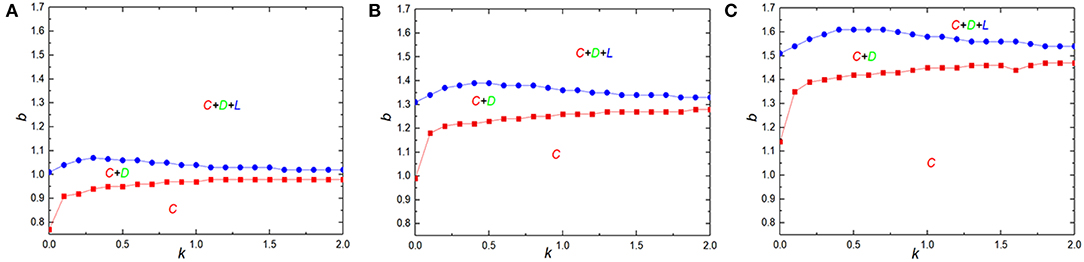
Figure 8. Full b − K phase diagrams for β = 0.0 (A), β = 0.3 (B), and β = 0.5 (C) from left to right. Red curve represents the boundary of defector extinction, and the blue curve stands for the boundary of the emergence of loners.
4. Conclusions and Discussions
In summary, within the framework of evolutionary graph theory and evolutionary game theory, we investigate the effect of reciprocal rewarding on the evolution of cooperation in the spatial voluntary PDG and voluntary SDG. In our model, the fitness of cooperators can be adjusted by reciprocal rewarding when there are cooperative neighbors around cooperators and the cooperators' additional incentive benefit is in direct proportion to the number of their cooperative neighbor. By means of Monte Carlo simulations, compared with the traditional case with voluntary participation, we confirm that the reciprocal rewarding factor β could effectively promote the evolution of cooperation from the macroscopic and microscopic perspective. After introducing the reciprocal rewarding into the model, pairs of cooperators have significantly remunerative advantages when b or r is relatively small so that the cooperative clusters could not only resist the invasion of defectors but also absorb the loners as cooperators, which ensures the cooperative strategy to spread on the lattice grid and even dominate the whole system under the evolutionary dynamics. In addition, loners' strategy enriches the diversity of spatial structured population strategies in voluntary PDG and voluntary SDG. Meanwhile, defectors are superior to cooperators, cooperators are better than loners, and loners outperform defectors, so that the system will inevitably fall into the so-called rock-paper-scissors game, where the cycle dominance of three strategies appears, and then cooperators always present in the system even when b or r is larger. But we also find that the loners is conditioned to maintain the cyclic dominance, that is, the payoff of loner cannot be too small or too large, which will destroy the situation of cyclic dominance. In particular, the lager contribution of reciprocal rewarding/payoff of loner, the more obvious the promoting effect. In terms of the border relevance of our research, since reciprocal rewarding behavior is common in nature, the results may further enrich our understanding of the emergence and persistence of the cooperative behavior in the real world. In the future, the reciprocal rewarding will be further extended into the networked topology so as to deeply explore the evolution of cooperation.
Data Availability
The raw data supporting the conclusions of this manuscript will be made available by the authors, without undue reservation, to any qualified researcher.
Author Contributions
XL and CX designed and performed the research. HW and MP proposed the advise. All authors analyzed the results, wrote the first draft paper, contributed to revisions, and reviewed the manuscript.
Funding
CX was supported in part by the National Natural Science Foundation of China (NSFC) under Grants 61773286 and 61374169, and China Scholarship Council under Grant 201808120001. MP was supported by the Slovenian Research Agency (Grants J4-9302, J1-9112, and P1-0403).
Conflict of Interest Statement
The authors declare that the research was conducted in the absence of any commercial or financial relationships that could be construed as a potential conflict of interest.
Acknowledgments
We would like to thank the reviewers for their constructive comments to improve manuscript.
References
1. Pennisi E. How did cooperative behavior evolve? Science. (2005) 309:93. doi: 10.1126/science.309.5731.93
2. Clutton-Brock T. Cooperation between non-kin in animal societies. Nature. (2009) 462:51–7. doi: 10.1038/nature08366
3. Axelrod R, Hamilton WD. The evolution of cooperation. Science. (1981) 211:1390–6. doi: 10.1126/science.7466396
5. Smith EA. Communication and collective action: language and the evolution of human cooperation. Evol Hum Behav. (2010) 31:231–45. doi: 10.1016/j.evolhumbehav.2010.03.001
6. Axelrod R, Axelrod DE, Pienta KJ. Evolution of cooperation among tumor cells. Proc Natl Acad Sci USA. (2006) 103:13474–9. doi: 10.1073/pnas.0606053103
7. Li JQ, Sun QL, Chen ZQ, Zhang JL. Changing the intensity of interaction based on individual behavior in the iterated prisoner's dilemma game. IEEE Trans Evolut Comput. (2017) 21:506–17. doi: 10.1109/TEVC.2016.2628385
8. Gómez-Gardeñes J, Reinares I, Arenas A, Floría LM. Evolution of cooperation in multiplex networks. Sci Rep. (2012) 2:620. doi: 10.1038/srep00620
9. Wang Z, Szolnoki A, Perc M. Self-organization towards optimally interdependent networks by means of coevolution. New J Phys. (2014) 16:033041. doi: 10.1088/1367-2630/16/3/033041
10. Deng WF, Huang KK, Yang CH, Zhu HQ, Yu ZF. Promote of cooperation in networked multiagent system based on fitness control. Appl Math Comput. (2018) 339:805–11. doi: 10.1016/j.amc.2018.08.002
11. Capraro V, Perc M. Grand challenges in social physics: in pursuit of moral behavior. Front Phys. (2018) 6:107. doi: 10.3389/fphy.2018.00107
12. Javarone MA. Solving optimization problems by the public goods game. Eur Phys J B. (2017) 90:171. doi: 10.1140/epjb/e2017-80346-6
14. Doebeli M, Hauert C. Models of cooperation based on the prisoner's dilemma and the snowdrift game. Ecol Lett. (2005) 8:748–66. doi: 10.1111/j.1461-0248.2005.00773.x
15. Perc M. Coherence resonance in a spatial prisoner's dilemma game. New J Phys. (2006) 8:022. doi: 10.1088/1367-2630/8/2/022
16. Wang WX, Ren J, Chen GR, Wang BH. Memory-based snowdrift game on networks. Phys Rev E. (2006) 74:056113. doi: 10.1103/PhysRevE.74.056113
17. Hauert C, Doebeli M. Spatial structure often inhibits the evolution of cooperation in the snowdrift game. Nature. (2004) 428:643–6. doi: 10.1038/nature02360
18. Wang Z, Jusup M, Shi L, Lee JH, Iwasa Y, Boccaletti S. Exploiting a cognitive bias promotes cooperation in social dilemma experiments. Nat Commun. (2018) 9:2594. doi: 10.1038/s41467-018-05259-5
19. Xia CY, Li XP, Wang Z, Perc M. Doubly effects of information sharing on interdependent network reciprocity. New J Phys. (2018) 20:0750025. doi: 10.1088/1367-2630/aad140
20. Hilbe C, Sigmund MA, Sigmund K. Evolution of extortion in iterated prisoner's dilemma games. Proc Natl Acad Sci USA. (2013) 110:6913–8. doi: 10.1073/pnas.1214834110
21. Hauert C. Replicator dynamics of reward & reputation in public goods games. J Theor Biol. (2010) 267:22–8. doi: 10.1016/j.jtbi.2010.08.009
22. Wang Z, Jusup M, Wang RW, Shi L, Iwasa Y, Moreno Y, et al. Onymity promotes cooperation in social dilemma experiments. Sci Adv. (2017) 3:e1601444. doi: 10.1126/sciadv.1601444
23. Li XL, Marko J, Wang Z, Li HJ, Shi L, Boris P, et al. Punishment diminishes the benefits of network reciprocity in social dilemma experiments. Proc Natl Acad Sci USA. (2018) 115:30–5. doi: 10.1073/pnas.1707505115
24. Liu DN, Huang CW, Dai QL, Li HH. Positive correlation between strategy persistence and teaching ability promotes cooperation in evolutionary prisoner's dilemma games. Proc Natl Acad Sci USA. (2019) 520:267–74. doi: 10.1016/j.physa.2019.01.041
25. Perc M, Jordan JJ, Rand DG, Wang Z, Boccaletti S, Szolnoki A. Statistical physics of human cooperation. Phys Rep. (2017) 687:1–51. doi: 10.1016/j.physrep.2017.05.004
26. Wang Z, Bauchc CT, Bhattacharyyad S, d'Onofrio A, Manfredi P, Perc M, et al. Statistical physics of vaccination. Phys Rep. (2016) 664:1–113. doi: 10.1016/j.physrep.2016.10.006
27. Hardin G. The tragedy of the commons. Science. (1968) 162:1243–8. doi: 10.1126/science.162.3859.1243
28. Hofbauer J, Sigmund K. Evolutionary Games and Population Dynamics. Cambridge: Cambridge University Press (1998).
29. Kang ZX, Lei LZ, Li K. An improved social force model for pedestrian dynamics in shipwrecks. Appl Math Comput. (2019) 348:355–62. doi: 10.1016/j.amc.2018.12.001
30. Javarone MA, Marinazzo D. Evolutionary dynamics of group formation. PLoS ONE. (2017) 12:e0187960. doi: 10.1371/journal.pone.0187960
31. Nowak MA, Tarnita CE, Antal T. Evolutionary dynamics in structured populations. Philosoph Trans Roy Soc London B Biol Sci. (2010) 365:19–30. doi: 10.1098/rstb.2009.0215
32. Szabó G, Fáth G. Evolutionary games on graphs. Phys Rep. (2007) 446:97–216. doi: 10.1016/j.physrep.2007.04.004
33. Li K, Szolnoki A, Cong R, Wang L. The coevolution of overconfdence and blufng in the resource competition game. Sci Rep. (2016) 6:21104. doi: 10.1038/srep21104
34. Javarone MA. Statistical Physics and Computational Methods for Evolutionary Game Theory. Cham: Springer Press (2018).
35. Nowak MA, May RM. Evolutionary games and spatial chaos. Nature. (1992) 359:826–9. doi: 10.1038/359826a0
36. Qin SM, Chen Y, Zhao XY, Shi J. Effect of memory on the Prisoner's Dilemma game in a square lattice. Phys Rev E. (2008) 78:041129. doi: 10.1103/PhysRevE.78.041129
37. Szolnoki A, Perc M, Szabo G. Phase diagrams for three-strategy evolutionary prisoner's dilemma games on regular graphs. Phys Rev E. (2009) 80:056104. doi: 10.1103/PhysRevE.80.056104
38. Masuda N, Aihara K. Spatial Prisoner's Dilemma optimally played in small-world networks. Phys Lett A. (2003) 313:55–61. doi: 10.1016/S0375-9601(03)00693-5
39. Fu F, Liu LH, Wang L. Evolutionary Prisoner's Dilemma on heterogeneous Newman-Watts small-world network. Eur Phys J B. (2007) 56:367–72. doi: 10.1140/epjb/e2007-00124-5
40. Barabási AL, Albert R. Emergence of scaling in random networks. Science. (1999) 286:509–12. doi: 10.1126/science.286.5439.509
41. Rong ZH, Li X, Wang XF. Roles of mixing patterns in cooperation on a scale-free networked game. Phys Rev E. (2007) 76:027101. doi: 10.1103/PhysRevE.76.027101
42. Hadjichrysanthou C, Broom M, Kiss IZ. Approximating evolutionary dynamics on networks using a neighbourhood configuration model. J Theoret Biol. (2012) 312:13–21. doi: 10.1016/j.jtbi.2012.07.015
43. He JZ, Wang RW, Li YT. Evolutionary stability in the asymmetric volunteer's dilemma. PLoS ONE. (2014) 8:e103931. doi: 10.1371/journal.pone.0103931
44. Wang Z, Wang L, Szolnoki A, Perc M. Evolutionary games on multilayer networks: a colloquium. Eur Phys J B. (2015) 88:124. doi: 10.1140/epjb/e2015-60270-7
45. Meng XK, Sun SW, Li XX, Wang L, Xia CY, Sun JQ. Interdependency enriches the spatial reciprocity in prisoner's dilemma game on weighted networks. Phys A. (2016) 442:388–96. doi: 10.1016/j.physa.2015.08.031
46. Wang CJ, Wang L, Sun SW, Xia CY. Inferring the reputation enhances the cooperation in the public goods game on interdependent lattices. Appl Math Comput. (2017) 293:18–29. doi: 10.1016/j.amc.2016.06.026
47. Xia CY, Wang ZS, Guo QT, Shi YT, Dehmer M, Chen ZQ. A new coupled disease-awareness spreading model with mass media on multiplex networks. Inf Sci. (2019) 471:185–200. doi: 10.1016/j.ins.2018.08.050
48. Wang ZS, Guo QT, Sun SW, Xia CY. The impact of awareness diffusion on SIR-like epidemics in multiplex networks. Appl Math Comput. (2019) 349:134–47. doi: 10.1016/j.amc.2018.12.045
49. Xia CY, Ding S, Wang CJ, Wang J, Chen ZQ. Risk analysis and enhancement of cooperation yielded by the individual reputation in the spatial public goods game. IEEE Syst J. (2017) 11:1516. doi: 10.1109/JSYST.2016.2539364
50. Li XP, Sun SW, Xia CY. Reputation-based adaptive adjustment of link weight among individuals promotes the cooperation in spatial social dilemmas. Appl Math Comput. (2019) 361:810–20. doi: 10.1016/j.amc.2019.06.038
51. Lu WW, Wang J, Xia CY. Role of memory effect in the evolution of cooperation based on spatial prisoner's dilemma game. Phys Lett A. (2018) 382:3058. doi: 10.1016/j.physleta.2018.07.049
52. Huang KK, Zhao XF, Yu ZF, Yang CH, Gui WH. Heterogeneous cooperative belief for social dilemma in multi-agent system. Appl Math Comput. (2018) 320:572–9. doi: 10.1016/j.amc.2017.10.018
53. Helbing D, Szolnoki A, Perc M, Szabó G. Punish, but not too hard: how costly punishment spreads in the spatial public goods game. New J Phys. (2010) 12:083005. doi: 10.1088/1367-2630/12/8/083005
54. Macy MW, Flache A. Learning dynamics in social dilemmas. Proc Natl Acad Sci USA. (2002) 99:7229–36. doi: 10.1073/pnas.092080099
55. Wu Y, Chang SH, Zhang ZP, Deng ZH. Impact of social reward on the evolution of the cooperation behavior in complex networks. Sci Rep. (2017) 7:41076. doi: 10.1038/srep41076
56. Dreber A, Rand DG, Fudenberg D, Nowak MA. Winners don't punish. Nature. (2008) 452:348–51. doi: 10.1038/nature06723
57. Szolnoki A, Perc M. Antisocial pool rewarding does not deter public cooperation. Proc Biol Sci. (2015) 282:20151975. doi: 10.1098/rspb.2015.1975
58. Li K, Cong R, Wu T, Wang L. Social exclusion in finite populations. Phys Rev E. (2015) 91:042810. doi: 10.1103/PhysRevE.91.042810
59. Ding CX, Wang J, Zhang Y. Impact of self interaction on the evolution of cooperation in social spatial dilemmas. Chaos Solit Fract. (2016) 91:393–9. doi: 10.1016/j.chaos.2016.06.021
60. Wu Y, Zhang ZP, Chang SH. Reciprocal reward promotes the evolution of cooperation in structured populations. Chaos Solit Fract. (2019) 119:230–6. doi: 10.1016/j.chaos.2019.01.006
62. Hauert C, Monte SD, Hofbauer J, Sigmund K. Volunteering as red queen mechanism for cooperation in public goods games. Science. (2002) 296:1129–32. doi: 10.1126/science.1070582
63. Szabó G, Hauert C. Evolutionary prisoner's dilemma games with voluntary participation. Phys Rev E. (2002) 66:062903. doi: 10.1103/PhysRevE.66.062903
64. Szabó G, Hauert C. Phase transitions and volunteering in spatial public goods games. Phys Rev Lett. (2002) 89:118101. doi: 10.1103/PhysRevLett.89.118101
65. Luo C, Zhang XL, Zheng YJ. Chaotic evolution of prisoner's dilemma game with volunteering on interdependent networks. Commun Nonlinear Sci. (2017) 47:407–15. doi: 10.1016/j.cnsns.2016.12.004
66. Niu ZX, Mao DM, Zhao TY. Impact of self-interaction on evolution of cooperation in voluntary prisoner's dilemma game. Chaos Solit Fract. (2018) 110:113–37. doi: 10.1016/j.chaos.2018.03.008
67. Szabó G, Töke G. Evolutionary prisoner's dilemma game on a square lattice. Phys Rev E. (1998) 58:69–73. doi: 10.1103/PhysRevE.58.69
68. Chen GR, Wang XF, Li X. Fundamentals of Complex Networks: Models, Structures and Dynamics. Singapore: Wiley Publishing (2015).
Keywords: evolutionary game theory, cooperative behavior, reciprocal rewarding, voluntary participation, social dilemmas, cyclic dominance
Citation: Li X, Wang H, Xia C and Perc M (2019) Effects of Reciprocal Rewarding on the Evolution of Cooperation in Voluntary Social Dilemmas. Front. Phys. 7:125. doi: 10.3389/fphy.2019.00125
Received: 06 June 2019; Accepted: 19 August 2019;
Published: 04 September 2019.
Edited by:
Feng Fu, Dartmouth College, United StatesReviewed by:
Kun Li, Hebei University of Technology, ChinaMarco Alberto Javarone, University College London, United Kingdom
Copyright © 2019 Li, Wang, Xia and Perc. This is an open-access article distributed under the terms of the Creative Commons Attribution License (CC BY). The use, distribution or reproduction in other forums is permitted, provided the original author(s) and the copyright owner(s) are credited and that the original publication in this journal is cited, in accordance with accepted academic practice. No use, distribution or reproduction is permitted which does not comply with these terms.
*Correspondence: Chengyi Xia, cyxia@email.tjut.edu.cn; xialooking@163.com