- 1Department of Pharmacy, University of Salerno, Fisciano, Italy
- 2Department of Industrial Engineering, University of Salerno, Fisciano, Italy
Cell membranes are commonly considered fundamental structures having multiple roles such as confinement, storage of lipids, sustain and control of membrane proteins. In spite of their importance, many aspects remain unclear. The number of lipid types is orders of magnitude larger than the number of amino acids, and this compositional complexity is not clearly embedded in any membrane model. A diffused hypothesis is that the large lipid palette permits to recruit and organize specific proteins controlling the formation of specialized lipid domains and the lateral pressure profile of the bilayer. Unfortunately, a satisfactory knowledge of lipid abundance remains utopian because of the technical difficulties in isolating definite membrane regions. More importantly, a theoretical framework where to fit the lipidomic data is still missing. In this work, we wish to utilize the amino acid sequence and frequency of the membrane proteins as bioinformatics sensors of cell bilayers. The use of an alignment-free method to find a correlation between the sequences of transmembrane portion of membrane proteins with the membrane physical state (MPS) suggested a new approach for the discovery of antimicrobial peptides.
Introduction
Cell membranes are complex structures made of hundreds of different types of molecules [1, 2]. In recent years, the concept of biological membrane evolved from a simple physical barrier with extremely low water permeability into a highly sophisticated system capable to control protein activity, intracellular signaling, and stress response. Most biological membranes are both laterally and transversally asymmetrical. The cross-sectional asymmetry reflects the lipid composition of each leaflet. The asymmetric distribution of phospholipid types explains most of the differences between inner and outer layers. The formation of lateral segregation is the result of chemical-physical differences in lipids and, in some cases, the effect of cytoskeletal structures supporting the membrane that restrict the movement of both lipids and proteins. The lateral asymmetry is observed both in model systems and in biological membranes and it is mainly due to limited lipid miscibility. Lipids influence the activity of membrane proteins by means of their chemical nature and the physical properties of lipid mixtures in function of their composition [3–5]. The proportions and types of membrane lipids define different types of membrane microdomains that regulate: (i) the activity of the proteins; (ii) their localization; and (iii) protein–protein interactions and ensuing signal propagation [6]. Furthermore, during pathological conditions such as cancer [7] or Alzheimer's disease [8] and ageing processes [9, 10] membrane lipid composition can change significantly. Each of these changes causes a membrane remodeling, with consequent modifications of the organization and dynamic properties of lipids. Consequently, the activities of many membrane-associated proteins and transporters also change dramatically. Therefore, it is conceivable that molecules capable of interacting with membrane lipids may induce modifications in membrane composition, protein function or gene expression and reversion of the pathological state. It is well known that when temperature changes, cells produce adaptive responses [11]. Recently a new hypothesis suggests the role of the membrane as a sensor [4, 12], in which the remodeling of microdomains and the physical proprieties of the membrane are involved in the heat shock response (HSR) without protein denaturation. Changes in membrane lipid composition and altered heat shock protein levels are often found in some disease such as diabetes, cancer or neurodegenerative diseases. The function of transmembrane proteins is extremely dependent on the lipid environment [13] and on the amino acid composition and the distribution of amino acids along the helix [14, 15]. Membranes control also recruitment and clusterization of proteins [5]. Generally, transmembrane segments (TM) are found to adopt α-helical conformations, and this requirement along with the hydrophobicity necessary for the insertion in the bilayer, should severely limit the variety of involved amino acids. In fact, compositional analyses of TM segments of membrane proteins have shown that they are dominated by hydrophobic amino acids such as Ile and Val, but the exact composition reflects, among others, the type of aggregation and the lipid environment.
Some antimicrobial peptides are synthesized in the absence of infection or inflammation, whereas others are upregulated in response to endogenous or infectious “alarm” signals, suggesting different functions for these peptides under different physiological settings. Some antimicrobial peptides (AMP) are produced in cell by proteolytic cleavage of a membrane protein [16, 17]. Others AMP can selectively intercalate and self-assembly in bacterial membranes only. The role of cholesterol in AMP selectivity was also extensively studied [18]. All these aspects rise several important questions: how is a TM selected for a particular lipid environment? Does exist any correlation between AMP and TM composition of a given organism? What is happening to the peptide-membrane interaction when the system is physically or chemically altered, for example by changing temperature, pressure, composition or upon the effect of a pathogen organism? Do membranes play a role in governing the AMP selectivity? The interplay between transmembrane regions and lipid environment become even more evident if we consider thermophiles. Thermophiles are bacteria or archaea that live at temperatures above 60°C. In some cases, they can survive at water temperature higher than 100°C and high pressures. Those organisms, regardless their evolution, learn to cope with high temperature using special lipids [19] that cannot be found in cells living at lower temperature because the membrane would be far too rigid to permit life. How do thermophiles adapt, if so, the amino acid composition of their TM portions to deal with exotic lipids?
The main goal of the present work is to try to answer these questions by means of comparative analysis of the membrane-interacting peptides composition.
Our main assumptions are summarized in Figure 1. The peptide function, for example, its capability to perturb a membrane, or to kill an organism, or to self-assembly, is a function of the amino acid sequence and of the membrane physical state (MPS). The MPS is a key concept to understand peptide-membrane interaction [3, 12] and, occasionally, it has been approximated by the lateral pressure profile [20]. Unfortunately, the MPS, like a previously used descriptor such as “fluidity,” cannot be uniquely determined in lipid membranes. In fact, fluidity refers to the viscosity of a fluid. In this case, the lipid bilayer of a cell membrane. Cell membranes are anisotropic and the internal frictions in the middle of a membrane is very different to that under the headgroups. Commonly used fluorescence measures, for example, rely on the assumption that the probe is uniformly distributed in the all membrane and that the probe itself does not alter the bilayer fluidity [21]. As suggested by several authors [22, 23], lateral stress profile could be considered a better descriptor of the physical state, but it cannot be measured experimentally [23]. Even the calculation cannot be easily done because the lipid composition of a membrane is difficult to access experimentally [24]. MPS depends on the temperature, external conditions, and the cell cycle phases.
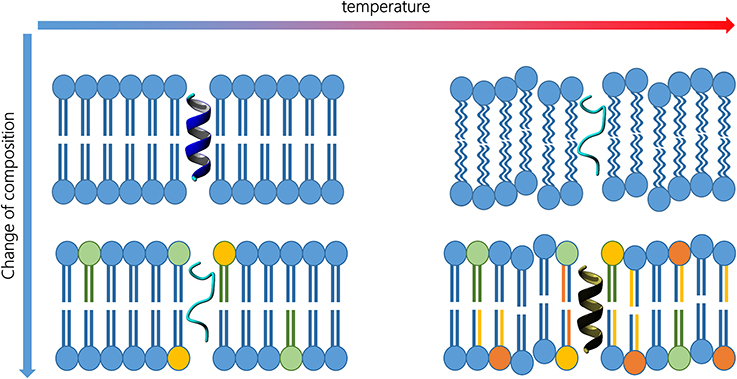
Figure 1. Peptide activity is a function of its 3D structure, which, in turn, is influenced by membrane composition and external conditions (top left). Changes in lipid composition (bottom left) or temperature (top right) may alter the secondary structure. Proper changes in lipid composition and in the amino acid sequence may reverse the temperature increase effect, and therefore, recover the peptide activity (bottom right).
For the sake of clarity, Figure 1 represents the typical case of a transmembrane peptide. On the top left, the peptide exhibit an alpha helix structure that fits a particular lipid environment. If the temperature rises (top right), both bilayer and peptide are influenced and the peptide can eventually lose its secondary structure. Analogously, an alteration in lipid composition may also affect the inner fluidity and the peptide geometry (bottom left). In fact, similar alteration of the peptide conformation can be achieved, and is experimentally observed, in different membranes. Organisms living at high temperature need more rigid membranes and the peptide sequence could, therefore, be modified to permit the best interaction. When an organism is forced to live at higher temperature, the lipid composition must change replacing some lipids with molecular species having higher transition temperatures. Consequently, the transmembrane peptide sequence can be modified to guarantee the best interaction in the new environment (bottom right) and, therefore, to permit the recovery of the functional secondary structure.
Summarizing, instead of considering the peptide sequence only, the peptide function must be understood in terms of both sequence and MPS. The MPS is, in turn, function of temperature and lipid composition.
If we assume that specific peptides are well adapted to a particular MPS to show a specific function, then we can use sequence analysis to infer information on the MPS that, more than the lipid composition, is the driving force of peptide-membrane interactions. Moreover, the MPS has a fundamental role in controlling the protein recruitment and the peptide self-assembly [5, 25].
It is reasonable to assume that a particular sequence has been selected by the evolution to offer the best interactions with other parts of the protein, or with the membrane, in a given condition. The best interactions mean we are considering more than simply the possibility to insert a membrane [26]. In fact, from an energetic point of view, the integration of a peptide into a bilayer is mainly a matter of hydrophobicity, and can be achieved with few amino acids only [27]. Even the helicity of a peptide can be obtained with the regular distribution of residues, whereas the ability to self-assemble into a pore, for example, requires a sophisticated adaptation to that particular lipid environment.
The different lipid composition is generally assumed of being a key factor for the selectivity of AMP toward bacterial cell [28] (Figure 1, bottom left) and, more generally, for the interaction of peptides with bilayers [29]. Along with lipid composition, other chemical or physical factors can influence the MPS. For example, temperature, water pressure, ionic strength or extreme pH can also severely affect the folding and integration of peptides. The understanding of the interplay between peptide sequence and MPS is favored by the study of membrane composition of thermophiles [30].
In fact, the temperature can induce some bacteria to modify the lipid composition of their membranes, and this alteration selects particular sequences for transmembrane peptides. The investigation of the amino acid distribution in thermophiles pave the way to the understanding of selectivity of peptides to different cell membranes. A relevant case is the one of antimicrobial peptides (AMP) that are, in many cases, capable to discriminate among different cell membranes [31].
Though the above considerations may appear acceptable and even obvious, the prediction of the peptide function and the design of novel peptides is surely not. Previous investigations [32–34] evidenced particular amino acids abundance or sequence pattern in some classes of proteins. The search of regularities in transmembrane regions made intensive use of pair-wise alignment techniques. Such techniques are well suited for the investigation of homolog proteins, but fail to detect regularity in secondary structures. Moreover, the computation of an accurate multiple-sequence alignment is an NP-hard problem, which means that the alignment cannot be solved in a realistic time frame [35].
The difficulty in defining a metric for sequence dissimilarity is also recognizable in the analysis of natural language texts [36]. The quantification of similarity between sequences is not unique and unambiguous, since it depends on the relative importance assigned to words and to the overall context of its occurrence. The vast majority of biological sequence comparison methods rely on first aligning reference homologous sequences and deriving a score for the alignment of individual units. Pair-wise tools are based on a very strong hypothesis: the contiguity conservation, which assumes that two similar sequences (representing two biologic entities) must derive from a common ancestor [37]. This assumption allowed the use of string similarity algorithms (global and local pair-wise methods, dynamic programming) on biologic sequences. When a pair-wise tool is used, the algorithms need to handle permutations, substitutions (with many different matrices depending on the kind of analysis to be performed), deletions and gaps. Moreover, most of the pair-wise algorithms (not the heuristic version) are related to Smith-Waterman and Needleman-Wunsch (two dynamic programming algorithms) and are not efficient on large sequences [38]. Since the presence of sequence patterns seems to be the exception rather than the rule, but assuming that some regularity must govern the lipid-peptide interactions, we considered an alternative paradigm called “alignment-free”: this paradigm avoids some problems related to pair-wise analysis [37].
For our sequence analysis, we concentrated on “alignment-free” method. In our approach, which exploits novel metrics to calculate similarity among sequences, we use transmembrane peptides as a sort of bioinformatics sensors of the MPS.
The experimental section is organized into three parts. First, we describe how we developed a new metric for calculating the distance between two strings. In the second part, we show how we collect the raw data to analyze. In the third part, we compress the information and we apply the metric.
In the Results and discussion section, we validate the approach on known systems and we demonstrate how lipid composition and membrane evolution is, in some ways, encoded in the transmembrane proteome composition.
Methods
To analyze the peptide sequences we have chosen an alignment-free approach. The metrics we proposed have been implemented in the software ProtComp (www.yadamp.unisa.it/protcomp). ProtComp can work on heterogeneous sequences, but it is optimized for working on proteomes and genome in FASTA format. It consists of python scripts to parse the input files and prepare them for the analysis. The preprocess consists mainly of the removal of all unneeded data, like FASTA headers. The preprocess script works on all plain text files and with some binary files (like BMP, PNG or JPEG). A second script permits the extraction of substrings of length k (k-mer). This step is computationally demanding and, for this reason, we used a sliding windows approach that is naturally parallelizable and distributable. The input file is divided into chunks and each chunk is assigned to a core that handles a sliding window. All the chunks share j symbols (also, two chunks are overlapped by j symbols) in the cut point, to avoid loss of information. When all chunks of an input file have been processed, they are joined in a single MEFr, k and this set are truncated to r length: all the pairs (ci, oi) in this set are sorted in descendent order by oi. Notice that an execution of ProtComp can produce multiple MEF sets, one for each k used by metrics. ProtComp uses metrics (described below) that needed multiple k-mer lengths (at the same time it uses k-mer of different length) so for each chunk different sliding window can be executed with different a value of k, to extract k-mer. This approach ensures computational speed benefits because for each computer and for each core we can assign a chunk and a value of k for k -mer extraction.
In general, we refer to the features that discriminate an entity, meaning all the common patterns more repeated that could be extracted. For example, if we consider strings of mRNA, a feature is the presence of the start or stop codons. Each biological entity (following named BE) could be represented by distinctive features. The conservative features (ci) for the BE X have high weight |ci| [39] compared to the total weight |X| = ∑|cj| ∀cj∈X of features that represent a BE. If ci is more expressed than cj in X then we will have:
Each BE evolves independently [39]. Therefore, the same feature ciis free to appear with different weight and position in two different BE (X and Y). In addition, two features ci and cj can derive from a common feature cr even containing insertion, transformations, deletions or swaps.
The second assumption has a very strong implication: during the calculation of the similarity between two sequences, the weight of the feature, expressed as the number of occurrences, determines the similarity and not the position of the conservative features.
Basic Definitions
It is possible to define an Expressed Feature (from now EF) of length l as a pair (ci, oi) where ci, is a segment of length l that occurs oi times in a sequence X with oi ≥ 1. The EFl set contains all pairs of features expressed as len(ci) = l sorted in descending order by value oi.
Consequently, it is possible to define the set of the Most Expressed Features MEFr, ml(X) of a X sequence as the set of the first r elements taken from the EFl subsets with l = 1 → ml (ml = string maximum length).
The definition of MEF (Most Expressed Features) is strictly linked to the concepts of “weighting characters” and “correlated characters” defined by Felsenstein [39], to the results reported in Attwood and Sims et al. (40, 41) and to two empirical observations described in the following. The value r in this work is defined as the maximum resolution and, therefore, the ml is the maximum length considered for the analysis. Increasing the values of r and ml the accuracy of the analysis also increases. The set MEF(X) (without subscripts) indicates the union ∪MEFr, ml(X) ∀r, ml. The strings considered here are formed by characters only: the amino acid one letter symbols.
The similarity between two strings is based on the dimension of the MEF(X). For the development of MEF, we defined two metrics: the Easy Similarity Score (ESS) and the Weighted Similarity Score (WSS) hereafter described. ESS is defined as:
where MIN(X, Y) indicates the min (|MEF(X)|, |MEF(Y)|), that is the size of the smallest MEF set.
The WSS is a variation of ESS, in which we add the number of times that the intersection MEFri(X)∩MEFri(Y) is not empty (iUsed):
Both metrics measure the similarity degree, so it is possible to define the distance as:
The metrics have the following properties:
1. ESS(X, Y) = WSS(X, Y) = 1 if X = Y
2. WSS(X, Y) = d ∈ [0, 1] if X and Y share common characteristics.
3. ESS(X, Y) = d ∈ [0, 1] if X and Y share common characteristics.
The metric WSS uses the index i to give more importance to longer strings, respecting the above assumption: if two features are both common and conserved in two entities, then the entities should be evolutionarily very close.
For the purposes of this work, the similarity is limited to 1. For the ESS metric, the range [0;1] is closed, so the similarity value could be used even as a probability. These metrics can be used to estimate the similarity between two text objects for example proteomes.
It is easy to observe that the use of MEF is robust to deal with insertions, substitutions and permutations. In particular, insertions at the end of a string are less penalized than insertions in the middle. Both metrics penalize substitutions more than the insertion, mimicking the behavior observed in natural systems.
MEF, by the definition, manage the problem of the insertion during the comparison of two sequences. It is possible to consider the segments X = {a1a2a3a4a5} and Y = {a1a2Za3a4a5} taken from the same BE in two different moments; in Y is occurred the insertion (Z before a3). In this case, the insertion is in an internal point of the segment. In the model presented in this work, the gap management is implicit, because the presence of a gap is equivalent to the presence of a blank character within the sequence. This character will be detected by metrics as an insertion (if the sequence length is different) or as a replacement.
The pseudocode of the algorithm is shown in Figure 2.
The detailed description of the algorithm and some mathematical subtleties will be given in an upcoming paper.
Substitutions reduce the algorithms performance more than insertions, and the substitutions in the external positions are less performing than the substitution in the internal positions.
We made these two metrics available with a tool that can be used in many types of architecture: singular PC, grid and cloud. For example, nowadays, it is common to provide web services that implement algorithms (like UNIPROT with BLAST or NCBI with FASTA) in such a way that final user can request a computation and download their results, when computation is completed. However, in some cases, a company may need private computation or want to build up their own private grid: in this case, they need a standalone software and not a web service. We have implemented the two metrics in the software ProtComp, available in two flavors: the first is a standalone package written in a C++ and enabled to use OpenMP. In this implementation, heavy emphasis was placed on performance, scalability and distributability because this version will be used as black box in a computational pipeline for other tools; this package uses a structure directory sub tree in order to maintain results for each dataset processed. The high number of execution parameters, described in Usability, permits the configuration of the level of granularity and the file parser to use. In this version, it is possible to use FASTA Parser and Plain-text parser. The second flavor of ProtComp is a web service (http://yadamp.unisa.it/protcomp) that allow the users to upload their files in their own space and permit to submit their computation in a batch queue: the service is written in ASP.NET and is based on IIS7 web server. The results of computation are stored in user-space and will be accessible for further analysis.
Set Definition
The AMP datasets were downloaded from the database Yadamp [31]. Yadamp permitted us to select peptides produced by specific class of organisms (see Supplementary Materials, Table 1). The AMP were chosen on the basis of their capability to interact with few selected organisms (namely, E. coli, B. subtilis, S. aureus), having a length comprised between 14 and 50 amino acids, with no disulfide bridges. The 3D structure of the peptides is available in the Yadamp database. The transmembrane (TM) portions of the proteomes (hereafter indicated as transmembrane proteomes TMP), were identified by means of a computational investigation. Among the many TM helix prediction tools we have chosen Phobius [42] a hidden Markov model (HMM) that models the different sequence regions of a signal peptide and the different regions of a transmembrane protein in a series of interconnected states. The false classifications of transmembrane helices are kept as low as 7.7%.
All proteomes (listed in the Supplementary Materials, Table 2) have been processed with Phobius, and the collection of all predicted transmembrane sequences of a given proteome called transmembrane proteome (TMP). Therefore, there is a TMP for any proteome. After the setting stage, we have three types of dataset. The collections of sequences of antimicrobial peptides active against a specific organism are called AMP. The collections of all the transmembrane sequences calculated in a given organisms are called TMP.
In order to calculate the distances between any pair of sets, we must extract the dictionaries Di. The routine that permits to extract the most abundant strings of the set, and therefore to create the dictionaries, is available, free of charge, at http://yadamp.unisa.it/protcomp/main.aspx.
The distances have been calculated applying the appropriate metrics to the dictionaries.
The evolutionary history was inferred using the Minimum Evolution (ME) method [43]. The ME tree was searched using the Close-Neighbor-Interchange (CNI) algorithm [44] at a search level of 1. The Neighbor-joining algorithm was used to generate the initial tree. Evolutionary analyses were conducted in MEGA7 [45].
Results and Discussion
Validation Test
We have followed the same validation protocol presented in Sims et al. (41). In that work, the authors used an alignment-free method to measure the similarity of whole genomes and books. In Figure 3, the result of the validation test is shown.
The first validation test was made on a set of English books as reported in Sims et al. (41). MEF was performed using as parameter r = 10 and ml = 10. The books were downloaded from the Free eBooks—Project Gutenberg (https://www.gutenberg.org) and were converted into a single long string by removing all spaces between words, all punctuation and all characters different from the sets [a–z] and [A–Z]. The list of eBooks is listed in the Supplementary Materials, Table 3. To avoid some phenomena of bias we removed common words such as CHAPTER, SECTION, PROJECT, GUTENBERG etc.
In the output tree, five branches are clearly defined. The title of each branch is our description of the books therein. The algorithm does not use any assumption nor any keywords to organize and cluster the books. Surprisingly, without any bias, ProtComp can find similarities among books of same authors, as well as similarities among authors. For example, Charles Dickens and Jane Austen are the closest pairs of authors. Another pair of related authors is H.G. Wells and J.M. Barries. These two pairs of authors are then related and correspond to what is easy to recognize as “Children's literature.” The only case in which books of the same author are in a separated branch, is Plato whose works are closely related to Spinoza, and Aristotle. The metric we proposed was capable, without any instruction or training, to catch the main differences among different works and authors of different periods.
The metric was then further applied to proteome analysis with similar results. The overall clustering follows the general topology obtained with the unrooted 16S-like rRNA-based and gene-shared phylogenies [46]. The results confirmed that the metric we developed permit to easily obtain the fundamental relationships among organisms.
The method we presented can be easily applied to calculate the distances among any arbitrary dictionary. We have applied the method to compare the relationships among complete proteomes and TMP.
Proteome Analysis
Alignment-free methods on whole proteomes do not rely on one of few proteins to derive the phylogeny and the results can differ from those conducted on one gene only. This aspect is particularly critical for the determination of the position of thermophiles in bacterial phylogeny. If for Woese [47] thermophiles are the earliest species of bacteria, Korbel [48] offered a different view.
In Figure 4, we report the phylogenetic tree obtained with substring length between 6 and 12 with the Equation (2). The calculation of the distance matrix among 51 full proteomes (see Supplementary Materials, Table 2) is extremely fast and lasts few seconds on i3 processor. It permitted to cluster clearly the organisms in different phyla. Only two bacteria with small proteome, M. tuberculosis and C. phaeobacteroides, fall in the archaea branch, but this is known to be an accuracy problem in the calculation of similarity.
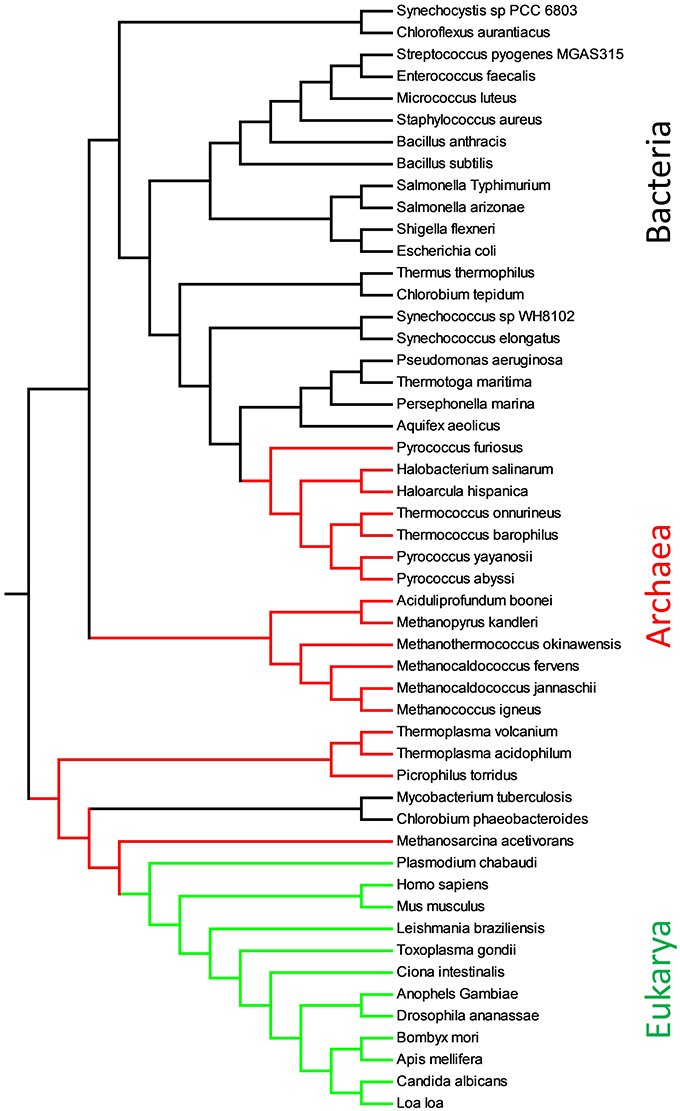
Figure 4. Evolutionary relationships of proteomes obtained with Equation (2) and substring length between 6 and 12. Eukarya are shown in green, Bacteria in black and Archaea in red.
Following the hypothesis that transmembrane portion of proteins might be well adapted to the particular chemical-physical environment in which they work, we conducted the same analysis of proteomes on transmembrane proteomes.
TMP Analysis
Figure 5 represents the ME tree of TMP. Contrarily to Figure 4, the organisms did not cluster according the phylogenetic relationships. The TMP are organized in a sort of temperature ramp with organisms living at low temperature separated by those living in extreme condition. The need to adapt to high temperatures, poses a dramatic challenge to a cell that cannot use ordinary lipids for their membranes. In fact, the living temperature cannot be more than few degrees hotter than the transition temperature of the lipid mixture. It is well known that thermophiles have a special lipid fingerprint [10, 19, 49, 50]. In the tree of TMP, few organisms do not respect the temperature rule. For example, B. anthracis is a Gram-positive, endospore-forming bacterium capable to survive at extremes of temperature. Another example is C. aurantiacus, a photosynthetic bacterium isolated from hot springs, belonging to the green non-sulfur bacteria. This organism is thermophilic and can grow at temperatures from 35°C to 70°C. The organism that, though extremely thermophilic, has a TMP related to non-thermophilic organisms, is Thermus thermophilus. T. thermophilus is a Gram-negative bacterium with an optimal growth temperature of about 65°C.
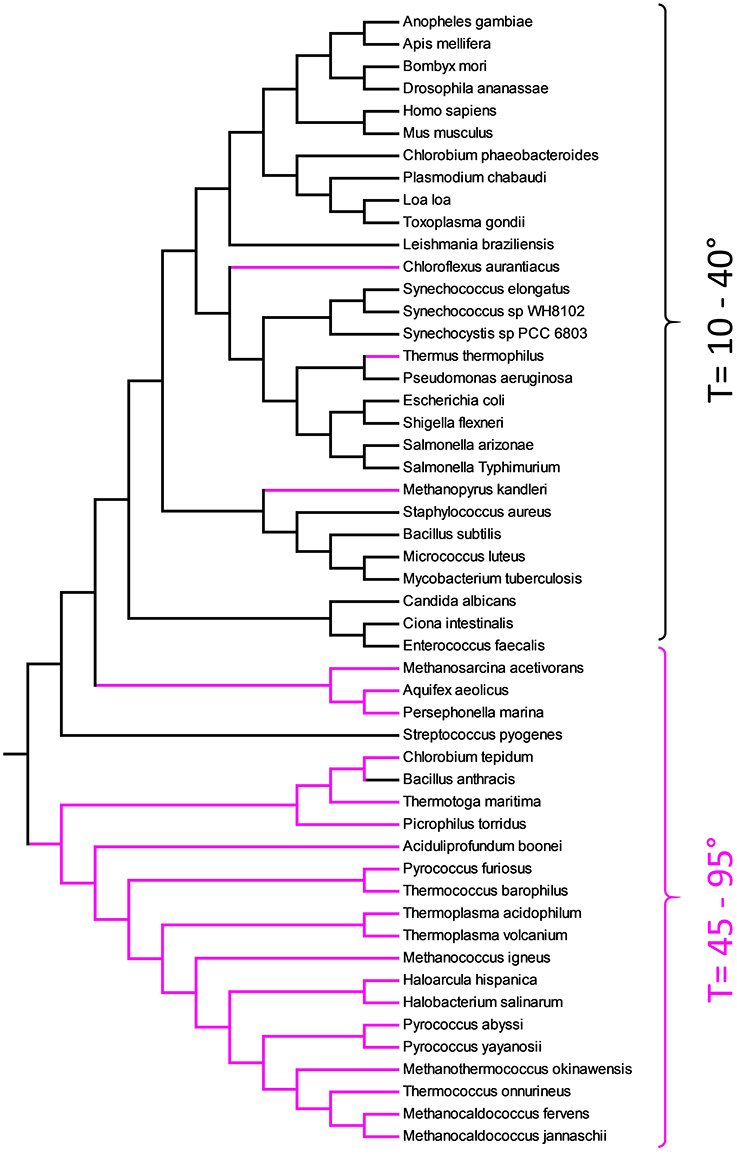
Figure 5. Evolutionary relationships of TMP obtained with Equation (2) and substring length between 6 and 12. Thermophiles are shown in pink.
A particular case is the one of bacteria belonging to the genus of Methanosarcina. These archaea contain pyrrolysine, a α-amino group (which is in the protonated form under biological conditions). Its pyrroline side-chain is similar to that of lysine in being basic and positively charged at neutral pH.
The presence, in the proteome, of this unique amino acid, puts M. acetivorans far from other more related organisms. In fact, whereas a pair-wise alignment can add simply a penalty to an extra character, alignment-free analysis is more sensitive to amino acid replacement, since any string containing pyrrolysine has no counterpart in other sets. Following our hypothesis that transmembrane peptides must be well adapted to the lipid environment, it is clear that we observed a kind of peptide coevolution for organisms sharing the same hostile medium. To the best of our knowledge, this is the first time that this type of coevolution was evidenced.
It is important to recall the fact that transmembrane portions of proteins contain a high percentage of hydrophobic residues (necessary to accommodate in membranes) and few or no charge amino acids. Consequently, the alphabet of TMP is limited if compared with that of proteomes. The metrics used in this work can successfully discriminate among TMP regardless the limited compositional variability. If the metric can calculate similarity between sets of TMP, we hypothesized that it could provide insights on AMP. Antimicrobial peptides interacting with membranes, that constitutes the vast majority of linear peptides [31], share the same amino acid frequency of TMP. As matter of example, in Figure 6 we show the ME tree obtained comparing the set of all linear peptides active on B. subtilis with MIC lower than 50 μM, and with activity on erythrocytes higher than 200 μM, with the set of TMP shown in Figure 5.
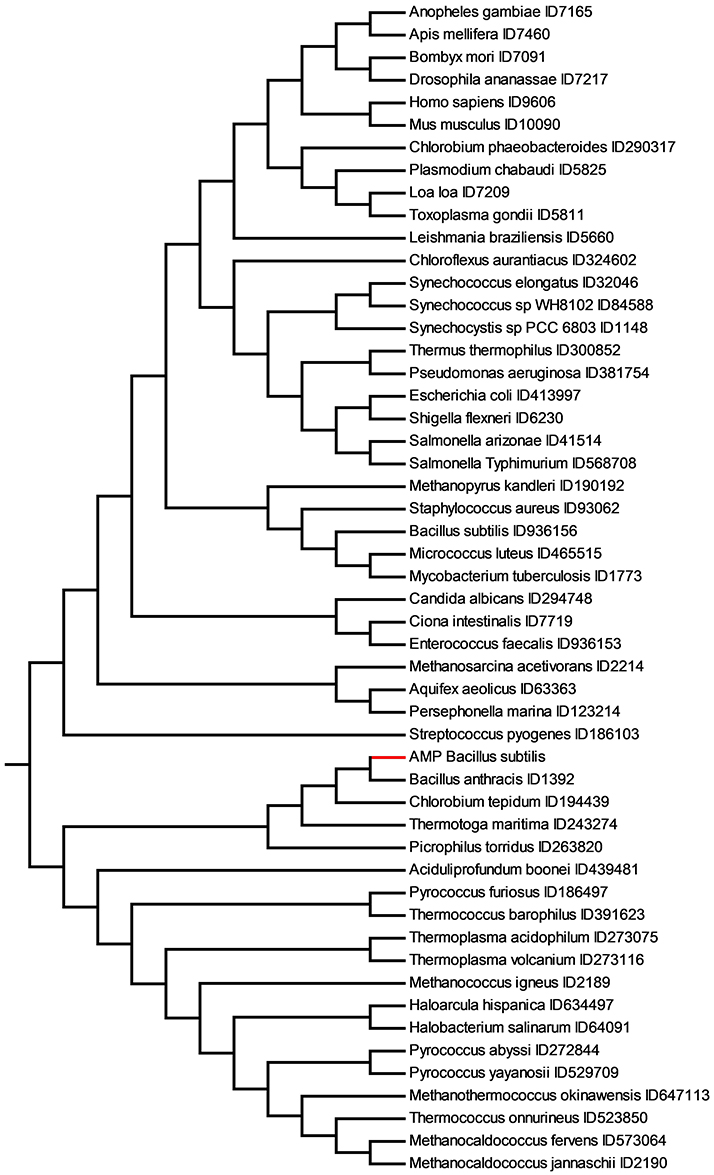
Figure 6. Similarity relationships among the set of 147 AMP active against B. subtilis (AMP Bacillus subtilis) and the TMP dataset obtained with Equation (2) and substring length between 6 and 12. The AMP active on B. subtilis are shown in red.
Eukarya TMP appear very distant from B. subtilis AMP and archaea appear to be more related. Interestingly, the closest TMP to the peptides active on B. subtilis is B. anthracis. These two bacteria have a considerable variation in protein-coding sequences. There are ca. 6,700 genes of B. anthracis predicted by open reading frame (ORF) analysis [51]. About 4,470 of these ORFs have functional assignments based on homology to known genes. The remaining 2,324 ORFs do not have database matches. Approximately 3,250 of the B. anthracis ORFs have orthologs identified in the B. subtilis genomic sequence. This leaves a large number of genes without orthologs in B. subtilis genome.
This kind of analysis may offer novel routes to identify antimicrobial peptides, considering related set of TMP as a sort of peptides mine. We explored this possibility and some results are shown in Figure 7.
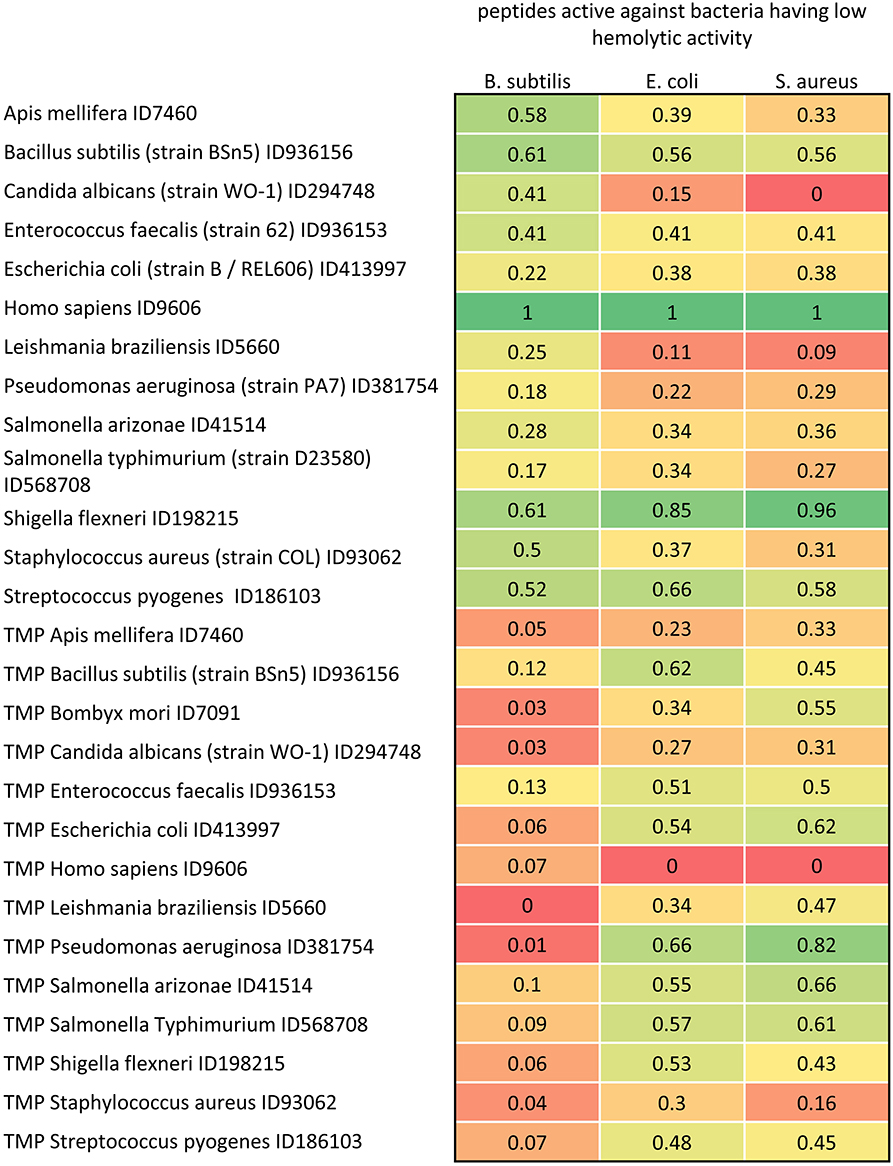
Figure 7. Distance matrix between sets of AMP having low hemolytic activity (MIC0 > 200 μM) active against B. subtilis, E. coli and S. aureus (with MIC0 < 50 μM), and proteome and TMP sets of 13 representative organisms. The distances are scaled from 0 (farthest) to 1 (closest).
In Figure 7, we reported the scaled distances among proteomes, TMP and AMP sets. Proteomes and TMP are taken from four eukarya and nine bacteria. The values represent the distances between TMP and proteomes with the set of AMP extracted by the Yadamp database and active against three common pathogens: B. subtilis, E. coli, and S. aureus. The AMP considered are those with low hemolytic activity only. Interestingly, peptides with the lowest hemolytic activity are those having the minimum distance from the human proteome and the maximum distance from human TMP. It would be easy to consider this distance as a kind of toxicity descriptor for further QSAR analysis. Even more interesting is the observation that this regularity appears with peptide sets active on the three pathogens. The same investigation with other sets of AMP led to the same conclusions and this will be the object of an upcoming paper where the distance herein defined will be used for a statistical analysis. Moreover, the distances between AMP sets and S. flexneri are similar to those of H. sapiens. Following the working hypothesis, this should indicate that peptides active on B. subtilis with low hemolytic activity should be ineffective on S. flexneri. Unfortunately, we do not have enough microbiological data to support this observation. These data could also be used for searching novel potential antimicrobial sequences. For example, S. flexneri could be regarded as a sort of reservoir of potential antimicrobial sequences.
Conclusions
Lipid membranes are complex structures with transversal and lateral asymmetry. Membranes of different organisms, as well as from different organelles, and from different life cycle, exhibit different lipid composition. Thousands of lipid species found in different organisms are a strong warning that membranes are much more than an envelope. From an experimental point of view, the determination of lipid composition is a challenging task, mainly because the composition can vary gradually in the same membrane and the separation of different sub membranes is hardly possible.
The present work is based on the hypothesis that the activity of any peptide acting in or on membranes depends on the peptide sequence and the MPS. The MPS depends on the local lipid composition that is, more often than not, not accessible experimentally. The MPS variations related with temperature and lipid composition are taken into account. To determine the similarity among proteomes and other peptide sets, we exploited an alignment-free method. We have demonstrated the coevolution of transmembrane peptides obtained by phylogenetically uncorrelated organisms. We have also correlated the transmembrane peptide sets with antimicrobial peptides acting on a selection of organisms. For some extents, we have used amino acid sequences of transmembrane peptides to infer information on the MPS and, therefore, we used peptides as bioinformatics sensors of membranes. This approach, though in its early stage, offers unprecedented possibility to design better antimicrobial peptides as well as partially unravels the fundamental role of lipids in organism evolution.
Author Contributions
SP designed the idea, the protocol of the study and wrote the manuscript. LD and SP developed the algorithm. SC validated the method. LS performed the Proteome analysis. All the authors revised the manuscript.
Conflict of Interest Statement
The authors declare that the research was conducted in the absence of any commercial or financial relationships that could be construed as a potential conflict of interest.
Acknowledgments
This research work was financially supported by the Italian Minister of Instruction, University and Research (MIUR)−300395FRB16.
Supplementary Material
The Supplementary Material for this article can be found online at: https://www.frontiersin.org/article/10.3389/fphy.2018.00048/full#supplementary-material
References
1. Sezgin E, Levental I, Mayor S, Eggeling C. The mystery of membrane organization: composition, regulation and roles of lipid rafts. Nat Rev Mol Cell Biol. (2017) 18:361. doi: 10.1038/nrm.2017.16
2. Harayama T, Riezman H. Understanding the diversity of membrane lipid composition. Nat Rev Mol Cell Biol. (2018) 19:281–96. doi: 10.1038/nrm.2017.138
3. Vigh L, Escribá PV, Sonnleitner A, Sonnleitner M, Piotto S, Maresca B, et al. The significance of lipid composition for membrane activity: new concepts and ways of assessing function. Prog Lipid Res. (2005) 44:303–44. doi: 10.1016/j.plipres.2005.08.001
4. Török Z, Crul T, Maresca B, Schütz GJ, Viana F, Dindia L, et al. Plasma membranes as heat stress sensors: from lipid-controlled molecular switches to therapeutic applications. Biochimica et Biophysica Acta (2014) 1838:1594–618. doi: 10.1016/j.bbamem.2013.12.015
5. Casas J, Ibarguren M, Álvarez R, Terés S, Lladó V, Piotto SP, et al. G protein-membrane interactions II: effect of G protein-linked lipids on membrane structure and G protein-membrane interactions. Biochim Biophys Acta (2017) 1859:1526–35. doi: 10.1016/j.bbamem.2017.04.005
6. Escribà PV, González-Ros JM, Goñi FM, Kinnunen PK, Vigh L, Sánchez-Magraner L, et al. Membranes: a meeting point for lipids, proteins and therapies. J Cell Mol Med. (2008) 12:829–75. doi: 10.1111/j.1582-4934.2008.00281.x
7. Fermor BF, Masters JR, Wood CB, Miller J, Apostolov K, Habib NA. Fatty acid composition of normal and malignant cells and cytotoxicity of stearic, oleic and sterculic acids in vitro. Eur J Cancer (1992) 28A:1143–7. doi: 10.1016/0959-8049(92)90475-H
8. Nakada T, Kwee IL, Ellis WG. Membrane fatty acid composition shows Δ6-desaturase abnormalities in Alzheimer's disease. Neuroreport (1990) 1:153–5. doi: 10.1097/00001756-199010000-00018
9. Parasassi T, Di Stefano M, Ravagnan G, Sapora O, Gratton E. Membrane aging during cell growth ascertained by Laurdan generalized polarization. Exp Cell Res. (1992) 202:432–9. doi: 10.1016/0014-4827(92)90096-Q
10. Alvarez E, Ruiz-Gutiérrez V, Santa María C, Machado A. Age-dependent modification of lipid composition and lipid structural order parameter of rat peritoneal macrophage membranes. Mech Ageing Dev. (1993) 71:1–12. doi: 10.1016/0047-6374(93)90030-U
11. Burns M, Wisser K, Wu J, Levental I, Veatch SL. Miscibility transition temperature scales with growth temperature in a zebrafish cell line. Biophys J. (2017) 113:1212–22. doi: 10.1016/j.bpj.2017.04.052
12. Vígh L, Török Z, Balogh G, Glatz A, Piotto S, Horváth I. (2007). Membrane-regulated stress response. Adv Exp Med Biol. 594:114–131. doi: 10.1007/978-0-387-39975-1_11
13. Blouin CM, Hamon Y, Gonnord P, Boularan C, Kagan J, de Lesegno CV, et al. Glycosylation-dependent IFN-gamma R partitioning in lipid and actin nanodomains is critical for JAK activation. Cell (2016) 166:920–34. doi: 10.1016/j.cell.2016.07.003
14. Baeza-Delgado C, Von Heijne G, Marti-Renom MA, Mingarro I. Biological insertion of computationally designed short transmembrane segments. Sci Rep. (2016) 6:23397. doi: 10.1038/srep23397
15. Grau B, Javanainen M, García-Murria MJ, Kulig W, Vattulainen I, Mingarro I, et al. The role of hydrophobic matching on transmembrane helix packing in cells. Cell Stress (2017) 1:90–106. doi: 10.15698/cst2017.11.111
16. Zaiou M, Nizet V, Gallo RL. Antimicrobial and protease inhibitory functions of the human cathelicidin (hCAP18/LL-37) prosequence. J Invest Dermatol. (2003) 120:810–6. doi: 10.1046/j.1523-1747.2003.12132.x
17. Zanetti M. Cathelicidins, multifunctional peptides of the innate immunity. J Leukoc Biol. (2004) 75:39–48. doi: 10.1189/jlb.0403147
18. Brender JR, McHenry AJ, Ramamoorthy A. Does cholesterol play a role in the bacterial selectivity of antimicrobial peptides? Front Immunol. (2012) 3:195. doi: 10.3389/fimmu.2012.00195
19. Hazel JR, Williams EE. The role of alterations in membrane lipid composition in enabling physiological adaptation of organisms to their physical environment. Prog Lipid Res. (1990) 29:167–227. doi: 10.1016/0163-7827(90)90002-3
20. Cantor RS. Lipid composition and the lateral pressure profile in bilayers. Biophys J. (1999) 76:2625–39. doi: 10.1016/S0006-3495(99)77415-1
21. Concilio S, Ferrentino I, Sessa L, Massa A, Iannelli P, Diana R, et al. A novel fluorescent solvatochromic probe for lipid bilayers. Supramol Chem. (2017) 29:887–95. doi: 10.1080/10610278.2017.1372583
22. Cantor RS. The lateral pressure profile in membranes: a physical mechanism of general anesthesia. Biochemistry (1997) 36:2339–44. doi: 10.1021/bi9627323
23. Franová MD, Vattulainen I, Ollila OS. Can pyrene probes be used to measure lateral pressure profiles of lipid membranes? Perspective through atomistic simulations. Biochimica et Biophysica Acta (2014) 1838:1406–11. doi: 10.1016/j.bbamem.2014.01.030
24. Brügger B. Lipidomics: analysis of the lipid composition of cells and subcellular organelles by electrospray ionization mass spectrometry. Annu Rev Biochem. (2014) 83:79–98. doi: 10.1146/annurev-biochem-060713-035324
25. Piotto S, Trapani A, Bianchino E, Ibarguren M, López DJ, Busquets X, et al. The effect of hydroxylated fatty acid-containing phospholipids in the remodeling of lipid membranes. Biochimica et Biophysica Acta (2014) 1838:1509–17. doi: 10.1016/j.bbamem.2014.01.014
26. Lorent JH, Diaz-Rohrer B, Lin X, Spring K, Gorfe AA, Levental KR, et al. (2017). Structural determinants and functional consequences of protein affinity for membrane rafts. Nat Commun. 8:1219. doi: 10.1038/s41467-017-01328-3
27. White SH, Wimley WC. Hydrophobic interactions of peptides with membrane interfaces. Biochimica et Biophysica Acta (1998) 1376:339–52. doi: 10.1016/S0304-4157(98)00021-5
28. Teixeira V, Feio MJ, Bastos M. Role of lipids in the interaction of antimicrobial peptides with membranes. Prog. Lipid Res. (2012) 51:149–77. doi: 10.1016/j.plipres.2011.12.005
29. Strandberg E, Tiltak D, Ehni S, Wadhwani P, Ulrich AS. Lipid shape is a key factor for membrane interactions of amphipathic helical peptides. Biochimica et Biophysica Acta (2012) 1818:1764–76. doi: 10.1016/j.bbamem.2012.02.027
30. Sohlenkamp C, Geiger O. Bacterial membrane lipids: diversity in structures and pathways. FEMS Microbiol Rev. (2016) 40:133–59. doi: 10.1093/femsre/fuv008
31. Piotto SP, Sessa L, Concilio S, Iannelli P. YADAMP: yet another database of antimicrobial peptides. Int. J. Antimicrob. Agents (2012) 39:346–51. doi: 10.1016/j.ijantimicag.2011.12.003
32. Cedano J, Aloy P, Perez-Pons JA, Querol E. Relation between amino acid composition and cellular location of proteins. J Mol Biol. (1997) 266:594–600. doi: 10.1006/jmbi.1996.0804
33. Tusnady GE, Simon I. Principles governing amino acid composition of integral membrane proteins: application to topology prediction. J Mol Biol. (1998) 283:489–506. doi: 10.1006/jmbi.1998.2107
34. Senes A, Gerstein M, Engelman DM. Statistical analysis of amino acid patterns in transmembrane helices: the GxxxG motif occurs frequently and in association with β-branched residues at neighboring positions. J Mol Biol. (2000) 296:921–36. doi: 10.1006/jmbi.1999.3488
35. Zielezinski A, Vinga S, Almeida J, Karlowski WM. Alignment-free sequence comparison: benefits, applications, and tools. Genome Biol. (2017) 18:186. doi: 10.1186/s13059-017-1319-7
36. Searls DB. Reading the book of life. Bioinformatics. (2001) 17:579–80. doi: 10.1093/bioinformatics/17.7.579
37. Zuckerkandl E, Pauling L. Evolutionary divergence and convergence in proteins. Evol Genes Proteins (1965) 97:97–166. doi: 10.1016/B978-1-4832-2734-4.50017-6
38. Schmollinger M, Nieselt K, Kaufmann M, Morgenstern B. DIALIGN P: fast pair-wise and multiple sequence alignment using parallel processors. BMC Bioinformatics (2004) 5:128. doi: 10.1186/1471-2105-5-128
39. Felsenstein J. Numerical methods for inferring evolutionary trees. Q Rev Biol. (1982) 57:379–404. doi: 10.1086/412935
40. Attwood TK. The Babel of bioinformatics. Science (2000) 290:471–3. doi: 10.1126/science.290.5491.471
41. Sims GE, Jun S-R, Wu GA, Kim S-H. Alignment-free genome comparison with feature frequency profiles (FFP) and optimal resolutions. Proc Natl Acad Sci USA. (2009) 106:2677–82. doi: 10.1073/pnas.0813249106
42. Käll L, Krogh A, Sonnhammer EL. A combined transmembrane topology and signal peptide prediction method. J Mol Biol. (2004) 338:1027–36. doi: 10.1016/j.jmb.2004.03.016
43. Rzhetsky A, Nei M. A simple method for estimating and testing minimum-evolution trees. Mol Biol Evol. (1992) 9:945–67.
44. Nei M, Kumar S. Molecular Evolution and Phylogenetics. New York, NY: Oxford university press (2000).
45. Kumar S, Stecher G, Tamura K. MEGA7: molecular evolutionary genetics analysis version 7.0 for bigger datasets. Mol Biol Evol. (2016) 33:1870–4. doi: 10.1093/molbev/msw054
46. Snel B, Bork P, Huynen MA. Genome phylogeny based on gene content. Nat Genet. (1999) 21:108–10. doi: 10.1038/5052
48. Korbel JO, Snel B, Huynen M, Bork P. SHOT: a web server for the construction of genome phylogenies. Trends Genet. (2002) 18:158–62. doi: 10.1016/S0168-9525(01)02597-5
49. Corcelli A The cardiolipin analogues of Archaea. Biochim Biophys Acta (2009) 1788:2101–6. doi: 10.1016/j.bbamem.2009.05.010
50. Vinçon-Laugier A, Cravo-Laureau C, Mitteau I, Grossi V. Temperature-dependent alkyl glycerol ether lipid composition of mesophilic and thermophilic sulfate-reducing bacteria. Front Microbiol. (2017) 8:1532. doi: 10.3389/fmicb.2017.01532
Keywords: peptides, membrane physical state, AMP, TMP, alignment-free, proteome
Citation: Piotto S, Di Biasi L, Sessa L and Concilio S (2018) Transmembrane Peptides as Sensors of the Membrane Physical State. Front. Phys. 6:48. doi: 10.3389/fphy.2018.00048
Received: 17 January 2018; Accepted: 03 May 2018;
Published: 24 May 2018.
Edited by:
Pushpendra Singh, Johns Hopkins University, United StatesReviewed by:
Erdinc Sezgin, University of Oxford, United KingdomJesus Perez-Gil, Complutense University of Madrid, Spain
Rajeshwer Singh Sankhala, Walter Reed Army Institute of Research, United States
Copyright © 2018 Piotto, Di Biasi, Sessa and Concilio. This is an open-access article distributed under the terms of the Creative Commons Attribution License (CC BY). The use, distribution or reproduction in other forums is permitted, provided the original author(s) and the copyright owner are credited and that the original publication in this journal is cited, in accordance with accepted academic practice. No use, distribution or reproduction is permitted which does not comply with these terms.
*Correspondence: Stefano Piotto, piotto@unisa.it