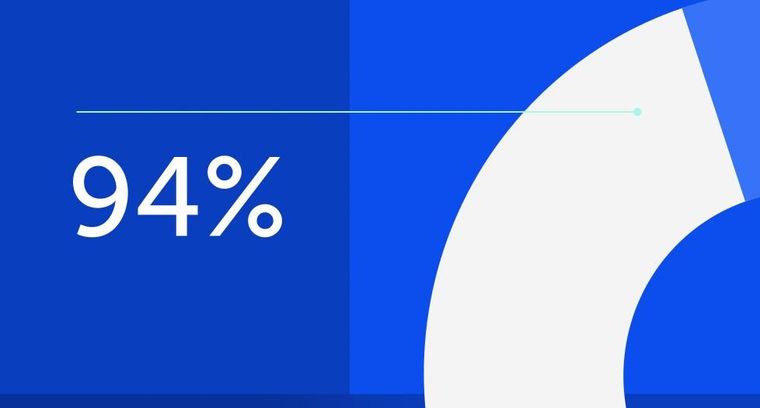
94% of researchers rate our articles as excellent or good
Learn more about the work of our research integrity team to safeguard the quality of each article we publish.
Find out more
ORIGINAL RESEARCH article
Front. Pharmacol., 22 April 2020
Sec. Pharmacogenetics and Pharmacogenomics
Volume 11 - 2020 | https://doi.org/10.3389/fphar.2020.00324
Pharmacogenetic analysis has generated translational data that could be applied to guide treatments according to individual genetic variations. However, pharmacogenetic counseling in some mestizo (admixed) populations may require tailoring to different patterns of admixture. The identification and clustering of individuals with related admixture patterns in such populations could help to refine the practice of pharmacogenetic counseling. This study identifies related groups in a highly admixed population-based sample from Mexico, and analyzes the differential distribution of actionable pharmacogenetic variants. A subsample of 1728 individuals from the Mexican Genomic Database for Addiction Research (MxGDAR/Encodat) was analyzed. Genotyping was performed with the commercial PsychArray BeadChip, genome-wide ancestry was estimated using EIGENSOFT, and model-based clustering was applied to defined admixture groups. Actionable pharmacogenetic variants were identified with a query to the Pharmacogenomics Knowledge Base (PharmGKB) database, and functional prediction using the Variant Effect Predictor (VEP). Allele frequencies were compared with chi-square tests and differentiation was estimated by FST. Seven admixture groups were identified in Mexico. Some, like Group 1, Group 4, and Group 5, were found exclusively in certain geographic areas. More than 90% of the individuals, in some groups (Group 1, Group 4 and Group 5) were found in the Central-East and Southeast region of the country. MTRR p.I49M, ABCG2 p.Q141K, CHRNA5 p.D398N, SLCO2B1 rs2851069 show a low degree of differentiation between admixture groups. ANKK1 p.G318R and p.H90R, had the lowest allele frequency of Group 1. The reduction in these alleles reduces the risk of toxicity from anticancer and antihypercholesterolemic drugs. Our analysis identified different admixture patterns and described how they could be used to refine the practice of pharmacogenetic counseling for this admixed population.
In recent years, pharmacogenetic (PGx) studies have generated substantial information that is useful in clinical settings (Relling and Evans, 2015; Van Der Wouden et al., 2016; Verbelen et al., 2017). PGx variation influences the efficacy and toxicity of drugs through the alteration of pharmacodynamic or pharmacokinetic processes. Pharmacogenetic studies have uncovered many relationships between drugs and specific genes, but not all of this information can be used to implement PGx-based treatment guidelines. Various initiatives have attempted to compile information and develop PGx evidence-based drug dosing guidelines, like the Pharmacogenomics Knowledge Base (PharmGKB; (Thorn et al., 2013)). The PharmGKB classifies pharmacogenes into four different evidence levels (Barbarino et al., 2018), where pharmacogenes at higher levels are termed “actionable”–that is, they can be used in treatment guidelines for PGx-based counseling.
Most pharmacogenetic studies have been carried out on individuals with a low degree of admixture. Actionable allele frequencies are dependent on ancestry, and such differences must be taken into account in clinical counseling (Abdul Jalil et al., 2015; Mizzi et al., 2016; Goh et al., 2017). A recent analysis of PGx variation across 26 global populations using data from Phase 3 of the 1000 Genomes Project identified clusters of individuals by continent, with high degrees of differentiation even among continental populations (Wright et al., 2018). Researchers have expressed concerns about the assignment of ancestry in PGx analysis, principally because of its potential impact on the differentiation of genetic variants among continental populations (Zhang and Finkelstein, 2019).
Mexico has a differential pattern of ancestry, made up primarily of three populations: Native American, European, and African (Wang et al., 2008; Moreno-Estrada et al., 2014). Its genomic ancestry has been divided into Native American (NA) and Mexican Mestizo (MM) classifications (Silva-Zolezzi et al., 2009). Differences have been reported between these two groups in actionable PGx variants, including greater allele frequency of VKORC1 (rs8050894), CYP2B6 (rs2279343), and CYP3A5 (rs776746) in the NA population, and of CYP2C19 (rs4244285), CYP2C9 (rs1799853, rs1057910), NAT2 (rs179930), SLCO1B1 (rs4149015), and APOE (rs7412) in the MM population (Gonzalez-Covarrubias et al., 2019). The MM population is the largest in Mexico, and the prevalence of admixture patterns in this population is high. Grouping all MM individuals together could thus hide differences in allele frequencies. These differences have not been estimated for the MM population based on the degree of admixture or the distribution of the genome-wide global ancestry. However, an analysis of the admixture patterns and clustering of individuals with similarities could improve pharmacogenetic counseling for individuals in this population. The aim of the present study is thus to analyze global admixture patterns in a population-based Mexican sample in order to assess their impact on actionable pharmacogenetic variants.
This study analyzed a subsample of the Mexican Genomic Database for Addiction Research (MxGDAR/Encodat), derived from the Mexican National Survey of Tobacco, Alcohol, and Drug use (Villatoro-Velázquez et al., 2017). All of the geographic regions where more than half of the population speaks a Native American (NA) language were excluded (Villarreal, 2014). The survey was carried out in two phases, with the sampling performed in the second phase. There were questionnaires in each phase: the first focused on sociodemographic, social, and interpersonal information, with a section on patterns of alcohol, tobacco, and drug use (Reséndiz-Escobar et al., 2018), and the second on screening for psychiatric symptomatology (Pato et al., 2013). In the second phase, a sample of buccal epithelial cells was also collected (Figure 1). All of the protocols in this study were approved by the Research Ethics Committees of the Instituto Nacional de Psiquiatría Ramón de la Fuente Muñiz (Approval No. CEI/C/083/2015) and the Instituto Nacional de Medicina Genómica (Approval No. 01/2017/I). The aims of the study were explained to each participant, and each was informed that they could end their participation at any time. All participants provided written informed consent; assent for a minor participant was obtained both from the participant and from a parent or legal guardian.
DNA was extracted using a commercial modified salting-out procedure (Qiagen, USA), according to the manufacturer’s instructions. DNA extraction quality and integrity were evaluated by analysis with a NanoDrop spectrophotometer (Thermofisher, USA) and 2% agarose gel. The MxGDAR/Encodat database included 7171 of the 13,228 buccal epithelial samples collected (54.21%) that met the following quality criteria: i) 230/260 and 260/230 ratios > 1.8, ii) concentration > 50 ng/µL, and iii) no signs of DNA degradation. The DNA extraction was divided evenly between the Laboratorio de Genómica de Enfermedades Psiquiátricas y Neurodegenerativas of the Instituto Nacional de Medicina Genómica and the Departamento de Farmacogenómica of the Instituto Nacional de Psiquiatría Ramón de la Fuente Muñiz. We found no difference in the quality of microarray samples between the laboratories; the insufficient quality of half of the samples could have been an effect of problems in sample collection procedures. In a household survey like the Encodat 2016, collecting biological samples is problematic because it cannot be done under the controlled conditions of a clinical environment (Gudiseva et al., 2016).
Genotyping was performed with the commercial microarray PsychArray BeadChip (Illumina, USA), according to the manufacturer’s instructions. In this preliminary analysis we genotyped a total of 1728 samples, with a random sampling that included at least 15 individuals from each of the 32 states in Mexico. The fluorescent intensities were read with the iScan system (Illumina, USA) and converted to genotype calls with Genome Studio software (Illumina, USA). Genotyping was carried out at the Unidad de Alta Tecnología para Expresión y Microarreglos of the Instituto Nacional de Medicina Genómica. The quality control of the genotyped data was performed using Plink (Purcell et al., 2007). Single nucleotide polymorphisms (SNPs) were excluded if they did not meet the following criteria: i) no-call rate > 5%, ii) minor allele frequency (MAF) < 5%, iii) p-value < 1E-06 in a chi-square test for Hardy-Weinberg equilibrium, or iv) they were duplicates. Individuals were excluded if they had no call rate > 5%. To identify cryptic familial relationships, we performed an identity-by-state (IBS): all individuals with an IBS > 1.6 were marked and those with the lower genotyping rate were excluded. This quality control left 1657 individuals for analysis.
Global ancestry estimations were performed with a genome-wide approach. For this purpose, the SNPs of the 1657 individuals that remained after quality filters were then filtered for independence. The linkage disequilibrium (LD) pruning algorithm was implemented in Plink, with a window size of 50 kb, a step size of 5, and a variance inflation factor (VIF) of 2. LD pruning left a total of 104,726 SNPs for analysis. The database was then merged with the SNPs of the Human Genome Diversity Project (HGDP; (Cavalli-Sforza, 2005)), and the SNPs not present in either database were excluded, leaving a total of 25,562 SNPs for genome-wide global ancestry estimations. EIGENSOFT (Price et al., 2006), was used to calculate ten global ancestry components. In this estimation, 133 individuals with values greater than three standard deviations were excluded, leaving 1524 individuals for analysis. The 133 excluded individuals had admixture proportions of European ancestry greater than 96%, as estimated through a model-based analysis with ADMIXTURE software (Scheet and Stephens, 2006). We removed these individuals, because we also filtered the communities with higher NA ancestry during the sampling procedure, to not deviate the calculation of admixture patterns to the founders’ populations. The sociodemographic characteristics of the sample are shown in Table 1.
Next, a model-based clustering algorithm using mclust software (Scrucca et al., 2016) carried out Gaussian mixture modeling to identify admixture groups in the subsample of 1524 individuals. A geographic distribution of the groups was then determined for the following regions of Mexico: i) Northwest (Baja California, Baja California Sur, Nayarit, Sinaloa, and Sonora), ii) North (Coahuila, Chihuahua, Durango, Nuevo León, San Luis Potosí, Tamaulipas, and Zacatecas), iii) Central-West (Aguascalientes, Colima, Jalisco, and Michoacán), iv) Central-East (Mexico City, Guerrero, Hidalgo, Estado de México, Morelos, Puebla, Quéretaro, and Tlaxcala), and v) Southeast (Campeche, Chiapas, Oaxaca, Quintana Roo, Tabasco, Veracruz, and Yucatán) (Borges et al., 2018).
Variants in genes with actionable pharmacogenetic effects were then identified using the hg19 coordinates for those genes. Genes were included for evidence levels of 1 to 2, according to the classification of the PharmGKB (Barbarino et al., 2018), a searchable pharmacogenetic knowledge database that categorizes genes according to levels of evidence for alterations in their response to different drugs, and that provides pharmacogenetic treatment guidance where those levels are high. For genes with evidence levels of 1 or 2, PsychArray has a total of 7955 single-nucleotide variants (65.83% of the total reported in the 1000 Genomes Database), of which 5809 in our population (73.02%) had an MAF < 0.05, 185 (23.33%) were excluded for a low call rate, and 11 (0.14%) were excluded for Hardy-Weinberger disequilibrium (Supplementary Table 1 contains the list of variants lost in these filtering steps). Some important pharmacogenetic variation was lost during these filtering processes, like those found in CYP2D6 (evidence level 1A). Of the twelve variants design on CYP2D6 that could be found in the PsychArray, 10 were lost in the MAF, and 2 in the call rate filtering.
We extracted the variants in the genes with actionable pharmacogenetic effect with annotations in the Ensembl Variant Effect Predictor (McLaren et al., 2016), and extracted all the variants that were missense (classifying these as damaging if a damaging effect was predicted by both SIFT and Polyphen2, but benign if only one algorithm predicted a damaging effect), synonymous, or annotated with a regulatory region. For regulatory variants, we extracted those with an annotated regulatory region based in the ENCODE identifier. A manual search was performed in PharmGKB for the evidence level for the benign missense, synonymous, and regulatory variants; for annotated variants a search was performed in PubMed for reports of at least 10 associations with drug-variant relationships. The global allele frequency of each actionable pharmacogenetic missense variant in the MxGDAR/Encodat was compared with those identified in the Genome Aggregation Database (gnomAD; (Kobayashi et al., 2017)) using a chi-square test and a delta de MAF calculation (dMAF). The degree of differentiation of variants between the admixture subgroups was analyzed with Wright’s fixation index (FST; (Patterson et al., 2006)).
An analysis of global ancestry found the distribution of European to Native American ancestry of individuals in the MxGDAR/Encodat (Figure 2A), and an analysis of admixture groups detected seven groups (Figure 2B). Groups 2 and 3 show the greatest frequency, with a total of 821 (53.87%) individuals in the two groups (Table 2). Groups 2, 3, and 7 have a heterogeneous distribution in different regions, with no local concentration. Groups 4 and 5, with a total of 270 (17.72%) individuals, are concentrated in the Southeast, with more than 90% of the individuals in each group in that region (Figure 2C). The 170 individuals in Group 1 (72.96%) are in the Central-East and Southeast, and the 160 (94.67%) in Group 6 are in the Northwest, North, and Central-West regions. Groups 1 and 4 are the most closely related to the Native American population (Figure 2B).
Figure 2 Admixture analysis of the MxGDAR/Encodat. Panels: (A) Principal component analysis with the Human Genome Diversity Project Database (HGDP) admixture reference; individuals from the MxGDAR/Encodat in red and individuals from the HGDP database in gray; (B) Plotting of the individuals from the MxGDAR/Encodat (those in red in panel A), color according to the admixture group identified by Gaussian model-based clustering; (C) Analysis of the geographical distribution of the identified admixture subgroups in each region. Each pie represents the percentages of admixture groups identified in that particular region.
Analysis of the actionable pharmacogenetic missense variants found a total of 32 variants in 31 genes, four of which have a PharmGkB evidence level of 1 (Table 3): SLCO1B1*5, NUDT15*3, and CYP4F2*3 with evidence level 1A, and XPC p.Q939K with level 1B. The difference in minor allele frequency (dMAF) in these variants between the individuals in the MxGDAR/Encodat and the gnomAD range from -0.27 to -0.36 and are statistically significant. The variant with the greatest difference, -0.36, was XPC p.Q939K. NUDT15*3 was the only variant with a greater allele frequency in the MxGDAR/Encodat than in the gnomAD, with a difference of 0.45.
There were 28 variants at the PharmGkB evidence level 2 (Table 4), 13 of which were at level 2A (MTHFR p.A22V, FCGR2A p.H166R, UGT1A1*6, ADRB2 p.G71R, ABCB1*2, NAT2*6, CYP2C8*3, KCNJ11 p.K23E, GSTP1 p.I188V, VDR p.M51T, NQO1 p.P187S, APOE-E2, and COMT p.V158M), and 15 at level 2B (EPHX1 p.Y113H and p.H139R, UMPS p.G213A, ADD1 p.G460W, UGT2B15*2, MTRR p.I49M, OPRM1 p.N40D, SOD2 p.V16A, CHRNA5 p.D398N, GP1BA p.T161M, XRCC1 p.Q399R, ERCC1 p.Q506K, ITPA p.P32T, CBR3 p.V244M, and PNPLA3 p.I148M). There were differences in allele frequencies ranging from -0.45 to 0.18 between the MxGDAR/Encodat and the gnomAD. The NQO1 p.P187S variant showed the greatest positive difference in minor allele frequency (dMAF = 0.1835) in the MxGDAR/Encodat, and the XRCC1 p.Q399R the greatest negative difference (dMAF = -0.45). The variants MTHFR p.A22V, GSTP1 p.I188V, NQO1 p.P187S, UMPS p.G213A, ERCC1 p.Q506K, and PNPLA3 p.I148M showed the largest positive differences in the MXGDAR/Encodat, and NAT2*6, KCNJ11 p.K23E, VDR p.M51T, EPHX1 p.Y113H, UGT2B15*2, MTRR p.I49M, SOD2 p.V16A, CHRNA5 p.D398N, XRCC1 p.Q399R, and CBR3 p.V244M the largest negative differences.
A total of 427 synonymous, benign, and regulatory variants were identified, only seven of which had been previously been associated with drug response (Table 5). Of these seven, there was one at evidence level 1A (CYP2C19*2), three at level 2A (ABCG2 p.Q141K, NAT2*7), and three at level 2B (GNB3 p.S274S, GP1BA p.T161M, NEDD4L p.Q8Q). The differences in minor allele frequency between the MxGDAR/Encodat and the gnomAD ranged from -0.1820 to 0.1387. The synonymous variant GNB3 p.S274S had the largest negative difference (dMAF = -0.1820), and the variant ABGCG2 p.Q141K the largest positive difference (dMAF = 0.1387).
Table 5 Synonymous, benign, and regulatory actionable pharmacogenetic variation in the MxGDAR/Encodat.
An analysis of differentiation of the identified actionable pharmacogenetic variants in the different admixture groups found a total of 105 PGx variants with a low degree of differentiation (FST > 0.01), of which 36 (34.29%) had a regulatory or synonymous function and 69 (65.71%) were missense variants (Figure 3). Of the latter, 20 variants had a FST > 0.03. In Groups 1 and 4, both with regional distribution (one in the Central-East region, and the other in the Southeast; see Figures 4 and 2C),15 of the 20 variants had lower frequencies, and five higher frequencies.
Figure 4 Differences in minor allelic frequencies (MAF) of the PGx variants with FST > 0.03: (A) MAF differences of the damaging missense variants between admixture groups; (B) MAF differences of regulatory, benign or synonymous variants between admixture groups.
The analysis of actionable pharmacogenetic variants in admixed populations has been centered on genes or groups recruited in specific regions (Bonifaz-Peña et al., 2014; Cuautle-Rodríguez et al., 2014; Gonzalez-Covarrubias et al., 2016; Gonzales-Covarrubias et al., 2017). The present study is one of the first to analyze the distribution of known actionable PGx variants in a population-based sample for all states of Mexico, and examining the possible contributions a consideration of admixture patterns could make to pharmacogenetic counseling. The analysis found that 50 actionable PGx variants (96.15%) were different in the MxGDAR/Encodat database than in the gnomAD database for the global population. The following sections will discuss the implications of these differences for the pharmacological parameters of response, toxicity, and dosage requirements, by PharmGKB evidence level.
The XPC p.Q939K variant, with evidence level 1B, reduces toxicity to individuals treated with platinum-based compounds (Caronia et al., 2009; Sakano et al., 2010). It was more than 30% less prevalent in the Mexican population, which could mean increased risk for toxicity. The only variant with evidence level 1 that showed greater MAF in the MxGDAR/Encodat was NUDT15*3 (dMAF = 0.0452), a variant that may increase the risk of leukopenia or neutropenia in those individuals treated with purine compounds (Yang et al., 2014). Differences were also found in CYP2C19*2, which has treatment guidelines that depend on different drug-gene relationships, and which had a lower MAF, which could mean a reduced response to drugs like escitalopram, citalopram, and clopidogrel. The reduction of this allele has been previously reported in a sample from western Mexico (Favela-Mendoza et al., 2015).
The variants with evidence level 2, ABCG2 p.Q141K, MTHFR p.A22V, GSTP1 p.I188V, NQO1 p.P187S, UMPS p.G213A, ERCC1 p.Q506K, and PNPLA3 p.I148M, showed higher allele frequencies in the Mexican population. These variants could affect the treatment of cancer patients. MTHFR p.A22V could have a greater degree of toxicity and decreased response in treatments with methotrexate and platinum-based compounds (Huang et al., 2008; López-Rodríguez et al., 2018), GSTP1 p.I188V could lessen the response to cyclophosphamide, epirubucin, uracil-based, and platinum-based compounds (Oliveira et al., 2010; Zhang et al., 2011), and NQO1 p.P187S could diminish the response to anthracyclines and uracil-based compounds (Fagerholm et al., 2008). PNPLA3 p.I148M could increase hepatotoxicity in individuals treated with asparaginase, ciclophosphamide, daunorubicin, prednisolone, or vincristine (Chambers et al., 2011; Gutierrez-Camino et al., 2017), while ERCC1 p.Q506K could decrease nephrotoxicity in patients treated with platinum-based compounds (Tzvetkov et al., 2011). UMPS p.G213A could increase the toxicity of the cancer drugs capacetabine and fluorouracil (Tsunoda et al., 2011) and of leucovirin, an agent used to reduce the effect of methotrexate treatment. ABCG2 p.Q141K increases the plasma concentration of rosuvastatin, which could reduce its effect in the Mexican population (Tomlinson et al., 2010).
The variants NAT2*6, KCNJ11 p.K23E, VDR p.M51T, EPHX1 p.H139R, UGT2B15*2, MTRR p.I49M, SOD2 p.V16A, CHRNA5 p.D398N, XRCC1 p.Q399R, GNB3 p.S274S, NEDD4L p.Q8Q, and CBR3 p.V244M all had reduced MAF in the Mexican population. These variants could potentially affect a variety of drugs, including those for cancer, psychiatric illness, tuberculosis, viruses, and diabetes. The reduction in the frequency of the NAT2*6 allele could reduce hepatotoxicity in treatment with ethambutol, pyramizid, and rifampin (Kim et al., 2009; Ben Mahmoud et al., 2012), and KCNJ11 p.K23E could increase the response to glibenclamide and sulfonamides (Javorsky et al., 2012). The reduced MAF of VDR p.M51T could increase response rates to peginterferon and ribavirin (García-Martín et al., 2013; El-Derany et al., 2016). The reduction of EPHX1 p.H139R could result in lower dose requirements for the psychiatric drug carbamazepine (Kamiya et al., 2005; Hung et al., 2012; Puranik et al., 2013), and that of UGT2B15*2 could reduce the clearance of lorazepam and oxazepam (He et al., 2009; Bhatt et al., 2019). For oncology patients, MTRR p.I49M could reduce the toxicity of metrotrexate (López-Rodríguez et al., 2018), XRCC1 p.Q399R could increase the response to platinum-based compounds (Yin et al., 2012), and SOD2 p.V16A could increase survival in those treated with cyclophosphamide (Glynn et al., 2009). CBR3 p.V244M could increase the risk of cardiac damage from anthracyclines (Blanco et al., 2008; Gonzalez-Covarrubias et al., 2008). Interestingly, the variant CHRNA5 p.D398N could reduce the dependence on nicotine in the Mexican population (Johnson et al., 2010; Gelernter et al., 2015; Hancock et al., 2017), which could help to explain the lower rates of cigarette consumption reported for Latino populations (Pagano et al., 2018). Further studies are needed to explore the effect of this variant on nicotine dependence.
The admixture of the Mexican population has been divided into two populations: Native Americans (NA) and Mexican Mestizos (MM) (Wang et al., 2008; Moreno-Estrada et al., 2014). There have been some attempts to identify how variants in the NA population affect pharmacology (Gonzalez-Covarrubias et al., 2016; Romero-Hidalgo et al., 2017; Gonzalez-Covarrubias et al., 2019), but in many analyses the entire MM population is grouped together, with no estimation of how admixture patterns within that group could affect the frequency of actionable pharmacogenetic variants or clinical counseling.
In the present study, a global ancestry model-based clustering algorithm identified seven admixture groups, some of which had a particular distribution in specific geographic areas in Mexico. Groups 4 and 5 were found only in the Central-East and Southeast, and Group 1 in the Northwest, North, and Central-West regions. Groups 1 and 4 are the most closely related to the NA population. The mapping of geographically dependent admixture patterns has been analyzed in the African-American and Latino populations in the United States (Bryc et al., 2015). Bryc et al., found that specific admixture groups of these populations were more prevalent in some regions, and they also reported that self-identification (used mainly in epidemiological studies) did not correlate with individuals’ ancestry. The identification of admixture patterns could help to more accurately identify individual ancestry and aid with pharmacogenetic counseling and treatment decisions guided by genomics.
The present study found 20 actionable Pgx variants with possible dependence on admixture patterns. The variants MTRR p.I49M and NAT2*6 are less frequent in individuals from Groups 1 and 4 (those with a greater degree of NA ancestry) and could predict a reduction in toxicity in cancer patients treated with methotrexate, reduce the risk of hepatotoxicity in tuberculosis patients, and the risk in its use for treatment of acute lymphoblastic leukemia (Gast et al., 2007; Huang et al., 2008), for which it is one of the main drugs used in Mexico (Medellin-Garibay et al., 2019). Another variant with a substantial difference in MAF between admixture groups was ABCG2 p.Q141K, which affects the response to rosuvastatin (Tomlinson et al., 2010), the most cost-effective option for dyslipidemia treatment in Mexico (Briseño and Mino-León, 2010).
Although we were able to identify missense variants in pharmacogenes with possible differential actionable effects in the Mexican population, one limitation of this study is that the MxGDAR/Encodat database does not have information about drug responses or adverse reactions, which limits the conclusions that can be drawn about the specific effects on the Mexican population. Another limitation is our use of a genotyping platform with reduced coverage and a fixed number of variants. Microarray genotyping technology eliminates the possibility of finding new variants in these pharmacogenes, but this could be accomplished with other techniques, such as next-generation technologies (NGS). An analysis of the Mexican population recently performed utilizing NGS found novel variation for some pharmacogenes between NA and MM populations (Gonzalez-Covarrubias et al., 2019). However, that analysis considered the MM population as a single group: an analysis of admixture patterns within the MM population, as performed in the present study, could be applied using NGS to refine the analysis of the Mexican population, and genomic guided some public health decisions in Mexico.
A description of the pharmacogenetic variants that can be actionable in this representative subsample of the Mexican population will help to understand and reduce treatment disparities in persons of admixed genetic background with differing pharmacogenetic variants. Such studies can serve as guides to precision medicine in the Mexican population and in other populations with mixed genetic backgrounds.
The datasets generated for this study can be found in the European Variation Archive (EVA).
The studies involving human participants were reviewed and approved by Instituto Nacional de Psiquiatría Ramón de la Fuente Muñiz and Instituto Nacional de Medicina Genómica. Written informed consent to participate in this study was provided by the participants’ legal guardian/next of kin.
JM-M, AG-M, JV, and HN developed the analyses and wrote the first version of the manuscript. JM-M, RM and JV performed bioinformatics and statistical analyses. JV, RM, CF, MB, ER, and MM-M contributed to data collection. BC, AA, JM-M, and AG-M contributed to the genetic experiments. HN, MM-M and JV conceived, designed, and coordinated the project.
This study received funding from the Instituto Nacional de Medicina Genómica (Grant No. 23/2015/I), and from the Comisión Nacional de Ciencia y Tecnología (CONACyT) 2016 Fund for the Development of Scientific Projects to Address National Problems (Grant No. PN22296). The development of the surveys was funded by the Comisión Nacional Contra las Adicciones (CONADIC).
The authors declare that the research was conducted in the absence of any commercial or financial relationships that could be construed as a potential conflict of interest.
We wish to thank the work committee and the field coordination team of the Encodat 2016; the microarray unit of the Instituto Nacional de Medicina Genómica, and Rául Mojica Espinoza and the expression and microarray unit of INMEGEN, for technical assistance with microarray processing; and Dr. Manuel Mondragón y Kalb and Dr. Nora Frías from the Comision Nacional de las Adicciones (CONADIC), for their invaluable support in the development of the whole project. We also wish to thank Katherine Alejandra Rojas Espinoza, Cecilia Britanny Pereo Sanchez, Angel Polanco, Alejandro Garrido, and Alejandro Cortez Meda at INMEGEN for assistance with the DNA extraction.
The Supplementary Material for this article can be found online at: https://www.frontiersin.org/articles/10.3389/fphar.2020.00324/full#supplementary-material
Supplementary File 1 | Variants filtered-out during quality controls steps.
Abdul Jalil, N. J., Bannur, Z., Derahman, A., Maskon, O., Darinah, N., Hamidi, H., et al. (2015). The implication of the polymorphisms of COX-1, UGT1A6, and CYP2C9 among cardiovascular disease patients treated with aspirin. J. Pharm. Pharm. Sci. 18, 474–483. doi: 10.18433/j3fc7f
Barbarino, J. M., Whirl-Carrillo, M., Altman, R. B., Klein, T. E. (2018). PharmGKB: A worldwide resource for pharmacogenomic information. Wiley Interdiscip. Rev. Syst. Biol. Med. 10 (4), e1417. doi: 10.1002/wsbm.1417
Ben Mahmoud, L., Ghozzi, H., Kamoun, A., Hakim, A., Hachicha, H., Hammami, S., et al. (2012). Polymorphism of the N-acetyltransferase 2 gene as a susceptibility risk factor for antituberculosis drug-induced hepatotoxicity in Tunisian patients with tuberculosis. Pathol. Biol. 60, 324–330. doi: 10.1016/j.patbio.2011.07.001
Bhatt, D. K., Mehrotra, A., Gaedigk, A., Chapa, R., Basit, A., Zhang, H., et al. (2019). Age- and Genotype-Dependent Variability in the Protein Abundance and Activity of Six Major Uridine Diphosphate-Glucuronosyltransferases in Human Liver. Clin. Pharmacol. Ther. 105, 131–141. doi: 10.1002/cpt.1109
Blanco, J. G., Leisenring, W. M., Gonzalez-Covarrubias, V. M., Kawashima, T. I., Davies, S. M., Relling, M. V., et al. (2008). Genetic polymorphisms in the carbonyl reductase 3 gene CBR3 and the NAD(P)H:quinone oxidoreductase 1 gene NQ01 in patients who developed anthracycline-related congestive heart failure after childhood cancer. Cancer 112, 2789–2795. doi: 10.1002/cncr.23534
Bonifaz-Peña, V., Contreras, A. V., Struchiner, C. J., Roela, R. A., Furuya-Mazzotti, T. K., Chammas, R., et al. (2014). Exploring the distribution of genetic markers of pharmacogenomics relevance in Brazilian and Mexican populations. PloS One 9 (11), e112640. doi: 10.1371/journal.pone.0112640
Borges, G., Orozco, R., Villatoro, J., Medina-Mora, M. E., Fleiz, C., Díaz-Salazar, J. (2018). Suicide ideation and behavior in Mexico: Encodat 2016. Salud Publica Mex. 61, 6. doi: 10.21149/9351
Briseño, G. G., Mino-León, D. (2010). Cost-effectiveness of rosuvastatin versus ezetimibe/simvastatin in managing dyslipidemic patients in Mexico. Curr. Med. Res. Opin. 26, 1075–1081. doi: 10.1185/03007991003694498
Bryc, K., Durand, E. Y., Macpherson, J. M., Reich, D., Mountain, J. L. (2015). The genetic ancestry of african americans, latinos, and european Americans across the United States. Am. J. Hum. Genet. 96, 37–53. doi: 10.1016/j.ajhg.2014.11.010
Caronia, D., Patiño-García, A., Milne, R. L., Zalacain-Díez, M., Pita, G., Alonso, M. R., et al. (2009). Common variations in ERCC2 are associated with response to cisplatin chemotherapy and clinical outcome in osteosarcoma patients. Pharmacogenomics J. 9, 347–353. doi: 10.1038/tpj.2009.19
Cavalli-Sforza, L. L. (2005). The Human Genome Diversity Project: past, present and future. Nat. Rev. Genet. 6, 333–340. doi: 10.1038/nrg1596
Chambers, J. C., Zhang, W., Sehmi, J., Li, X., Wass, M. N., Van der Harst, P., et al. (2011). Genome-wide association study identifies loci influencing concentrations of liver enzymes in plasma. Nat. Genet. 43 (11), 1131–1138. doi: 10.1038/ng.970
Cuautle-Rodríguez, P., Llerena, A., Molina-Guarneros, J. (2014). Present status and perspective of pharmacogenetics in Mexico. Drug Metabol. Drug Interact. 29, 37–45. doi: 10.1515/dmdi-2013-0019
El-Derany, M. O., Hamdy, N. M., Al-Ansari, N. L., El-Mesallamy, H. O. (2016). Integrative role of vitamin D related and Interleukin-28B genes polymorphism in predicting treatment outcomes of Chronic Hepatitis C. BMC Gastroenterol. 16, 19. doi: 10.1186/s12876-016-0440-5
Fagerholm, R., Hofstetter, B., Tommiska, J., Aaltonen, K., Vrtel, R., Syrjäkoski, K., et al. (2008). NAD(P)H:quinone oxidoreductase 1 NQO1*2 genotype (P187S) is a strong prognostic and predictive factor in breast cancer. Nat. Genet. 40, 844–853. doi: 10.1038/ng.155
Favela-Mendoza, A. F., Martinez-Cortes, G., Hernandez-Zaragoza, M., Salazar-Flores, J., Muñoz-Valle, ,. J. F., Martinez-Sevilla, V. M., et al. (2015). Genetic variability of CYP2C19 in a Mexican population: contribution to the knowledge of the inheritance pattern of CYP2C19*17 to develop the ultrarapid metabolizer phenotype. J. Genet. 94, 3–7. doi: 10.1007/s12041-015-0477-1
García-Martín, E., Agúndez, J. A. G., Maestro, M. L., Suárez, A., Vidaurreta, M., Martínez, C., et al. (2013). Influence of Vitamin D-Related Gene Polymorphisms (CYP27B and VDR) on the Response to Interferon/Ribavirin Therapy in Chronic Hepatitis C. PloS One 8 (9), e74764. doi: 10.1371/journal.pone.0074764
Gast, A., Bermejo, J. L., Flohr, T., Stanulla, M., Burwinkel, B., Schrappe, M., et al. (2007). Folate metabolic gene polymorphisms and childhood acute lymphoblastic leukemia: A case-control study. Leukemia 21, 320–325. doi: 10.1038/sj.leu.2404474
Gelernter, J., Kranzler, H. R., Sherva, R., Almasy, L., Herman, A. I., Koesterer, R., et al. (2015). Genome-wide association study of nicotine dependence in American populations: identification of novel risk loci in both African-Americans and European-Americans. Biol. Psychiatry 77, 493–503. doi: 10.1016/j.biopsych.2014.08.025
Glynn, S. A., Boersma, B. J., Howe, T. M., Edvardsen, H., Geisler, S. B., Goodman, J. E., et al. (2009). A mitochondrial target sequence polymorphism in manganese superoxide dismutase predicts inferior survival in breast cancer patients treated with cyclophosphamide. Clin. Cancer Res. 15, 4165–4173. doi: 10.1158/1078-0432.CCR-09-0119
Goh, L. L., Lim, C. W., Sim, W. C., Toh, L. X., Leong, K. P. (2017). Analysis of genetic variation in CYP450 genes for clinical implementation. PloS One 12 (1), e0169233 doi: 10.1371/journal.pone.0169233
Gonzalez-Covarrubias, V., Kalabus, J. L., Blanco, J. G. (2008). Inhibition of polymorphic human carbonyl reductase 1 (CBR1) by the cardioprotectant flavonoid 7-monohydroxyethyl rutoside (monoHER). Pharm. Res. 25, 1730–1734. doi: 10.1007/s11095-008-9592-5
Gonzalez-Covarrubias, V., Martinez-Magana, J. J., Coronado-Sosa, R., Villegas-Torres, B., Genis-Mendoza, A. D., Canales-Herrerias, P., et al. (2016). Exploring Variation in Known Pharmacogenetic Variants and its Association with Drug Response in Different Mexican Populations. Pharm. Res. 33, 2644–2652. doi: 10.1007/s11095-016-1990-5
Gonzalez-Covarrubias, V., Urena-Carrion, J., Villegas-Torres, B., Cossío-Aranda, J. E., Trevethan-Cravioto, S., Izaguirre-Avila, R., et al. (2017). Pharmacogenetic Variation in Over 100 Genes in Patients Receiving Acenocumarol. Front. Pharmacol. 8, 863. doi: 10.3389/fphar.2017.00863
Gonzalez-Covarrubias, V., Morales-Franco, M., Cruz-Correa, O. F., Martínez-Hernández, A., García-Ortíz, H., Barajas-Olmos, F., et al. (2019). Variation in actionable pharmacogenetic markers in natives and mestizos from Mexico. Front. Pharmacol. 10, 1169. doi: 10.3389/fphar.2019.01169
Gudiseva, H. V., Hansen, M., Gutierrez, L., Collins, D. W., He, J., Verkuil, L. D., et al. (2016). Saliva DNA quality and genotyping efficiency in a predominantly elderly population. BMC Med. Genomics 9, 17. doi: 10.1186/s12920-016-0172-y
Gutierrez-Camino, A., Martin-Guerrero, I., Garcia-Orad, A. (2017). PNPLA3 rs738409 and Hepatotoxicity in Children With B-cell Acute Lymphoblastic Leukemia: A Validation Study in a Spanish Cohort. Clin. Pharmacol. Ther. 102, 906. doi: 10.1002/cpt.756
Hancock, D. B., Guo, Y., Reginsson, G. W., Gaddis, N. C., Lutz, S. M., Sherva, R., et al. (2017). Genome-wide association study across European and African American ancestries identifies a SNP in DNMT3B contributing to nicotine dependence. Mol. Psychiatry. 23 (9), 1911–1919. doi: 10.1038/mp.2017.193
He, X., Hesse, L. M., Hazarika, S., Masse, G., Harmatz, J. S., Greenblatt, D. J., et al. (2009). Evidence for oxazepam as an in vivo probe of UGT2B15: Oxazepam clearance is reduced by UGT2B15 D85Y polymorphism but unaffected by UGT2B17 deletion. Br. J. Clin. Pharmacol. 68, 721–730. doi: 10.1111/j.1365-2125.2009.03519.x
Huang, L., Tissing, W. J. E., de Jonge, R., van Zelst, B. D., Pieters, R. (2008). Polymorphisms in folate-related genes: Association with side effects of high-dose methotrexate in childhood acute lymphoblastic leukemia. Leukemia 22, 1798–1800. doi: 10.1038/leu.2008.66
Hung, C. C., Chang, W. L., Ho, J. L., Tai, J. J., Hsieh, T. J., Huang, H. C., et al. (2012). Association of polymorphisms in EPHX1, UGT2B7, ABCB1, ABCC2, SCN1A and SCN2A genes with carbamazepine therapy optimization. Pharmacogenomics 13, 159–169. doi: 10.2217/pgs.11.141
Javorsky, M., Klimcakova, L., Schroner, Z., Zidzik, J., Babjakova, E., Fabianova, M., et al. (2012). KCNJ11 gene E23K variant and therapeutic response to sulfonylureas. Eur. J. Intern. Med. 23, 245–249. doi: 10.1016/j.ejim.2011.10.018
Johnson, E. O., Chen, L. S., Breslau, N., Hatsukami, D., Robbins, T., Saccone, N. L., et al. (2010). Peer smoking and the nicotinic receptor genes: An examination of genetic and environmental risks for nicotine dependence. Addiction 105, 2014–2022. doi: 10.1111/j.1360-0443.2010.03074.x
Kamiya, A., Kubo, K., Tomoda, T., Takaki, M., Youn, R., Ozeki, Y., et al. (2005). A schizophrenia-associated mutation of DISC1 perturbs cerebral cortex development. Nat. Cell Biol. 7, 1167–1178. doi: 10.1038/ncb1328
Kim, S. H., Kim, S. H., Bahn, J. W., Kim, Y. K., Chang, Y. S., Shin, E. S., et al. (2009). Genetic polymorphisms of drug-metabolizing enzymes and anti-TB drug-induced hepatitis. Pharmacogenomics 10, 1767–1779. doi: 10.2217/pgs.09.100
Kobayashi, Y., Yang, S., Nykamp, K., Garcia, J., Lincoln, S. E., Topper, S. E. (2017). Pathogenic variant burden in the ExAC database: an empirical approach to evaluating population data for clinical variant interpretation. Genome Med. 9, 13. doi: 10.1186/s13073-017-0403-7
López-Rodríguez, R., Ferreiro-Iglesias, A., Lima, A., Bernardes, M., Pawlik, A., Paradowska-Gorycka, A., et al. (2018). Replication study of polymorphisms associated with response to methotrexate in patients with rheumatoid arthritis. Sci. Rep. 8, 7342. doi: 10.1038/s41598-018-25634-y
McLaren, W., Gil, L., Hunt, S. E., Riat, H. S., Ritchie, G. R. S., Thormann, A., et al. (2016). The Ensembl Variant Effect Predictor. Genome Biol. 17, 122. doi: 10.1186/s13059-016-0974-4
Medellin-Garibay, S. E., Hernández-Villa, N., Correa-González, L. C., Morales-Barragán, M. N., Valero-Rivera, K. P., Reséndiz-Galván, J. E., et al. (2019). Population pharmacokinetics of methotrexate in Mexican pediatric patients with acute lymphoblastic leukemia. Cancer Chemother. Pharmacol. 85, 21–31. doi: 10.1007/s00280-019-03977-1
Mizzi, C., Dalabira, E., Kumuthini, J., Dzimiri, N., Balogh, I., Başak, N., et al. (2016). A European spectrum of pharmacogenomic biomarkers: Implications for clinical pharmacogenomics. PloS One 11 (9), e0162866. doi: 10.1371/journal.pone.0162866
Moreno-Estrada, A., Gignoux, C. R., Fernandez-Lopez, J. C., Zakharia, F., Sikora, M., Contreras, A. V., et al. (2014). Human genetics. The genetics of Mexico recapitulates Native American substructure and affects biomedical traits. Science 344, 1280–1285. doi: 10.1126/science.1251688
Oliveira, A. L., Rodrigues, F. F. O., Santos, R. E., Aoki, T., Rocha, M. N., Longui, C. A., et al. (2010). GSTT1, GSTM1, and GSTP1 polymorphisms and chemotherapy response in locally advanced breast cancer. Genet. Mol. Res. 9, 1045–1053. doi: 10.4238/vol9-2gmr726
Pagano, A., Gubner, N., Le, T., Guydish, J. (2018). Cigarette smoking and quit attempts among Latinos in substance use disorder treatment. Am. J. Drug Alcohol Abuse 44, 660–667. doi: 10.1080/00952990.2017.1417417
Pato, M. T., Sobell, J. L., Medeiros, H., Abbott, C., Sklar, B. M., Buckley, P. F., et al. (2013). The genomic psychiatry cohort: partners in discovery. Am. J. Med. Genet. B. Neuropsychiatr. Genet. 162B, 306–312. doi: 10.1002/ajmg.b.32160
Patterson, N., Price, A. L., Reich, D. (2006). Population structure and eigenanalysis. PloS Genet. 2, 2074–2093. doi: 10.1371/journal.pgen.0020190
Price, A. L., Patterson, N. J., Plenge, R. M., Weinblatt, M. E., Shadick, N. A., Reich, D. (2006). Principal components analysis corrects for stratification in genome-wide association studies. Nat. Genet. 38, 904–909. doi: 10.1038/ng1847
Puranik, Y. G., Birnbaum, A. K., Marino, S. E., Ahmed, G., Cloyd, J. C., Remmel, R. P., et al. (2013). Association of carbamazepine major metabolism and transport pathway gene polymorphisms and pharmacokinetics in patients with epilepsy. Pharmacogenomics 14, 35–45. doi: 10.2217/pgs.12.180
Purcell, S., Neale, B., Todd-Brown, K., Thomas, L., Ferreira, M. A. R., Bender, D., et al. (2007). PLINK: a tool set for whole-genome association and population-based linkage analyses. Am. J. Hum. Genet. 81, 559–575. doi: 10.1086/519795
Relling, M. V., Evans, W. E. (2015). Pharmacogenomics in the clinic. Nature 526, 343–350. doi: 10.1038/nature15817
Reséndiz-Escobar, E., Bustos Gamiño, M. N., Mujica Salazar, R., Soto Hernández, I. S., Cañas Martínez, V., Fleiz Bautista, C., et al. (2018). National trends in alcohol consumption in Mexico: results of the National Survey on Drug, Alcohol and Tobacco Consumption 2016-2017. Salud Ment. 41, 7–15. doi: 10.17711/SM.0185-3325.2018.003
Romero-Hidalgo, S., Ochoa-Leyva, A., Garciarrubio, A., Acuna-Alonzo, V., Antunez-Arguelles, E., Balcazar-Quintero, M., et al. (2017). Demographic history and biologically relevant genetic variation of Native Mexicans inferred from whole-genome sequencing. Nat. Commun. 8, 1005. doi: 10.1038/s41467-017-01194-z
Sakano, S., Hinoda, Y., Sasaki, M., Wada, T., Matsumoto, H., Eguchi, S., et al. (2010). Nucleotide excision repair gene polymorphisms may predict acute toxicity in patients treated with chemoradiotherapy for bladder cancer. Pharmacogenomics 11, 1377–1387. doi: 10.2217/pgs.10.106
Scheet, P., Stephens, M. (2006). A fast and flexible statistical model for large-scale population genotype data: Applications to inferring missing genotypes and haplotypic phase. Am. J. Hum. Genet. 78, 629–644. doi: 10.1086/502802
Scrucca, L., Fop, M., Murphy, T. B., Raftery, A. E. (2016). Mclust 5: Clustering, classification and density estimation using Gaussian finite mixture models. R. J. 8, 289–317. doi: 10.32614/rj-2016-021
Silva-Zolezzi, I., Hidalgo-Miranda, A., Estrada-Gil, J., Fernandez-Lopez, J. C., Uribe-Figueroa, L., Contreras, A., et al. (2009). Analysis of genomic diversity in Mexican Mestizo populations to develop genomic medicine in Mexico. Proc. Natl. Acad. Sci. U. S. A. 106, 8611–8616. doi: 10.1073/pnas.0903045106
Thorn, C. F., Klein, T. E., Altman, R. B. (2013). PharmGKB: The pharmacogenomics knowledge base. Methods Mol. Biol. 1015, 311–320. doi: 10.1007/978-1-62703-435-7_20
Tomlinson, B., Hu, M., Lee, V. W. Y., Lui, S. S. H., Chu, T. T. W., Poon, E. W. M., et al. (2010). ABCG2 Polymorphism Is Associated With the Low-Density Lipoprotein Cholesterol Response to Rosuvastatin. Clin. Pharmacol. Ther. 87, 558–562. doi: 10.1038/clpt.2009.232
Tsunoda, A., Nakao, K., Watanabe, M., Matsui, N., Ooyama, A., Kusano, M. (2011). Associations of various gene polymorphisms with toxicity in colorectal cancer patients receiving oral uracil and tegafur plus leucovorin: A prospective study. Ann. Oncol. 22, 355–361. doi: 10.1093/annonc/mdq358
Tzvetkov, M. V., Behrens, G., O’Brien, V. P., Hohloch, K., Brockmöller, J., Benhr, P. (2011). Pharmacogenetic analyses of cisplatin-induced nephrotoxicity indicate a renoprotective effect of ERCC1 polymorphisms. Pharmacogenomics 12, 1417–1427. doi: 10.2217/pgs.11.93
Van Der Wouden, C. H., Swen, J. J., Samwald, M., Mitropoulou, C., Schwab, M., Guchelaar, H. J. (2016). A brighter future for the implementation of pharmacogenomic testing. Eur. J. Hum. Genet. 24, 1658–1660. doi: 10.1038/ejhg.2016.116
Verbelen, M., Weale, M. E., Lewis, C. M. (2017). Cost-effectiveness of pharmacogenetic-guided treatment: Are we there yet? Pharmacogenomics J. 17, 395–402. doi: 10.1038/tpj.2017.21
Villarreal, A. (2014). Ethnic identification and its consequences for measuring inequality in Mexico. Am. Sociol. Rev. 79, 775–806. doi: 10.1177/0003122414541960
Villatoro-Velázquez, J. A., Resendiz-Escobar, E., Mujica-Salazar, A., Bretón-Cirett, M., Cañas-Martínez, V., Soto-Hernández, I. (2017). “Encuesta Nacional de Consumo de Drogas, Alcohol y Tabaco 2016-2017: Reporte de Drogas”, Instituto Nacional de Psiquiatría Ramón de la Fuente Muñiz, Instituto Nacional de Salud Pública, Comisión Nacional Contra las Adicciones and Secretaría de Salud (Ciudad de México, México: INPRFM).
Wang, S., Ray, N., Rojas, W., Parra, M. V., Bedoya, G., Gallo, C., et al. (2008). Geographic patterns of genome admixture in latin American mestizos. PloS Genet. 4 (3), e1000037. doi: 10.1371/journal.pgen.1000037
Wright, G. E. B., Carleton, B., Hayden, M. R., Ross, C. J. D. (2018). The global spectrum of protein-coding pharmacogenomic diversity. Pharmacogenomics J. 18, 187–195. doi: 10.1038/tpj.2016.77
Yang, S. K., Hong, M., Baek, J., Choi, H., Zhao, W., Jung, Y., et al. (2014). A common missense variant in NUDT15 confers susceptibility to thiopurine-induced leukopenia. Nat. Genet. 46, 1017–1020. doi: 10.1038/ng.3060
Yin, J. Y., Huang, Q., Zhao, Y. C., Zhou, H. H., Liu, Z. Q. (2012). Meta-analysis on pharmacogenetics of platinum-based chemotherapy in non small cell lung cancer (NSCLC) patients. PloS One 7 (6), e38150. doi: 10.1371/journal.pone.0038150
Zhang, F., Finkelstein, J. (2019). Inconsistency in race and ethnic classification in pharmacogenetics studies and its potential clinical implications. Pharmgenomics. Pers. Med. 12, 107–123. doi: 10.2147/PGPM.S207449
Keywords: Mexican population-based sample, MxGDAR/Encodat, pharmacogenetics, genomics, admixture
Citation: Martínez-Magaña JJ, Genis-Mendoza AD, Villatoro Velázquez JA, Camarena B, Martín del Campo Sanchez R, Fleiz Bautista C, Bustos Gamiño M, Reséndiz E, Aguilar A, Medina-Mora ME and Nicolini H (2020) The Identification of Admixture Patterns Could Refine Pharmacogenetic Counseling: Analysis of a Population-Based Sample in Mexico. Front. Pharmacol. 11:324. doi: 10.3389/fphar.2020.00324
Received: 16 December 2019; Accepted: 05 March 2020;
Published: 22 April 2020.
Edited by:
Luis Abel Quiñones, University of Chile, ChileReviewed by:
Evangelia Eirini Tsermpini, University of Patras, GreeceCopyright © 2020 Martínez-Magaña, Genis-Mendoza, Villatoro Velázquez, Camarena, Martín del Campo Sanchez, Fleiz Bautista, Bustos Gamiño, Reséndiz, Aguilar, Medina-Mora and Nicolini. This is an open-access article distributed under the terms of the Creative Commons Attribution License (CC BY). The use, distribution or reproduction in other forums is permitted, provided the original author(s) and the copyright owner(s) are credited and that the original publication in this journal is cited, in accordance with accepted academic practice. No use, distribution or reproduction is permitted which does not comply with these terms.
*Correspondence: María Elena Medina-Mora, bWV0bW1vcmFAZ21haWwuY29t; Humberto Nicolini, aG5pY29saW5pQGlubWVnZW4uZ29iLm14
Disclaimer: All claims expressed in this article are solely those of the authors and do not necessarily represent those of their affiliated organizations, or those of the publisher, the editors and the reviewers. Any product that may be evaluated in this article or claim that may be made by its manufacturer is not guaranteed or endorsed by the publisher.
Research integrity at Frontiers
Learn more about the work of our research integrity team to safeguard the quality of each article we publish.