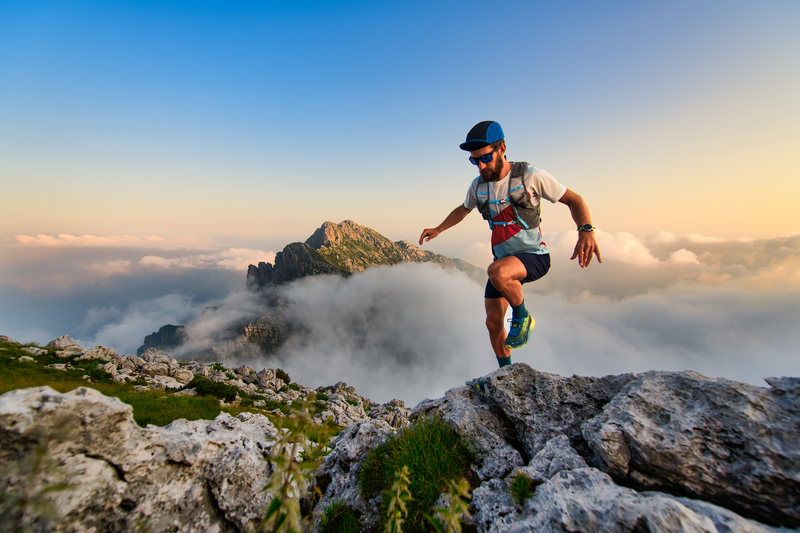
95% of researchers rate our articles as excellent or good
Learn more about the work of our research integrity team to safeguard the quality of each article we publish.
Find out more
ORIGINAL RESEARCH article
Front. Pharmacol. , 03 October 2019
Sec. Ethnopharmacology
Volume 10 - 2019 | https://doi.org/10.3389/fphar.2019.01132
This article is part of the Research Topic Network Pharmacology and Traditional Medicine View all 31 articles
The ancient tradition of taking parts of a plant or preparing plant extracts for treating certain discomforts and maladies has long been lacking a scientific rationale to support its preparation and still widespread use in several parts of the world. In an attempt to address this challenge, we collected and integrated data connecting metabolites, plants, diseases, and proteins. A mechanistic hypothesis is generated when a metabolite is known to be present in a given plant, that plant is known to be used to treat a certain disease, that disease is known to be linked to the function of a given protein, and that protein is finally known or predicted to interact with the original metabolite. The construction of plant–protein networks from mutually connected metabolites and diseases facilitated the identification of plausible mechanisms of action for plants being used to treat analgesia, hypercholesterolemia, diarrhea, catarrh, and cough. Additional concrete examples using both experimentally known and computationally predicted, and subsequently experimentally confirmed, metabolite–protein interactions to close the connection circle between metabolites, plants, diseases, and proteins offered further proof of concept for the validity and scope of the approach to generate mode of action hypotheses for some of the therapeutic uses of remedial herbs.
Plant leaves, roots, barks, and extracts have been used since the dawn of human history to treat various discomforts and maladies. The healing properties of remedial herbs were most likely identified through a long and serendipitous learning process that once acquired was carefully passed through generations. Still today, traditional medicines represent a well-established therapeutic alternative to synthetic drugs in vast parts of the world (Tao et al., 2014). However, there is still a profound lack of understanding about the specific chemical ingredient(s) and the exact mechanism(s) of action by which medicinal plants exert their therapeutic effect.
In recent years, global efforts to generate, collect, store, and make publicly available data connecting plants with their endogenous metabolites (phytoconstituents), interacting proteins, and disease indications have set the ground to develop novel systems approaches to unveiling the mode of action of remedial herbs (Liu et al., 2013; Lagunin et al., 2014; Chen et al., 2017). This is schematically illustrated in Figure 1. A number of publicly available well annotated databases on medicinal plants in use in different regions of the planet exist already (Chen, 2011; James et al., 2013; Ntie-Kang et al., 2013; Tota et al., 2013; Pathania et al., 2015; Mohanraj et al., 2018). Once data connecting the different aspects of ethnopharmacological relevance are known, the circle is closed and mechanistic hypotheses emerge naturally. The problem arises when gaps of data exist and the circles cannot be closed. In this respect, most current ethnomedicinal studies still focus on which parts of the plant are used to treat common ailments (Chassagne et al., 2016). Initiatives to identify and isolate some of the chemical structures present in those parts of therapeutic interest are expensive and inefficient. This notwithstanding, at least 50,000 endogenous plant metabolites have been already identified (Hounsome et al., 2008).
Figure 1 Scheme showing the data sources and the process of closing the gap between the therapeutic use of plants and the protein targets predicted for the endogenous plant metabolites.
However, in vitro affinity data between plant metabolites and human proteins are scarce to find in public repositories (Bolton et al., 2008; Gaulton et al., 2012). Therefore, more efforts are needed in this direction to close the gap between therapeutic use and mode of action in remedial herbs (represented as a dotted line in Figure 1). One option is to process large libraries of isolated small molecules from plants through in vitro high-throughput screening assays to identify affinities for therapeutically relevant proteins. This is a highly tedious and expensive endeavor if one wants to be comprehensive. Alternatively, modern state-of-the-art computational methods to predict the affinity of small molecules across thousands of proteins can be used to prioritize any further in vitro testing of selected small molecules on particular proteins (Vidal et al., 2011; Garcia-Serna et al., 2015). Applications on predicting the targets of natural medicines are increasingly being reported (Keum et al., 2016; Fang et al., 2017; Sawada et al., 2018; Yi et al., 2018).
The last step involves connecting those confirmed interacting proteins with the actual disease for which the plant is prescribed. This task is now facilitated by the recent construction of databases connecting human genes with diseases (Piñero et al., 2017). The aim of this work is to collect and integrate all pieces of data and processes that allow for automatically generating mechanistic hypotheses for the known therapeutic uses of plants.
Among the 372 medicinal plants present in our integrated database, Sambucus nigra (black elder) is the plant associated with the highest number of therapeutic uses (31). It is recommended for bronchitis, migraine, diarrhea, nausea, hyperuricemia, and influenza, to name just a few. Genus Sambucus belongs to the Caprifoliaceae family of flowering plants, whose leaves, flowers, and berries are traditionally used worldwide for a wide variety of medicinal applications (Dulf et al., 2013; Mahmoudi et al., 2014). Following Sambucus nigra in the list of plants with widest therapeutic use are Allium sativum (24), Rosmarinus officinalis (22), Mentha spicata (21), Urtica dioica (21), Salvia officinalis (21), and Thymus vulgaris (21), all of them found easily in many parts of the world and used as food and/or spice.
If we focus on cardiovascular diseases, a total of 171 plants were found to be associated with 46 different therapeutic uses. For illustrative purposes, the network of plants linked to cardiovascular diseases is shown in Figure 2. Among those, Ginkgo biloba is the plant with the most cardiovascular uses (with 14), followed by Camellia sinensis (with 8) and Allium cepa, Crataegus monogyna, Olea europea, Urtica doica, and Vitis vinifera (with 7). Among diseases, hypertension, hypercholesterolemia, hyperglycemia, and haemorrhoids are clearly the cardiovascular aspects being most addressed by remedial herbs.
Figure 2 Network of remedial herbs (gray circles) linked to therapeutic uses in cardiovascular diseases (white circles).
Ginkgo biloba and Camellia sinensis are indigenous plants from Asia (Cybulska-Heinrich et al., 2012; Moore et al., 2009). The extracts of the leaves and nuts from Ginkgo biloba have been used for hundreds of years to treat a wide variety of disorders, such as asthma, vertigo, tinnitus, as well as general circulatory problems (Cybulska-Heinrich et al., 2012). Camellia sinensis is a plant from which green tea can be produced. This beverage has a long traditional use as social drink but also as medicine in the treatment and prevention of disorders, dysfunctions, or diseases in humans and other animals (Batista et al., 2009; Moore et al., 2009). Aesculus hippocastanum (horse chestnut) is native to the countries of the Balkan Peninsula, but it is cultivated worldwide for its beauty. Historically, seed extracts from this plant have been used as a treatment for many ailments (Anonymous, 2009). Crataegus monogyna (hawthorn) is known as a traditional medicinal plant in many countries, growing in shrub communities and decidious thin forests (Öztürk and Tunçel, 2011). Vitis vinifera (grapevine) is an indigenous plant from southern and Western Asia, but it is cultivated today in all temperature regions of the world (Nassiri-Asl and Hosseinzadeh, 2009). Finally, Allium cepa (onion) is one of the most important vegetables worldwide and is extensively cultivated. It is an herbaceous bulbous plant that has a long tradition of being beneficial against inflammation, general cardiovascular diseases, and cancer (Slimestad et al., 2007).
Regarding knowledge on the chemical composition of plants, Camellia sinensis (green tea) is the plant with the highest number of chemical structures identified (710), followed by Zea mays (677) and Panax ginseng (601). Other chemically well characterized plants are Citrus sinensis (orange tree), Apium graveolens (celery), and Daucus carota (carrot), with 589, 533, and 507 known molecules, respectively. In contrast, many plants in the database have only one or very few endogenous metabolites identified, such as Rhamnus alaternus (Mediterranean blackthorn), Lonicera etrusca (honeysuckle), or Hernaria glabra (herniaria).
A detailed analysis of all the links between plants, metabolites, targets, and diseases in our integrated database (Figure 1) identified a total of 31,808 mechanistic hypotheses. At this stage, a mechanistic hypothesis is generated if a given plant known to have some therapeutic use contains at least one endogenous metabolite that is either known or predicted to interact with a human protein associated with its original therapeutic use. It ought to be stressed here that the concentration of any plant metabolite in a herbal preparation is very low and that, by any means, the results presented below imply that the metabolite assigned to the mechanistic hypothesis is the sole responsible of the therapeutic action of the plant but it will somehow contribute it. In this respect, a total of 893 mechanistic hypotheses for its different therapeutic uses could be generated for Glycine max (soybean). Among the plants with the highest number of mechanistic hypotheses generated, we found Ginkgo biloba (793), Camellia sinensis (781), Citrus limon (578), and Vitis vinifera (563). Out of the total number of 31,808 mechanistic hypotheses generated, 14,308 involved known interactions between endogenous metabolites and protein targets, whereas the remaining 17,500 hypotheses emerged from predicted interactions (see Materials and Methods).
Among the molecules involved in the mechanistic hypotheses generated with known metabolite–protein interactions, we identified some well-known single active principles, such as atropine, morphine, and digitoxin, as well as a mixture of active principles, such as the one composed of quercetin, luteolin, and apigenin (Figure 3).
Figure 3 Scheme showing some of the closed circles confirmed retrospectively for some of the plants being used in cardiovascular diseases.
Atropine is found mainly in Atropa belladonna and Datura stramonium (Kurzbaum et al., 2001; Caksen et al., 2003), both used commonly for their analgesic action (Duttaroy et al., 2002; Overington et al., 2006). This molecule is known to be active against the muscarinic acetylcholine receptor M4, a therapeutic target associated with some analgesics. Therefore, we have all links described in Figure 1 confirmed and thus forming a mechanistic hypothesis for the analgesic action of these plants (Soni et al., 2012; Owais et al., 2014).
Another widely recognized molecule for its analgesic activity is morphine. It is found in Papaver somniferum (opium poppy), and it was the first active alkaloid extracted from this plant (Jurna, 2003). Opium has been used in traditional medicinal as sedative and analgesic (Calixto et al., 2001). According to all links established in our database, morphine would be directly identified as a candidate to contribute to the analgesic action of opium through its interaction with µ-type opioid receptor (Choi et al., 2006; Yamada et al., 2006), a receptor well known for its association with analgesia (Inturrisi, 2002).
Digitoxin is a glycoside with known activity against the sodium/potassium-transporting ATPase subunit α-1, a protein associated with heart failure (Müller-Ehmsen et al., 2002; Hauck et al., 2009). Digitoxin is found in Digitalis purpurea (Chen et al., 2001), a plant used in traditional medicine for treating precisely this particular disease. Digitoxin has not only been proven to interact with the sodium/potassium-transporting ATPase subunit α-1, but it has also been shown to be indeed effective in heart failure (Belz et al., 2001).
Finally, we selected an example in which three compounds, namely, quercetin, luteolin, and apigenin, all confirmed endogenous metabolites of Achillea millefolium (yarrow), a plant used traditionally for treating depression, are known to have biologically relevant affinities for monoamine oxidase A (Lemmens-Gruber et al., 2006; Han et al., 2007; Benetis et al., 2008; Bandaruk et al., 2014), which, in turn, is one of the target proteins for depression (Thase et al., 1995).
A more systematic analysis of all the mechanistic hypotheses that could be derived directly from known data and associations revealed that, among all disease categories, the circulatory, respiratory, and musculoskeletal systems collectively represented over 47% of all mechanistic hypotheses generated. The plant–protein networks derived for some specific diseases within these categories are shown in Figure 4.
Figure 4 Network of remedial herbs (gray circles) linked to proteins (white circles) associated with various maladies, namely, analgesia, hypercholesterolemia, diarrhea, catarrh, and cough.
In the analgesia network (Figure 4, top), one can observe that plants have multiple connections with a diverse range of proteins. This reflects the fact that some of the endogenous metabolites found in those plants have biologically relevant affinities for many of those proteins. Among them, aldose reductase (Young et al., 1983), muscarinic acetylcholine receptors (Duttaroy et al., 2002), and opioid receptors (Inturrisi, 2002) are the ones being most targeted by metabolites from these plants. Altogether, the formation of this complex network is indicative of a variety of plausible mechanisms of action relevant to analgesia, although one cannot exclude the possibility that the proteins involved in this analgesia network are closely related by cross-pharmacology, that is, they interact with similar ligands (Keiser et al., 2007; Briansó et al., 2011).
In contrast, the catarrh and cough networks (Figure 4, bottom) show that plants indicated for these therapeutic uses have endogenous metabolites targeting very much the same proteins. For catarrh, all plants contain some chemical entity that is active on the dopamine D1B, D2, and D3 receptors, all of them well known to be associated with respiratory diseases (Birrell et al., 2002). For cough, all plants contain at least one chemical entity with affinity for the µ and δ opioid receptors (Kotzer et al., 2000).
In between these two limit situations, the plant–protein networks obtained for hypercholesterolemia and diarrhea (Figure 4, middle) are consistent with different plants linked to these therapeutic uses acting through a small number of different mechanistic hypotheses. In this respect, most plants used for treating hypercholesterolemia seem to contain some chemical ingredient biologically active on the peroxisome proliferator-activated receptor α (56) and the hydroxycarboxylic receptor 2 (Karpe and Frayn, 2004). However, other plants may be exerting their therapeutic effect through interactions with some enzymes also associated with hypercholesterolemia, such as fatty acid synthase (Marseille-Tremblay et al., 2007) and squalene monooxygenase (Belter et al., 2011). Likewise, plants used for treating diarrhea contain chemical entities that have affinities for the µ and κ opioid receptors (Callahan, 2002), the 5-hydroxytryptamine 3A receptor channel (Sikander et al., 2009), and/or the calcium-activated potassium channel subunit α-1 (Deng et al., 2015). Even though many plants are targeting all of them, others seem to target only one or two.
It is important to highlight at this stage that the use of known data only is prone to the effects of completeness, and thus, several links may actually be missing in the networks discussed. In fact, looking at the distribution of the affinity values for those known interactions, we observe that in many cases these interacting chemicals are found also in plants that are not used for treating the disease associated with the interacting protein. Some of the reasons why these plants have not been used for these illnesses could be, for example, that the compound concentration is not enough in the plant or the plant really has this therapeutic action but it is simply not used for it. The same plant may have different uses in different parts of the world. Last but not least, it could well be that the action of some of these compounds requires the presence of some bioenhancer (Dudhatra et al., 2012), the therapeutic action being ultimately the result of multiple compounds acting synergistically. Overall, from the initial number of 372 plants associated with at least one therapeutic use, only 193 contain known data for all necessary links to derive a mechanistic hypothesis. Accordingly, the following section illustrates the use of high-confidence predictions as a means to enlarge the coverage of plants for which mechanistic hypotheses can be derived.
Before embarking into the analysis of some of the mechanistic hypotheses emerging from predicted interactions, we validated the expected accuracy of those predictions for which known data was available. Overall, a good correlation was found between known and predicted affinity values for the same molecule–protein interactions. The median of the difference in affinities was 0.332, with 25% and 75% quartiles being at −0.1 and 0.7 with respect to the median, respectively, with a standard deviation of 0.794. Then, for those predicted interactions only, we focused on those providing a balance between potency of the predicted affinity and novelty of the prediction, as regarded by the similarity to the closest molecule for which the affinity for the same protein is known already. Among those, we prioritised the confirmation of the proposed mechanistic hypotheses for two single compounds, namely, rybosylzeatin and isorhamnetin, and one compound mixture, composed of cyanidin, delphinidin, and malvidin. The results are compiled in Figure 5.
Figure 5 Scheme showing some of the closed circles confirmed prospectively for some of the plants being used in cardiovascular diseases.
Ribosylzeatin is an endogenous metabolite present in Ginkgo biloba (gingko), Glycine max (soybean), and Vitis vinifera (grapevine). This small molecule was predicted to have low micromolar affinity for the adenosine A1 and A3 receptors and in vitro testing performed subsequently confirmed 57% and 65% binding, respectively, at 10mM concentration. Accordingly, a mechanistic hypothesis could be derived suggesting that the interaction of ribosylzeatin with the adenosine A1 and A3 receptors may be contributing to the beneficial effects of those plants in the treatment of a number of cardiovascular diseases where these receptors are known to play a role, namely, cardiac dysrhythmias, supraventricular tachycardia, acute ischaemic heart disease, and mycocardial ischemia (Kiesman et al., 2009; Fishman et al., 2012). In this respect, soybean is known as an important source of proteins in diet, widely used as herbal medicine for the treatment of several cardiovascular diseases. Also, the cardioprotector properties of grapevine have been exploited in folk medicine since ancient times. In particular, the therapeutic action of grapevine against ventricular tachycardia was recently demonstrated in rats (Zhao et al., 2010). However, in this study, the authors used a proanthocyanidin grape seed extract. On the basis of the hypothesis generated here, we would suggest that ribosylzeatin is one of the active ingredients in grapevine participating in this therapeutic effect in synergy with other proanthocyanidins.
Isorhamnetin is a phytochemical present in multiple plants used for the treatment of hypertension. A low micromolar affinity between isorhamnetin and the dopamine D4 receptor was predicted. Upon in vitro testing, the experimental value obtained was only 28% binding. Despite this rather low affinity value, we could suggest that this compound may well be contributing to some extent to the effect on hypertension of the plants in which it is present. In fact, a similar chemical present in most of those plants listed and suggested to be partly responsible for their therapeutic action on hypertension is quercetin, reported to have also a low experimental affinity value of 5 µM against the same dopamine D4 receptor.
Finally, very much along the same lines reported above for the hypotheses generated from known metabolite–protein interactions, we were also keen on having a prospective mixture example. Accordingly, we predicted activity of cyanidin, delphinidin, and malvidin, all present in Vaccinium myrtillus (bilberry), on the arachidonate 5-lipoxygenase (ALOX5). In vitro testing of its mixture confirmed a 41% inhibition. This result provides a mechanistic hypothesis for the therapeutic use of bilberry for atherosclerosis and ischemic heart disease. It has been already suggested that quercetin is partially responsible for the therapeutic action of this plant due to its affinity for the ALOX5. We could add now delphinidin, cyanidin, and malvidin to the list of potential chemical effectors of this plant. Indeed, bilberry fruit contains high concentration of several anthocyanidins (Cassinese et al., 2007; Chu et al., 2011). In fact, other anthocyanidins present in bilberry, such as peonidin and petunidin, were also predicted to be active against this protein. So all these compounds may actually contribute synergistically to the therapeutic effect attributed to bilberry for the treatment of atherosclerosis and ischemic heart disease.
An effort to integrate data linking metabolites, plants, diseases, and proteins has been shown to be useful to generate mechanistic hypotheses for some of the therapeutic uses of remedial herbs. In this respect, the use of predicted interactions largely increases our ability to generate mechanistic hypotheses for plants for which known data is scarce. This notwithstanding, one ought to admit that many computationally derived hypotheses may either be false positives or not truly contribute to the therapeutic effect exhibited by the medicinal plant. Unfortunately, it is impossible to pursue experimental confirmation of all hypotheses generated and offer general statistics of this limitation. Nonetheless, the examples presented offer clear potential for the use of this type of systems approaches to contribute to finding a scientific rationale for traditional medicines. There is much more to learn about nature and its use for therapeutic purposes, and more research in this direction is certainly necessary.
Linking plants to diseases. A very first version of the database was created containing the therapeutic use of plants in traditional Catalan medicine (Gausachs, 2008). Plants were stored using their scientific name in Latin, whereas therapeutic uses were mapped to their corresponding disease identifier in ICD-10 (International Classification of Diseases Version 10). This initial database was complemented with additional therapeutic uses found for those plants in other public sources (Raja et al., 1997; Rigat et al., 2007; James et al., 2013). Data from the different sources was integrated using Latin names for plants and ICD-10 identifiers for diseases from 18 categories. In total, 372 medicinal plants associated with 187 therapeutic uses were collected at this stage (Supplementary Material).
Linking plants to metabolites. The endogenous metabolites identified at present for every single plant in the database were extracted from three different sources, namely, Dr Duke Phytochemical and Ethnobotanical Database (U.S. Department of Agriculture, Agricultural Research Service., 1992-2016), the KNApSAcK database (Nakamura et al., 2014), and Gausachs’ work on Catalan remedial herbs (Gausachs, 2008). Among those, only KNApSacK contains chemical structures linked to chemical names. Structures for the chemical names available only in the other two sources were extracted from PubChem (Bolton et al., 2008). The final set of chemical structures from all sources was unified and stored using InChI Keys. In the end, a total of 7,443 unique chemical structures present in 322 of those 372 medicinal plants could be gathered and added to the database (Supplementary Material).
Linking proteins to diseases. Next, a list of both known and explored human proteins associated with diseases was extracted from the Therapeutic Target Database (Zhu et al., 2012). These data was complemented with curated protein−disease links, with focus on cardiovascular diseases, available in the literature (Cases and Mestres, 2009). The final list of proteins was stored and unified using their UniProt identifiers (The UniProt Consortium, 2015). A final number of 724 unique proteins known to be relevant for 166 out of the initial 187 therapeutic uses were ultimately entered into the database (Supplementary Material).
Linking metabolites to proteins. Finally, affinity data (pKi, pKd, pIC50, pEC50) between chemical structures and proteins was extracted from various public sources (Roth et al., 2004; Bolton et al., 2008; Gaulton et al., 2012; Gilson et al., 2016; Southan et al., 2016). Up to 3,171 known interactions between 705 phytoconstituents and 228 proteins were identified and collected into the database at this stage. In addition, since affinity data are well recognised to be suffering from completeness issues (Mestres et al., 2008; Cases and Mestres, 2009), known interactions between molecules and proteins were complemented with high-confidence predictions obtained using ligand-based computational models implemented in the CT-link software (Vidal et al., 2011; Garcia-Serna et al., 2015). Accordingly, 16,897 additional interactions predicted between 2,796 molecules, present in 297 plants, and 313 proteins were generated. However, the vast majority of the interactions predicted were assigned relatively low confidence scores (CScore). If only high confidence predictions (CScore ≥ 0.7) are considered, a total of 1,555 predicted interactions remain. In the end, affinity data for a total of 4,726 interactions between 1,157 endogenous metabolites and 320 therapeutically relevant proteins were assembled and stored in the database (Supplementary Material).
Predicting metabolite–protein interactions. All metabolite two-dimensional structures were processed with the CT-link software to obtain predicted affinities for a list of over 2,000 protein targets (Vidal et al., 2011; Garcia-Serna et al., 2015). Predictions are based on six independent ligand-based approaches that include similarity-based methods, pharmacophore clusters, quantitative structure–activity relationships, machine learning techniques, and cross-pharmacology relationships (Vidal et al., 2011). Ligand-based target models were constructed from small molecules for which in vitro affinity data was publicly available (Bolton et al., 2008; Gaulton et al., 2012). Each prediction is assigned a confidence score (CScore) that depends on the number and type of methods as well as on the affinity range of the prediction (see Supplementary Material). In the last decade, predictions from CT-link have been validated both retrospectively (Vidal and Mestres, 2010; Flachner et al., 2012; Spitzmüller and Mestres, 2013) and prospectively (Areias et al., 2010; Mestres et al., 2011; Antolin et al., 2012; Montolio et al., 2012; Antolin and Mestres, 2015; van Voorhuis et al., 2016; Ellis et al., 2019) in a wide range of applications and therapeutic areas.
Experimental in vitro assays. For the prospective validation, two molecules and one herbal extract were selected for testing with in vitro assays at Cerep (CEREP Inc). Ribosylzeatin was tested in binding assays to confirm the predicted interactions with adenosine A1 and A3 receptors. Cellular assays were used to confirm the predicted interactions between isorhamnetin and the dopamine D4 receptor, as well as between a compound mixture (containing cyanidin, delphinidin, and malvidin) and 5-lipoxygenase.
For the binding assay, ribosylzeatin was tested twice at a test concentration of 10 µM. The reference agonist ligands used to calculate the compound activity were CPA for the adenosine A1 receptor and IB-MECA for the adenosine A3 receptor, which have IC50 values of 0.75 nM and 0.31 nM, respectively. The adenosine A1 receptor assay was performed in the presence of 1 nM of 3H.CCPA. After 60 min of incubation with shaking, bound radioactivity was separated from free by vacuum filtration and determined by scintillation counting. A similar procedure was followed for the adenosine A3 receptor assay. In this case, it was performed in the presence of 0.15 nM of 125I.AB-MECA. It was incubated with shaking during 120 min. After that, bound radioactivity was filtered and measured with scintillation counting. For these binding assays the results are expressed as a percent of measured specific binding relative to control specific binding.
For the dopamine D4 receptor assay, isorhamnetin was tested at a concentration of 10 µM. The reference agonist ligand was dopamine, with an EC50 value of 28 nM. D4.4 was incubated for 10 min at 37ºC and, after that, cAMP was detected and measured with HTRF. Results are expressed as a percentage of measured response relative to control response.
Finally, for the testing of the compound mixture of cyanidin, delphinidin, and malvidin in the 5-lipoxygenase enzyme assay, the reference compound used was NDGA, which has an IC50 of 910 nM. 5-Lipoxygenase was incubated 20 min with shaking and 25 µM arachidonic acid as substrate. Thereafter, rhodamine 123 was measured using fluorimetry. Results are expressed as a percentage of measured specific binding relative to control specific binding.
Compounds showing an inhibition or stimulation higher than 50% were considered to be active for the proteins tested, whereas interactions showing activity values between 25% and 50% were considered to be indicative of at least weak to moderate effects.
All datasets generated for this study are included in the manuscript/Supplementary Files.
JM conceived and designed the study. JO acquired the data for the analysis. JO and JM analyzed and interpreted the data, discussed the results, and wrote the manuscript.
This research was supported by the Spanish Ministerio de Ciencia Innovación y Universidades (project SAF2017-83614-R).
The authors declare that the research was conducted in the absence of any commercial or financial relationships that could be construed as a potential conflict of interest.
The Supplementary Material for this article can be found online at: https://www.frontiersin.org/articles/10.3389/fphar.2019.01132/full#supplementary-material
Supplementary Table 1 | Collected data connecting diseases and plants, plants and metabolites, metabolites and proteins, and proteins and diseases. Also provided are the different subsets used to construct the plant-disease and plant-protein networks of Figures 2 and 4, respectively.
Antolin, A. A., Mestres, J. (2015). Distant polypharmacology among MLP chemical probes. ACS Chem. Biol. 10 (2), 395–400. doi: 10.1021/cb500393m
Antolin, A. A., Jalencas, X., Yélamos, J., Mestres, J. (2012). Identification of pim kinases as novel targets for PJ34 with confounding effects in PARP biology. ACS Chem. Biol. 7 (12), 1962–1967. doi: 10.1021/cb300317y
Areias, F. M., Brea, J., Gregori-Puigjané, E., Zaki, M. E. A., Carvalho, M. A., Domínguez, E., et al. (2010). In silico directed chemical probing of the adenosine receptor family. Bioorg. Med. Chem. 18 (9), 3043–3052. doi: 10.1016/j.bmc.2010.03.048
Bandaruk, Y., Mukai, R., Terao, J. (2014). Cellular uptake of quercetin and luteolin and their effects on monoamine oxidase-A in human neuroblastoma SH-SY5Y cells. Toxicol. Rep. 1, 639–649. doi: 10.1016/j.toxrep.2014.08.016
Batista, G. de A. P., da Cunha, C. L. P., Scartezini, M., von der Heyde, R., Bitencourt, M. G., et al. (2009). Prospective double-blind crossover study of Camellia sinensis (green tea) in dyslipidemias. Arq. Bras. Cardiol. 93 (2), 128–134. doi: 10.1590/S0066-782X2009000800010
Belter, A., Skupinska, M., Giel-Pietraszuk, M., Grabarkiewicz, T., Rychlewski, L., Barciszewski, J. (2011). Squalene monooxygenase – a target for hypercholesterolemic therapy. Biol. Chem. 392 (12), 1053–1075. doi: 10.1515/BC.2011.195
Belz, G. G., Breithaupt-Grogler, K., Osowski, U. (2001). Treatment of congestive heart failure – current status of use of digitoxin. Eur. J. Clin. Invest. 31 Suppl 2, 10–17. doi: 10.1046/j.1365-2362.2001.0310s2010.x
Benetis, R., Radusiene, J., Janulis, V. (2008). Variability of phenolic compounds in flowers of Achillea millefolium wild populations in Lithuania. Med. Kaunas Lith. 44 (10), 775–781. doi: 10.3390/medicina44100097
Birrell, M. A., Crispino, N., Hele, D. J., Patel, H. J., Yacoub, M. H., Barnes, P. J., et al. (2002). Effect of dopamine receptor agonists on sensory nerve activity: possible therapeutic targets for the treatment of asthma and COPD. Br. J. Pharmacol. 136 (4), 620–628. doi: 10.1038/sj.bjp.0704758
Bolton, E. E., Wang, Y., Thiessen, P. A., Bryant, S. H. (2008). PubChem: integrated platform of small molecules and biological activities. Annual Rep. Comput. Chem. 4, 217–241. doi: 10.1016/S1574-1400(08)00012-1
Bonet, M. A., Parada, M., Selga, A., Vallès, J. (1999). Studies on pharmaceutical ethnobotany in the regions of l’Alt Empordà and Les Guilleries (Catalonia, Iberian Peninsula). J. Ethnopharmacol. 68 (1–3), 145–168. doi: 10.1016/S0378-8741(99)00083-5
Briansó, F., Carrascosa, M. C., Oprea, T. I., Mestres, J. (2011). Cross-pharmacology analysis of G protein-coupled receptors. Curr. Top. Med. Chem. 11 (15), 1956–1963. doi: 10.2174/156802611796391285
Caksen, H., Odabaş, D., Akbayram, S., Cesur, Y., Arslan, S., Uner, A., et al. (2003). Deadly nightshade (Atropa belladonna) intoxication: an analysis of 49 children. Hum. Exp. Toxicol. 22 (12), 665–668. doi: 10.1191/0960327103ht404oa
Calixto, J. B., Scheidt, C., Otuki, M., Santos, A. R. (2001). Biological activity of plant extracts. Expert Opin. Emerg. Drugs 6 (2), 261–279. doi: 10.1517/14728214.6.2.261
Callahan, M. J. (2002). Irritable bowel syndrome neuropharmacology. A review of approved and investigational compounds. J. Clin. Gastroenterol. 35 (1 Suppl), 58–67. doi: 10.1097/00004836-200207001-00011
Cases, M., Mestres, J. (2009). A chemogenomic approach to drug discovery: focus on cardiovascular diseases. Drug Discov. Today 14 (9–10), 479–485. doi: 10.1016/j.drudis.2009.02.010
Cassinese, C., de Combarieu, E., Falzoni, M., Fuzzati, N., Pace, R., Sardone, N. (2007). New liquid chromatography method with ultraviolet detection for analysis of anthocyanins and anthocyanidins in Vaccinium myrtillus fruit dry extracts and commercial preparations. J. AOAC Int. 90 (4), 911–919.
CEREP Inc. http://www.cerep.fr/.
Chassagne, F., Hul, S., Deharo, E., Bourdy, G. (2016). Natural remedies used by Bunong people in Mondulkiri province (Northeast Cambodia) with special reference to the treatment of 11 most common ailments. J. Ethnopharmacol. 191, 41–70. doi: 10.1016/j.jep.2016.06.003
Chen, C. Y.-C. (2011). TCM Database@Taiwan: the world’s largest traditional Chinese medicine database for drug screening in silico. PLoS ONE 6 (1), e15939. doi: 10.1371/journal.pone.0015939
Chen, J. J., Wang, P. S., Chien, E. J., Wang, S. W. (2001). Direct inhibitory effect of digitalis on progesterone release from rat granulosa cells. Br. J. Pharmacol. 132 (8), 1761–1768. doi: 10.1038/sj.bjp.0703974
Chen, Y., de Bruyn Kops, C., Kirchmair, J. (2017). Data resources for the computer-guided discovery of bioactive natural products. J. Chem. Inf. Model. 57 (9), 2099–2111. doi: 10.1021/acs.jcim.7b00341
Choi, H. S., Kim, C. S., Hwang, C. K., Song, K. Y., Wang, W., Qiu, Y., et al. (2006). The opioid ligand binding of human mu-opioid receptor is modulated by novel splice variants of the receptor. Biochem. Biophys. Res. Commun. 343 (4), 1132–1140. doi: 10.1016/j.bbrc.2006.03.084
Chu, W., Cheung, S. C. M., Lau, R. A. W., Benzie, I. F. F. (2011). “Bilberry (Vaccinium myrtillus L.),” in Herbal Medicine: Biomolecular and Clinical Aspects (Boca Raton (FL): CRC Press/Taylor & Francis). chapter 4. doi: 10.1201/b10787-5
Cybulska-Heinrich, A. K., Mozaffarieh, M., Flammer, J. (2012). Ginkgo bilobal: an adjuvant therapy for progressive normal and high tension glaucoma. Mol. Vis. 18, 390–402.
Deng, Y., Han, X., Tang, S., Xiao, W., Tan, Z., Zhou, C., et al. (2015). Magnolol and honokiol regulate the calcium-activated potassium channels signaling pathway in Enterotoxigenic Escherichia coli-induced diarrhea mice. Eur. J. Pharmacol. 755, 66–73. doi: 10.1016/j.ejphar.2015.03.002
Dudhatra, G. B., Mody, S. K., Awale, M. M., Patel, H. B., Modi, C. M., Kumar, A., et al. (2012). A comprehensive review on pharmacotherapeutics of herbal bioenhancers. Sci. World J. 637953. doi: 10.1100/2012/637953
Dulf, F. V., Oroian, I., Vodnar, D. C., Socaciu, C., Pintea, A. (2013). Lipid clases and fatty acid regiodistribution in triacylglycerols of seed oils of two Sambucus species (S. nigra L. and S. ebulus L.). Molecules 18 (10), 11768–11782. doi: 10.3390/molecules181011768
Duttaroy, A., Gomeza, J., Gan, J.-W., Siddiqui, N., Basile, A. S., Harman, W. D., et al. (2002). Evaluation of muscarinic agonist-induced analgesia in muscarinic acetylcholine receptor knockout mice. Mol. Pharmacol. 62 (5), 1084–1093. doi: 10.1124/mol.62.5.1084
Ellis, C. R., Racz, R., Kruhlak, N. L., Kim, M. T., Hawkins, E. G., Strauss, D. G., et al. (2019). Assessing the structural and pharmacological similarity of newly identified drugs of abuse to controlled substances using public health assessment via structural evaluation. Clin. Pharmacol. Ther. 106 (1), 116–122. doi: 10.1002/cpt.1418
Fang, J., Wu, Z., Cai, C., Wang, Q., Tang, Y., Cheng, F. (2017). Quantitative and systems pharmacology. 1. In silico prediction of drug-target interactions of natural products enables new targeted cancer therapy. J. Chem. Inf. Model. 57 (11), 2657–2671. doi: 10.1021/acs.jcim.7b00216
Fishman, P., Bar-Yehuda, S., Liang, B. T., Jacobson, K. A. (2012). Pharmacological and therapeutic effects of A3 adenosine receptor antagonists. Drug Discov. Today 17 (7–8), 359–366. doi: 10.1016/j.drudis.2011.10.007
Flachner, B., Lörincz, Z., Carotti, A., Nicolotti, O., Kuchipudi, P., Remez, N., et al. (2012). A chemocentric approach to the identification of cancer targets. PLoS ONE 7 (4), e35582. doi: 10.1371/journal.pone.0035582
Garcia-Serna, R., Vidal, D., Remez, N., Mestres, J. (2015). Large-scale predictive drug safety: from structural alerts to biological mechanisms. Chem. Res. Toxicol. 28 (10), 1875–1887. doi: 10.1021/acs.chemrestox.5b00260
Gaulton, A., Bellis, L. J., Bento, A. P., Chambers, J., Davies, M., Hersey, A., et al. (2012). ChEMBL: a large-scale bioactivity database for drug discovery. Nucleic Acids Res. 40 (Database issue), D1100–D1107. doi: 10.1093/nar/gkr777
Gilson, M. K., Liu, T., Baitaluk, M., Nicola, G., Hwang, L., Chong, J. (2016). BindingDB in 2015: a public database for medicinal chemistry, computational chemistry and systems pharmacology. Nucleic Acids Res. 44 (D1), D1045–D1053. doi: 10.1093/nar/gkv1072
Han, X. H., Hong, S. S., Hwang, J. S., Lee, M. K., Hwang, B. Y., Ro, J. S. (2007). Monoamine oxidase inhibitory components from Cayratia japonica. Arch. Pharm. Res. 30 (1), 13–17. doi: 10.1007/BF02977772
Hauck, C., Potter, T., Bartz, M., Wittwer, T., Wahlers, T., Mehlhorn, U., et al. (2009). Isoform specificity of cardiac glycosides binding to human Na+,K+-ATPase alpha1beta1, alpha2beta1 and alpha3beta1. Eur. J. Pharmacol. 622 (1–3), 7–14. doi: 10.1016/j.ejphar.2009.08.039
Hounsome, N., Hounsome, B., Tomos, D., Edwards-Jones, G. (2008). Plant metabolites and nutritional quality of vegetables. J. Food Sci. 73 (4), 48–65. doi: 10.1111/j.1750-3841.2008.00716.x
Inturrisi, C. E. (2002). Clinical pharmacology of opioids for pain. Clin. J. Pain 18 (4 Suppl), S3–S13. doi: 10.1097/00002508-200207001-00002
James, P., Mathai, V. A., Shajikumar, S., Pereppadan, P. A., Sudha, P., Keshavachandran, R., et al. (2013). DIACAN: integrated database for antidiabetic and anticancer medicinal plants. Bioinformation 9 (18), 941–943. doi: 10.6026/97320630009941
Jurna, I. (2003). Sertürner und Morphin – eine historische Vignette. Schmerz 17, 280–283. doi: 10.1007/s00482-003-0220-3
Karpe, F., Frayn, K. N. (2004). The nicotinic acid receptor – a new mechanism for an old drug. Lancet 363 (9424), 1892–1894. doi: 10.1016/S0140-6736(04)16359-9
Keiser, M. J., Roth, B. L., Armbruster, B. N., Ernsberger, P., Irwin, J. J., Shoichet, B. K. (2007). Relating protein pharmacology by ligand chemistry. Nat. Biotechnol. 25 (2), 197–206. doi: 10.1038/nbt1284
Keum, J., Yoo, S., Lee, D., Nam, H. (2016). Prediction of compound-target interactions of natural products using large-scale drug and protein information. BMC Bioinformatics 17 (Suppl 6), 219. doi: 10.1186/s12859-016-1081-y
Kiesman, W. F., Elzein, E., Zablocki, J. (2009). A1 adenosine receptor antagonists, agonists, and allosteric enhancer. Handb. Exp. Pharmacol. 193, 25–58. doi: 10.1007/978-3-540-89615-9_2
Kotzer, C. J., Hay, D. W., Dondio, G., Giardina, G., Petrillo, P., Underwood, D. C. (2000). The antitussive activity of delta-opioid receptor stimulation in guinea pigs. J. Pharmacol. Exp. Ther. 292 (2), 803–809.
Kurzbaum, A., Simsolo, C., Kvasha, L., Blum, A. (2001). Toxic delirium due to Datura stramonium. Isr. Med. Assoc. J. 3 (7), 538–539.
Lagunin, A. A., Goel, R. K., Gawande, D. Y., Pahwa, P., Gloriozova, T. A., Dmitriev, A. V., et al. (2014). Chemo- and bioinformatics resources for in silico drug discovery from medicinal plants beyond their traditional use: a critical review. Nat. Prod. Rev. 31 (11), 1585–1611. doi: 10.1039/C4NP00068D
Lemmens-Gruber, R., Marchart, E., Rawnduzi, P., Engel, N., Benedek, B., Kopp, B. (2006). Investigation of the spasmolytic activity of the flavonoid fraction of Achillea millefolium s.l. on isolated guinea-pig ilea. Arzneimittelforschung 56 (8), 582–588. doi: 10.1055/s-0031-1296755
Liu, H., Wang, J., Zhou, W., Wang, Y., Yang, L. (2013). Systems approaches and polypharmacology for drug discovery from herbal medicines: an example using licorise. J. Ethnopharmacol. 146 (3), 773–793. doi: 10.1016/j.jep.2013.02.004
Mahmoudi, M., Ebrahimzadeh, M. A., Dooshan, A., Arimi, A., Ghasemi, N., Fathiazad, F. (2014). Antidepressant activities of Sambucus ebulus and Sambucus nigra. Eur. Rev. Med. Pharmacol. Sci. 18 (22), 3350–3353.
Marseille-Tremblay, C., Gravel, A., Lafond, J., Mounier, C. (2007). Effect of an enriched cholesterol diet during gestation on fatty acid synthase, HMG-CoA reductase and SREBP-1/2 expressions in rabbits. Life Sci. 81 (9), 772–778. doi: 10.1016/j.lfs.2007.07.016
Mestres, J., Gregori-Puigjané, E., Valverde, S., Solé, R. V. (2008). Data completeness – the Achilles heel of drug-target networks. Nat. Biotechnol. 26 (9), 983–984. doi: 10.1038/nbt0908-983
Mestres, J., Seifert, S. A., Oprea, T. I. (2011). Linking pharmacology to clinical reports: cyclobenzaprine and its possible association with serotonin syndrome. Clin. Pharmacol. Ther. 90 (5), 662–665. doi: 10.1038/clpt.2011.177
Mohanraj, K., Karthikeyan, B. S., Vivek-Ananth, R. P., Chand, R. P. B., Aparna, S. R., Mangalapandi, P., et al. (2018). IMPPAT: a curated database of Indian medicinal plants, phytochemistry and therapeutics. Sci. Rep. 8 (1), 4329. doi: 10.1038/s41598-018-22631-z
Montolio, M., Gregori-Puigjané, E., Pineda, D., Mestres, J., Navarro, P. (2012). Identification of small molecule inhibitors of amyloid b-induced neuronal apoptosis acting through the imidazoline I(2) receptor. J. Med. Chem. 55 (22), 9838–9846. doi: 10.1021/jm301055g
Moore, R. J., Jackson, K. G., Minihane, A. M. (2009). Green tea (Camellia sinensis) catechins and vascular function. Br. J. Nutr. 102 (12), 1790–1802. doi: 10.1017/S0007114509991218
Müller-Ehmsen, J., McDonough, A. A., Farley, R. A., Schwinger, R. H. G. (2002). Sodium pump isoform expression in heart failure: implication for treatment. Basic Res. Cardiol. 97 Suppl 1, 25–30. doi: 10.1007/s003950200025
Nakamura, Y., Afendi, F. M., Parvin, A. K., Ono, N., Tanaka, K., Hirai, A., et al. (2014). KNApSAcK metabolite activity database for retrieving the relationships between metabolites and biological activities. Plant Cell Physiol. 55 (1), e7. doi: 10.1093/pcp/pct176
Nassiri-Asl, M., Hosseinzadeh, H. (2009). Review of the pharmacological effects of Vitis vinifera (grape) and its bioactive compounds. Phytother. Res. 23 (9), 1197–1204. doi: 10.1002/ptr.2761
Ntie-Kang, F., Zofou, D., Babiaka, S. B., Meudom, R., Scharfe, M., Lifongo, L. L., et al. (2013). AfroDb: a select highly potent and diverse natural product library from African medicinal plants. PLoS ONE 8 (10), e78085. doi: 10.1371/journal.pone.0078085
Overington, J. P., Al-Lazikani, B., Hopkins, A. L. (2006). How many drug targets are there? Nat. Rev. Drug Discov. 5 (12), 993–996. doi: 10.1038/nrd2199
Owais, F., Anwar, S., Saeed, F., Muhammad, S., Ishtiaque, S., Mohiuddin, O. (2014). Analgesic, anti-inflammatory and neuropharmacological effects of Atropa belladonna. Pak. J. Pharm. Sci. 27 (6 Spec No.), 2183–2187.
Öztürk, N., Tunçel, M. (2011). Assessment of phenolic acid content and in vitro antiradical characteristics of hawthorn. J. Med. Food 14 (6), 664–669. doi: 10.1089/jmf.2010.0063
Pathania, S., Ramakrishnan, S. M., Bagler, G. (2015). Phytochemica: a platform to explore phytochemicals of medicinal plants. Database bav75. doi: 10.1093/database/bav075
Piñero, J., Bravo, A., Queralt-Rosinach, N., Gutiérrez-Sacristán, A., Deu-Pons, J., Centeno, E., et al. (2017). DisGeNET: a comprehensive platform integrating information on human disease-associated genes and variants. Nucleic Acids Res. 45 (D1), D833–D839. doi: 10.1093/nar/gkw943
Raja, D., Blanché, C., Vallès, J. (1997). Contribution to the knowledge of the pharmaceutical ethnobotany of La Segarra region (Catalonia, Iberian Peninsula). J. Ethnopharmacol. 57 (3), 149–160. doi: 10.1016/S0378-8741(97)00059-7
Rigat, M., Bonet, M. A., Garcia, S., Garnatje, T., Vallès, J. (2007). Studies on pharmaceutical ethnobotany in the high river Ter valley (Pyrenees, Catalonia, Iberian Peninsula). J. Ethnopharmacol. 113 (2), 267–277. doi: 10.1016/j.jep.2007.06.004
Rimando, A. M., Nagmani, R., Feller, D. R., Yokoyama, W. (2005). Pterostilbene, a new agonist for the peroxisome proliferator-activated receptor alpha-isoform, lowers plasma lipoproteins and cholesterol in hypercholesterolemic hamsters. J. Agric. Food Chem. 53 (9), 3403–3407. doi: 10.1021/jf0580364
Roth, B. L., Sheffler, D. J., Kroeze, W. K. (2004). Magic shotguns versus magic bullets: selectively non-selective drugs for mood disorders and schizophrenia. Nat. Rev. Drug Discov. 3 (4), 353–359. doi: 10.1038/nrd1346
Sawada, R., Iwata, M., Umezaki, M., Usui, Y., Kobayahi, T., Kubono, T., et al. (2018). KampoDB, database of predicted targets and functional annotations of natural medicines. Sci. Rep. 8 (1), 11216. doi: 10.1038/s41598-018-29516-1
Sikander, A., Rana, S. V., Prasad, K. K. (2009). Role of serotonin in gastrointestinal motility and irritable bowel syndrome. Clin. Chim. Acta 403 (1–2), 47–55. doi: 10.1016/j.cca.2009.01.028
Slimestad, R., Fossen, T., Vågen, I. M. (2007). Onions: a source of unique dietary flavonoids. J. Agric. Food Chem. 55 (25), 10067–10080. doi: 10.1021/jf0712503
Soni, P., Siddiqui, A. A., Dwivedi, J., Soni, V. (2012). Pharmacological properties of Datura stramonium L. as a potential medicinal tree: an overview. Asian Pac. J. Trop. Biomed. 2 (12), 1002–1008. doi: 10.1016/S2221-1691(13)60014-3
Southan, C., Sharman, J. L., Benson, H. E., Faccenda, E., Pawson, A. J., Alexander, S. P. H., et al. (2016). The IUPHAR/BPS Guide to PHARMACOLOGY in 2016: towards curated quantitative interactions between 1300 protein targets and 6000 ligands. Nucleic Acids Res. 44 (D1), D1054–D1068. doi: 10.1093/nar/gkv1037
Spitzmüller, A., Mestres, J. (2013). Prediction of the P. Falciparum target space relevant to malaria drug discovery. PLoS Comput. Biol. 9 (10), e1003257. doi: 10.1371/journal.pcbi.1003257
Tao, L., Zhu, F., Qin, C., Zhang, C., Xu, F., Tan, C. Y., et al. (2014). Nature’s contribution to today’s pharmacopeia. Nat. Biotechnol. 32 (10), 979–980. doi: 10.1038/nbt.3034
Thase, M. E., Trivedi, M. H., Rush, A. J. (1995). MAOIs in the contemporary treatment of depression. Neuropsychopharmacology 12 (3), 185–219. doi: 10.1016/0893-133X(94)00058-8
The UniProt Consortium (2015). UniProt: a hub for protein information. Nucleic Acids Res. 43 (Database issue), D204–D212. doi: 10.1093/nar/gku989
Tota, K., Rayabarapu, N., Moosa, S., Talla, V., Bhyravbhatla, B., Rao, S. (2013). InDiaMed: a comprehensive database of Indian medicinal plants for diabetes. Bioinformation 9 (7), 378–380. doi: 10.6026/97320630009378
U.S. Department of Agriculture, Agricultural Research Service. (1992). Dr. Duke’s Phytochemical and Ethnobotanical Databases [Internet version] Boca Raton, Fla: CRC Press, 183. doi: 10.15482/USDA.ADC/1239279
van Voorhuis, W. C., Adams, J. H., Adelfio, R., Ahyong, V., Akabas, M.H., Alano, P., et al. (2016). Open source drug discovery with the Malaria Box compound collection for neglected diseases and beyond. PLoS Pathogens 12 (7), e1005763. doi: 10.1371/journal.ppat.1005763
Vidal, D., Mestres, J. (2010). In silico receptorome screening of antipsychotic drugs. Mol. Inform. 29 (6–7), 543–551. doi: 10.1002/minf.201000055
Vidal, D., Garcia-Serna, R., Mestres, J. (2011). Ligand-based approaches to in silico pharmacology. Methods Mol. Biol. 672, 489–502. doi: 10.1007/978-1-60761-839-3_19
Yamada, H., Shimoyama, N., Sora, I., Uhl, G. R., Fukuda, Y., Moriya, H., et al. (2006). Morphine can produce analgesia via spinal kappa opioid receptors in the absence of mu opioid receptors. Brain Res. 1083 (1), 61–69. doi: 10.1016/j.brainres.2006.01.095
Yi, F., Li, L., Xu, L.-J., Meng, H., Dong, Y.-M., Liu, H.-B., et al. (2018). In silico approach in reveal traditional medicine plants pharmacological material basis. Chin. Med. 13, 33. doi: 10.1186/s13020-018-0190-0
Young, R. J., Ewing, D. J., Clarke, B. F. (1983). A controlled trial of sorbinil, an aldose reductase inhibitor, in chronic painful diabetic neuropathy. Diabetes 32 (10), 938–942. doi: 10.2337/diabetes.32.10.938
Zhao, J., Jiang, P., Zhang, W. (2010). Molecular networks for the study of TCM pharmacology. Brief. Bioinform. 11 (4), 417–430. doi: 10.1093/bib/bbp063
Keywords: ethnopharmacology, traditional medicine, network pharmacology, mechanism of action, phytochemicals, endogenous metabolites, plant metabolomics
Citation: Olivés J and Mestres J (2019) Closing the Gap Between Therapeutic Use and Mode of Action in Remedial Herbs. Front. Pharmacol. 10:1132. doi: 10.3389/fphar.2019.01132
Received: 01 March 2019; Accepted: 30 August 2019;
Published: 03 October 2019.
Edited by:
Yuanjia Hu, University of Macau, ChinaReviewed by:
Pinarosa Avato, University of Bari Aldo Moro, ItalyCopyright © 2019 Olivés and Mestres. This is an open-access article distributed under the terms of the Creative Commons Attribution License (CC BY). The use, distribution or reproduction in other forums is permitted, provided the original author(s) and the copyright owner(s) are credited and that the original publication in this journal is cited, in accordance with accepted academic practice. No use, distribution or reproduction is permitted which does not comply with these terms.
*Correspondence: Jordi Mestres, am1lc3RyZXNAaW1pbS5lcw==
Disclaimer: All claims expressed in this article are solely those of the authors and do not necessarily represent those of their affiliated organizations, or those of the publisher, the editors and the reviewers. Any product that may be evaluated in this article or claim that may be made by its manufacturer is not guaranteed or endorsed by the publisher.
Research integrity at Frontiers
Learn more about the work of our research integrity team to safeguard the quality of each article we publish.