- 1College of Medicine and Public Health, Flinders University, Adelaide, SA, Australia
- 2ADME Sciences, Medicine Design, Pfizer Inc, Groton, CT, United States
- 3Clinical Pharmacology, Global Product Development, Pfizer Inc, New York, NY, United States
Background: Drug probe phenotyping is used extensively in academic and industry research to evaluate cytochrome P450 (CYP) phenotype in order to account for sources of between- and within- subject variability in metabolic clearance. In terms of application, CYP3A is the most important drug metabolizing enzyme the most frequently studied. Currently, phenotyping studies for CYP3A involve the administration of midazolam and collection of timed blood samples up to 24-48 hours in order to determine an area under the plasma concentration time curve (AUC). The key challenge that limits the use of midazolam-based phenotyping for CYP3A in academic research settings and preclude the use of this approach in a clinical setting is the logistical burden of collecting frequent blood samples for up to 48 h post dose following the administration of a probe drug ± an interacting drug.
Aim: The current study sought to validate if a reduced sampling interval could be used to accurately define both between-subject variability in CYP3A phenotype and the magnitude of changes in CYP3A activity due to either induction or mechanism-based inhibition.
Methods: The area under the curve (AUC) for midazolam was assessed under baseline, induction (7 days rifampin, 300 mg daily) and, following a washout period of 4 days, mechanism based inhibition (3 days clarithromycin, 250 mg daily) conditions in a cohort of 30 health males. The capacity of normalized reduced sampling interval AUCs measured over 0 to 1, 0 to 2, 0 to 3, and 0 to 4 h to accurately define the AUC0-6 was evaluated with respect to precision (R2 for correlation), bias (slope of normalized correlation), agreement (Bland Altman analysis) and proportional bias (linear regression of Bland Altman parameters).
Results: Robust concordance was observed between the AUC calculated from PK collection intervals of 0 to 3 and 0 to 6 h in terms of both the measurement of between-subject variability in midazolam AUC and changes in midazolam AUC due to induction and mechanism-based inhibition of CYP3A4.
Conclusion: On this basis, it is proposed that a 3-h assessment of midazolam AUC (AUC0-3) represents a viable strategy to reduce the logistical burden associated with the assessment of CYP3A phenotype.
Introduction
In vivo drug probe phenotyping is the current gold standard approach to evaluate cytochrome P450 (CYP) phenotype in order to account for sources of between- and within- subject variability in metabolic clearance (Rowland et al., 2016). This approach, which is used extensively in academic and industry research, involves the administration of a drug that is a selective substrate for an enzyme of interest and subsequent collection of timed blood samples, typically over 24–48 h, in order to determine the area under the plasma drug concentration time curve (AUC) (Ryu et al., 2007). By assessing the probe drug’s AUC across different populations (e.g., racial/ethic groups, genotype) or before and after the administration of an interacting drug (e.g., potential enzyme inducer), it is possible to define the impact of an interacting drug or a population characteristic on the phenotype of the target CYP.
In terms of the application, CYP3A4 and 3A5 (collectively referred to as CYP3A) are the most clinically important drug metabolizing enzymes thus the most frequently investigated in phenotyping studies (Zanger and Schwab, 2013). By way of example, in drug development when a new chemical entity (NCE) is identified in pre-clinical testing as a potential ‘perpetrator’ of a metabolic drug-drug interaction (DDI) involving CYP3A, a phenotyping study is required to assess the change in AUC for a sensitive CYP3A substrate pre-/post-dosing of the NCE (Rowland et al., 2018; Kapetas et al., 2019). When evaluating CYP3A phenotype, midazolam is the most commonly used probe and is recommended as such in US Food and Drug Administration (FDA) guidance (US Food and Drug Administration, 2017). Midazolam is an intermediate hepatic clearance (CLH = 27 L/h) drug that is extensively metabolized by CYP3A4, CYP3A5, and CYP3A7 (Williams et al., 2002) making it an ideal probe to determine both hepatic and intestinal CYP3A phenotypes when administered orally (Thummel et al., 1994a; Thummel et al., 1994b; Thummel et al., 1996).
The key challenge that limits the use of phenotyping for CYP3A in academic research settings and preclude the use of this approach in a clinical setting is the logistical burden of collecting blood samples for up to 24–48 h post dose following the administration of a probe drug with or without an interacting drug (Rowland et al., 2017). In order to circumvent this limitation, several endogenous biomarker based strategies have been proposed to characterize CYP3A phenotype (Bjorkhem-Bergman et al., 2013; Mårde Arrhén et al., 2013; Rodrigues and Rowland, 2018; Rowland et al., 2019). While these strategies may ultimately replace the need to administer a drug probe, none have been adopted to date due to either inherent biological limitations (e.g., the complexity of steroid metabolism which involves multiple sequential, parallel or alternate metabolic pathways for the substrate (probe) synthesis, substrate metabolism and product metabolism) (Winters and Clark, 2003) or a current limited body of evidence (e.g., extracellular vesicle derived protein, ex vivo activity or mRNA biomarkers) (Rodrigues and Rowland, 2018). Similarly, several strategies including the administration of midazolam micro-dosing (Eap et al., 2004; Hohmann et al., 2015), evaluation of a plasma hydroxy-midazolam to midazolam metabolic ratios (Shih and Huang, 2002; Chaobal and Kharasch, 2005), and limited PK sampling strategies (Lee et al., 2006; Katzenmaier et al., 2010; Mueller and Drewelow, 2013; Tai et al., 2013; Masters et al., 2015; Yang et al., 2018) have been proposed, but have not been widely adopted. Of these strategies, to date only the use of a 75 µg oral micro-dose has been rigorously verified (Hohmann et al., 2015). The notable limitation with this micro-dose strategy that continues to limit its application is that it requires access to a sufficiently sensitive analytical platform capable of quantifying femtomolar midazolam concentrations in plasma (Burhenne et al., 2012; Hohmann et al., 2015). Likewise, the use of a single hydroxy-midazolam to midazolam concentration ratio collected at a specific time point as a surrogate for midazolam AUC has largely been discredited as a viable option given the poor concordance of this metabolic ratio with midazolam exposure (Lee et al., 2006).
Similarly, the performance of existing limited sampling protocols (Kim et al., 2002; Lee et al., 2006; Katzenmaier et al., 2010; Mueller and Drewelow, 2013; Tai et al., 2013; Masters et al., 2015; Yang et al., 2018) have only been verified with respect to the concordance of describing between-subject variability in CYP3A phenotype, in cases with sporadic sampling under baseline, inhibited or induced conditions. To date, no reduced sampling protocol has been verified with respect to performance in terms of defining changes in CYP3A phenotype due to either inhibition or induction of catalytic activity within a single cohort of individuals. Notably in this regard, the prior study of Katzenmaier et al. (2010) proposed a sampling interval (2–4 h) that fails to capture the midazolam absorption and distribution phases (hence cannot account for differences in Cmax). The importance of this omission being that the time course and magnitude of CYP3A induction and mechanism-based inhibition in the liver and intestine are not equivalent (Kapetas et al., 2019), meaning that changes in CYP3A phenotype described by midazolam AUC cannot be robustly defined unless the sampling protocol captures both Cmax and a sufficient window of the elimination phase. Similarly, in many cases the focus has been on minimizing the number of sample draws rather than the duration over which the samples are collected (Mueller and Drewelow, 2013; Tai et al., 2013), by way of example Mueller et al. (Mueller and Drewelow, 2013) demonstrated that midazolam AUC can be accurately defined using only 4 data-points, albeit over an 8-h interval. The current study sought to validate if a reduced sampling interval that retains rich sampling during the first hour (i.e. focus on shorter timeframe, not fewer samples), could be used to accurately define both between-subject variability in CYP3A phenotype as well as the magnitude of change in CYP3A activity due to either induction or mechanism-based inhibition.
Methods
Study Protocol
EPOK-15 is a single-center, open-label, single-sequence metabolic phenotyping study (van Dyk et al., 2018; Rowland et al., 2019). The study protocol was approved by the Southern Adelaide Clinical Human Research Ethics Committee (SAHREC 11.15), and written informed consent was obtained from each participant prior to study enrolment (Figure 1). The study was conducted according to the principles stated in the Declaration of Helsinki, was compliant with CPMP/ICH/135/95 GCP standards and is registered with the Australian New Zealand Clinical Trials Registry (ACTRN 12614001289606).
Midazolam pharmacokinetics following the administration of a 1 mg oral midazolam dose was assessed in a cohort of healthy males (n = 30; Table 1) at baseline (Day 1), following a 7 day course of rifampin (Induction phase; Day 8) and following a 3 day course of immediate release clarithromycin (Mechanism-based inhibition phase; Day 15) with a four day washout period between the induction and mechanism-based inhibition phases. Blood samples collected prior to dosing and at 0.25, 0.5, 0.75, 1, 2, 3, 4 and 6 h post-dosing were centrifuged and plasma were stored at -80°C until analysis for the determination of midazolam concentrations. Participants self-administered rifampin (300 mg daily PO) and clarithromycin (250 mg twice daily PO) from days 1–8 and days 12–14, respectively.
Sample Preparation and Analyses
The sample preparation and analysis for the determination of midazolam and 1-OH midazolam concentrations has been reported previously (van Dyk et al., 2018). Briefly, protein from 100 μl of plasma sample was precipitated using 300 μl of methanol containing 0.1% formic acid and 7.5 ng/ml d6-midazolam (assay internal standard). Samples were vortexed for 3 min, then centrifuged at 16,000 g for 5 min. Analytes in a 2.5 μl aliquot of the resulting supernatant were separated from the sample matrix by ultra-performance liquid chromatography (UPLC) performed on a Waters ACQUITY™ BEH C18 column (100 mm × 2.1 mm, 1.7 μm; Waters Corp., Milford, USA) using a Waters ACQUITY™ UPLC system. Column elutant was monitored by mass spectrometry, performed on a Waters Q-ToF Premier™ quadrupole, orthogonal acceleration time-of-flight tandem mass spectrometer operating in positive electron spray ionization (ESI+) mode. Selected ion data was extracted at the analyte [M+H]+ precursor m/z. Resulting pseudo-MRM spectra were analyzed using Waters TargetLynx™ software. Plasma analyte concentrations were determined by comparison of normalized peak areas in participant samples to those of external calibrators. As described previously, rifampin and clarithromycin plasma concentrations were also determined pre-midazolam dosing on day 8 and day 15, respectively (van Dyk et al., 2018).
Data Analysis
Non-compartmental methods were used to calculate the area under the midazolam plasma concentration time curve (AUC0-t) for PK sampling intervals of 0–1, 0–2, 0–3, 0–4, and 0–6 h post midazolam dosing on study day 1 (baseline), day 8 (following a 7-day course of rifampin), and day 15 (following a 3-day course of clarithromycin). Similarly, the pharmacokinetic parameters maximal concentration (Cmax), time to maximal concentration (Tmax), elimination half life (t1/2), elimination rate constant (k) and full area under the curve (AUC0-inf) were determined using non-compartmental methods (Microsoft Excel, PK Functions for Microsoft Excel, Department of Pharmacokinetics and Drug Metabolism, Irvine, CA, USA). AUC0-inf was calculated as AUC0-6 plus AUC6-inf, where AUC6-inf (the extrapolated AUC) was calculated by dividing the final measured concentration (Clast) the elimination rate constant (ke) determined over the period tmax to 6 h. In all cases the % extrapolated (AUC6_inf/AUC0-inf) was less than 20%.
The performance of test sampling intervals (AUC0-1, AUC0-2, AUC0-3 and AUC0-4) and the 1-OH midazolam to midazolam ratio measured at 3, 4, and 6 h were evaluated with respect to agreement of the normalized reduced sampling interval AUCs (or metabolite ratios) with the midazolam AUC0-6. Participant AUC data grouped by study phase was randomised 1:2 into training and validation data sets. The training dataset (n = 10 participants) was used to derive linear scaling factors (Equation 1) to normalize each of the AUC0-1, AUC0-2, AUC0-3 and AUC0-4 to the AUC0-6.
where “factor” is the scaling factor determined for each sampling interval (Table 4), and “t” is the final sampling time for each interval (i.e., 1, 2, 3, or 4 h).
Validation datasets (n = 60) for midazolam AUC0-1, AUC0-2, AUC0-3 and AUC0-4 were normalized to AUC0-6 using the scaling factor derived from the respective training sets. Bland-Altman analyses were performed to evaluate the correlation between AUC0-6 and the normalized reduced sampling interval AUCs calculated from reduced PK sampling intervals. Linear regression was performed to assess proportional bias based on the influence of mean AUC (normalized reduced sampling interval AUC and AUC0-6) on the difference in AUC (normalized reduced sampling interval AUC versus AUC0-6).
The performance of each reduced sampling interval AUC was also evaluated with respect to the concordance of the change (Δ) in midazolam exposures due to induction and mechanism-based inhibition of CYP3A. Using the same validation data set described for the assessment of the performance of the absolute AUC, midazolam AUC ratios were calculated for induction (AUCday8/AUCday1) and inhibition (AUCday15/AUCday1). Bland-Altman analyses were performed to evaluate the correlation between the respective AUC ratios for the reduced sampling interval AUCs and the corresponding AUC0-6 ratio. Linear regression was performed to assess proportional bias based on the influence of mean of the normalized reduced sampling interval AUCs and AUC0-6 parameters on the difference between the normalized reduced sampling interval AUCs and the AUC0-6 parameters. Bland-Altman plots were created by plotting the difference between the normalized reduced interval AUC and the AUC0-6 on the y-axis and the mean of the reduced interval AUC and the AUC0-6 on the y-axis.
Criteria for the acceptance of a reduced sampling interval AUC in the validation dataset were:
● An R2 for the correlation of the test parameter (AUC or AUC ratio) with the AUC0-6 parameter of >0.85 with and without applying a forced zero intercept.
● A slope for the correlation of the test parameter with the AUC0-6 parameter in the range 0.9 to 1.1 when applying a forced zero intercept.
● A lack of statistical significance for a one sample t-test of the difference in test and AUC0-6 parameters versus 0.
● A lack of statistical significance for the linear regression of the effect of the mean of normalized reduced sampling interval AUC and AUC0-6 on the difference between the normalized reduced sampling interval AUC and the AUC0-6. That is to exclude a proportional bias in the dataset, and to ensure that the magnitude and direction of difference between the normalized reduced sampling interval AUC and the AUC0-6 is independent of the magnitude of the mean of these AUCs.
Results
Midazolam Exposure
A summary of the geometric mean (95% CI) midazolam AUC baseline, post- induction and post- mechanism-based inhibition are reported in Table 2. Additional pharmacokinetic parameters defining midazolam exposure for each study phase are reported in Table 3. The distribution of midazolam concentration time profiles within the training and verification datasets for each of the three study phases (baseline, induction and mechanism based inhibition) is shown in Figure 2. There were no statistically significant differences in parameter estimates between the full, training and validation data sets. Dosing of rifampin (300 mg daily) for 7 days resulted in mean 69% and 68% reductions in midazolam AUC0-6 and Cmax, respectively. Dosing of clarithromycin (250 mg twice daily) for 3 days resulted in mean 49% and 40% increases in midazolam AUC0-6 and Cmax, respectively.
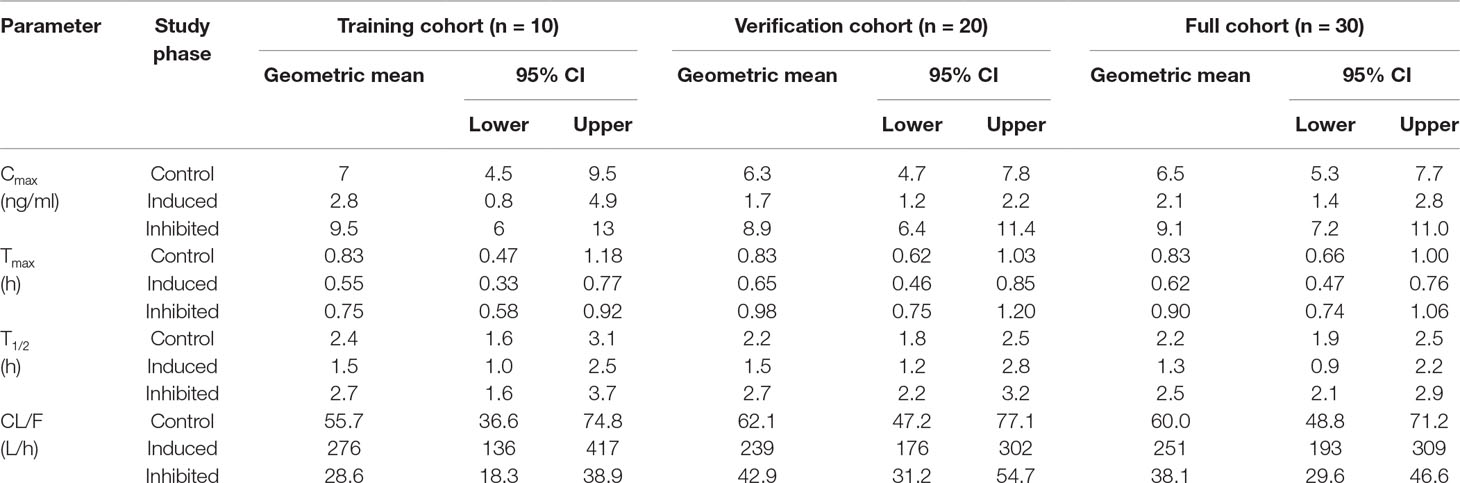
Table 3 Pharmacokinetic parameters defining midazolam concentration time profile in the training, verification and full cohorts.
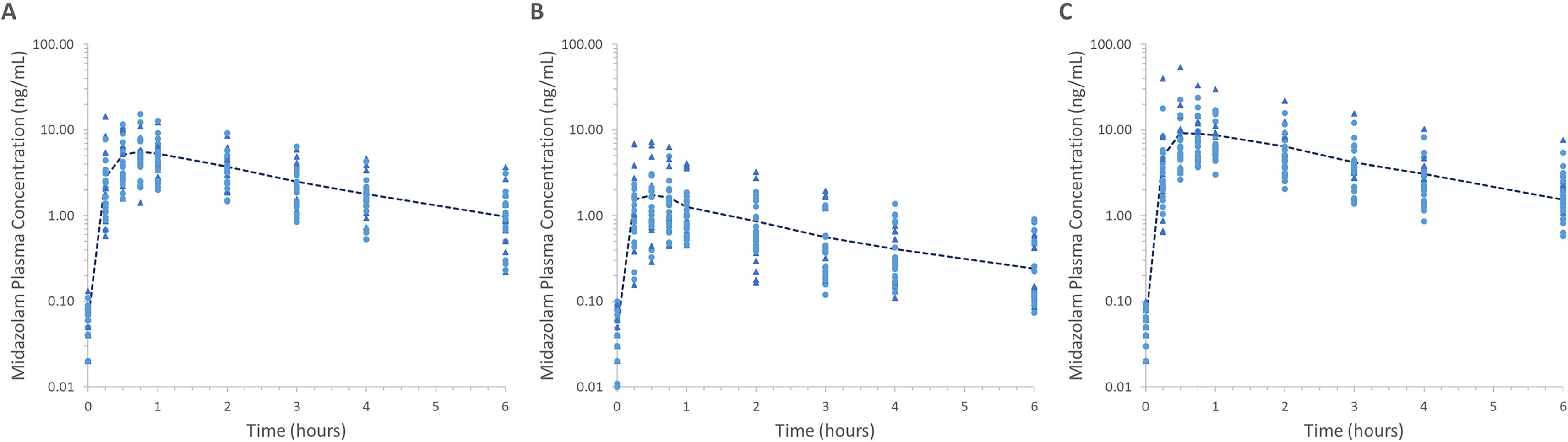
Figure 2 Midazolam plasma concentration time plots for baseline (A), induction (B) and Mechanism-based inhibition (C) study phases. Triangles represent training dataset, circles represent verification dataset, dashed line represents mean profile for the full dataset.
Capacity of Reduced Sampling Interval to Define Absolute Midazolam AUC
A training dataset (n = 10; 30 data points) was used to derive equations that normalized each reduced sampling interval AUC (AUC0-1, AUC0-2, AUC0-3, and AUC0-4) to midazolam AUC0-6 (Table 4). The concordance of each normalized reduced interval AUC with the AUC0-6 is visualized as correlation and Bland-Altman plots (Figure 3). With the exception of AUC0-1, the R2 for the correlation of each normalized reduced interval AUC with the AUC0-6 was >0.85. One-sample t-tests demonstrated that in all cases the difference between the normalized reduced interval AUC and AUC0-6 were not significantly different from 0 (p ≥ 0.76). Linear regression analysis demonstrated that there was no effect of the mean midazolam AUC on the difference in midazolam AUC, indicating a lack of proportional bias within each dataset (p ≥ 0.621). Based on fulfilment of the criteria for acceptance of precision and accuracy, the shortest acceptable sampling interval for assessment of absolute midazolam AUC was 0 to 3 hours. For absolute AUC, precision was also assessed at each data point in the validation dataset (n = 20; 60 data points) as the difference between the normalized reduced interval AUC and the corresponding AUC0-6. In all cases the difference between the normalized reduced interval AUC estimates in the AUC0-3 and AUC0-4 datasets were <20% of the corresponding mean parameter estimate. Similarly, analysis of the correlation of the raw midazolam AUC measured over 0 to 3, 0 to 4, and 0 to 6 h versus the full midazolam AUC (AUC0-inf) demonstrated that in all cases the R2 for the correlation was greater than 0.875 (Figure 4).
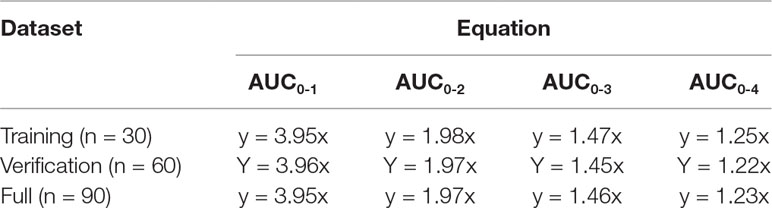
Table 4 Equations relating reduced sampling duration AUCs to AUC0-6 in the training, verification and full (pooled) datasets.
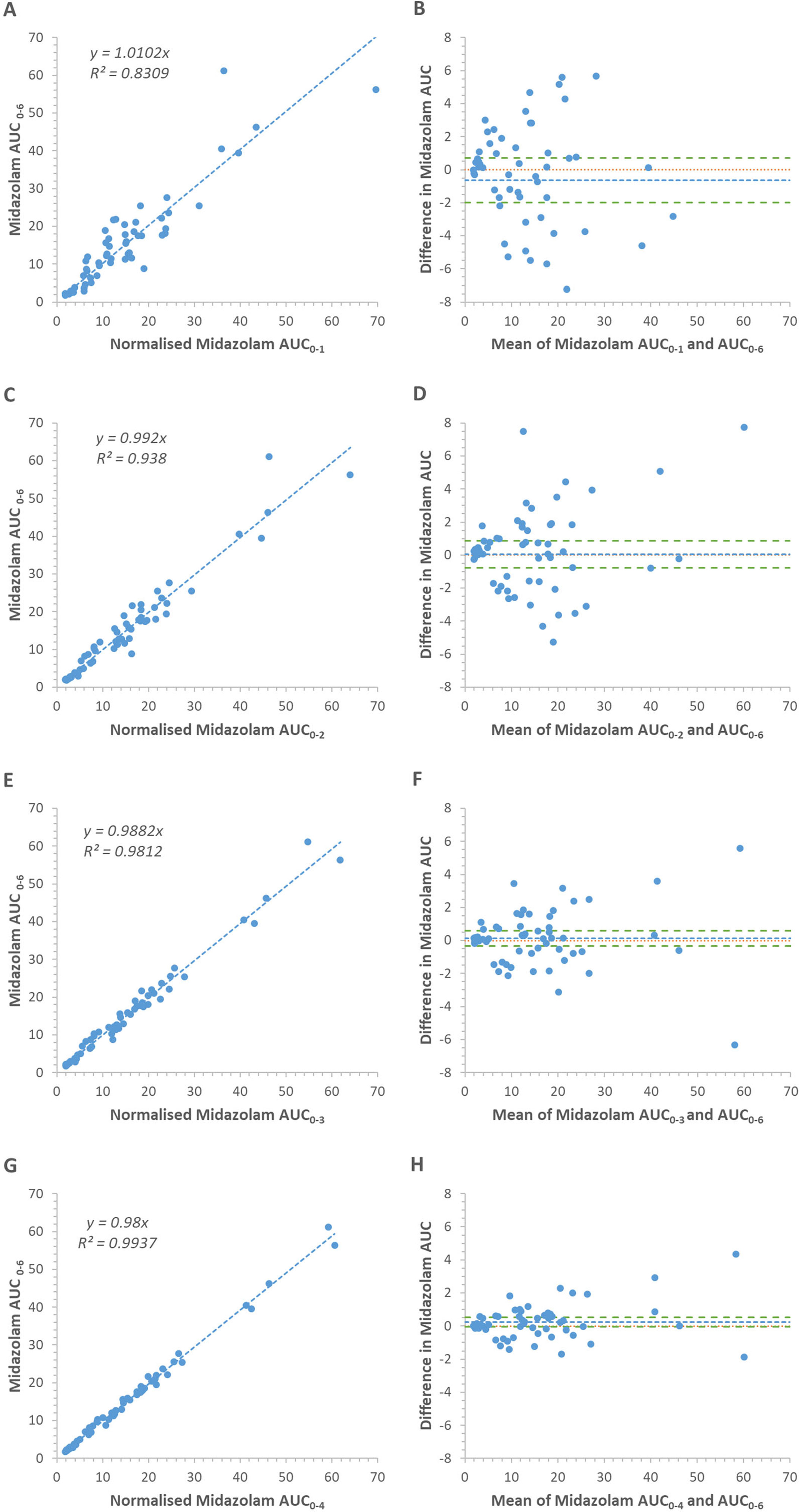
Figure 3 Concordance of midazolam exposure between normalized reduced sampling interval AUCs and AUC0-6 in the validation cohort. (A, C, E, and G) Correlation plots for the AUC0-1, AUC0-2, AUC0-3 and AUC0-4 sampling intervals respectively. (B, D, F, and H) Bland-Altman plots (mean of normalized reduced sampling interval AUCs and AUC0-6 parameters versus difference between normalized reduced sampling interval AUCs and AUC0-6 parameters) for the AUC0-1, AUC0-2, AUC0-3, and AUC0-4 sampling intervals, respectively. Lines describe the mean difference (blue line), 95% confidence interval around the mean difference (green lines) and the zero difference reference (orange line). Units: AUC; ng/mL/h.
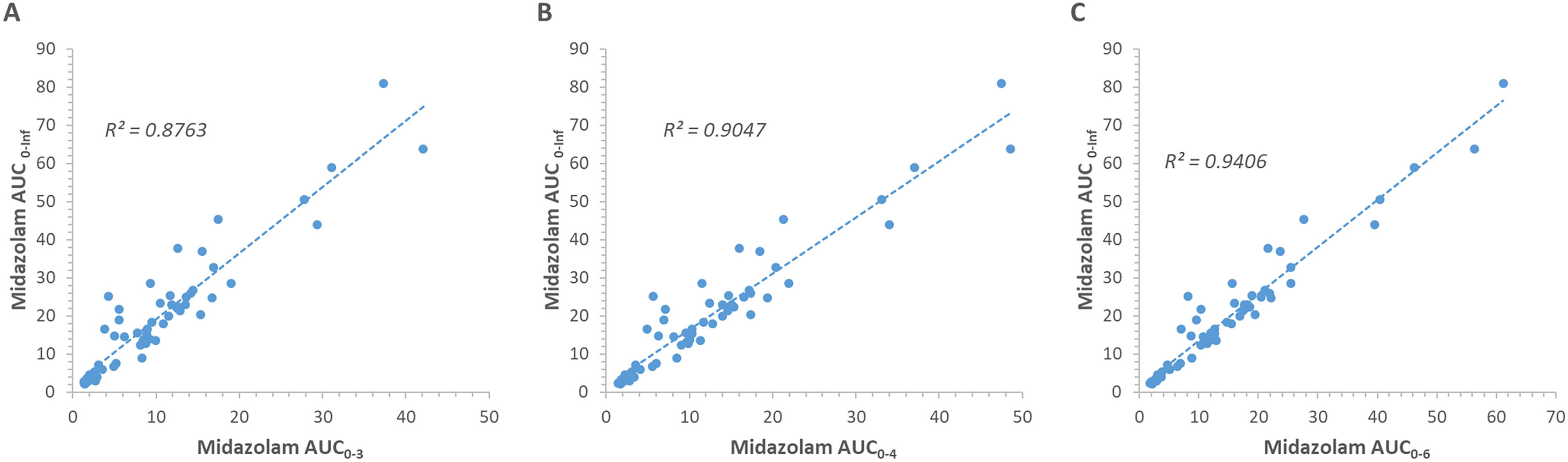
Figure 4 Concordance of midazolam exposure between AUC0-3, AUC0-4, and AUC0-6 and the full AUC (AUC0-inf) in the validation cohort. (A, B, C) Correlation plots for the AUC0-3, AUC0-4, and AUC0-6 sampling intervals, respectively. Units: AUC; ng/mL/h.
Capacity of Reduced Sampling Interval to Define Change in Midazolam AUC Due to Induction
Correlation of the AUC0-3 (R2 = 0.94) and AUC0-4 (R2 = 0.98) ratios with the AUC0-6 ratio fulfilled the criteria of an R2 > 0.85, whereas the R2 for the correlation of the AUC0-1 and AUC0-2 ratios with the AUC0-6 ratio failed to meet this criteria. One-sample t-tests demonstrated that in all cases the difference between the reduced interval AUC ratios and the AUC0-6 ratio were not significantly different from 0 (p ≥ 0.062). Linear regression analysis demonstrated that there was no effect of the mean AUC ratio on the difference in the AUC ratio, indicating a lack of proportional bias within each dataset (p ≥ 0.065). Based on fulfilment of the criteria for acceptance of precision and accuracy, the shortest acceptable sampling interval for assessment of induction of midazolam AUC was 0 to 3 h. The correlation of the Day 8/Day 1 (induction) AUC ratio for the AUC0-3 reduced sampling interval compared to the AUC0-6 ratio is presented in Figure 5A. Bland-Altman plots for each reduced sampling interval AUC are presented in Supplemental Figure 1.
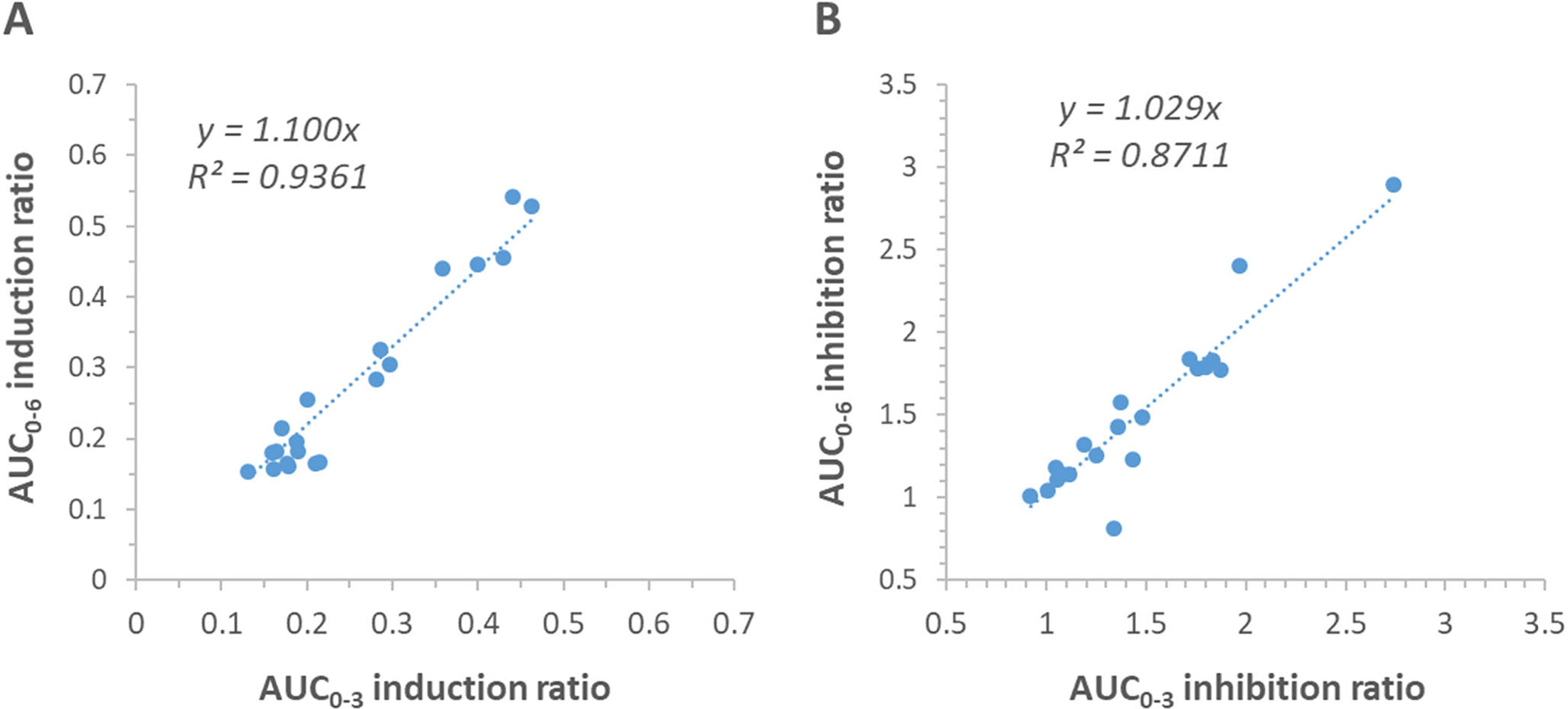
Figure 5 Correlation plots demonstrating the concordance of the change in midazolam AUC due to induction (A) and mechanism-based inhibition (B) between the normalized 0 to 3 h reduced sampling interval AUC (AUC0-3) and AUC0-6 in the verification cohort.
Capacity of Reduced Sampling Interval to Define Change in Midazolam AUC Due to Inhibition
Correlation of the AUC0-3 (R2 = 0.87) and AUC0-4 (R2 = 0.97) ratios with the AUC0-6 ratio fulfilled the criteria of an R2 > 0.85, whereas the R2 for the correlation of the AUC0-1 and AUC0-2 ratios with the AUC0-6 ratio failed to meet this criteria. One-sample t-tests demonstrated that in all cases the difference between the reduced interval AUC ratios and AUC0-6 ratio were not significantly different from 0 (p ≥ 0.148). Linear regression analysis demonstrated that there was no effect of the mean midazolam AUC ratio on the difference in midazolam AUC ratio, indicating a lack of proportional bias within each dataset (p ≥ 0.277). Based on fulfilment of the criteria for acceptance of precision and accuracy, the shortest acceptable sampling interval for assessment of induction of midazolam AUC was 0 to 3 h. The correlation of the Day 15/Day 1 (mechanism-based inhibition) AUC ratio for the AUC0-3 reduced sampling interval compared to the AUC0-6 ratio is presented in Figure 5B. Bland-Altman plots for each reduced sampling interval AUC are presented in Supplemental Figure 2.
Comparison of Midazolam AUC0-3 With Reported Alternate Metrics
Results described above evaluate the absolute performance of the midazolam AUC0-3 with respect to defining midazolam exposure. The capacity of midazolam AUC0-3 to define midazolam exposure was also evaluated with respect to comparative performance by reproducing previously proposed metrics in the current validation dataset.
Consistent with the prior analysis of Chaobal and Kharasch (2005), the correlation of single time point midazolam concentrations at 3, 4, and 6 h with midazolam AUC0-6 varied between time point and study phases; R2 values ranged from 0.357 (6 h control phase) and 0.917 (3 h inhibition phase) (Supplemental Figures 3A–C). Correlations of single time point measures with AUC0-6 were consistently strongest at the 3 h time point (R2 0.809, 0.879, and 0.917 for baseline, induced, and inhibited phases, respectively), and for the inhibition study phase (R2 0.917, 0.888, and 0.742 for 3, 4, and 6 h time points, respectively). In comparison, the R2 vales for the correlation of midazolam AUC0-3 with AUC0-6 for the control, induction, and inhibition study phases were 0.931, 0.906, and 0.971, respectively (Supplemental Figure 3D).
Similarly, consistent with the conclusion of Lee et al. (2006), correlation of single time point OH-midazolam to midazolam ratios at 3, 4, and 6 h with AUC0-6 consistently yielded R2 values <0.8 for both individual study phases and pooled study phases (not shown).
Discussion
Here we describe, for the first time, the validation of a 3-h midazolam sampling interval to support the assessment of between-subject variability in CYP3A phenotype across specific sub-populations as well as the evaluation of changes in CYP3A function due to induction and mechanism-based inhibition of this enzyme. The key distinctions between the current analysis and prior analyses (Lee et al., 2006; Katzenmaier et al., 2010; Mueller and Drewelow, 2013; Tai et al., 2013; Masters et al., 2015; Yang et al., 2018) are a) the current study design has allowed for not only the validation of performance under normal, induced and inhibited conditions, but critically also the validation of performance with respect to describing the change in CYP3A function caused by induction and inhibition, and b) the primary focus of the current analysis was to reduce the duration over which samples are collected (i.e. retained rich sampling in the first 2 h), whereas prior analyses have focused on minimizing the number of sample collections (i.e., fewer samples, but at times over a longer period up to 8 h). The robustness of the approach is supported by post-hoc analysis demonstrating that the scaling factor used to normalize the reduced interval AUCs in the training dataset were independently reproducible in the verification and full datasets. Based on these data, a reproducible scaling factor of 1.46 (full dataset value) is proposed to facilitate future normalizations of AUC0-3 data to prior or alternate studies where AUC0-6 has been measured.
In this regard, prior pooled analyses of three (Kim et al., 2002) and seven (Masters et al., 2015) trials did evaluate the performance of reduced sampling interval AUCs under normal, induced and inhibited conditions, however, data for each set of conditions came from different study cohorts. Critically the use of different cohorts for each set of conditions precluded these prior studies from evaluating performance with respect to the change in CYP3A function caused by induction or inhibition. Similarly, the prior study of Katzenmaier et al. (2010) only considered the performance of reduced sampling interval AUCs under normal conditions. Indeed, the sampling interval proposed by Katzenmaier et al. (2010) explicitly prevents interpretation of the changes midazolam bioavailability (which are predominantly observed as changes in the maximal plasma concentration; Cmax), which, given the low midazolam basal fg of 0.26, is a major factor driving the reduction in midazolam AUC associated with induction of CYP3A (Kapetas et al., 2019). Furthermore, it is worth noting that while the current study validated a longer reduced PK sampling interval compared to Katzenmaier et al. (2010) (3 h versus 2 h), the actual time commitment for study participants and staff following midazolam dosing proposed in the current study is 1 h shorter (3 h versus 4 h) as the sampling interval of Katzenmaier et al. has a 2-h lag phase between midazolam dosing and sample collection. Similarly, consistent with the findings of Lee et al. (2006) and Chaobal and Kharasch (2005), AUC0-3 outperformed both single time point midazolam and single time point OH-midazolam to midazolam ratios with respect to defining variability in midazolam AUC0-6.
Caution is recommended when extrapolating AUC0-inf based on a measured AUC defining <80% due to a risk of bias and variance associated with the estimation of the elimination rate constant (Purves, 1992). In the cohort analyzed here (n = 30; 90 data points), extrapolation of the midazolam AUC measured over six hours (AUC0-6) to infinity accounted for 82%, 83%, and 80% of the AUC0-inf for the baseline, induced, and inhibited study phases, respectively. By comparison, extrapolation of the AUC0-4 accounted for only 66%, 68%, and 64% of the AUC0-inf for the baseline, induced, and inhibited phases, respectively. This limitation is important to consider when determination of midazolam apparent oral clearance (CL/F) is the primary variable being considered, as calculation of CL/F requires robust determination of AUC0-inf. Under such circumstances the use of a reduced sampling interval AUC may not be appropriate as this strategy does not meet the criteria for extrapolation of AUC0-inf. It is worth noting however, that data presented in Figure 4 support the capacity of reduced sampling intervals measured over 0 to 3 and 0 to 4 h to define >87% of the variability in AUC0-inf. Two further limitations that are specific to the current analysis were 1) that while the cohort contained individuals of an appropriate age range for healthy volunteer studies (i.e., 21 to 35 years), the cohort was exclusively male, and 2) the full AUC (AUC0-inf) was estimated by extrapolation of the AUC0-6 data. However, 1) there is no reason to suspect a gender difference in the performance of a reduced sampling interval AUC given that there is not a statistically significant gender difference observed for the change in midazolam AUC0-6 due to induction of CYP3A4 (Kapetas et al., 2019). Similarly, 2) the AUC0-6 data accounted for >80% of the full AUC, and thus is likely to provide a robust estimation of this parameter, although full validation of this approach metric should be undertaken in either a prospective study, or by reanalysis of an existing dataset where AUC0-24 has been captured.
The reduction in logistical burden associated with reducing the sampling interval for midazolam-based CYP3A phenotyping is likely to have the greatest impact for research performed in academia and clinical sites. Typically, in the pharmaceutical industry, drug-drug interaction (DDI) studies with midazolam are typically designed to assess drug concentration out to 24–48 h to calculate AUC over this PK sampling interval as well as AUCinf. Depending on the mechanism of the DDI (inhibition vs induction) and magnitude (none-weak-moderate-strong), such protocols make use of all available data points to calculate midazolam AUC (within assay limits). There may however be industry applications to a reduced sampling interval AUC for midazolam-based CYP3A phenotyping, in terms of reducing blood draws when phenotyping pediatrics, elderly or other vulnerable populations.
A number of recent studies have attempted to remove the requirement to dose a drug probe to assess CYP3A phenotype by measuring either an endogenous small molecule substrate for this enzyme (Wang et al., 1997; Diczfalusy et al., 2011; Bjorkhem-Bergman et al., 2013; Mårde Arrhén et al., 2013), or endogenous protein/nucleic acid biomarker for this enzyme (Rodrigues and Rowland, 2018). While endogenous exosome derived biomarkers (Rowland et al., 2019) appear to represent a promising avenue moving forward, the robust validation of such strategies in both healthy volunteer and patient cohorts require substantial work, thus clinical phenotyping for CYP3A, based on midazolam PK assessment, will likely remain an important tool in both academic and industry research settings for many years.
In conclusion, the current study demonstrates a robust concordance between midazolam AUC calculated by either 0 to 3 and 0 to 6 h PK sampling intervals in terms of both the measurement of between subject variability and changes in midazolam exposure due to induction and mechanism-based inhibition of CYP3A4. On this basis, it is proposed that assessment of a 0 to 3hr midazolam AUC (AUC0-3) represents a viable strategy to reduce the logistical burden associated with the assessment of CYP3A phenotype and perturbations of phenotype caused by CYP3A induction or mechanism-based inhibition.
Data Availability Statement
All datasets generated for this study are included in the manuscript/Supplementary Files.
Ethics Statement
The study protocol was approved by the Southern Adelaide Clinical Human Research Ethics Committee (SAHREC 11.15), and written informed consent was obtained from each participant prior to study enrolment. The study was conducted according to the principles stated in the Declaration of Helsinki, was compliant with CPMP/ICH/135/95 GCP standards, and is registered with the Australian New Zealand Clinical Trials Registry (ACTRN 12614001289606).
Author Contributions
Designed the research: MD, ADR, MV, MS, and AR. Sample collection and analysis: MD and AR. Data analysis: AK, AH, and AR. Wrote the manuscript: MD, AK, AH, ADR, MV, MS, and AR.
Funding
This study was supported by a project grant [1100179] from the National Health and Medical Research Council of Australia. AR is supported by a Beat Cancer Mid-Career Fellowship from Cancer Council, Australia. AH is supported by a Postdoctoral Fellowship from the National Breast Cancer Foundation, Australia.
Conflict of Interest
MS and AR are recipients of industry funding for investigator initiated clinical trials from Pfizer Inc. Pfizer Inc provided support with study design and preparation of the manuscript. ADR and MV are employees and stock holders of Pfizer Inc.
The remaining authors declare that the research was conducted in the absence of any commercial or financial relationships that could be construed as a potential conflict of interest.
Supplementary Material
The Supplementary Material for this article can be found online at: https://www.frontiersin.org/articles/10.3389/fphar.2019.01120/full#supplementary-material
References
Bjorkhem-Bergman, L., Backstrom, T., Nylen, H., Ronquist-Nii, Y., Bredberg, E., Andersson, T. B., et al. (2013). Comparison of endogenous 4beta-hydroxycholesterol with midazolam as markers for CYP3A4 induction by rifampicin. Drug Metab. Dispos. 41 (8), 1488–1493. doi: 10.1124/dmd.113.052316
Burhenne, J., Halama, B., Maurer, M., Riedel, K.-D., Hohmann, N., Mikus, G., et al. (2012). Quantification of femtomolar concentrations of the CYP3A substrate midazolam and its main metabolite 1’-hydroxymidazolam in human plasma using ultra performance liquid chromatography coupled to tandem mass spectrometry. Anal. Bioanal. Chem. 402 (7), 2439–2450. doi: 10.1007/s00216-011-5675-y
Chaobal, H. N., Kharasch, E. D. (2005). Single-point sampling for assessment of constitutive, induced, and inhibited cytochrome P450 3A activity with alfentanil or midazolam. Clin. Pharmacol. Ther. 78 (5), 529–539. doi: 10.1016/j.clpt.2005.08.004
Diczfalusy, U., Nylén, H., Elander, P., Bertilsson, L. (2011). 4β-hydroxycholesterol, an endogenous marker of CYP3A4/5 activity in humans. Br. J. Clin. Pharmacol. 71 (2), 183–189. doi: 10.1111/j.1365-2125.2010.03773.x
Eap, C. B., Buclin, T., Cucchia, G., Zullino, D., Hustert, E., Bleiber, G., et al. (2004). Oral administration of a low dose of midazolam (75 microg) as an in vivo probe for CYP3A activity. Eur. J. Clin. Pharmacol. 60 (4), 237–246. doi: 10.1007/s00228-004-0762-z
US Food and Drug Administration (FDA). (2017). Clinical drug interaction studies: study design, data analysis, and clinical implications guidance for industry center for drug evaluation and research.
Hohmann, N., Kocheise, F., Carls, A., Burhenne, J., Haefeli, W. E., Mikus, G. (2015). Midazolam microdose to determine systemic and pre-systemic metabolic CYP3A activity in humans. Br. J. Clin. Pharmacol. 79 (2), 278–285. doi: 10.1111/bcp.12502
Kapetas, A., Sorich, M., Rodrigues, A. (2019). A R. Guidance for rifampin and midazolam dosing protocols to study intestinal and hepatic cytochrome P450 (CYP) 3A4 induction and de-induction. AAPS J. 21 (5), 78. doi: 10.1208/s12248-019-0341-y
Katzenmaier, S., Markert, C., Mikus, G. (2010). Proposal of a new limited sampling strategy to predict CYP3A activity using a partial AUC of midazolam. Eur. J. Clin. Pharmacol. 66 (11), 1137–1141. doi: 10.1007/s00228-010-0878-2
Kim, J. S., Nafziger, A. N., Tsunoda, S. M., Choo, E. E., Streetman, D. S., Kashuba, A. D., et al. (2002). Limited sampling strategy to predict AUC of the CYP3A phenotyping probe midazolam in adults: application to various assay techniques. J. Clin. Pharmacol. 42 (4), 376–382. doi: 10.1177/00912700222011418
Lee, L. S., Bertino, J. S., Jr., Nafziger, A. N. (2006). Limited sampling models for oral midazolam: midazolam plasma concentrations, not the ratio of 1-hydroxymidazolam to midazolam plasma concentrations, accurately predicts AUC as a biomarker of CYP3A activity. J. Clin. Pharmacol. 46 (2), 229–234. doi: 10.1177/0091270005283466
Mårde Arrhén, Y., Nylén, H., Lövgren-Sandblom, A., Kanebratt, K. P., Wide, K. (2013). Diczfalusy U. A comparison of 4β-hydroxycholesterol:cholesterol and 6β-hydroxycortisol : cortisol as markers of CYP3A4 induction. Br. J. Clin. Pharmacol. 75 (6), 1536–1540. doi: 10.1111/bcp.12016
Masters, J. C., Harano, D. M., Greenberg, H. E., Tsunoda, S. M., Jang, I.-J., Ma, J. D. (2015). Limited sampling strategy of partial area-under-the-concentration-time-curves to estimate midazolam systemic clearance for cytochrome P450 3A phenotyping. Ther. Drug Monit. 37 (1), 84–89. doi: 10.1097/FTD.0000000000000116
Mueller, S. C., Drewelow, B. (2013). Evaluation of limited sampling models for prediction of oral midazolam AUC for CYP3A phenotyping and drug interaction studies. Eur. J. Clin. Pharmacol. 69 (5), 1127–1134. doi: 10.1007/s00228-012-1437-9
Purves, R. D. (1992). Bias and variance of extrapolated tails for area-under-the-curve (AUC) and area-under-the-moment-curve (AUMC). J. Pharmacokinet. Biopharm. 20 (5), 501–510. doi: 10.1007/BF01061468
Rodrigues, A., Rowland, A. (2018). From endogenous compounds as biomarkers to plasma-derived nanovesicles as liquid biopsy; has the golden age of translational PK-ADME-DDI science finally arrived? Clin. Pharmacol. Ther. 105 (6), 1407–1420. doi: 10.1002/cpt.1328
Rowland, A., Mangoni, A. A., Hopkins, A., Sorich, M. J., Rowland, A. (2016). Optimized cocktail phenotyping study protocol using physiological based pharmacokinetic modeling and in silico assessment of metabolic drug-drug interactions involving modafinil. Front. Pharmacol. 7, 517. doi: 10.3389/fphar.2016.00517
Rowland, A., van Dyk, M., Mangoni, A. A., Miners, J. O., McKinnon, R. A., Wiese, M. D., et al. (2017). Kinase inhibitor pharmacokinetics: comprehensive summary and roadmap for addressing inter-individual variability in exposure. Expert Opin. Drug Metab. Toxicol. 13 (1), 31–49. doi: 10.1080/17425255.2016.1229303
Rowland, A., van Dyk, M., Warncken, D., Mangoni, A., Sorich, M., Rowland, A. (2018). Evaluation of modafinil as a perpetrator of metabolic drug-drug interactions. Br. J. Clin. Pharmacol. 84, 501–509. doi: 10.1111/bcp.13478
Rowland, A., Ruanglertboon, W., van Dyk, M., Wijayakumara, D., Wood, L., Meech, R., et al. (2019). Plasma extracellular nanovesicle (exosome) derived biomarkers for ADME pathways: a novel approach to characterise variability in drug exposure. Br. J. Clin. Pharmacol. 85 (1), 216–226. doi: 10.1111/bcp.13793
Ryu, J. Y., Song, I. S., Sunwoo, Y. E., Shon, J. H., Liu, K. H., Cha, I. J., et al. (2007). Development of the “Inje cocktail” for high-throughput evaluation of five human cytochrome P450 isoforms in vivo. Clin. Pharmacol. Ther. 82 (5), 531–540. doi: 10.1038/sj.clpt.6100187
Shih, P.-S., Huang, J.-D. (2002). Pharmacokinetics of midazolam and 1′-hydroxymidazolam in Chinese with different CYP3A5 genotypes. Drug Metab. Dispos. 30 (12), 1491–1496. doi: 10.1124/dmd.30.12.1491
Tai, W., Gong, S. L., Tsunoda, S. M., Greenberg, H. E., Gorski, J. C., Penzak, S. R., et al. (2013). Evaluation of partial area under the concentration time curve to estimate midazolam apparent oral clearance for cytochrome P450 3A phenotyping. Drug Metab. Drug Interact. 28 (4), 217–223. doi: 10.1515/dmdi-2013-0040
Thummel, K. E., Shen, D. D., Podoll, T. D., Kunze, K. L., Trager, W. F., Bacchi, C. E., et al. (1994a). Use of midazolam as a human cytochrome P450 3A probe: II. Characterization of inter- and intraindividual hepatic CYP3A variability after liver transplantation. J. Pharmacol. Exp. Ther. 271 (1), 557–566.
Thummel, K. E., Shen, D. D., Podoll, T. D., Kunze, K. L., Trager, W. F., Hartwell, P. S., et al. (1994b). Use of midazolam as a human cytochrome P450 3A probe: I. In vitro-in vivo correlations in liver transplant patients. J. Pharmacol. Exp. Ther. 271 (1), 549–556.
Thummel, K. E., O’Shea, D., Paine, M. F., Shen, D. D., Kunze, K. L., Perkins, J. D., et al. (1996). Oral first-pass elimination of midazolam involves both gastrointestinal and hepatic CYP3A-mediated metabolism. Clin. Pharmacol. Ther. 59 (5), 491–502. doi: 10.1016/S0009-9236(96)90177-0
van Dyk, M., Marshall, J. C., Sorich, M. J., Wood, L. S., Rowland, A. (2018). Assessment of inter-racial variability in CYP3A4 activity and inducibility among healthy adult males of Caucasian and South Asian ancestries. Eur. J. Clin. Pharmacol. 74 (7), 913–920. doi: 10.1007/s00228-018-2450-4
Wang, R. W., Newton, D. J., Scheri, T. D., Lu, A. Y. H. (1997). Human Cytochrome P450 3A4-Catalyzed testosterone 6β-hydroxylation and erythromycinN-demethylation: competition during catalysis. Drug Metab. Dispos. 25 (4), 502–507.
Williams, J. A., Ring, B. J., Cantrell, V. E., Jones, D. R., Eckstein, J., Ruterbories, K., et al. (2002). Comparative metabolic capabilities of CYP3A4, CYP3A5, and CYP3A7. Drug Metab. Dispos. 30 (8), 883–891. doi: 10.1124/dmd.30.8.883
Winters, S. J., Clark, B. J. (2003). “Testosterone Synthesis, Transport, and Metabolism,” in Androgens in Health and Disease. Eds. Bagatell, C. J., Bremner, W. J. (Totowa, NJ: Humana Press), 3–22. doi: 10.1385/1-59259-388-7:3
Yang, J., Patel, M., Nikanjam, M., Capparelli, E. V., Tsunoda, S. M., Greenberg, H. E., et al. (2018). Midazolam single time point concentrations to estimate exposure and cytochrome P450 (CYP) 3A constitutive activity utilizing limited sampling strategy with a population pharmacokinetic approach. J. Clin. Pharmacol. 58 (9), 1205–1213. doi: 10.1002/jcph.1125
Keywords: clarithromycin, cytochrome P450 3A4, induction, mechanism-based inhibition, midazolam, phenotyping studies, rifampin
Citation: van Dyk M, Kapetas AJ, Hopkins AM, Rodrigues AD, Vourvahis M, Sorich MJ and Rowland A (2019) Validation of a 3-h Sampling Interval to Assess Variability in Cytochrome P450 3A Phenotype and the Impact of Induction and Mechanism-Based Inhibition Using Midazolam as a Probe Substrate. Front. Pharmacol. 10:1120. doi: 10.3389/fphar.2019.01120
Received: 02 May 2019; Accepted: 30 August 2019;
Published: 27 September 2019.
Edited by:
David Stec, University of Mississippi Medical Center, United StatesReviewed by:
Paavo Honkakoski, University of Eastern Finland, FinlandYvonne Lin, University of Washington, United States
Copyright © 2019 van Dyk, Kapetas, Hopkins, Rodrigues, Vourvahis, Sorich and Rowland. This is an open-access article distributed under the terms of the Creative Commons Attribution License (CC BY). The use, distribution or reproduction in other forums is permitted, provided the original author(s) and the copyright owner(s) are credited and that the original publication in this journal is cited, in accordance with accepted academic practice. No use, distribution or reproduction is permitted which does not comply with these terms.
*Correspondence: Andrew Rowland, QW5kcmV3LnJvd2xhbmRAZmxpbmRlcnMuZWR1LmF1