- 1Department of Clinical Pharmacy and Pharmacology, University Medical Center Groningen, University of Groningen, Groningen, Netherlands
- 2Department of General Practice, University Medical Center Groningen, University of Groningen, Groningen, Netherlands
- 3Department of Clinical Pharmacology and Pharmacy, VU University Medical Center, Amsterdam, Netherlands
- 4Department of Guideline Development and Research, The Dutch College of General Practitioners, Utrecht, Netherlands
- 5Department of Clinical Pharmacy and Toxicology, Leiden University Medical Center, Leiden, Netherlands
- 6Unit of PharmacoTherapy, Epidemiology and Economics, Groningen Research Institute of Pharmacy, University of Groningen, Groningen, Netherlands
Aim: To develop and pilot an algorithm to select older people for different types of medication review based on their case complexity.
Methods: Experts rated complexity of patient cases through a Delphi-consensus method. The case characteristics were included in a regression model predicting complexity to develop a criteria-based algorithm. The algorithm was piloted in four community pharmacies with 38 patients of high and low complexity. Pharmacists conducted medication reviews according to their personal judgment and rated the patients' complexity. Time needed for reviewing and number of interventions (proposed and implemented) were assessed. Feasibility was evaluated with in-depth interviews.
Results: We developed the algorithm with 75 cases proceeding from patients in average 79 years old and using 10 prescribed medications. The regression model (adjusted R2 = 0.726, P < 0.0001) resulted in the following criteria for the algorithm: “number of medications” × 1 + “number of prescribers” × 3 + “recent fall incident” × 7 + “does not collect own medication” × 4. The pharmacists performed advanced medication reviews with all patients. The time needed to perform the medication review did not differ significantly according to case complexity (76.9 min for high complexity; 66.1 min for low complexity). Agreement between the algorithm scores and the pharmacists' ratings on complexity degree was slight to moderate (Kappa 0.16–0.42). The pharmacists had mixed opinions about the feasibility of applying the algorithm, particularly regarding the criterion “fall incidents.”
Conclusion: We developed an algorithm with four criteria that distinguished between high and low complexity patients as rated by experts. Additional validation steps are needed before implementation.
Introduction
Aging and polypharmacy are associated with an increased risk of medication-related harm (Leendertse et al., 2008; Kaufmann et al., 2015). Medication reviews have shown to reduce the number of inappropriately used medications and resultant drug-related problems (Holland et al., 2008). Medication reviews have been implemented in many countries (Bulajeva et al., 2014), although effects on outcomes like hospital admissions or quality of life have not been consistently demonstrated (Holland et al., 2005, 2008; Lenaghan et al., 2007; Hatah et al., 2014; Huiskes et al., 2017; Hurmuz et al., 2018). Three types of medication reviews have been put forward (Blenkinsopp et al., 2012; Griese-Mammen et al., 2018). These are simple reviews based on the pharmacy medication history only, intermediate reviews for which the patient provides additional information and advanced reviews that make use of more extensive clinical information. Currently there are no uniform or evidence-based strategies to select patients for different types of medication reviews. Commonly used criteria to select patients for medication reviews include an age of 65 years or older and the chronic use of multiple medications (Huiskes et al., 2017). In the Netherlands, advanced clinical medication reviews are recommended for all older patients with a high case complexity (Nederlands Huisartsen Genootschap, 2012). Case complexity refers to the patient's overall status, including polypharmacy, multimorbidity, and psycho-social problems (Huyse et al., 2007). The Dutch guideline states that patients should be selected for an advanced medication review if they are ≥65 years of age, take ≥5 chronic medications and have at least one additional risk factor. These risk factors include having a reduced kidney function, a diminished cognition, an increased fall risk, low medication adherence, an unplanned hospitalization in the past year, or living in a nursing home.
Selection of patients according to the Dutch guideline recommendations turned out to be not feasible in practice. Information regarding the additional risk factors is not routinely available to the community pharmacists. Without this information large numbers of patients were selected, though not all needed an advanced medication review (Pharmaceutisch Weekblad, 2015). The laborious nature of advanced reviews unnecessarily burdens healthcare professionals. Therefore, the Dutch Healthcare Inspectorate decided that advanced medication reviews should at least be conducted for all patients of ≥75 years of age, with ≥7 chronic medications and a decreased kidney function (Landelijke Huisartsen Vereniging, 2016; Pharmaceutisch Weekblad, 2016). This pragmatic approach received criticism because it would lead to the exclusion of a large group of older patients for whom a medication review could be beneficial. A new approach with different criteria is needed that allows patients to be allocated to different types of medication reviews.
The aim of our study is firstly to develop a criteria-based algorithm for the allocation of older people (≥65 years and with ≥5 medications) for simple or advanced medication reviews, based on their case complexity. Secondly, we piloted the algorithm in the community pharmacy setting.
Methods
Algorithm Development
We used judgment analysis to derive the criteria predicting case complexity to be included in the algorithm (Cooksey, 1996). Two panels of experts provided patient cases and rated their complexity. Panel A consisted of four community pharmacists and four general practitioners (GPs) and panel B consisted of five community pharmacists and three GPs.
Patient Cases
The experts were asked to submit four to six cases of patients using at least five chronic medications with varying complexity. High complexity was defined as a case for which existing treatment guidelines cannot be easily applied and an advanced medication review is required to optimize treatment. Low complexity was defined as a case for which existing treatment guidelines can be applied as part of a simple medication review in order to optimize treatment. The experts completed a structured case report form with case characteristics (Appendix I). This consisted of the patient's medication, morbidity, laboratory measurements, demographics, estimated medication use issues, estimated beliefs about medication and other possible risk factors for drug-related problems such as diminished cognition. The goal was to obtain 80 cases; using the rule of 10 subjects per predictor, this would be sufficient to develop an algorithm with eight criteria using regression modeling (Morgan et al., 2007). To achieve this number, we used all cases provided by the experts and generated additional cases. We modified a number of characteristics from a subset of randomly selected experts' cases. Level of education, eyesight, age, sex, and medication intake issues were systematically changed. Also, we exchanged one health issue and its corresponding medication with another health issue and its corresponding medication. All additional cases were checked by two researchers to ensure they remained realistic.
Expert Ratings
All cases were rated by the two expert panels using a modified Delphi method. For this, each panel rated the complexity of cases provided by the opposite panel on a 9-point Likert scale (1 = low complexity 9 = high complexity). Consensus per case was calculated according to the Interpercentile Range Adjusted for Symmetry method (Fitch et al., 2001). This method corrects for outliers by using the range between the 0.3 and the 0.7 percentile instead of the full range. A correction factor is used to adjust for symmetry of the ratings. Cases for which there was no consensus were discussed in a consensus meeting. A case was presented, the experts explained and discussed why they had given a specific rating of complexity, and after the discussion the experts were asked whether they wanted to reconsider their rating. For each case, the median of the individual complexity ratings of the experts was calculated.
Building the Algorithm
The algorithm was built using all cases for which consensus had been reached. The median complexity rating was used as the outcome in linear regression models, where potential criteria were included as predictors. A wide range of clinical, medication and patient characteristics were included in the models, some of which could be proxies for criteria that may be difficult to measure. In total 48 potential criteria in 25 categories were tested as predictors (Appendix II). Predictors were clustered when they were derived from the same information or represented a similar concept. A complete case linear regression procedure was followed, with only one predictor from the same cluster included so as to avoid collinearity. Predictors with a P-value of 0.25 or less in the univariate testing were included in the multivariate regression model. Per cluster the predictor with the lowest P-value in univariate testing was included in the first model. Predictors were excluded from the model until excluding another predictor would reduce the adjusted R2 by ≥0.05. When a predictor was removed from the model during the backward stepwise process, alternative predictor(s) from the same cluster were tested. The weights of the predictors of the final regression model were multiplied by and rounded to the nearest whole number. The final algorithm consisted of the weighted predictors (criteria). With this the algorithm scores were calculated where a higher score indicated higher complexity.
Pilot Study
The algorithm was piloted in four community pharmacies. The algorithm scores were compared with complexity ratings of the pharmacists as well as experts. The feasibility of applying the algorithm in practice was assessed with in-depth interviews with the participating pharmacists. The medication review process was evaluated.
Setting
The four participating community pharmacies, located in the northern part of the Netherlands, offer standard pharmaceutical care, including the monitoring of drug-drug interactions, contra-indications, along with dose control, and patient counseling. Furthermore, they have implemented advanced medication reviews in accordance with the Dutch guideline (van der Meer et al., 2015). All pharmacists routinely collaborate with the GPs including regular pharmacotherapy audit meetings.
Patient Selection and Medication Review
Patients for whom a medication review is reimbursed in the Netherlands were eligible for the pilot study. Thus, eligibility was defined as ≥65 years, ≥5 chronic prescriptions and not having received a medication review in the previous 12 months. The pharmacists invited a random sample of 100 patients per pharmacy to participate. Informed consent was collected from patients who accepted to participate. Consenting patients completed a questionnaire either by mail or by phone to collect the additional information needed for the algorithm, that is whether they collect their own medication, the number of prescribers and whether they experienced a fall recently (Appendix III). For each patient, the number of used medication was calculated including all prescriptions for medication at the lowest level ATC code, excluding non-therapeutic ophthalmologicals (S01K, S01J, S02DC), caries prophylactic agents (A01AA), over-the-counter vitamins (belonging to A11), blood substitutes and perfusion solutions (B05), emollients, protective, and other dermatologicals (D02, D11) (ATC/DDD Index, 2018). After applying the algorithm to all respondents we randomly selected five from the highest tertile and five from the lowest tertile of the algorithm scores for a medication review in each pharmacy. We used a random number generator in excel 2010 for this selection. We expected that patients from the highest tertile represented patients with a high complexity and patients from the lowest percentile represented patients with a low complexity. We selected five patients per complexity degree and per pharmacy to comply with time constraints. The pharmacists were instructed to perform an advanced medication review as usual for patients with a high algorithm score and were allowed to conduct any type of medication review for low complexity patients based on their clinical judgment.
Agreement
The participating community pharmacists were asked to rate case complexity of the selected patients on a 9-point Likert scale (1 = low complexity, 9 = high complexity). The need to perform a medication review for these patients was rated on a separate 9-point Likert scale. Similarly, for each case a pharmacist and a GP from the expert panels rated the complexity of the case and the need for a medication review. These ratings were averaged to provide one expert rating per case. Kappa statistics were used to assess the agreement on the complexity degree (high or low) between the algorithm scores and the community pharmacists' ratings. Similarly, this was done for the agreement between the algorithm scores and the experts' ratings. Different cut-off points were tested for the ratings on the 9-point scale, for which the agreement and Kappa were calculated. The Kappa values were interpreted as followed: <0.00 (poor), 0.00–0.20 (slight), 0.21–0.40 (fair), 0.41–0.60 (moderate), 0.61–0.80 (substantial), and 0.81–1.00 almost perfect agreement (Fau and Koch, 1977). Finally, we assessed agreement between the complexity degree (high or low) indicated by the algorithm and the need for a medication review indicated by the participating pharmacist and the experts.
Pharmacists' Views on the Feasibility
To assess feasibility, pharmacists were asked to share their positive and negative views about the use of the new criteria as compared to the current criteria (primary outcome). In-depth interviews were held with the four participating pharmacists by SC. These were face-to-face interviews using a topic list which was developed to assess their views about the criteria of the algorithm and applying them in practice (two domains with eight topics) and explore the setting and procedures regarding the conduct of medication reviews (two domains with six topics). An overview of the topics can be found in Appendix IV. The interviews were recorded, transcribed and thematically coded by SC. A content analysis was conducted to summarize information about the setting in relation to the conducting of medication reviews. A deductive approach was used to attain thematic codes related to the participants' positive and negative views about various criteria and the feasibility of applying such criteria in practice. The data coding related to the setting was checked by KT and thematic codes related to criteria and feasibility were checked by PD.
Medication Review Evaluation
As process evaluation, we assessed the number of proposed and implemented interventions as well as the time needed for each step in the review of patients with high and low complexity scores. The steps were preparation, anamnesis, analysis, consultation with GP, and feedback to patient (van der Meer et al., 2015). For each patient with a medication review a structured case report form was completed by a pharmacist in training together with the pharmacist containing the following data: laboratory outcomes and morbidity from GP medical records, background information obtained during anamnesis in the pharmacy, the proposed and implemented interventions as documented in the pharmacy medication record. Per review step the pharmacist recorded the time involved. T-tests were used to compare the time investment of the pharmacists and the number of proposed and implemented interventions between patients with high and low complexity scores.
Results
The 59 cases provided by the experts combined with 21 additional cases developed from these cases created a total of 80 cases, which were rated by the experts on complexity. In the first Delphi round, consensus was reached on 59 of the 80 cases, including 18 of the additional cases. During the second round consensus was reached on another 16 cases, including three additional cases. Consensus was not obtained for five cases, which were excluded from further analysis. The patient characteristics of the cases included in the algorithm development are shown in Table 1.
Algorithm Development
A total of 14 potential criteria with a p-value of ≥0.25 in the univariate testing were included in the first regression model predicting the experts' complexity rating (Appendix II). An additional 10 alternative potential criteria were tested during the stepwise procedure. In the final model, four criteria remained which significantly predicted the complexity rating (P < 0.0001) with an adjusted R2 of 0.73 (Table 2). The regression model results were used to construct the algorithm by multiplying the coefficients with and rounding this to the nearest whole number. This resulted in the algorithm with the following four criteria and weighting:
′Number of different medications′ × 1 + ′Number of prescribers′ × 3 + ′Fall incident′ × 7 + ′Does not collect own medication′ × 4. This algorithm has a minimum score of 8, since eligible patients have at least five medications and one prescriber. The maximum algorithm score is dependent on the number of medications and prescribers a patient can have.
Pilot Study
Of the 400 invited patients, 166 accepted to participate and completed the questionnaire with information needed for the algorithm. Forty patients were randomly selected for a medication review, two of whom dropped out due to illness. Of the remaining patients, 19 were from the lowest and 19 were from the highest tertile of algorithm scores. General patient characteristics of those two groups, such as age and sex, were similar (Table 3).The pharmacists decided to conduct advanced medication reviews for all 38 patients.
Agreement
Kappa values for agreement on complexity ratings ranged from 0.13 to 0.55 (Table 4). For the pharmacists' ratings, the highest agreement (Kappa of 0.42) was seen for the cut-off point of ≥7 (83.3% of the patients rated with a high complexity of 7 or more by the experts were equally scored by the algorithm). For the experts' ratings, the highest agreement (Kappa of 0.55) was seen for a lower cut-off point of ≥5 (74.3% of the patients rated with a complexity of 5 or more by the pharmacists were equally scored by the algorithm). Agreement on the need for a review between the algorithm scores and the community pharmacists' or the experts' ratings ranged from 0.052 to 0.32 (Table 4). The highest observed Kappa was seen for cut-off point ≥8 for the pharmacists and ≥6 for the experts.
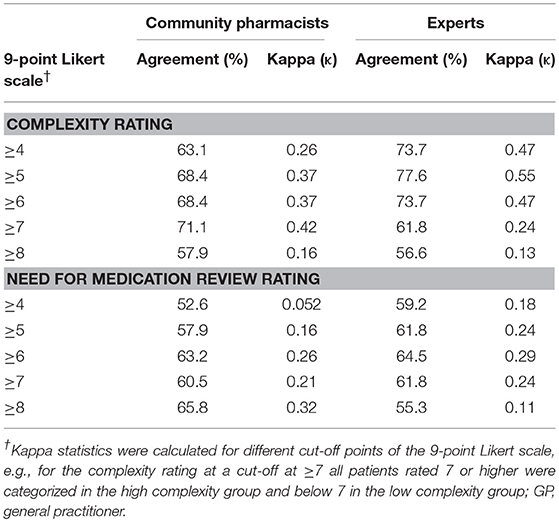
Table 4. Agreement of the algorithm score with the community pharmacists' and experts' ratings on complexity and on need for medication review at different cut-off points.
Pharmacists' Views on the Algorithm
From the interviews, the following five main themes were identified: (1) views about the criteria included in the algorithm, (2) criteria the pharmacists believed that could be relevant for selection but were not included in the algorithm, (3) views about the criteria as proposed by the Healthcare Inspectorate, (4) feasibility of collecting the data needed to apply the algorithm, (5) reliability of the data needed to apply the algorithm (Table 5).
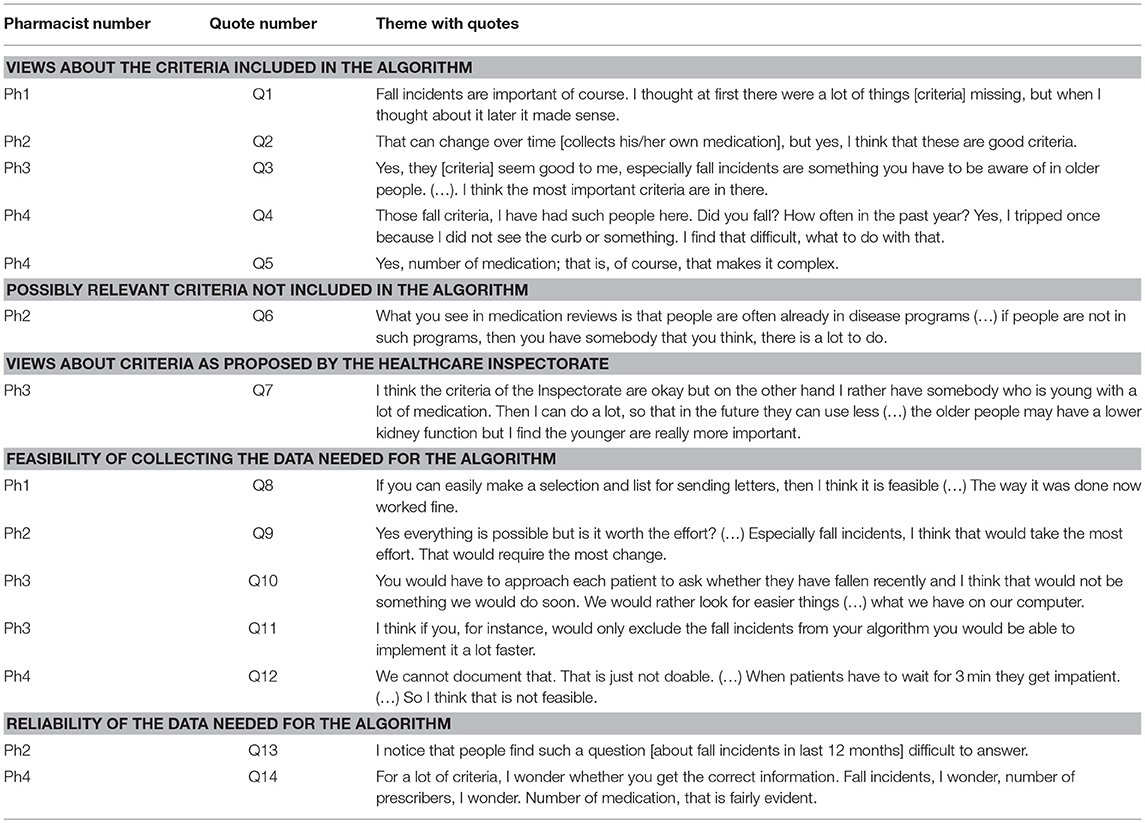
Table 5. Themes with quotes from semi-structured interviews with the pharmacists (translated from Dutch).
Three pharmacists were supportive of including “the number of medications” (Table 5, Q5) and two were supportive of “not collecting their own medication” (Q2) as criteria for medication reviews. One pharmacist saw “the number of prescribers” as a relevant criterion. Using “fall incidents” as criterion elicited mixed reactions with two pharmacists seeing this as important (Q1, Q3) while two others saw “fall incidents” as less relevant in clinical practice (Q4). Three pharmacists suggested “living in a care home,” “diminished cognition,” “patients not included in a healthcare program” (e.g., diabetes or cardiovascular risk management) (Q6) and “patients avoiding healthcare” as potential relevant criteria. Two pharmacists were critical of the Healthcare Inspectorate's current criteria. One observed that the high age criterion would exclude younger patients with polypharmacy, who may benefit from a medication review (Q7). Another expressed a strong negative opinion saying that these criteria were ridiculous, since almost no eligible patients can be selected.
Mixed opinions were expressed as to the feasibility of collecting data needed to apply the algorithm. Sending questionnaires to all eligible patients was considered feasible by two pharmacists (Q8) but judged as not worth the effort by one of them (Q9), whilst a third pharmacist stated that the extra step of collecting the required data would take too much time (Q12). A fourth pharmacist preferred the use of criteria easily obtained from the pharmacy information system (Q10). Collecting the information on the recent fall incidents was considered most difficult and time consuming. Three pharmacists mentioned that asking patients could also lead to unreliable data (Q13, Q14).
Medication Review Evaluation
The total time involved for all the steps of the review was on average 76.9 min for patients with a high complexity score and 66.1 min for patients with a low complexity score (Table 6). The 11 min difference was not statistically significant (P = 0.134).
No difference was found in the number of interventions resulting from the medication review. On average, 2.1 interventions (SD 1.2) were proposed for patients with high scores and 2.3 interventions (SD 1.6) for patients with low scores (P = 0.658). The number of implemented interventions did not differ between patients with high and low complexity scores (1.6 +/− 1.3 vs. 1.6 +/− 1.2; P = 0.51). There was also no difference in the type of proposed interventions (Table 7).
Discussion
We developed an algorithm to select older people with polypharmacy for different types of medication review based on their case complexity. The algorithm used the following criteria to calculate a score: number of different medications, number of prescribers, collecting own medication, and having had a severe fall in the last 12 months. It explained 73% of the variation in complexity as rated by the experts. The agreement between the participating pharmacists' ratings and the algorithm scores on complexity was slight to moderate. Pharmacists in the pilot study had mixed opinions about the feasibility of applying the algorithm in practice. The time needed to collect the information for the criteria in the algorithm appeared to be a major barrier. There was no significant difference in time needed for a medication review between patients with high and low complexity scores.
The number of prescribed medication, as included in our algorithm, is often used as a selection criterion for medication reviews or other medication risk management interventions (Huiskes et al., 2017). In the backward stepwise regression procedure, we included other more sophisticated medication scores but a simple count of the number of medications was found to be the best predictor. This is in line with research showing that the Medication Regimen Complexity Index (MRCI) and the number of prescribed medications are equally predictive for hospitalization (Wimmer et al., 2016). A clear benefit of using a simple medication count compared to more complex scores is that it can be calculated relatively easily from the pharmacy information systems. Whether or not a patient collects his or her own medication is a new and possibly meaningful criterion for selecting patients. This criterion can be seen as a proxy for frailty and vulnerability of the patient. We found that not collecting his/her own medication was correlated with diminished mobility and a smaller social network in the patients on which the algorithm was based (data not shown). Furthermore, patients who do not collect their own medication have less contact with the pharmacy personnel. Reduced opportunities for pharmacy personnel to communicate with the patient complicate patient counseling and detecting medication problems. Fall risk has been proposed before as criterion for conducting medication reviews (Nederlands Huisartsen Genootschap, 2012). Severe falls in the older population are recognized as an important cause of trauma and hospitalizations (Gelbard et al., 2014). In part, these events are medication-related (de Jong et al., 2013). However, evidence is still limited that the withdrawal of high risk fall medication as a singular intervention reduces the number of falls (Gillespie et al., 2012; Boye et al., 2017). The number of prescribers has not yet been used as criterion for medication reviews. It has been shown, however, that patients with a larger number of prescribers have a higher risk of inappropriate prescriptions (Hajjar et al., 2005). Being under the care of multiple physicians possibly indicates higher clinical complexity. Several criteria such as age or kidney function, that are commonly used for selecting patients for medication review (Huiskes et al., 2017), were not part of our selection algorithm. The algorithm was developed for patients of 65 years or older. Differentiating further on age within this group did not contribute to predicting case complexity. This is in line with research showing that in older populations the number of potentially inappropriate medications is not associated with age but rather with frailty (Wawruch et al., 2008; Ruggiero et al., 2010; Poudel et al., 2014). Impaired kidney function has often been associated with an increased risk of medication-related hospitalizations and adverse drug reactions (Leendertse et al., 2008; Onder et al., 2010; Sikdar et al., 2012). However, in the Dutch setting this might be less relevant as criterion since community pharmacists already monitor medication safety in patients with a reduced kidney function (Koster et al., 2016).
The agreement between the algorithm scores on complexity and the pharmacists' ratings was at best moderate. In particular, several patients with low complexity scores as calculated by the algorithm were rated complex by the pharmacists. Agreement was better regarding patients with high complexity, as indicated by the higher Kappa value when using a cut-off score of 7 or more. This implies that the algorithm can be useful to select the more complex patients for an advanced medication review. Some of the remaining patients classified as simple by the algorithm, however, may also be in need of an advanced medication review based on clinical judgment of the healthcare provider. Nonetheless, there were also patients with low complexity scores that received similar low ratings by the pharmacists. In the current system, they received an advanced medication review but it is expected that such less complex patients can receive a more simple and less time consuming review. Reservations on the feasibility of applying the algorithm in practice were primarily seen for collecting information related to recent fall incidents. In contrast to the other criteria, data on fall incidents cannot be acquired from the pharmacy information system. However, eligible patients could be asked by pharmacy staff about recent falls when dispensing the medication. Implementation of pharmaceutical care services in community pharmacies is often impeded by a lack of time (van Mil et al., 2001; Kaae and Christensen, 2012). Hence, in order to successfully implement a criteria-based algorithm the time investment should be minimized by making the selection process automated where possible. Furthermore, time invested in collecting data for the algorithm may be compensated by performing shorter medication reviews for low complexity patients.
All patients received advanced medication reviews regardless of their complexity, possibly because only advanced reviews are currently recommended and reimbursed in the Netherlands. Consequently, differences in time investment for patients with high or low algorithm scores were small and did not reach statistical significance in our pilot study. Time restrictions are among the most important barriers in performing medication reviews (Niquille et al., 2010). A time difference of more than 10 min for low complexity patients would allow a pharmacist to perform one additional review for every six reviews performed in this group. Less time consuming types of medication review, like a concordance or prescription review, might be sufficient in certain low complexity patients (Blenkinsopp et al., 2012).
Strengths and Limitations
A strength of our study is that we used the experts' judgments on case complexity to develop the criteria-based algorithm. This allows for the identification of criteria that can be proxies for criteria that may be difficult to acknowledge or measure, and to search for the criteria that provide an independent contribution. For instance, in the univariate analysis an unplanned hospitalization in the last 12 months was a good predictor for the complexity rating of the experts (Appendix II). However, adding unplanned hospitalization to the regression model failed to substantially improve the model. Thus, although an unplanned hospitalization might have been considered a relevant criterion from an expert's point of view, it did not provide additional information in combination with the other criteria used in the model. Secondly, we used a Delphi method with assessments on 9-point Likert scales, which allowed for achieving a group statistical response, and included two expert panels to increase the reliability of the consensus ratings. There were several limitations to this study. Firstly, the algorithm was based on 80 cases of which 21 were modified. Since the modified cases were fairly similar to the original cases, this might have increased the weight that certain characteristics of these cases had on the algorithm. On the other hand, by changing certain characteristics we may have introduced more variety to these characteristics. Although the characteristics that were varied were not significant in the final model some bias in model building cannot be excluded. Secondly, only patients with high and low algorithm scores were invited for a medication review in the pilot study. Patients in the intermediate group did not receive a review, and no conclusions can be made about this group. Thirdly, out of 400 patients invited, 166 were willing to participate, possibly introducing a selection bias. This rate is similar in relation to other Dutch studies in which advanced medication reviews are conducted (Kwint et al., 2012; Willeboordse et al., 2017). Lastly, the algorithm was applied in four community pharmacies in the northern part of the Netherlands. To obtain more generalizable results, a larger study is warranted.
Implications for Practice and Research
Although the algorithm performed well in predicting case complexity as rated by the experts, the fair to moderate performance for distinguishing between high and low complexity patients in the pilot study indicates that it should only be used as a screening tool for selecting complex patients for advanced medication reviews. This pilot study is a first step toward a new approach which allows for a more evidence-based differentiation in the type of medication reviews conducted in practice. Given the fact that our algorithm was developed using expert consensus on a set of partly modified cases, it needs to be validated in a larger set of patients. This process may lead to adapting the algorithm to improve its performance. Furthermore, optimal cut-off levels for the degree of complexity in relation to the type of medication review need to be confirmed. Finally, studies should be conducted to assess the effects of this new approach on clinical outcomes.
In conclusion, we developed an algorithm with four criteria that differentiates between high and low complexity patients and allows allocation to simple or advanced medication reviews accordingly. In practice, most feasibility problems were seen regarding collection of information about recent falls. Additional steps are needed to confirm the correctness of the four criteria. Furthermore, steps are needed to facilitate data collection for the algorithm, and to evaluate and improve the algorithm before implementing it in practice.
Ethics Statement
The Medical Ethics Review Board from the University Medical Center Groningen considered the pilot study as falling outside the scope of the Dutch Medical Research involving Human Subjects Act.
Author Contributions
SC, JS, JH, MV, MT, KT, and PD: research idea and study design. SC, JS, KT, and PD: data acquisition. SC, KT, and PD: analysis and interpretation. KT and PD: supervision or mentorship. All authors contributed substantially to the intellectual content during manuscript drafting or revision. All authors approved the manuscript and this submission.
Funding
This study has been funded by KNMP- The Royal Dutch Pharmacists Association (Grant No. PR18_0101).
Conflict of Interest Statement
The authors declare that the research was conducted in the absence of any commercial or financial relationships that could be construed as a potential conflict of interest.
Acknowledgments
We would like to thank Zorgbelang Groningen and especially Birgitta Marijnissen for facilitating input from a patient perspective in this research project. We would also like to thank the general practitioners and pharmacists that participated in our expert panels and the community pharmacists involved in the pilot study. Lastly we would like to thank Ebian J. P. Brill for his contributions during this research project.
Supplementary Material
The Supplementary Material for this article can be found online at: https://www.frontiersin.org/articles/10.3389/fphar.2019.00217/full#supplementary-material
References
ATC/DDD Index (2018). Available online at: https://www.whocc.no/atc_ddd_index (Accessed June, 2018).
Blenkinsopp, A., Bond, C., and Raynor, D. K. (2012). Medication reviews. Br. J. Clin. Pharmacol. 74, 573–580. doi: 10.1111/j.1365-2125.2012.04331.x
Boye, N. D., van der Velde, N., de Vries, O. J., van Lieshout, E. M., Hartholt, K. A., Mattace-Raso, F. U., et al. (2017). Effectiveness of medication withdrawal in older fallers: results from the improving medication prescribing to reduce risk Of FALLs (IMPROveFALL) trial. Age Ageing 46, 142–146. doi: 10.1093/ageing/afw161
Bulajeva, A., Labberton, L., Leikola, S., Pohjanoksa-Mantyla, M., Geurts, M. M., de Gier, J. J., et al. (2014). Medication review practices in European countries. Res. Social Adm. Pharm. 10, 731–740. doi: 10.1016/j.sapharm.2014.02.005
Carey, I. M., Shah, S. M., Harris, T., DeWilde, S., and Cook, D. G. (2013). A new simple primary care morbidity score predicted mortality and better explains between practice variations than the Charlson index. J. Clin. Epidemiol. 66, 436–444. doi: 10.1016/j.jclinepi.2012.10.012
Cooksey, R. W. (1996). Judgement Analysis: Theory, Methods and Applications. San Diego, CA: Academic Press.
de Jong, M. R., Van der Elst, M., and Hartholt, K. A. (2013). Drug-related falls in older patients: implicated drugs, consequences, and possible prevention strategies. Ther. Adv. Drug Saf. 4, 147–154. doi: 10.1177/2042098613486829
Fau, L. J., and Koch, G. G. (1977). The measurement of observer agreement for categorical data. Biometrics 33, 159–174.
Fitch, K., Bernstein, S. J., Aguilar, M. D., Burnand, B., LaCalle, J. R., Lázaro, P., et al. (2001). The RAND/UCLA Appropriateness Method User's Manual. Santa Monica, CA: RAND Corporation.
Gelbard, R., Inaba, K., Okoye, O. T., Morrell, M., Saadi, Z., Lam, L., et al. (2014). Falls in the elderly: a modern look at an old problem. Am. J. Surg. 208, 249–253. doi: 10.1016/j.amjsurg.2013.12.034
Gillespie, L. D., Robertson, M. C., Gillespie, W. J., Sherrington, C., Gates, S., Clemson, L. M., et al. (2012). Interventions for preventing falls in older people living in the community. Cochrane Database Syst. Rev. 15:CD007146. doi: 10.1002/14651858.CD007146.pub3
Griese-Mammen, N., Hersberger, K. E., Messerli, M., Leikola, S., Horvat, N., van Mil, J. W. F., et al. (2018). PCNE definition of medication review: reaching agreement. Int J Clin Pharm. 40, 1199–1208. doi: 10.1007/s11096-018-0696-7
Hajjar, E. R., Hanlon, J. T., Sloane, R. J., Lindblad, C. I., Pieper, C. F., Ruby, C. M., et al. (2005). Unnecessary drug use in frail older people at hospital discharge. J. Am. Geriatr. Soc. 53, 1518–1523. doi: 10.1111/j.1532-5415.2005.53523.x
Hatah, E., Braund, R., Tordoff, J., and Duffull, S. B. (2014). A systematic review and meta-analysis of pharmacist-led fee-for-services medication review. Br. J. Clin. Pharmacol. 77, 102–115. doi: 10.1111/bcp.12140
Holland, R., Desborough, J., Goodyer, L., Hall, S., Wright, D., and Loke, Y. K. (2008). Does pharmacist-led medication review help to reduce hospital admissions and deaths in older people? a systematic review and meta-analysis. Br. J. Clin. Pharmacol. 65, 303–316. doi: 10.1111/j.1365-2125.2007.03071.x
Holland, R., Lenaghan, E., Harvey, I., Smith, R., Shepstone, L., Lipp, A., et al. (2005). Does home based medication review keep older people out of hospital? the HOMER randomised controlled trial. BMJ 330:293. doi: 10.1136/bmj.38338.674583.AE
Huiskes, V. J. B., Burger, D. M., van den Ende, C. H. M., and van den Bemt, B. J. F. (2017). Effectiveness of medication review: a systematic review and meta-analysis of randomized controlled trials. BMC Family Prac. 18:5. doi: 10.1186/s12875-016-0577-x
Hurmuz, M. Z. M., Janus, S. I. M., and van Manen, J. G. (2018). Changes in medicine prescription following a medication review in older high-risk patients with polypharmacy. Int. J. Clin. Pharm. 40, 480–487. doi: 10.1007/s11096-018-0602-3
Huyse, F. J., Fau, S. F., and de Jonge, P. (2007). Identifiers, or “red flags,” of complexity and need for integrated care. Med. Clin. 90, 703–712. doi: 10.1016/j.mcna.2006.05.003
Kaae, S., and Christensen, S. T. (2012). Exploring long term implementation of cognitive services in community pharmacies - a qualitative study. Pharm. Pract. 10, 151–158. doi: 10.4321/S1886-36552012000300005
Kaufmann, C. P., Stämpfli, D., Hersberger, K. E., and Lampert, M. L. (2015). Determination of risk factors for drug-related problems: a multidisciplinary triangulation process. BMJ Open 5:e006376. doi: 10.1136/bmjopen-2014-006376
Koster, E. S., Philbert, D., Noordam, M., Winters, N. A., Blom, L., and Bouvy, M. L. (2016). Availability of information on renal function in Dutch community pharmacies. Int. J. Clin. Pharm. 38, 797–801. doi: 10.1007/s11096-016-0332-3
Kwint, H. F., Faber, A., Gussekloo, J., and Bouvy, M. L. (2012). The contribution of patient interviews to the identification of drug-related problems in home medication review. J. Clin. Pharm. Ther. 12, 674–680. doi: 10.1111/j.1365-2710.2012.01370.x
Landelijke Huisartsen Vereniging (2016). Toezicht IGZ op Medicatiebeoordeling. Available online at: https://noordhollandnoord.lhv.nl/actueel/nieuws/toezicht-igz-op-medicatiebeoordeling (Accessed June, 2018).
Leendertse, A. J., Egberts, A. C., Stoker, L. J., and van den Bemt, P. M. (2008). HARM study group frequency of and risk factors for preventable medication-related hospital admissions in the Netherlands. Arch. Intern. Med. 168, 1890–1896. doi: 10.1001/archinternmed.2008.3
Lenaghan, E., Holland, R., and Brooks, A. (2007). Home-based medication review in a high risk elderly population in primary care–the POLYMED randomised controlled trial. Age Ageing 36, 292–297. doi: 10.1093/ageing/afm036
Morgan, B. L., Voorhis, V. W., and Carmen, R. (2007). Understanding power and rules of thumb for determining sample sizes. Tutor. Quant. Methods Psychol. 3, 43–50. doi: 10.20982/tqmp.03.2.p043
Nederlands Huisartsen Genootschap (2012). Multidisciplinary Guideline on Polypharmacy in Older Individuals. Available online at: https://www.nhg.org/sites/default/files/content/nhg_org/uploads/polyfarmacie_bij_ouderen.pdf (Accessed June, 2018).
Niquille, A., Lattmann, C., and Bugnon, O. (2010). Medication reviews led by community pharmacists in Switzerland: a qualitative survey to evaluate barriers and facilitators. Pharm. Pract. 8, 35–42. doi: 10.4321/S1886-36552010000100004
Onder, G., Petrovic, M., Tangiisuran, B., Meinardi, M. C., Markito-Notenboom, W. P., Somers, A., et al. (2010). Development and validation of a score to assess risk of adverse drug reactions among in-hospital patients 65 years or older: the GerontoNet ADR risk score. Arch. Intern. Med. 170, 1142–1148. doi: 10.1001/archinternmed.2010.153
Pharmaceutisch Weekblad (2015). SFK: Kwart 75-Plussers Gebruikt Zeven of Meer Geneesmiddelen. Available online at: https://www.sfk.nl/publicaties/PW/2015/kwart-75-plussers-gebruikt-zeven-of-meer-geneesmiddelen (Accessed March, 2019).
Pharmaceutisch Weekblad (2016). IGZ: Medicatiebeoordeling. Available online at: http://www.pw.nl/nieuws/2015/igz-medicatiebeoordeling (Accessed Junuary, 2018).
Poudel, A., Peel, N. M., Nissen, L., Mitchell, C., Gray, L. C., and Hubbard, R. E. (2014). Potentially inappropriate prescribing in older patients discharged from acute care hospitals to residential aged care facilities. Ann. Pharmacother. 48, 1425–1433. doi: 10.1177/1060028014548568
Ruggiero, C., Dell'Aquila, G., Gasperini, B., Onder, G., Lattanzio, F., Volpato, S., et al. (2010). Potentially inappropriate drug prescriptions and risk of hospitalization among older, Italian, nursing home residents: the ULISSE project. Drugs Aging 27, 747–758. doi: 10.2165/11538240-000000000-00000
Sikdar, K. C., Dowden, J., Alaghehbandan, R., MacDonald, D., Peter, P., and Gadag, V. (2012). Adverse drug reactions in elderly hospitalized patients: a 12-year population-based retrospective cohort study. Ann. Pharmacother. 46, 960–971. doi: 10.1345/aph.1Q529
van der Meer, H. G., Wouters, H., van Hulten, R., Pras, N., and Taxis, K. (2015). Decreasing the load? Is a multidisciplinary multistep medication review in older people an effective intervention to reduce a patient's drug burden index? Protocol of a randomised controlled trial. BMJ Open 5:e009213. doi: 10.1136/bmjopen-2015-009213
van Mil, J. W. F., de Boer, W. O., and Tromp, T. H. F. J. (2001). European barriers to the implementation of pharmaceutical care. Int. J. Pharm. Pract. 9, 163–168. doi: 10.1111/j.2042-7174.2001.tb01044.x
Wawruch, M., Fialova, D., Zikavska, M., Wsolova, L., Jezova, D., Kuzelova, M., et al. (2008). Factors influencing the use of potentially inappropriate medication in older patients in Slovakia. J. Clin. Pharm. Ther. 33, 381–392. doi: 10.1111/j.1365-2710.2008.00929.x
Willeboordse, F., Schellevis, F. G., Chau, S. H., Hugtenburg, J. G., and Elders, P. J. M. (2017). The effectiveness of optimised clinical medication reviews for geriatric patients: Opti-Med a cluster randomised controlled trial. Fam. Pract. 34, 437–445. doi: 10.1093/fampra/cmx007
Wimmer, B. C., Bell, J. S., Fastbom, J., Wiese, M. D., and Johnell, K. (2016). Medication regimen complexity and number of medications as factors associated with unplanned hospitalizations in older people: a population-based cohort study. J. Gerontol. A Biol. Sci. Med. Sci. 71, 831–837. doi: 10.1093/gerona/glv219
Keywords: medication reviews, polypharmacy, patient selection, aged, primary care
Citation: Crutzen S, Schuling J, Hugtenburg JG, Verduijn M, Teichert M, Taxis K and Denig P (2019) Development and Piloting of an Algorithm to Select Older Patients for Different Types of Medication Review. Front. Pharmacol. 10:217. doi: 10.3389/fphar.2019.00217
Received: 20 August 2018; Accepted: 22 February 2019;
Published: 19 March 2019.
Edited by:
Elena Ramírez, University Hospital La Paz, SpainReviewed by:
Allison Mari Dering-Anderson, University of Nebraska Medical Center, United StatesIsabelle Arnet, Universität Basel, Switzerland
Copyright © 2019 Crutzen, Schuling, Hugtenburg, Verduijn, Teichert, Taxis and Denig. This is an open-access article distributed under the terms of the Creative Commons Attribution License (CC BY). The use, distribution or reproduction in other forums is permitted, provided the original author(s) and the copyright owner(s) are credited and that the original publication in this journal is cited, in accordance with accepted academic practice. No use, distribution or reproduction is permitted which does not comply with these terms.
*Correspondence: Petra Denig, p.denig@umcg.nl