- 1CAS Key Laboratory of Synthetic Biology, CAS Center for Excellence in Molecular Plant Sciences, Institute of Plant Physiology and Ecology, Shanghai Institutes for Biological Sciences, Chinese Academy of Sciences, Shanghai, China
- 2University of Chinese Academy of Sciences, Beijing, China
- 3CAS-JIC Centre of Excellence in Plant and Microbial Sciences, Shanghai, China
Huperzine A (HupA) is a powerful and selective inhibitor of acetylcholinesterase. It has attracted widespread attention endangering the ultimate plant sources of Lycopodiaceae family. In this study, we used Huperzia serrata, extensively used in Traditional Chinese medicine (TCM), a slow growing vascular plant as the model plant of the Lycopodiaceae family to develop and validate the reference genes. We aim to use gene expression platform to understand the gene expression of different tissues and developmental stages of this medicinal herb. Eight candidate reference genes were selected based on RNA-seq data and evaluated with qRT-PCR. The expression of L/ODC and cytochrome P450s genes known for their involvement in lycopodium alkaloid biosynthesis, were also studied to validate the selected reference genes. The most stable genes were TBP, GAPDH, and their combination (TBP + GAPDH). We report for the first time the reference gene of H. serrata’s different tissues which would provide important insights into understanding their biological functions comparing other Lycopodiaceae plants and facilitate a good biopharming approach.
Introduction
The Lycopodiaceae family comprises three main genera, namely, Huperzia, Phlegmariurus, and monotypic Phylloglossum. The morphological variability between Phlegmariurus and Huperzia has presented a taxonomic challenge. Interestingly, they possess similar chemical diversity, especially lycopodium alkaloids, such as huperzine A (HupA), a highly potent, selective, and reversible inhibitor of AchE (Zhao and Tang, 2002), hence, a lead candidate for Alzheimer’s disease. HupA was initially isolated from the traditional Chinese medicine Qian Ceng Ta (Huperzia serrata). H. serrata is an economically important traditional Chinese herb that is used extensively for treatment of contusions, strains, swellings, schizophrenia, myasthenia gavis, and organophosphate poisoning since the Tang Dynasty (Ma et al., 2007; Xu et al., 2017). In the United States, H. serrata is marketed as a memory-enhancing dietary supplement (Ma and Gang, 2004). However, the wide clinical investigation and application of HupA are hampered by its poor supply from natural resource or uneconomical synthesis route (Benca, 2014). Moreover, extensive harvest for HupA has endangered H. serrata and other species in the Lycopodiaceae family. Synthetic biology approach offers an alternative potential source of HupA, but the inadequate understanding of its biosynthetic pathway restricts its production by metabolic engineering.
Current understanding of the biosynthesis of HupA and other lycopodium alkaloids originates from lysine and/or ornithine from feeding experiments and the pathway was initially proposed lysine/ornithine decarboxylase (L/ODC) as the first enzyme (Ma and Gang, 2004). Bunsupa and coauthors reported that L/ODC can catalyze the first step in the biosynthesis pathway of lysine-derived alkaloids, quinolizidine, and lycopodium alkaloids (Figure 1; Bunsupa et al., 2012, 2016). Furthermore, we cloned six HsL/ODC genes from H. serrata by degenerate method and characterized the function of one HsL/ODC in vitro and in vivo (Xu et al., 2017). A comprehensive relative quantitative metabolomic analysis of these alkaloids in different tissues of H. serrata was also performed by our group (Wu et al., 2018). However, the genes involved in skeleton formation and modification remain unclear (Figure 1, blue color; Yang et al., 2017).
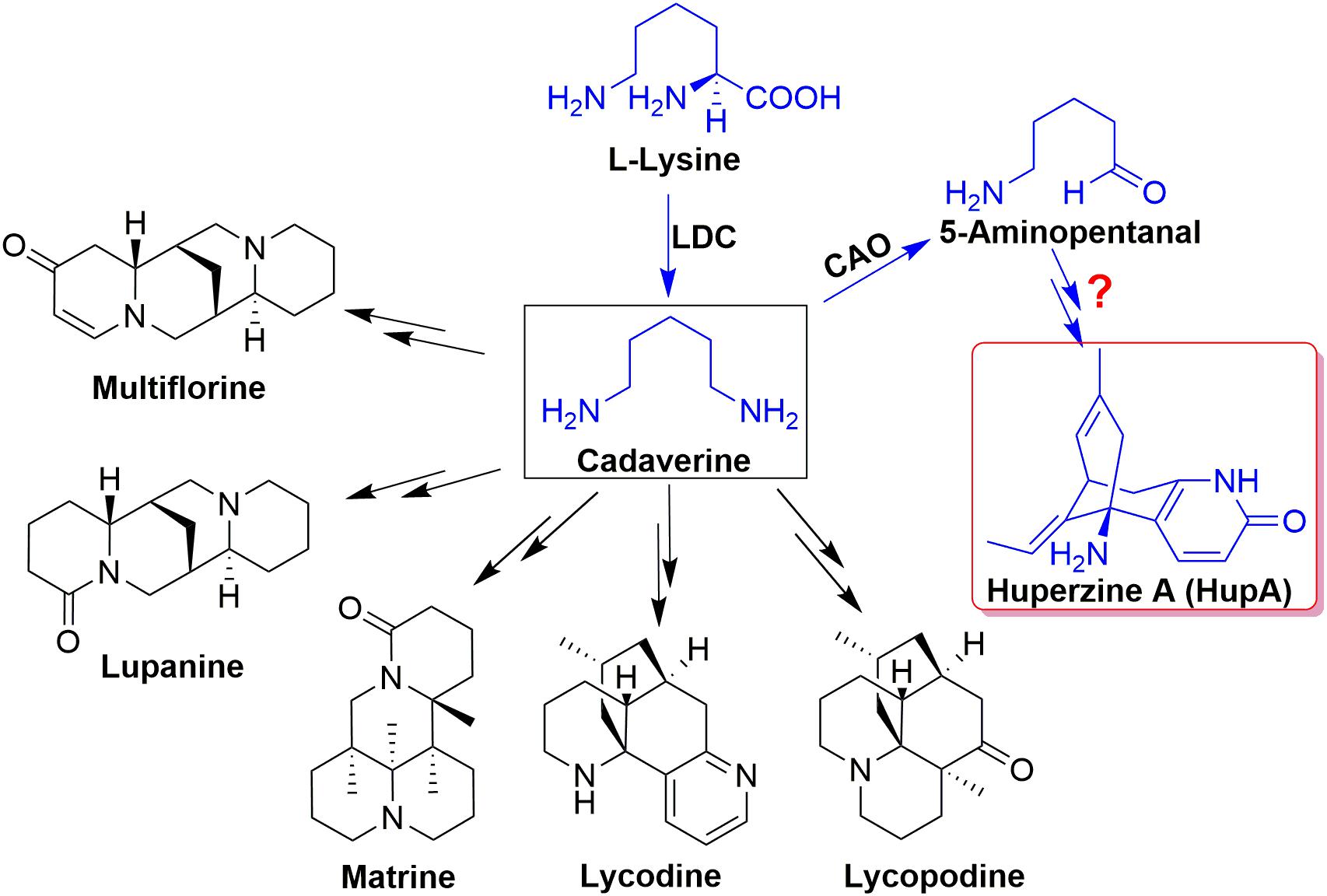
Figure 1. The proposed biosynthesis pathway of Lysine-derived alkaloids, quinolizidine, and lycopodium alkaloids.
Gene expression patterns in different plant tissues and growth developmental stages provide important insights into understanding their biological functions (Bustin, 2000; Vandesompele et al., 2002; Kozera and Rapacz, 2013). Transcriptome analysis and data mining have helped identify differentially expressed genes and measure the relative levels of their transcripts. Quantitative real-time PCR (qRT-PCR) provides a rapid, efficient, accurate, and reproducible method to present the mRNA transcription level in different samples or tissues and to validate data obtained from other methods (Vandesompele et al., 2002; Kozera and Rapacz, 2013; Zhang et al., 2017). The selection and validation of reference genes are the first steps in any qRT-PCR gene expression studies. The most commonly used genes for normalization of gene expression in different plant species include housekeeping genes, glyceraldehyde-3-phosphate dehydrogenase (GAPDH), β-actin, tubulin, and 18S (Radonic et al., 2004; Niu et al., 2015; Martins et al., 2017). However, the transcript expression level of such genes is not always stable, especially in samples of different developmental stages and tissues and those subjected to stresses, leading to erroneous results (Radonic et al., 2004; Petriccione et al., 2015; Pombo et al., 2017). Hence, screening and validating reference genes for normalization of the gene expression levels are pivotal.
In this study, actin, tubulin, 18S, TBP, GAPDH, HSP90, MUB, and SAM were selected as candidate reference genes based on global RNA-seq data. Their expression stabilities in the roots, stems, leaves, and sporangia of H. serrata in different developmental stages (2-, 3-, 4-, and 5-year old) were evaluated using geNorm, NormFinder, BestKeeper programs, comparative ΔCq method, and comprehensive stability rankings obtained from RefFinder. The expression of targeted genes, namely, L/ODC and cytochrome P450s, which are potentially involved in HupA biosynthesis, were used to validate the selected reference genes. This study is the first report to evaluate the expression stability of the reference genes in H. serrata. Results will be particularly useful in the selection of structural genes involved in HupA biosynthesis and research of Lycopodiaceae plants.
Materials and Methods
Plant Materials
Plants of different growth periods (2-, 3-, 4-, and 5-year old) were collected from Xiangxi, Hunan, China in January 2017, identified as H. serrata (Thunb.) Trevis1, and deposited at the Chinese herbarium with Barcode ID: 000196902. The plants were carefully rinsed in running tap water, and soil was removed by hand. Root, stem, leaf, and sporangia were kept in collection tubes immediately after being separated from the plant, immersed in liquid nitrogen, and stored at -80°C until further use.
RNA Isolation and cDNA Synthesis
Total RNA was extracted from four different tissues of H. serrata, namely, root, stem, leaf, and sporangia with TIANGEN RNAprep Pure Plant Kit [Tiangen Biotech (Beijing) Co., Ltd.] according to the kit instructions. DNase I was used to digest contaminated DNA. The purified total RNA was quantified using Nanodrop (Agilent 2100, Agilent Technologies, United States) and 1% agarose gel. cDNA was synthesized as previously reported (Yang et al., 2017).
Reference Gene Selection and Primer Design
Eight reference genes (actin, tubulin, 18S, TBP, GAPDH, HSP90, MUB, and SAM) were selected as potential candidates. All homologous in H. serrata were gathered by BLAST-search against the global RNA-seq data (Yang et al., 2017), and the candidate reference genes were selected with similar fragments per kilobase per million (FPKM) values determined in the four tissues (Figure 2). The primers of the candidate reference genes were designed, as listed in Table 1. The primer specificities were verified by the presence of a single DNA band with the expected size in 1.0% agarose gel electrophoresis and the presence of a single peak in qRT-PCR melting curve assays (Figure 4).
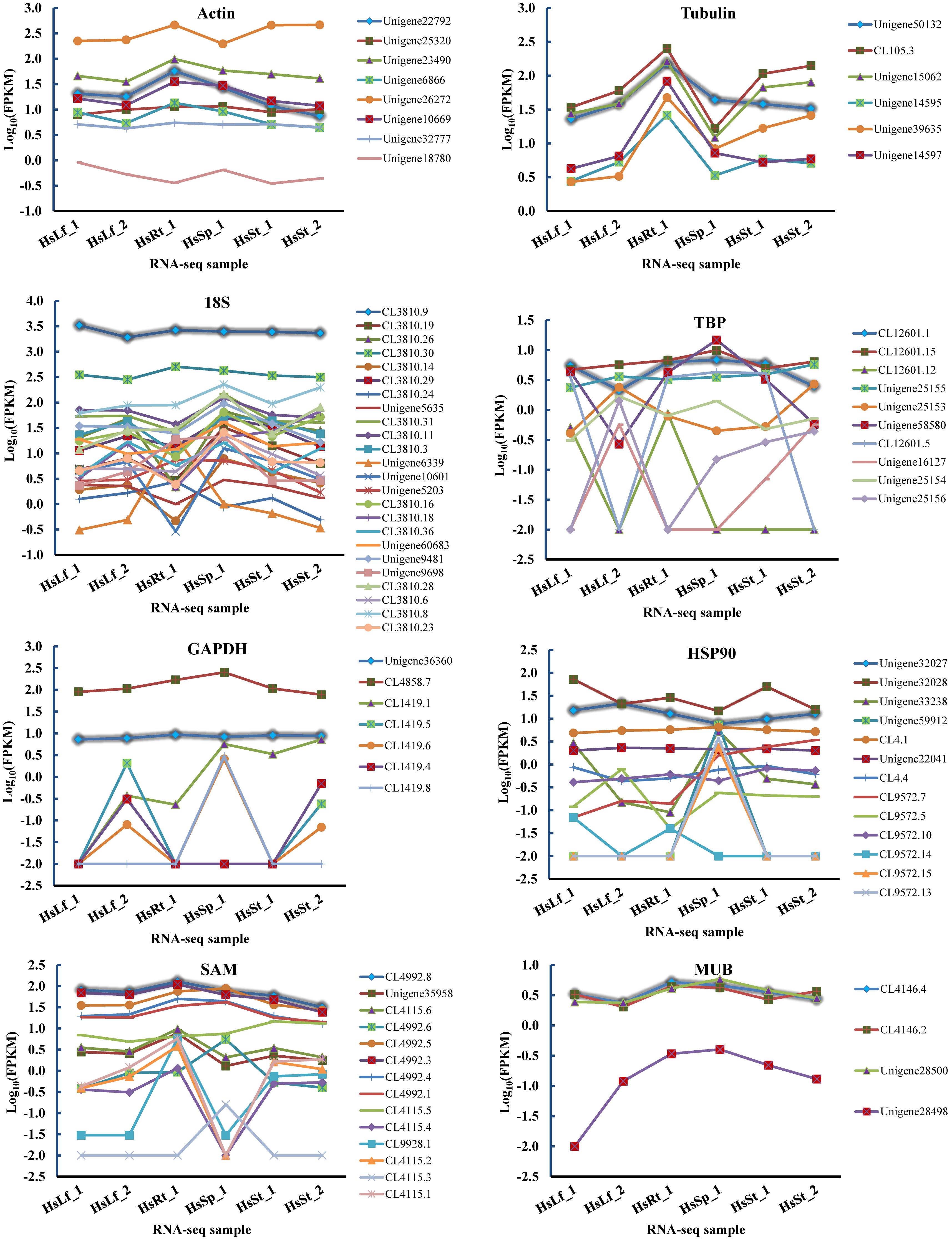
Figure 2. FPKMs of candidate reference genes’ homologs in six RNA-seq samples. The highlight lines indicate the selected candidate reference genes used in this study. Leaf: HsLf_1, HsLf_2; Root: HsRt_1; Sporangia: HsSp_1; Stem: HsSt_1, HsSt_2.
qRT-PCR Analysis
qRT-PCR amplification was performed as previously reported (Yang et al., 2017). Expression levels were recorded as cycle quantification (Cq). The PCR efficiency of each primer pair (E = 10-1/slope-1) was determined through slope of the amplification curve in the exponential phase, obtained by four fold series dilution of cDNA (Rutledge and Stewart, 2008).
Gene Expression Stability Analysis
The expression stability of the eight candidate reference genes across all tissues was evaluated with four algorithms, namely, geNorm (Vandesompele et al., 2002), NormFinder (Andersen et al., 2004), BestKeeper (Pfaffl et al., 2004), and ΔCq method. RefFinder (Xie et al., 2012), a web-based user-friendly comprehensive tool, was employed to generate the comprehensive ranking.
Validation of Identified Reference Genes
Previous studies have shown that L/ODC is the first key structure gene for precursor formation of HupA (Bunsupa et al., 2012, 2016; Xu et al., 2017). The homologues of the reported L/ODC (Unigene94988, Unigene94617) and four cytochrome P450 genes (CL9415.8, CL1143.2, Unigene1166, and Unigene25121) which were proposed to participate in the modification of HupA skeleton were also used to confirm the reliability of the selected reference genes by using the most two stable versus the least two stable genes.
Results
RNA-Seq-Assisted Selection of Candidate Reference Genes and Primer Design
The eight reference genes (actin, tubulin, 18S, TBP, GAPDH, HSP90, MUB, and SAM) with similar FPKMs in four tissues were gathered, and primers were designed (Table 1, Figure 3, and Supplementary Table S1).
Expression Levels and Variations of Candidate Reference Genes
The PCR product of candidate reference genes were verified by electrophoresis in 1.0 % agarose gel showed only a single band. The presence of a single peak in qRT-PCR melting curve analysis for each of the eight sets of primers indicated high specificity (Figure 4). qRT-PCR was performed to determine the expression levels of each candidate reference genes, and the Cq values showed differential transcript levels in the samples examined with low Cq values, which suggested transcript abundance. The mean Cq value of the eight candidate reference genes ranged from 13.57 to 31.72 (Figure 5 and Supplementary Table S1). In all sample set, the mean Cq values showed a minimum of 15.35 and a maximum of 25.23 for the highest and lowest expression levels for 18S and TBP, respectively. The coefficient of variation (CV) of the Cq values was also calculated to evaluate the expression levels of candidate reference genes of the four tissues, where low values represent low variability or maximum stability. The CV values of the eight reference genes among all samples ranged from 6.91 to 14.60%. TBP was the least variable reference gene with a CV of 6.91% among the eight candidate reference genes studied, and HSP90 was the most variable with a CV of 14.60%. The stability ranking of all candidate reference genes on the basis of CV values is as follows: (most stable to least stable): HSP90 < MUB < SAM < 18S < GAPDH < Actin < TBP (Figure 5).
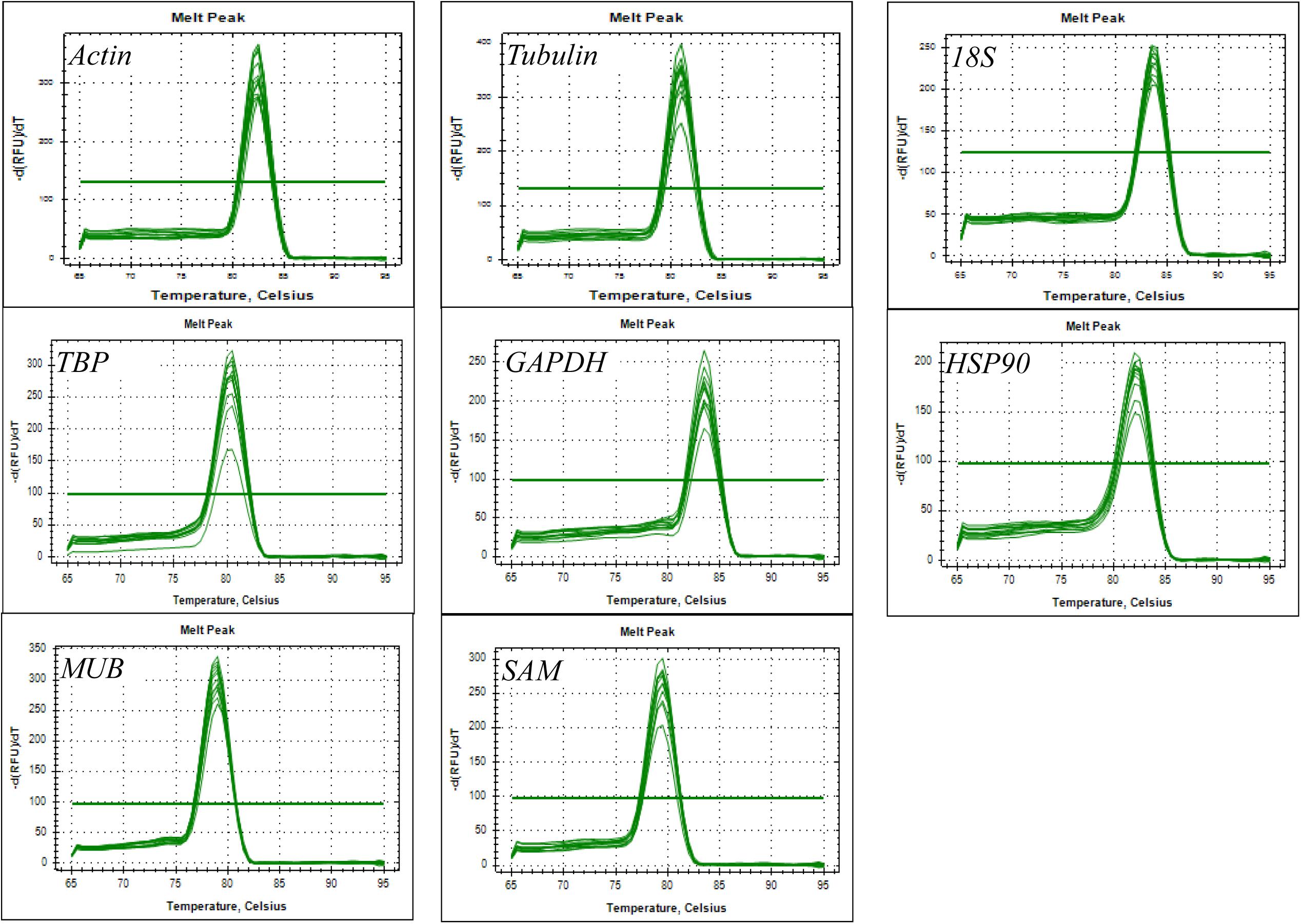
Figure 4. Amplification specificity of primers for qRT-PCR amplification. Melting curves of eight candidate reference genes.
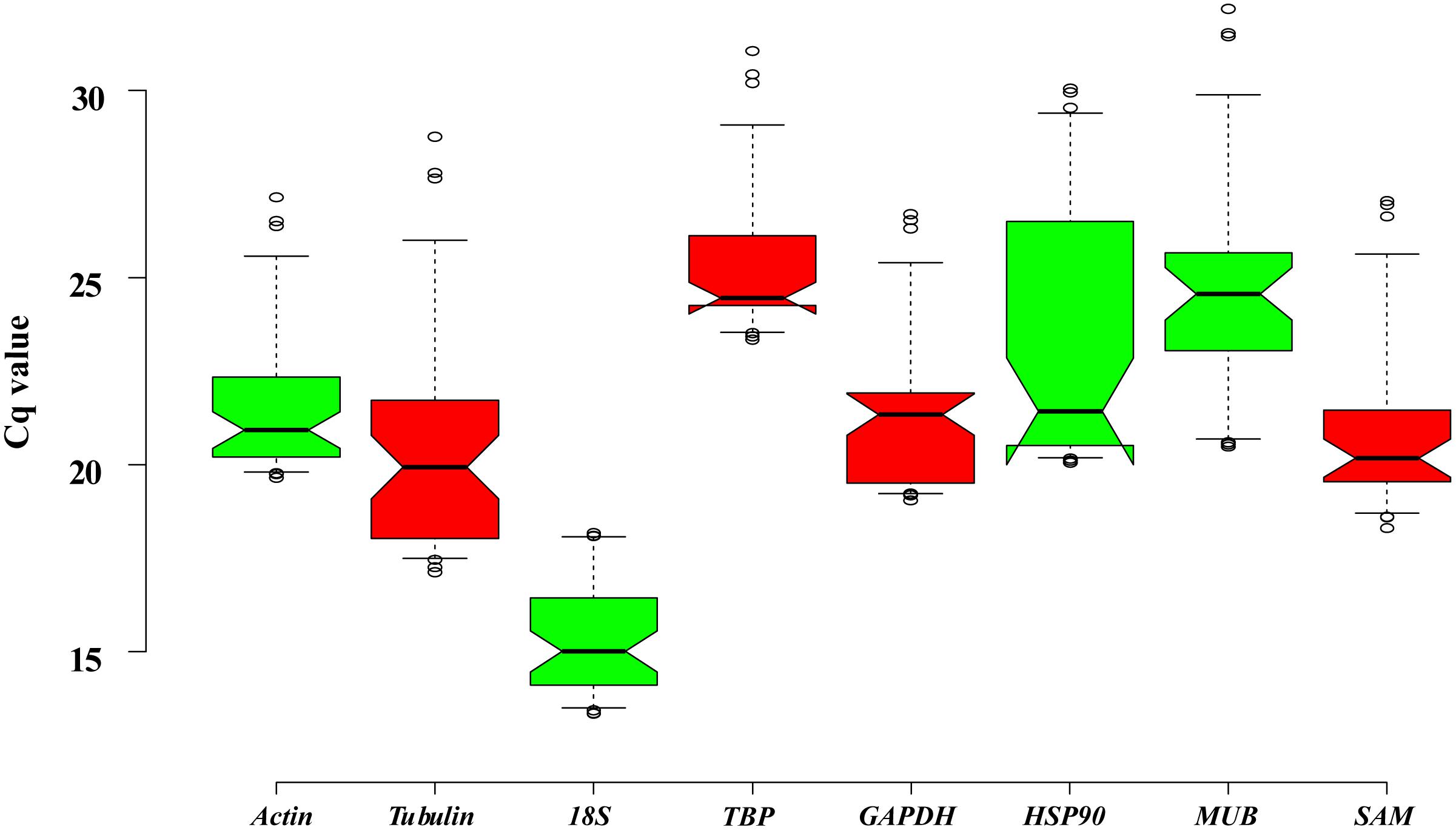
Figure 5. Expression levels of eight candidate reference genes across all experimental samples. Distribution of Cq values of candidate reference genes in all samples. Boxplots show the 25 and 75th percentiles, mean, and outliers.
Stability of the Reference Gene
Expression stabilities of the eight candidate reference genes were determined using ΔCq, geNorm, NormFinder, and BestKeeper, and their overall stabilities were ranked by RefFinder across all the tissue samples.
ΔCq Analysis
The eight candidate reference genes from the most to least stable expression, as calculated by the ΔCq method, are listed in Table 2. GAPDH and TBP were the most stable reference genes in the root and leaf. Actin and TBP were the most stable genes for the stem and sporangia, respectively. In sum, TBP, Actin, and GAPDH were the top three ideal reference genes.
geNorm Analysis
The stabilities of the eight candidate reference genes of H. serrata calculated using geNorm were ranked in the different tissues according to their M values, as shown in Figure 6. The lowest M value indicates the most stable reference gene, and the highest M value indicates the least stable one. Using the criteria of M < 0.5, TBP and GAPDH were stable reference genes in the four tissues of root, stem, leaf, and sporangia. When the stabilities from all the samples were combined, TBP and GAPDH were also determined to be the most stable reference genes. By contrast, HSP90 and MUB were two common unstable reference genes in all tissues and developmental stages.
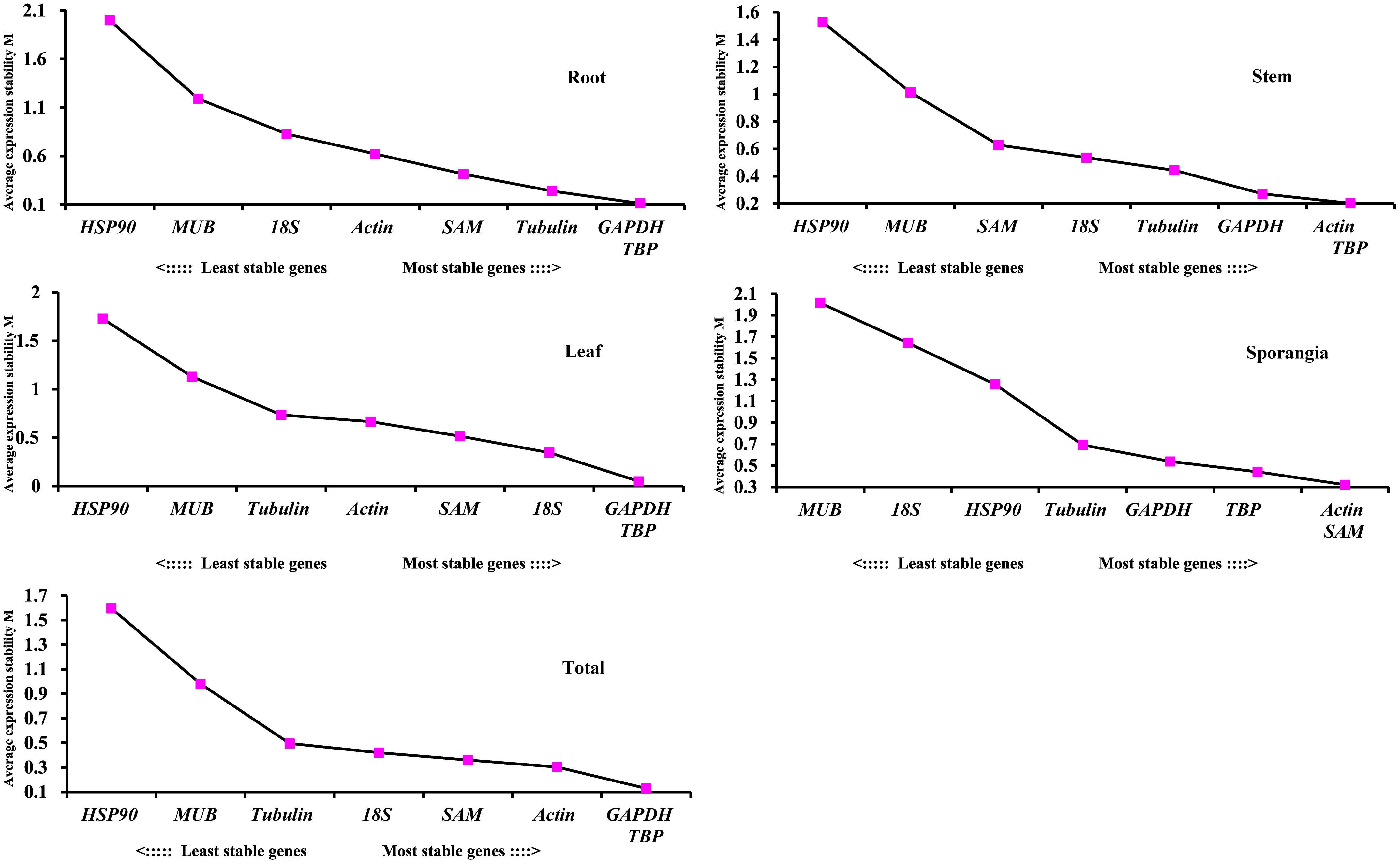
Figure 6. Average expression stability values (M) of the eight candidate reference genes assayed with geNorm software.
The pairwise variation (Vn/Vn+1) between two sequential normalization factors NFn and NFn+1 was calculated by the geNorm algorithm to determine the optimal number of reference genes for accurate normalization. A cutoff value of 0.15 is the recommended threshold, which indicates that an additional reference gene will inconsiderably contribute to the normalization. The V3/4 values in the root and stem were less than 0.15 (Figure 7), which suggested that the top two reference genes were sufficient for accurate normalization. For the leaf, V5/6 was 0.126, which indicated that the top five reference genes (TBP, GAPDH, 18S, SAM, and actin) were needed for accurate normalization. For the sporangia, V3/4 was 0.148, which showed that three reference genes (actin, SAM, and TBP) were required. The value V2/3 for total was 0.129, which indicated that the most stable genes, TBP and GAPDH, could be used as the reference genes for the normalization of gene expression in H. serrata.
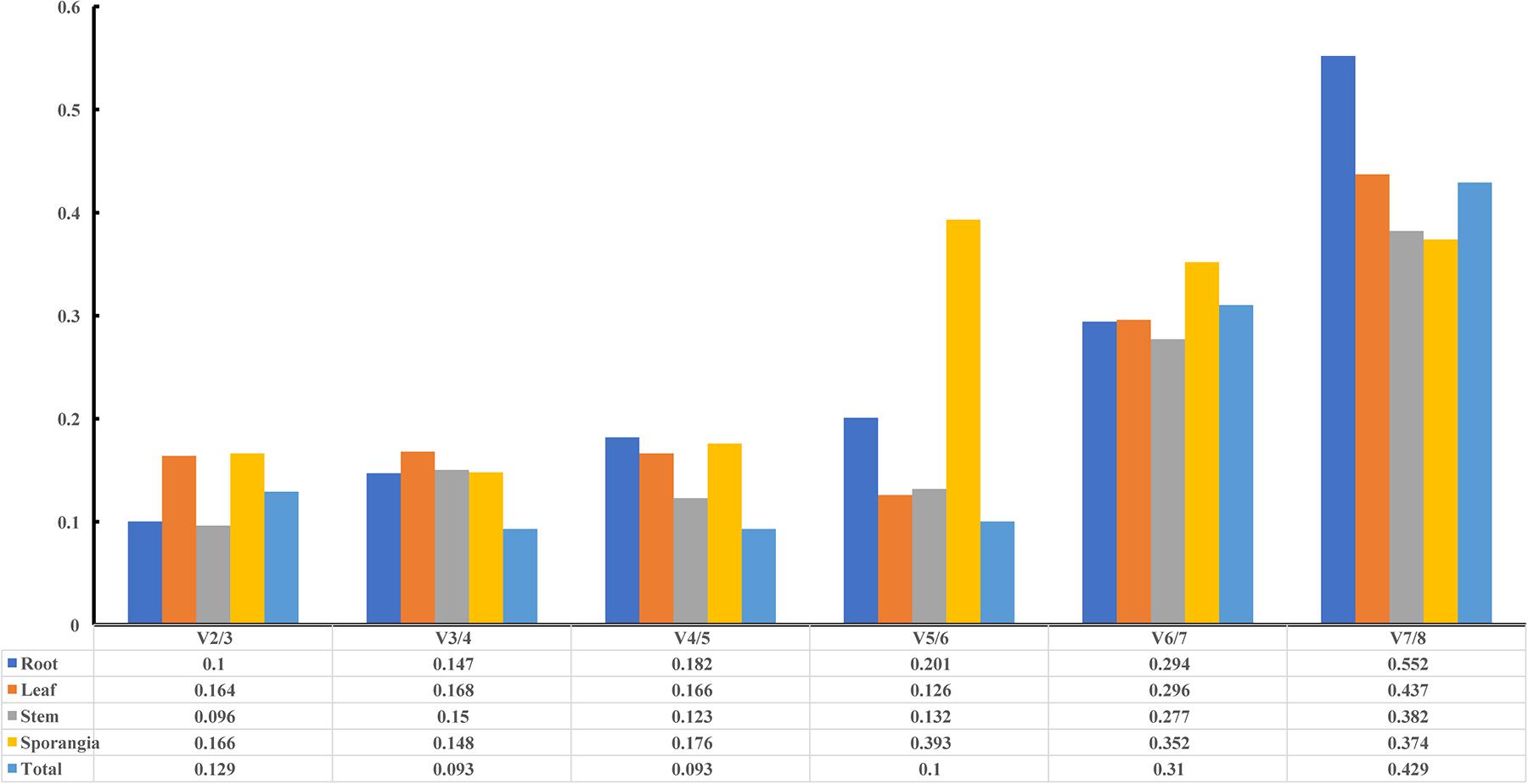
Figure 7. Pairwise variation (V) of candidate reference genes, as calculated by geNorm software. Vn/Vn+1 values were used to determine the optimal number of reference genes.
NormFinder Analysis
As shown in Table 3, TBP and GAPDH were the most stable genes (lowest stability value) in the root, leaf, and total subsets calculated using NormFinder. For the stem and sporangia samples, actin and TBP were the most stable reference genes. When all samples were taken to determine the stability of reference genes, the two most stable genes were TBP and GAPDH. SAM and actin also had low stability values, which indicated that the two reference genes were also suitable for qRT-PCR normalization, although not the most stable candidates.
BestKeeper Analysis
BestKeeper determined the stabilities of the candidate reference genes on the basis of their standard deviation (SD). Genes with SD>1 were considered unacceptable reference genes. The genes are listed from most to least stability in Table 4. Actin was the most stable gene in the root and total subsets, GAPDH was the most stable genes in the stem and leaf subsets, and 18S was the most stable gene in the sporangia. Only MUB and HSP90 were unstable genes.
RefFinder Analysis
The rankings of the four algorithms were integrated by RefFinder to acquire reliable results for the expression stabilities of the eight candidate reference genes of H. serrata, and the results are shown in Table 5. The expression of GAPDH was ranked as the most stable in the root and leaf, and the expression of actin was ranked as the most stable in the stem and sporangia. The expression of TBP was ranked the most stable in total. By contrast, MUB and HSP90 were two least stable reference genes almost in all tissues calculated by all five programs. Overall, the best reference genes for accurate transcript normalization in all of the samples were actin, GAPDH, and TBP, which had the lowest geometric mean of the ranking values.
Validation of the Identified Reference Genes
The expression levels of HupA biosynthesis-related genes, L/ODC (Unigene94617, Unigene94988), and cytochrome P450s (CL94158.8, CL11443.2, Unigene1166, and Unigene25121) were investigated using different reference genes in different tissues at different developmental stages to validate the selected candidate reference genes. Each of the two most stable reference genes (TBP and GAPDH), its combination (TBP + GAPDH), and the two least stable reference genes (HSP90 and MUB) were used as internal controls. When using TBP alone, GAPDH alone, MUB alone, and the combination of TBP + GAPDH for normalization, the expression patterns were similar in all six validated genes. However, when the least stable gene HSP90 was used for normalization, the expression patterns showed some differences (Figure 8). Thus, RNA Seq-assisted selection of candidate reference genes was helpful.
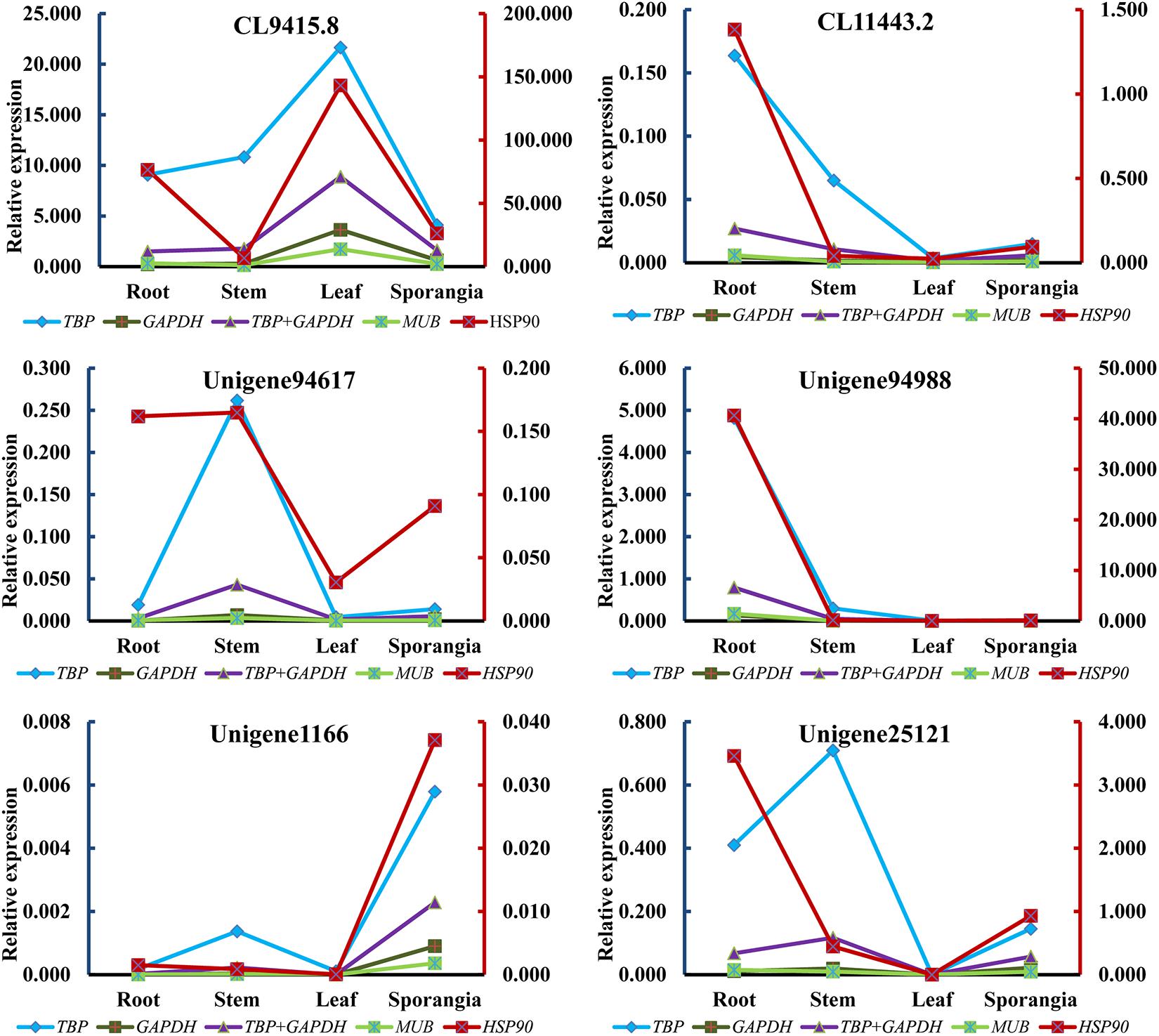
Figure 8. Relative expression patterns of CL94158.8, CL11443.2, Unigene94617, Unigene94988, Unigene1166, and Unigene25121.
Discussion
Standardization and quality assessment of traditional herbal formulations is of paramount importance in order to modernize. However, still major bottlenecks faced by the herbal industry is the unavailability of rigid quality control profiles, primarily because of the complexity and incomplete knowledge of the active medicinal compounds. H. serrata, a vulnerable group of slow-growing plant, extensively harvested by the traditional medicinal practitioners. It contains many active compounds, especially HupA whose contents differed significantly among the organs, varieties, age, and production areas of the herbal medicines (Ma et al., 2006). Hence, to address such variation in quality of medicinal material, studies has been directed towards understanding the molecular regulatory mechanisms of secondary metabolism through transcriptomics or functional genomics approaches.
Most of the modern plant research is often underpinned by the genetic approach creating transgenic lines to test the gene functions in planta. Inability to genetically transform any lycophytes species such as H. serrata has been challenging, and as such our understanding of Huperzia development lags significantly behind almost all other land plant lineages despite its traditional medicinal application. Recently, our group published the global RNA-seq of four different tissues which assisted us for the gene mining regards to the HupA biosynthesi (Yang et al., 2017). Elucidating the biosynthetic pathway is a prerequisite to heterologous production of targeted metabolites limiting the overexploitation of the natural habitat. To our knowledge no suitable reference gene for this plant is available. It is important to select a suitable reference gene to study the different expression patterns in different varieties and different tissues in medicinal plants. Here, we report the use of eight genes (actin, tubulin, 18S, TBP, GAPDH, HSP90, MUB, and SAM), to select and validate the suitable reference genes for the qRT-PCR normalization in different tissues and developmental growth stages.
qRT-PCR is one of the most commonly used technologies for transcript expression analysis owing to its sensitivity and reproducibility (Derveaux et al., 2010). Coexpression analysis is a useful method to screen the candidate structure genes involved in specialized metabolite biosynthesis (Saito et al., 2008). Normalization with stable reference genes is critical for obtaining accurate results from qRT-PCR data. Differential and coexpression analyses of the structural genes derived from qRT-PCR have been successful to screen UDP-dependent glucuronosyltransferase, which can catalyze continuous two-step glucuronosylation of glycyrrhetinic acid to yield (Xu et al., 2016). Hence, differential analysis coupled with coexpression analysis will be a useful method to screen the specific genes in the plants without genome information.
For H. serrata, the global RNA-seq data from four different tissues have been published, which can be directly used for differential and coexpression analyses. However, the suitable reference gene for this plant is still not selected, which may result in different expression patterns in different tissues or treatments. Here, eight reported reference genes (actin, tubulin, 18S, TBP, GAPDH, HSP90, MUB, and SAM) were selected and validated to discover the suitable reference genes for the qRT-PCR normalization in different tissues.
In the current study, four housekeeping genes (GAPDH, actin, tubulin, and 18S) and other four genes (TBP, SAM, HSP90, and MUB) were used as query genes for the blast against the RNA-seq data (Yang et al., 2017) to find the homologous genes (Stanton et al., 2017). Genes with similar expression levels in four different tissues were selected as candidates as previously reported (Tan et al., 2015). In this study, the traditional reference genes, GAPDH, actin, tubulin, and 18S, had good performances in CV values in qRT-PCR Cq values (CV < 10%), in line with the previous reports (Shivhare and Lata, 2016; Martins et al., 2017). The four most extensively used programs (ΔCq, geNorm, NormFinder, and BestKeeper) were used in this study for analyzing the stabilities of candidate reference genes to avoid selection of coregulated genes. The four programs showed a few differences in results; TBP and GAPDH were the most two stable reference genes, MUB and HSP90 were the least stable reference genes, and others were midstable candidates with different rankings calculated by different programs.
Although TBP was the most stable candidate for all samples in our study, its expression level was very low, which was also observed in equine milk somatic cells and in Aedes aegypti (Cieslak et al., 2015; Dzaki et al., 2017). This low level was due to that the Cq values in qRT-PCR assays varied from 21.34 to 31.05 in all experiments with the five dilution cDNAs, which indicated that the TBP is a new plant species-dependent reference gene, hence suggesting a proper validation in each case. While, GAPDH exhibited good performance on qRT-PCR normalization in different tissues of plants of different developmental stages, as calculated by most of the programs. Hence, GAPDH alone is suitable for qRT-PCR normalization as a reference gene under different tissues. The few differences in reference genes showed in different programs were also in agreement with earlier studies (Jain et al., 2006; Cruz et al., 2009; Jain, 2009; Qi et al., 2016). The different rankings of the reference genes showed in different programs were also observed in chrysanthemum (Gu et al., 2011; Wang et al., 2015); thus, all these programs must be combined to evaluate the candidates for each species.
We further performed RT-qPCR experiments to investigate the expression levels of L/ODC genes, which were previously characterized in H. serrata, Lycopodium clavatum, and Leguminosae (Bunsupa et al., 2012; Bunsupa et al., 2016; Xu et al., 2017) by using the two most stable reference genes (TBP and GAPDH) and the two least stable reference genes (MUB and HSP90), to evaluate the eight selected reference genes. According to the pairwise analysis by geNorm software, two reference genes were sufficient for the normalization; thus, the combination of TBP and GAPDH was also used to calculate the expression level of targeted genes. Regardless of which reference gene was used, the expression patterns of L/ODC (Unigene94988) were the same. To further validate, Unigene94617, a homologous gene of Unigene94988, and four cytochrome P450 genes (CL9415.8, CL1143.2, Unigene1166, and Unigene25121) proposed to participate in HupA biosynthesis (Yang et al., 2017) were employed for the normalization. All reference genes, with exception of HSP90, acquired a similar expression pattern for all targeted genes. Hence, only HSP90 was unsuitable for qRT-PCR normalization in all tissues of H. serrata, which suggested that RNA-seq-assisted selection was a useful method for selecting suitable reference genes. Previous studies in Arabidopsis thaliana, Coffeea arabica, Gossypium hirsutum, and Chrysanthemum showed that the novel reference genes exhibited better performance than traditional reference genes (Czechowski et al., 2005; Cruz et al., 2009; Artico et al., 2010; Qi et al., 2016). Taken all together, although we observed some inconsistency on the expression patterns of the some genes in HupA biosynthesis between RNA-seq and qRT-PCR, this might be due to the plant growth condition differences (season and climate) when we collected (Supplementary Table S1). The major reason for this is likely due to the seasonal and climatic factors or growth as this plant takes years to grow (Ma et al., 2006). Similarly, inconsistency was also observed previous reports. In many cases, the gene expressions quantified with different methods were dramatically different (Wang et al., 2006; Marioni et al., 2008; Qin et al., 2013; Rajkumar et al., 2015; Dapas et al., 2017). Due to the lack of successful in vitro propagation approach of Lycopodiaceae family, its important to design such functional genomics study from the control climatic conditions and/or established in vitro platform. Our lab is currently exploring the approach of in vitro propagation of endangered species of Lycopodiaceae family. This study state possible use of housekeeping genes as a stable candidate for qRT-PCR normalization of plants belonging to Lycopodiaceaea family especially H. serrata.
Conclusion
In this study, we proposed H. serrata as a model plant for functional genomics study in the Lycopodiaceae family. The qRT-PCR reference gene normalization in tissues of H. serrata showed that TBP and GAPDH were the two most suitable reference genes. The combination of the two genes as reference genes was accurate for qRT-PCR normalization, as performed in different tissues of H. serrata according to the pairwise variation analysis by geNorm program. The reference genes identified and validated here through RNA-seq data for qRT-PCR normalization will facilitate the establishment of standardized qRT-PCR program for other genetically close plants.
Author Contributions
YX conceived the research. YX and SW designed the experiments. WY, SW, and MY, performed the experiments. MY and SW analyzed the data, wrote the manuscript, and coordinated its revision. YX and AJ revised the manuscript. All authors provided helpful discussions and approved the final version.
Funding
This work was financially supported by Chinese Academy of Sciences (CAS) (Grant XDB27020203, 153D31KYSB20170121, and 153D31KYSB20160074) and CAS-JIC center of Excellence in Plant and Microbial Sciences (CEPAMS) funding.
Conflict of Interest Statement
The authors declare that the research was conducted in the absence of any commercial or financial relationships that could be construed as a potential conflict of interest.
Supplementary Material
The Supplementary Material for this article can be found online at: https://www.frontiersin.org/articles/10.3389/fphar.2019.00044/full#supplementary-material
TABLE S1 | The consistency of target genes expression and the expression data of qRT-PCR and RNA-seq.
Footnotes
References
Andersen, C. L., Jensen, J. L., and Ørntoft, T. F. (2004). Normalization of real-time quantitative reverse transcription-PCR data: a model-based variance estimation approach to identify genes suited for normalization, applied to bladder and colon cancer data sets. Cancer Res. 64, 5245–5250. doi: 10.1158/0008-5472.CAN-04-0496
Artico, S., Nardeli, S. M., Brilhante, O., Grossi-De-Sa, M. F., and Alves-Ferreira, M. (2010). Identification and evaluation of new reference genes in Gossypium hirsutum for accurate normalization of real-time quantitative RT-PCR data. BMC Plant Biol. 10:49. doi: 10.1186/1471-2229-10-49
Benca, J. P. (2014). Cultivation techniques for terrestrial clubmosses (Lycopodiaceae): conservation, research, and horticultural opportunities for an early-diverging plant lineage. Am. Fern J. 104, 25–48. doi: 10.1640/0002-8444-104.2.25
Bunsupa, S., Hanada, K., Maruyama, A., Aoyagi, K., Komatsu, K., Ueno, H., et al. (2016). Molecular evolution and functional characterization of a bifunctional decarboxylase involved in Lycopodium alkaloid biosynthesis. Plant Physiol. 171, 2432–2444. doi: 10.1104/pp.16.00639
Bunsupa, S., Katayama, K., Ikeura, E., Oikawa, A., Toyooka, K., Saito, K., et al. (2012). Lysine decarboxylase catalyzes the first step of quinolizidine alkaloid biosynthesis and coevolved with alkaloid production in leguminosae. Plant Cell 24, 1202–1216. doi: 10.1105/tpc.112.095885
Bustin, S. A. (2000). Absolute quantification of mRNA using real-time reverse transcription polymerase chain reaction assays. J. Mol. Endocrinol. 25, 169–193. doi: 10.1677/jme.0.0250169
Cieslak, J., Mackowski, M., Czyzak-Runowska, G., Wojtowski, J., Puppel, K., Kuczynska, B., et al. (2015). Screening for the most suitable reference genes for gene expression studies in equine milk somatic cells. PLoS One 10:e0139688. doi: 10.1371/journal.pone.0139688
Cruz, F., Kalaoun, S., Nobile, P., Colombo, C., Almeida, J., Barros, L. M. G., et al. (2009). Evaluation of coffee reference genes for relative expression studies by quantitative real-time RT-PCR. Mol. Breed. 23, 607–616. doi: 10.1007/s11032-009-9259-x
Czechowski, T., Stitt, M., Altmann, T., Udvardi, M. K., and Scheible, W. R. (2005). Genome-wide identification and testing of superior reference genes for transcript normalization in Arabidopsis. Plant Physiol. 139, 5–17. doi: 10.1104/pp.105.063743
Dapas, M., Kandpal, M., Bi, Y. T., and Davuluri, R. V. (2017). Comparative evaluation of isoform-level gene expression estimation algorithms for RNA-seq and exon-array platforms. Brief. Bioinform. 18, 260–269. doi: 10.1093/bib/bbw016
Derveaux, S., Vandesompele, J., and Hellemans, J. (2010). How to do successful gene expression analysis using real-time PCR. Methods 50, 227–230. doi: 10.1016/j.ymeth.2009.11.001
Dzaki, N., Ramli, K. N., Azlan, A., Ishak, I. H., and Azzam, G. (2017). Evaluation of reference genes at different developmental stages for quantitative real-time PCR in Aedes aegypti. Sci. Rep. 7:43618. doi: 10.1038/srep43618
Gu, C., Chen, S., Liu, Z., Shan, H., Luo, H., Guan, Z., et al. (2011). Reference gene selection for quantitative real-time PCR in Chrysanthemum subjected to biotic and abiotic stress. Mol. Biotechnol. 49, 192–197. doi: 10.1007/s12033-011-9394-6
Jain, M. (2009). Genome-wide identification of novel internal control genes for normalization of gene expression during various stages of development in rice. Plant Sci. 176, 702–706. doi: 10.1016/j.plantsci.2009.02.001
Jain, M., Nijhawan, A., Tyagi, A. K., and Khurana, J. P. (2006). Validation of housekeeping genes as internal control for studying gene expression in rice by quantitative real-time PCR. Biochem. Biophys. Res. Commun. 345, 646–651. doi: 10.1016/j.bbrc.2006.04.140
Kozera, B., and Rapacz, M. (2013). Reference genes in real-time PCR. J. Appl. Genet. 54, 391–406. doi: 10.1007/s13353-013-0173-x
Ma, X., and Gang, D. R. (2004). The Lycopodium alkaloids. Nat. Prod. Rep. 21, 752–772. doi: 10.1039/b409720n
Ma, X., Tan, C., Zhu, D., and Gang, D. R. (2006). A survey of potential huperzine A natural resources in China: the Huperziaceae. J. Ethnopharmacol. 104, 54–67. doi: 10.1016/j.jep.2005.08.042
Ma, X., Tan, C., Zhu, D., Gang, D. R., and Xiao, P. (2007). Huperzine A from Huperzia species–an ethnopharmacolgical review. J. Ethnopharmacol. 113, 15–34. doi: 10.1016/j.jep.2007.05.030
Marioni, J. C., Mason, C. E., Mane, S. M., Stephens, M., and Gilad, Y. (2008). RNA-seq: an assessment of technical reproducibility and comparison with gene expression arrays. Genome Res. 18, 1509–1517. doi: 10.1101/gr.079558.108
Martins, M. Q., Fortunato, A. S., Rodrigues, W. P., Partelli, F. L., Campostrini, E., Lidon, F. C., et al. (2017). Selection and validation of reference genes for accurate RT-qPCR data normalization in Coffea spp. under a climate changes context of interacting elevated [CO2] and temperature. Front. Plant Sci. 8:307. doi: 10.3389/fpls.2017.00307
Niu, X., Qi, J., Zhang, G., Xu, J., Tao, A., Fang, P., et al. (2015). Selection of reliable reference genes for quantitative real-time PCR gene expression analysis in Jute (Corchorus capsularis) under stress treatments. Front. Plant Sci. 6:848. doi: 10.3389/fpls.2015.00848
Petriccione, M., Mastrobuoni, F., Zampella, L., and Scortichini, M. (2015). Reference gene selection for normalization of RT-qPCR gene expression data from Actinidia deliciosa leaves infected with Pseudomonas syringae pv. actinidiae. Sci. Rep. 5:16961. doi: 10.1038/srep16961
Pfaffl, M. W., Tichopad, A., Prgomet, C., and Neuvians, T. P. (2004). Determination of stable housekeeping genes, differentially regulated target genes and sample integrity: BestKeeper – Excel-based tool using pair-wise correlations. Biotechnol. Lett. 26, 509–515. doi: 10.1023/B:BILE.0000019559.84305.47
Pombo, M. A., Zheng, Y., Fei, Z., Martin, G. B., and Rosli, H. G. (2017). Use of RNA-seq data to identify and validate RT-qPCR reference genes for studying the tomato-Pseudomonas pathosystem. Sci. Rep. 7:44905. doi: 10.1038/srep44905
Qi, S., Yang, L., Wen, X., Hong, Y., Song, X., Zhang, M., et al. (2016). Reference gene selection for RT-qPCR analysis of flower development in Chrysanthemum morifolium and Chrysanthemum lavandulifolium. Front. Plant Sci. 7:287. doi: 10.3389/fpls.2016.00287
Qin, J., Scheuring, C. F., Wei, G., Zhi, H., Zhang, M. P., Huang, J. J., et al. (2013). Identification and characterization of a repertoire of genes differentially expressed in developing top ear shoots between a superior hybrid and its parental inbreds in Zea mays L. Mol. Genet. Genomics 288, 691–705. doi: 10.1007/s00438-013-0781-5
Radonic, A., Thulke, S., Mackay, I. M., Landt, O., Siegert, W., and Nitsche, A. (2004). Guideline to reference gene selection for quantitative real-time PCR. Biochem. Biophys. Res. Commun. 313, 856–862. doi: 10.1016/j.bbrc.2003.11.177
Rajkumar, A. P., Qvist, P., Lazarus, R., Lescai, F., Ju, J., Nyegaard, M., et al. (2015). Experimental validation of methods for differential gene expression analysis and sample pooling in RNA-seq. BMC Genomics 16:548. doi: 10.1186/s12864-015-1767-y
Rutledge, R. G., and Stewart, D. (2008). Critical evaluation of methods used to determine amplification efficiency refutes the exponential character of real-time PCR. BMC Mol. Biol. 9:96. doi: 10.1186/1471-2199-9-96
Saito, K., Hirai, M. Y., and Yonekura-Sakakibara, K. (2008). Decoding genes with coexpression networks and metabolomics–‘majority report by precogs’. Trends Plant Sci. 13, 36–43. doi: 10.1016/j.tplants.2007.10.006
Shivhare, R., and Lata, C. (2016). Selection of suitable reference genes for assessing gene expression in pearl millet under different abiotic stresses and their combinations. Sci. Rep. 6:23036. doi: 10.1038/srep23036
Stanton, K. A., Edger, P. P., Puzey, J. R., Kinser, T., Cheng, P., Vernon, D. M., et al. (2017). A whole-transcriptome approach to evaluating reference genes for quantitative gene expression studies: a case study in mimulus. G3 7, 1085–1095. doi: 10.1534/g3.116.038075
Tan, Q. Q., Zhu, L., Li, Y., Liu, W., Ma, W. H., Lei, C. L., et al. (2015). A de novo transcriptome and valid reference genes for quantitative real-time PCR in Colaphellus bowringi. PLoS One 10:e0118693. doi: 10.1371/journal.pone.0118693
Vandesompele, J., De Preter, K., Pattyn, F., Poppe, B., Van Roy, N., De Paepe, A., et al. (2002). Accurate normalization of real-time quantitative RT-PCR data by geometric averaging of multiple internal control genes. Genome Biol. 3:RESEARCH0034. doi: 10.1186/gb-2002-3-7-research0034
Wang, H., Chen, S., Jiang, J., Zhang, F., and Chen, F. (2015). Reference gene selection for cross-species and cross-ploidy level comparisons in Chrysanthemum spp. Sci. Rep. 5:8094. doi: 10.1038/srep08094
Wang, Y. L., Barbacioru, C., Hyland, F., Xiao, W. M., Hunkapiller, K. L., Blake, J., et al. (2006). Large scale real-time PCR validation on gene expression measurements from two commercial long-oligonucleotide microarrays. BMC Genomics 7:59.
Wu, S., Fan, Z., and Xiao, Y. (2018). Comprehensive relative quantitative metabolomics analysis of Lycopodium alkaloids in different tissues of Huperzia serrata. Synth. Syst. Biotechnol. 3, 44–55. doi: 10.1016/j.synbio.2017.12.003
Xie, F., Xiao, P., Chen, D., Xu, L., and Zhang, B. (2012). miRDeepFinder: a miRNA analysis tool for deep sequencing of plant small RNAs. Plant Mol. Biol. 80, 75–84. doi: 10.1007/s11103-012-9885-2
Xu, B., Lei, L., Zhu, X., Zhou, Y., and Xiao, Y. (2017). Identification and characterization of L-lysine decarboxylase from Huperzia serrata and its role in the metabolic pathway of Lycopodium alkaloid. Phytochemistry 136, 23–30. doi: 10.1016/j.phytochem.2016.12.022
Xu, G., Cai, W., Gao, W., and Liu, C. (2016). A novel glucuronosyltransferase has an unprecedented ability to catalyse continuous two-step glucuronosylation of glycyrrhetinic acid to yield glycyrrhizin. New Phytol. 212, 123–135. doi: 10.1111/nph.14039
Yang, M., You, W., Wu, S., Fan, Z., Xu, B., Zhu, M., et al. (2017). Global transcriptome analysis of Huperzia serrata and identification of critical genes involved in the biosynthesis of huperzine A. BMC Genomics 18:245. doi: 10.1186/s12864-017-3615-8
Zhang, Y. X., Han, X. J., Chen, S. S., Zheng, L., He, X. L., Liu, M. Y., et al. (2017). Selection of suitable reference genes for quantitative real-time PCR gene expression analysis in Salix matsudana under different abiotic stresses. Sci. Rep. 7:40290. doi: 10.1038/srep40290
Keywords: Huperzia serrata, lycopodium alkaloids, reference gene, real-time quantitative PCR, gene expression
Citation: Yang M, Wu S, You W, Jaisi A and Xiao Y (2019) Selection of Reference Genes for Expression Analysis in Chinese Medicinal Herb Huperzia serrata. Front. Pharmacol. 10:44. doi: 10.3389/fphar.2019.00044
Received: 28 August 2018; Accepted: 14 January 2019;
Published: 01 February 2019.
Edited by:
Jiang Xu, China Academy of Chinese Medical Sciences, ChinaReviewed by:
Mingqian Li, Zhejiang Institute of Traditional Chinese MedicineShu Shaohua, Huazhong Agricultural University, China
Copyright © 2019 Yang, Wu, You, Jaisi and Xiao. This is an open-access article distributed under the terms of the Creative Commons Attribution License (CC BY). The use, distribution or reproduction in other forums is permitted, provided the original author(s) and the copyright owner(s) are credited and that the original publication in this journal is cited, in accordance with accepted academic practice. No use, distribution or reproduction is permitted which does not comply with these terms.
*Correspondence: Youli Xiao, eWx4aWFvQHNpYnMuYWMuY24=
†These authors have contributed equally to this work