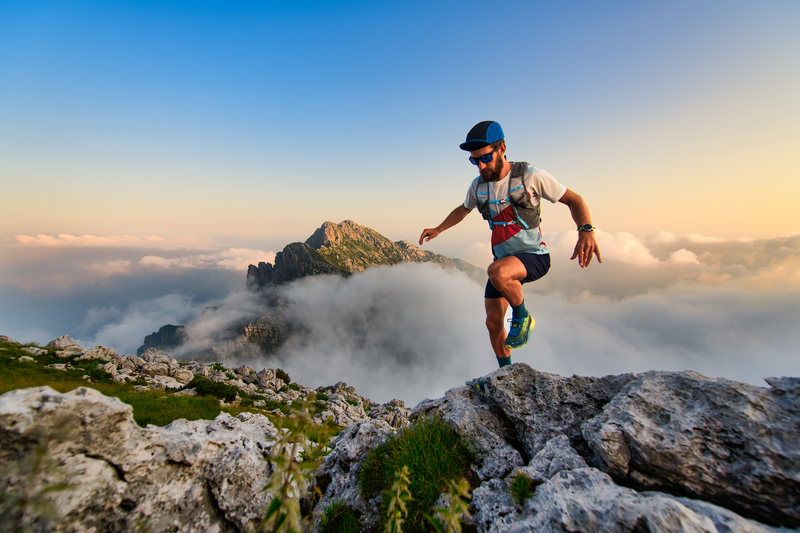
94% of researchers rate our articles as excellent or good
Learn more about the work of our research integrity team to safeguard the quality of each article we publish.
Find out more
ORIGINAL RESEARCH article
Front. Pharmacol. , 19 June 2018
Sec. Ethnopharmacology
Volume 9 - 2018 | https://doi.org/10.3389/fphar.2018.00651
This article is part of the Research Topic Frailty and Herbal Medicines- From Molecular Mechanisms to Clinical Efficacy View all 31 articles
Background: Li-Ru-Kang (LRK) has been used in the treatment of hyperplasia of mammary glands (HMG) for several decades and can effectively improve clinical symptoms. This study aims to investigate the mechanism by which LRK intervenes in HMG based on an integrated approach that combines metabolomics and network pharmacology analyses.
Methods: The effects of LRK on HMG induced by estrogen-progesterone in rats were evaluated by analyzing the morphological and pathological characteristics of breast tissues. Moreover, UPLC-QTOF/MS was performed to explore specific metabolites potentially affecting the pathological process of HMG and the effects of LRK. Pathway analysis was conducted with a combination of metabolomics and network pharmacology analyses to illustrate the pathways and network of LRK-treated HMG.
Results: Li-Ru-Kang significantly improved the morphological and pathological characteristics of breast tissues. Metabolomics analyses showed that the therapeutic effect of LRK was mainly associated with the regulation of 10 metabolites, including prostaglandin E2, phosphatidylcholine, leukotriene B4, and phosphatidylserine. Pathway analysis indicated that the metabolites were related to arachidonic acid metabolism, glycerophospholipid metabolism and linoleic acid metabolism. Moreover, principal component analysis showed that the metabolites in the model group were clearly classified, whereas the metabolites in the LRK group were between those in the normal and model groups but closer to those in the normal group. This finding indicated that these metabolites may be responsible for the effects of LRK. The therapeutic effect of LRK on HMG was possibly related to the regulation of 10 specific metabolites. In addition, we further verified the expression of protein kinase C alpha (PKCα), a key target predicted by network pharmacology analysis, and showed that LRK could significantly improve the expression of PKCα.
Conclusion: Our study successfully explained the modulatory properties of LRK treatment on HMG using metabolomics and network pharmacology analyses. This systematic method can provide methodological support for further understanding the complex mechanism underlying HMG and possible traditional Chinese medicine (TCM) active ingredients for the treatment of HMG.
Hyperplasia of mammary glands is one of the most common breast diseases in middle-aged women and accounts for more than 70% of all breast disease (Chen et al., 2015). HMG is easily overlooked because of its clinical characteristics until the generation of mammary carcinoma. (Zhang et al., 2012). The morbidity of HMG has increased due to increased work-related stress and competitive career pressures along with the fast pace of modern life (Li et al., 2017a). However, there is still not a sufficient understanding of the etiology of HMG, and pertinent therapeutic strategies are limited. Hormone or endocrine therapy is one of the most commonly used methods to mitigate the clinical symptoms of HMG. Nevertheless, the side effects also decrease the quality of life for patients who receive long-term treatment. Surgical treatment as a form of therapy is hardly accepted by most patients with recurring symptoms (Henry, 2014). Therefore, finding a more appropriate treatment with fewer side effects and more therapeutic advantages is the current goal standard for treating HMG.
Traditional Chinese medicine (TCM) has been practiced in China for thousands of years to treat acute and chronic diseases. Its application in the prevention and treatment of HMG has garnered increasing attention. To explore the mechanism of action and active substances of TCM, a variety of constructive technologies have been used over the past few decades, such as metabolomics, proteomics, genomic arrays and network pharmacology (Xu et al., 2017). However, few researchers fully integrate the aforementioned techniques.
Li-Ru-Kang is composed of Curcumae radix, Prunellae spica, Pseudobulbus cremastrae Seu pleiones, Radix bupleuri, licorice, Polygonum multiflorum, Crassostrea gigas and Cornu cervi, and has been used for the treatment of HMG for several decades as a cipher prescription based on the clinical experience of many medical experts. Clinical researches showed that the total effective rate of LRK in the treatment of HMG was 88.0%. Moreover, LRK showed obvious superiority in improving the patients’ symptoms and abnormalities of gonadal hormone (Qian et al., 2007).
As a rapidly developing technology, metabolomics can discover the pathogenesis of diseases by detecting more than 1,000 molecules in various biological fluids, such as urine, saliva, and blood. This technology has been successfully applied to the diagnosis and identification of various diseases, such as coronary heart disease (Li et al., 2017c), early chronic kidney disease (Benito et al., 2018) and thyroid cancer (Navas-Carrillo et al., 2017). Metabolomics has also been widely applied in the field of TCM (Li et al., 2017b; Liu et al., 2017) and provides insight into the complex network mechanisms of HMG. In addition, network pharmacology has also been successfully applied to the study of TCM molecular mechanisms and can provide a deep understanding of the complex relationship between TCM components and diseases.
In this study, we elucidated the bioactive components, potential biomarkers and possible mechanisms of LRK in the treatment of HMG using metabolomics and network pharmacology.
Curcumae radix, Prunellae spica, Pseudobulbus cremastrae Seu pleiones, Radix bupleuri, licorice, Polygonum multiflorum, Crassostrea gigas and Cornu cervi were purchased from He yanling, Co., Ltd. (Beijing, China). The origin and quality of the 8 herbs were identified according to the Chinese Pharmacopeia (2015 Edition). Water extract from LRK was prepared by extracting the mixture of the 8 herbs (at a ratio of 12: 12: 9: 10: 30: 10: 9: 6, respectively) twice with water for 1 h. The extract was then decanted, filtered and dried under reduced pressure. The final ratio of powder to raw herb was 9.2%.
Female Sprague-Dawley rats weighing 200 ± 5 g (license number: SCXK-(A) 2012-0004) were obtained from the laboratory animal center of Military Medical Science Academy of the PLA. All studies were performed in accordance with the Guiding Principles for the Care and Use of Laboratory Animals of China. The animals were housed in the same feeding environment (temperature: 25 ± 2°C; humidity: 50 ± 10%) and fed based on lighting conditions (12:12 h light: dark cycle) with a standard diet and water ad libitum. After acclimatization for 7 days, all animals were randomly divided into six groups (n = 6): a normal group (A), a model group (B), a tamoxifen-treated HMG group (C), a low dose LRK-treated HMG group (D), a medium dose LRK-treated HMG group (E) and a high dose LRK-treated HMG group (F). Rats in the B, C, D, E, and F groups were intramuscularly injected with estrogen (0.5 mg/kg/d) for 25 days, followed by progestogen (5 mg/kg/d) for another 5 days to induce the experimental HMG model (Wang et al., 2011). Rats in groups D, E, and F were given 0.6, 1.2 and 2.4 g/kg LRK orally for 30 days, respectively. Rats in groups A and B were treated with an equal volume of saline. Rats in group C were given oral tamoxifen (4 mg/kg/d) for 30 days. All animal studies were approved by the Ethical Committee of 302 Military Hospital of China.
After the last treatment, all rats were sacrificed. Blood samples were collected and centrifuged at 3000 ×g for 10 min to obtain serum for mass spectrometry. The mammary samples were rapidly excised and fixed in 10% paraformaldehyde solution for histopathological analysis. Paraffin-embedded sections ranging from 4 to 5 μm in thickness were stained with haematoxylin-eosin (H & E). The stained sections were analyzed with a Nikon microscope (Nikon Instruments Corporation, Shanghai, China) and Image-Pro Plus 7200 software.
Briefly, 200 μL of serum samples and 600 μL of methanol were mixed uniformly and allowed to stand for 20 min at 4°C. The samples were centrifuged at 12,000 rpm at 4°C for 10 min to obtain the supernatant and then filtered through a syringe filter (0.22 μm) to obtain the sample for injection.
Chromatography was carried out using an Agilent 1290 series UHPLC system. The sample injection volume was 4 μL, and all samples were detected at 4°C on a ZORBAX RRHD 300 SB-C18 column (2.1 mm × 100 mm, 1.8 μm; Agilent, United States). The mobile phases were composed of 0.1% formic acid in acetonitrile (solvent A) and 0.1% formic acid in water (solvent B), and the flow rate was 0.30 mL/min. The gradient was set as follows: the first minute: 95% A; 1.0 to 9.0 min: 95–60% A; 9.0 to 19.0 min: 60–10% A; 19.0 to 21.0 min: 10–0% A; and 21.0 to 25.0 min: 0% A. After injection of the 10 samples and the QC sample compounded with all samples, a blank was injected to guarantee the stability and repeatability of the UPLC-QTOF/MS systems.
Mass spectrometry was performed using an Agilent 6550 Q-TOF/MS instrument (Agilent Technologies, Santa Clara, CA, United States) with an electrospray ionization source (ESI) in both positive and negative ionization mode. The following electrospray source parameters were used: the electrospray capillary voltages were 3.0 kV (negative ionization mode) and 4.0 kV (positive ionization mode); the gas temperature was 200°C (negative ionization mode) and 225°C (positive ionization mode); the mass range ranged from m/z 80 to 1000; the gas flow was 11 L/min; the nebulizer was 35 pisg in negative ionization mode and 45 pisg in positive ionization mode; the sheath gas temperature was set to 350°C, and the sheath gas flow was 12 L/min; the nozzle voltage was 500 V in both negative and positive ionization modes.
MassHunter Profinder software (Agilent, California, United States) was used to extract sample data for peak detection and alignment. Full scan mode was applied in the mass range of 80–1000 m/z. The initial and final retention times were set for data collection. The resultant data matrices were normalized using MetaboAnalyst 3.01 and then introduced to SIMCA-P 13.0 software (Umetrics, Umea, Sweden) for PCA and PLSDA analysis. PCA was used as an unsupervised pattern recognition approach to reduce the dimension of the UPLC-QTOF/MS data and disclose intrinsic clustering of samples. PLS-DA analysis was employed to maximize the differences in inter-class discrimination and minimize the differences in inter-class discrimination. The variables with VIP >1.5 and |p(corr)|≥0.58 in the PLS-DA analysis were further evaluated with an independent sample t-test.
The significant variables (p < 0.05 in ANOVA) were selected as potential biomarkers for further pathway enrichment analysis. These biomarkers were identified by METLIN2, and the identified compound names were resubmitted to MetaboAnalyst 3.0 to analyse their signaling pathways.
To systematically elucidate the complex relationships between potential metabolites and their associated targets, we conducted network analysis using network pharmacology. The ingredients with an oral bioavailability (OB) ≥30% and a drug-likeness (DL) >0.18 from Radix bupleuri, licorice, Pseudobulbus cremastrae Seu pleiones, Prunellae spica, Polygonum multiflorum and Curcumae radix were put into the TCMSP database3, and their corresponding chemical components were also collected from the same database (Huang et al., 2017). The ingredients and corresponding targets of Cornu cervi and Crassostrea gigas were retrieved from the BATMAN-TCM database4. The protein targets of potential metabolites were collected from the MBROLE 2.0 database5 (Lopez-Ibanez et al., 2016). Different protein ID types were converted to UniProt IDs. Then, the “metabolite-target-chemical components” interactive network was established by using protein–protein interaction (PPI) information. Cytoscape 2.8.3 (National Institute of General Medical Sciences, United States) was applied to visualize and analyse the network.
To evaluate the effect of LRK on the expression of PKCα in breast tissue of HMG rats, immunohistochemistry was performed as described previously (Chen et al., 2014). Breast tissue slides were incubated with anti- PKCα (Abcam, Cambridge, MA, United States) for 12 h at 4°C. After treatment with HRP-conjugated goat anti-mouse IgG and 50 μL of streptavidin-peroxidase solutions for 30 min at RT, the sections were stained with DAB and counterstained with hematoxylin. The positive areas showed the color of brown yellow.
Data were expressed as the mean ± SE and analyzed with the SPSS 13.0 software program (Chicago, United States). The differences between the group means were calculated by ANOVA. p < 0.05 was considered statistically significant, and p < 0.01 was considered highly significant. The results of the three-dimensional matrix containing the peak index, sample name, and peak area were introduced into SIMCA-P 13.0 software for pattern recognition analysis of PCA and PLS-DA.
The average diameter and height of nipples (Left 2 and Right 2) were measured as indices of HMG. As shown in Figure 1, rats given estrogen and progesterone displayed remarkable increases in the average nipple diameter and height. The administration of LRK (2.4 and 1.2 g/kg) markedly decreased the average nipple diameter and height, respectively. Pathological studies provided direct evidence of protective effects of LRK on HMG. In Figure 2, the mammary tissues of the rats in the normal group exhibited normal morphological structures with no abnormal features. By contrast, the mammary tissues of the rats in the model group displayed more acinus and lobules, expanded mammary lumens, and hyperplastic ducts. Conversely, treatment with LRK (2.4 and 1.2 g/kg) ameliorated these morphological changes. These results preliminarily verified that 2.4 and 1.2 g/kg LRK significantly protected the mammary tissue in the rats with HMG induced by estrogen and progesterone.
FIGURE 1. The average diameter and height of nipples in the HMG rats. (A) The average diameter of nipples. (B) The average height of nipples. 1: normal group. 2: model group. 3: tamoxifen-treated HMG group (4 mg/kg). 4: low-dose LRK-treated HMG group (0.6 g/kg). 5: medium-dose LRK-treated HMG group (1.2 g/kg). 6: high-dose LRK-treated HMG group (2.4 g/kg). Data are expressed as the mean ± SE (n = 6). ∗p < 0.01 compared with the normal group; ##p < 0.01, #p < 0.05 compared with the model group.
FIGURE 2. Effects of LRK on the histopathology of mammary tissues using haematoxylin and eosin staining (200× and 400×). (A) Normal group. (B) Model group. (C) Tamoxifen-treated HMG group (4 mg/kg). (D) Low-dose LRK-treated HMG group (0.6 g/kg). (E) Medium-dose LRK-treated HMG group (1.2 g/kg). (F) High dose LRK-treated HMG group (2.4 g/kg). The magnified areas (×400) are marked with a black arrow in the pathological tissue figures (200×).
Principal component analysis (PCA) and orthogonal partial least squares-discriminant analysis (OPLS-DA) are pattern recognition approaches frequently used to classify metabolic phenotypes and identify different metabolites to evaluate variation among complex data sets. Score plots revealed a direct image of observational clusters. A distinguished classification between the clustering of the normal and model groups and the normal and LRK groups was observed in both the positive (Figure 3A) and negative modes (Figure 3B). Further multivariate analysis was needed to explore which metabolites caused these differences.
FIGURE 3. Principal component analysis (PCA) score plot of the control, model and LRK (2.4 g/kg) groups. (A) ESI+ model. (B) ESI- model.
Orthogonal partial least squares-discriminant analysis was used to identify potential biomarkers that were significantly changed between the normal, model and LRK groups. The parameters R2X (cum), R2Y (cum), and Q2 (cum) were used to provide an estimate of how well the model fit the data. We further determined whether the metabolite fingerprints in the serum differed between the normal, model, and LRK groups by constructing an OPLS-DA model. A distinguished classification between the clustering of the normal, model, and LRK groups was observed in both the positive (Figure 4) and negative models (Supplementary Figure S1), suggesting a significant serum biochemical perturbation in the model and LRK groups. The R2X (cum), R2Y (cum), and Q2 (cum) of OPLS-DA in our positive model were 0.506, 0.936, 0.79, respectively, using the data from the control and model groups and 0.304, 0.87, 0.748, respectively, using the data from the model and LRK groups. The R2X (cum), R2Y (cum), and Q2 (cum) of OPLS-DA in our negative model were 0.261, 0.908, 0.768, respectively, using the data from the control and model groups and 0.233, 0.97, 0.806, respectively, using the data from the model and LRK groups. The corresponding parameter results indicated that models were of good quality and provided accurate predictions. Variables further away from the origin in the corresponding S-plots were thought to contribute more significantly were more thus responsible for the separation between the normal and model groups as well as the model and LRK groups in both the positive (Figures 4B,E) and negative ionization models (Supplementary Figure S1). These variables may therefore be regarded as potential biomarkers. Permutation tests with 100 iterations were performed to validate the model. These tests compared the goodness of fit of the original model with the goodness of fit of randomly permuted models. As shown in Figures 4C,F, the validation plots indicated that the original models were valid.
FIGURE 4. The OPLS-DA score plots, S-plots and 100-permutation test generated from the OPLS-DA data of the normal, model and LRK groups in ESI+ mode. OPLS-DA score plots were the pair-wise comparisons between the normal and model groups (A) as well as between the model and LRK groups (D). S-plots of the OPLS-DA model for the normal and model groups (B) as well as for the model and LRK groups (E). The 100-permutation test of the OPLS-DA model was for the normal and model groups (C) as well as for the model and LRK groups (F).
Among the 1986 signals detected in the control, model and 2.4 g/kg LRK groups, variables that significantly contributed to the clustering and discrimination were identified according to a threshold of VIP ≥1.5 and |p(corr)|≥ 0.58. Those thresholds were obtained after OPLS-DA processing these variables. According to the VIP and |p(corr)|, 366 variables were selected from the control, model and 2.4 g/kg LRK groups as the candidates for fold-changes and ANOVA analyses. The candidates that significantly differed among the groups with fold change exceeding two were identified as candidate biomarkers for METLIN and Metaboanalyst identification. Ten potential biomarkers were summarized in Table 1 with their corresponding formula, retention time, m/z, and differences by group.
Herein, 10 potential metabolites were expressed at significant levels. Pathway analysis was performed in detail using Metaboanalyst. HMG-associated metabolites were responsible for metabolizing arachidonic acid, glycerophospholipid, linoleic acid, sphingolipid, alpha-linolenic acid, glycine, serine, threonine, arginine, proline, and purine (Table 2 and Figure 5). The top three metabolic pathways were arachidonic acid, glycerophospholipid and linoleic acid.
FIGURE 5. (A) Metabolomic Pathway construction of the metabolic pathways involved in the effects of LRK on HMG. (B) Signaling networks associated with the differentially expressed metabolic pathways. 1: Arachidonic acid metabolism. 2: Glycerophospholipid metabolism. 3: Linoleic acid metabolism. 4: Sphingolipid metabolism. 5: alpha-linolenic acid metabolism. 6: Glycine, serine and threonine metabolism. 7: Arginine and proline metabolism. 8: Purine metabolism. The red solid box represents the peak area of the LRK/model >1. The blue solid box represents the peak area of LRK/model < 1.
To reveal the proteins targets and chemical components of LRK regulation, the “potential metabolite-target-component” interactive network was structured by combing the drug targets and the targets associated with potential metabolites. As shown in Figure 6, 445 drug targets, 170 targets associated with potential metabolites, and 3 potential metabolites, including phosphatidylserine (C02737), prostaglandin E2 (C00584) and phosphatidylcholine (C00157), participated in the “potential metabolite-target-component” interactive network (Figure 6A). Five drug targets, including protein kinase C alpha type (P17252), prostaglandin E synthase (O14684), prostaglandin E2 receptor EP3 subtype (P43115), group IIE secretory phospholipase A2 (Q9NZK7) and phospholipase B1 (Q6P1J6), directly regulated the 3 potential metabolites (Figure 6B). The 5 drug targets were directly regulated by multiple chemical components, including beta-sitosterol, quercetin, p-coumaric acid and naringenin, which were collected from 4 herbs, including Curcumae radix, Prunellae spica, Radix bupleuri and Pseudobulbus cremastrae Seu pleiones in LRK (Figure 7).
FIGURE 6. The “potential metabolite-target-component” interactive network with all target information (A) and key target information (B) participating in the treatment of HMG by LRK. The red triangles represent active chemical constituents of LRK. The blue dots represent the protein targets of drugs. The yellow dots represent potential metabolites. The purple dots represent the targets associated with potential metabolites.
FIGURE 7. The “potential metabolite-target-component-herb” interactive network participating in the treatment of HMG by LRK. The red triangles represent active chemical constituents of LRK. The blue dots represent the protein targets of herbs. The yellow dots represent the potential metabolites. The blue rectangles represent herbs.
Analysis of the network pharmacology prediction results showed that the greatest number of chemical components directly connected to PKCα, suggesting that PKCα may play a more important role in the treatment of HMG by LRK. Therefore, to verify the authenticity of the network pharmacology prediction results, we evaluated the expression of PKCα in breast tissue by immunohistochemistry. The results indicated the expression of PKCα was significantly increased in the breast tissues of rats in the model group, while the different doses of LRK significantly reduced the expression of PKCα (Figure 8).
FIGURE 8. Effects of LRK on the expression of PKCα in breast tissue of HMG rats using immunohistochemistry (200× and 400×). (A) (200×) and a (400×) : normal group. (B) (200×) and b (400×): model group. (C) (200×) and c (400×): low-dose LRK-treated HMG group (0.6 g/kg). (D) (200×) and d (400×): medium-dose LRK-treated HMG group (1.2 g/kg). (E) (200×) and e (400×): high dose LRK-treated HMG group (2.4 g/kg).
The morbidity rate of HMG is increasing annually and continues to affect younger women (Zhang et al., 2008). HMG is classified in the “Rupi” category according to TCM theory (Fan et al., 2013). While network pharmacology and metabonomics have been applied to TCM research (Su et al., 2017; Wang et al., 2017), few studies have attempted to combine these two approaches. Metabolomics can analyze and detect small molecules in bodily fluids to determine which compounds have significant abnormalities and to uncover the mechanism of disease development. Network pharmacology can analyze the interaction of macromolecule targets corresponding to chemical components and diseases and reveal the molecular mechanisms of drug treatment. By combining metabolomics and network pharmacology, we were able to gain a deeper understanding of the molecular mechanisms underlying TCM treatment.
Li-Ru-Kang is made up of 8 kinds of herbs. It has been effective in treating HMG for decades. In this study, we combined metabonomics and network pharmacology analyses to evaluate the efficacy, active components and possible molecular mechanisms of LRK in the treatment of HMG. LRK significantly improved the morphological characteristics of nipples and reduced the pathological state of HMG in rats. Furthermore, we described the metabolomic feature profile and metabolite interaction network of LRK in treating HMG. LRK exhibited protective effects against HMG by reversing potential metabolites to normal levels. Ten metabolites were significantly regulated by LRK. Seven of these metabolites, including prostaglandin E2, leukotriene B4, phosphatidylcholine, coenzyme Q9, ceramide, gamma-glutamyl-L-putrescine and 5-amino-4-imidazole carboxylate, could interact in different ways. The results indicated that the occurrence and development of HMG were caused by changes in many aspects of physiologically and pathologically related molecules and that most of the changes in the body’s molecules were interrelated. LRK regulated these 10 biomarkers to normalize their expression levels, indicating that LRK could be used to treat HMG via multiple pathways and multiple targets.
To obtain a deeper understanding of the LRK mechanism for treating HMG and the correlation between LRK chemical components and metabolites, we further combined the network pharmacology method to establish the “potential metabolite-target-component” interactive network. The results showed that four chemical components, beta-sitosterol, quercetin, p-coumaric acid and naringenin, which come from Curcumae radix, Prunellae spica, Radix bupleuri and Pseudobulbus cremastrae Seu pleiones, acted directly on multiple targets that are directly related to metabolites, including prostaglandin E synthase, protein kinase C alpha type, prostaglandin E2 receptor EP3 subtype, group IIE secretory phospholipase A2 and phospholipase B1. The results suggested that multiple components in LRK played an important role in regulating the endogenous physical disturbance of the body, thus indicating the advantages of LRK in the clinical treatment of HMG. By investigating the pathogenesis of HMG, we found that inflammation and oxidative stress mechanisms played a key role in the development of HMG (Holloway et al., 2012). At the same time, some drugs act on HMG by exerting anti-inflammatory and antioxidant pharmacological effects (Das Gupta et al., 2015; Huang et al., 2017). Studies have shown that beta-sitosterol (Villasenor et al., 2002; Tan et al., 2008), quercetin (Kamaraj et al., 2007), p-coumaric acid (Ilavenil et al., 2016) and naringenin (Cavia-Saiz et al., 2010; Chen et al., 2013) in LRK have anti-inflammatory and antioxidant pharmacological activities. The network pharmacology results showed that PKCα was the target directly connected to the four chemical components. This target is also the most closely related target to inflammation and oxidative stress (Kopach et al., 2013; Andersson et al., 2016). Therefore, to verify the accuracy of the network pharmacology prediction results, we further examined the expression of PKCα in the breast tissues of HMG rats. The expression of PKCα was increased significantly in HMG rats, and LRK significantly reduced PKCα expression. These results suggested that the network pharmacology prediction results were credible. This experiment also demonstrated the significance of PKCα in the pathogenesis of HMG and LRK treatment.
This study systematically explored the molecular mechanism of LRK for the treatment of HMG by combining metabonomics and network pharmacology analyses. In addition, this innovative research method can provide new ideas for research on the molecular mechanisms of TCM in the treatment of complex diseases.
SW and LQ performed the experiments, analyzed the data, and wrote the manuscript. MN, HHL, YY, YW, LZ, XZ, and HTL collected and prepared samples. RW, KL, and YZ performed the analyses.
This work was financially supported by Project of the Project of Chinese Medicine Education Association (No. 2016SKT-M035), Chinese Medicine Education Association (No. 2016SKT-Z003) and Beijing Municipal Science & Technology Commission (No. Z171100001717013), and State Natural Science Foundation (No. 81573631).
The authors declare that the research was conducted in the absence of any commercial or financial relationships that could be construed as a potential conflict of interest.
The Supplementary Material for this article can be found online at: https://www.frontiersin.org/articles/10.3389/fphar.2018.00651/full#supplementary-material
Andersson, A., Bluwstein, A., Kumar, N., Teloni, F., Traenkle, J., Baudis, M., et al. (2016). PKCalpha and HMGB1 antagonistically control hydrogen peroxide-induced poly-ADP-ribose formation. Nucleic Acids Res. 44, 7630–7645. doi: 10.1093/nar/gkw442
Benito, S., Sanchez-Ortega, A., Unceta, N., Jansen, J. J., Postma, G., Andrade, F., et al. (2018). Plasma biomarker discovery for early chronic kidney disease diagnosis based on chemometric approaches using LC-QTOF targeted metabolomics data. J. Pharm. Biomed. Anal. 149, 46–56. doi: 10.1016/j.jpba.2017.10.036
Cavia-Saiz, M., Busto, M. D., Pilar-Izquierdo, M. C., Ortega, N., Perez-Mateos, M., and Muniz, P. (2010). Antioxidant properties, radical scavenging activity and biomolecule protection capacity of flavonoid naringenin and its glycoside naringin: a comparative study. J. Sci. Food Agric. 90, 1238–1244. doi: 10.1002/jsfa.3959
Chen, T., Li, J., Chen, J., Song, H., and Yang, C. (2015). Anti-hyperplasia effects of Rosa rugosa polyphenols in rats with hyperplasia of mammary gland. Environ. Toxicol. Pharmacol. 39, 990–996. doi: 10.1016/j.etap.2015.02.014
Chen, Y., Nie, Y. C., Luo, Y. L., Lin, F., Zheng, Y. F., Cheng, G. H., et al. (2013). Protective effects of naringin against paraquat-induced acute lung injury and pulmonary fibrosis in mice. Food Chem. Toxicol. 58, 133–140. doi: 10.1016/j.fct.2013.04.024
Chen, Y. W., Tsai, M. Y., Pan, H. B., Tseng, H. H., Hung, Y. T., and Chou, C. P. (2014). Gadoxetic acid-enhanced MRI and sonoelastography: non-invasive assessments of chemoprevention of liver fibrosis in thioacetamide-induced rats with Sho-Saiko-To. PLoS One 9:e114756. doi: 10.1371/journal.pone.0114756
Das Gupta, S., So, J. Y., Wall, B., Wahler, J., Smolarek, A. K., Sae-Tan, S., et al. (2015). Tocopherols inhibit oxidative and nitrosative stress in estrogen-induced early mammary hyperplasia in ACI rats. Mol. Carcinog. 54, 916–925. doi: 10.1002/mc.22164
Fan, Y., Pei, X., Liu, Z., Xia, Z., Zhang, D., Song, A., et al. (2013). Effectiveness of external Sanjierupi Gao on mastalgia caused by mammary gland hyperplasia: a placebo controlled trial. J. Tradit. Chin. Med. 33, 603–607. doi: 10.1016/S0254-6272(14)60028-5
Henry, N. L. (2014). Endocrine therapy toxicity: management options. Am. Soc. Clin. Oncol. Educ. Book e25–e30. doi: 10.14694/EdBook_AM.2014.34.e25
Holloway, R. W., Bogachev, O., Bharadwaj, A. G., McCluskey, G. D., Majdalawieh, A. F., Zhang, L., et al. (2012). Stromal adipocyte enhancer-binding protein (AEBP1) promotes mammary epithelial cell hyperplasia via proinflammatory and hedgehog signaling. J. Biol. Chem. 287, 39171–39181. doi: 10.1074/jbc.M112.404293
Huang, J., Tang, H., Cao, S., He, Y., Feng, Y., Wang, K., et al. (2017). Molecular targets and associated potential pathways of Danlu capsules in hyperplasia of mammary glands based on systems pharmacology. Evid. Based Complement. Alternat. Med. 2017:1930598. doi: 10.1155/2017/1930598
Ilavenil, S., Kim da, H., Srigopalram, S., Arasu, M. V., Lee, K. D., Lee, J. C., et al. (2016). Potential application of p-coumaric acid on differentiation of C2C12 skeletal muscle and 3T3-L1 preadipocytes-an in vitro and in silico approach. Molecules 21:E997. doi: 10.3390/molecules21080997
Kamaraj, S., Vinodhkumar, R., Anandakumar, P., Jagan, S., Ramakrishnan, G., and Devaki, T. (2007). The effects of quercetin on antioxidant status and tumor markers in the lung and serum of mice treated with benzo(a)pyrene. Biol. Pharm. Bull. 30, 2268–2273. doi: 10.1248/bpb.30.2268
Kopach, O., Viatchenko-Karpinski, V., Atianjoh, F. E., Belan, P., Tao, Y. X., and Voitenko, N. (2013). PKCalpha is required for inflammation-induced trafficking of extrasynaptic AMPA receptors in tonically firing lamina II dorsal horn neurons during the maintenance of persistent inflammatory pain. J. Pain 14, 182–192. doi: 10.1016/j.jpain.2012.10.015
Li, X., Xin, P., Wang, C., Wang, Z., Wang, Q., and Kuang, H. (2017a). Mechanisms of traditional Chinese medicine in the treatment of mammary gland hyperplasia. Am. J. Chin. Med. 45, 443–458. doi: 10.1142/S0192415X17500276
Li, X., Zhang, A., Sun, H., Liu, Z., Zhang, T., Qiu, S., et al. (2017b). Metabolic characterization and pathway analysis of berberine protects against prostate cancer. Oncotarget 8, 65022–65041. doi: 10.18632/oncotarget.17531
Li, Y., Zhang, D., He, Y., Chen, C., Song, C., Zhao, Y., et al. (2017c). Investigation of novel metabolites potentially involved in the pathogenesis of coronary heart disease using a UHPLC-QTOF/MS-based metabolomics approach. Sci. Rep. 7:15357. doi: 10.1038/s41598-017-15737-3
Liu, P., Shang, E. X., Zhu, Y., Yu, J. G., Qian, D. W., and Duan, J. A. (2017). Comparative analysis of compatibility effects on invigorating blood circulation for cyperi rhizoma series of herb pairs using untargeted metabolomics. Front. Pharmacol. 8:677. doi: 10.3389/fphar.2017.00677
Lopez-Ibanez, J., Pazos, F., and Chagoyen, M. (2016). MBROLE 2.0-functional enrichment of chemical compounds. Nucleic Acids Res. 44, W201–W204. doi: 10.1093/nar/gkw253
Navas-Carrillo, D., Rodriguez, J. M., Montoro-Garcia, S., and Orenes-Pinero, E. (2017). High-resolution proteomics and metabolomics in thyroid cancer: deciphering novel biomarkers. Crit. Rev. Clin. Lab. Sci. 54, 446–457. doi: 10.1080/10408363.2017.1394266
Qian, L. Q., Pei, X. H., Xu, Z. Y., and Wang, C. (2007). Clinical observation on treatment of hyperplasia of mammary gland by Lirukang Granule. Chin. J. Integr. Med. 13, 120–124. doi: 10.1007/s11655-007-0120-y
Su, G., Wang, H., Gao, Y., Chen, G., Pei, Y., and Bai, J. (2017). 1H-NMR-based metabonomics of the protective effect of Coptis chinensis and berberine on cinnabar-induced hepatotoxicity and nephrotoxicity in rats. Molecules 22:1855. doi: 10.3390/molecules22111855
Tan, M. A., Takayama, H., Aimi, N., Kitajima, M., Franzblau, S. G., and Nonato, M. G. (2008). Antitubercular triterpenes and phytosterols from Pandanus tectorius Soland. var. laevis. J. Nat. Med. 62, 232–235. doi: 10.1007/s11418-007-0218-8
Villasenor, I. M., Angelada, J., Canlas, A. P., and Echegoyen, D. (2002). Bioactivity studies on beta-sitosterol and its glucoside. Phytother. Res. 16, 417–421. doi: 10.1002/ptr.910
Wang, L., Zhao, D., Di, L., Cheng, D., Zhou, X., Yang, X., et al. (2011). The anti-hyperplasia of mammary gland effect of Thladiantha dubia root ethanol extract in rats reduced by estrogen and progestogen. J. Ethnopharmacol. 134, 136–140. doi: 10.1016/j.jep.2010.11.071
Wang, N., Zhao, G., Zhang, Y., Wang, X., Zhao, L., Xu, P., et al. (2017). A network pharmacology approach to determine the active components and potential targets of Curculigo orchioides in the treatment of osteoporosis. Med. Sci. Monit. 23, 5113–5122. doi: 10.12659/MSM.904264
Xu, T., Pi, Z., Liu, S., Song, F., and Liu, Z. (2017). Chemical profiling combined with "omics" technologies (CP-Omics): a strategy to understand the compatibility mechanisms and simplify herb formulas in traditional Chinese medicines. Phytochem. Anal. 28, 381–391. doi: 10.1002/pca.2685
Zhang, G., Li, D., Guo, H., Ma, Y., Liao, R., Tan, B., et al. (2012). Modulation of expression of p16 and her2 in rat breast tissues of mammary hyperplasia model by external use of rupifang extract. J. Tradit. Chin. Med. 32, 651–656. doi: 10.1016/S0254-6272(13)60087-4
Keywords: Li-Ru-Kang, hyperplasia of mammary glands, modulatory properties, metabolomics, network pharmacology
Citation: Wei S, Qian L, Niu M, Liu H, Yang Y, Wang Y, Zhang L, Zhou X, Li H, Wang R, Li K and Zhao Y (2018) The Modulatory Properties of Li-Ru-Kang Treatment on Hyperplasia of Mammary Glands Using an Integrated Approach. Front. Pharmacol. 9:651. doi: 10.3389/fphar.2018.00651
Received: 03 January 2018; Accepted: 31 May 2018;
Published: 19 June 2018.
Edited by:
Jiang Bo Li, The Second People’s Hospital of Wuhu, ChinaReviewed by:
Weijun Kong, Institute of Medicinal Plant Development (CAMS), ChinaCopyright © 2018 Wei, Qian, Niu, Liu, Yang, Wang, Zhang, Zhou, Li, Wang, Li and Zhao. This is an open-access article distributed under the terms of the Creative Commons Attribution License (CC BY). The use, distribution or reproduction in other forums is permitted, provided the original author(s) and the copyright owner are credited and that the original publication in this journal is cited, in accordance with accepted academic practice. No use, distribution or reproduction is permitted which does not comply with these terms.
*Correspondence: Ming Niu, bm1icmlnaHRAMTYzLmNvbQ== Yanling Zhao, emhhb3lsMjg1NUAxMjYuY29t
Disclaimer: All claims expressed in this article are solely those of the authors and do not necessarily represent those of their affiliated organizations, or those of the publisher, the editors and the reviewers. Any product that may be evaluated in this article or claim that may be made by its manufacturer is not guaranteed or endorsed by the publisher.
Research integrity at Frontiers
Learn more about the work of our research integrity team to safeguard the quality of each article we publish.