- Department of Pediatrics, Zhongnan Hospital of Wuhan University, Wuhan University, Wuhan, China
The association of preterm or low birth weight (LBW) with the risk of metabolic syndrome is still unclear. This study aimed to assess the association between preterm or LBW and metabolic syndrome risk according to study or participants' characteristics. PubMed, Web of Science, and EMBASE were searched for epidemiologic studies on the association published up to April 30, 2020. Pooled odds ratio (ORs) and weighted mean differences (WMDs) with 95% confidence intervals (CIs) were calculated using the random-effects model. Low birth weight was associated with an increased risk of metabolic syndrome (OR, 1.37; 95% CI, 1.17–1.61). In the subgroup analysis by study design, the pooled ORs for LBW and metabolic syndrome in the cohort and cross-sectional studies were 1.79 and 1.22. In the subgroup analysis by sex, LBW was found to be associated with an increased risk of metabolic syndrome in pooled studies including both men and women or studies including only women. The association between premature birth and risk of metabolic syndrome was significant in cohort studies (OR, 1.72; 95% CI, 1.12–2.65). Also, LBW or preterm was significantly associated with a higher Homeostasis Model Assessment of Insulin Resistance (WMD, 0.28; 95% CI, 0.19–0.36). Low birth weight and preterm might be risk factors for metabolic syndrome.
Introduction
Metabolic syndrome is defined as a cluster of any three or more of these features: elevated waist circumference, elevated triglyceride level, reduced high-density lipoprotein cholesterol level, elevated blood pressure, elevated fasting glucose level (1–3), and insulin resistance is the pathogenesis (4, 5). The median prevalence of metabolic syndrome in the whole population was 3.3%, which ranged from 0 to 19.2% (6). Previous studies found that a cluster of symptoms of metabolic syndrome was associated with various chronic diseases, including cardiovascular disease, type 2 diabetes mellitus, and cancer at various sites (7–9). Therefore, clarifying the independent risk factors for metabolic syndrome is particularly important in the general population.
Several epidemiological studies were conducted to look for the cause of metabolic syndrome. Many dietary, behavioral, and psychological factors have been confirmed to be associated with metabolic syndrome, such as sugar-sweetened and artificially sweetened beverage intake (10), low levels of physical activity and sedentary behavior (11), and anxiety (12). In recent years, many studies found an association of several perinatal risk factors, such as low birth weight (LBW) and premature birth, with the increased risk of metabolic syndrome, but some others showed contradictory results (13–27). Therefore, this meta-analysis was conducted to evaluate the association of LBW and premature birth with metabolic syndrome. Moreover, the stratified analyses according to study design, sex, and continent were also illustrated.
Materials and Methods
This meta-analysis was performed following the Preferred Reporting Items for Systematic Reviews and Meta-Analysis Statement Checklist (28).
Literature Search Strategy
The databases PubMed, Web of Science, and EMBASE up to April 30, 2020, were searched, using the following terms: [(prematurity) OR (premature birth) OR (premature infant) OR (low birth weight) OR (preterm)] AND [(metabolic syndrome) OR (metabolic syndrome, components) OR (hypertension) OR (high blood pressure) OR (insulin resistance) OR (glucose intolerance) OR (obesity) OR (overweight) OR (fat mass) OR (dyslipidemia) OR (hypercholesterolemia), as text words or Medical Subject Heading terms. In addition, the reference lists of the included studies were reviewed for undetected relevant studies.
Inclusion Criteria
The details of inclusion criteria were as follows: (1) study design: the study was original research from observational studies; (2) participants: general population; (3) exposure: LBW or premature birth; (4) comparator: normal birth weight or full-term birth; and (5) outcome: metabolic syndrome. The most recent and complete study was selected if data from the same population had been published repeatedly. The exclusion criteria were as follows: review, comments, animal experiments, patients diagnosed with other diseases, and studies that did not report the effect estimates between LBW or preterm and metabolic syndrome.
All identified studies were searched and reviewed by three investigators (L.L.H., D.Y.P., and Z.D.C.) independently. Disagreements on the eligibility of a study were resolved by consensus by the primary author (Z.D.C.) referring to the original article.
Data Extraction and Quality Assessment
Data extraction from each study by two investigators independently included the first author's name, publication year, country where the study was conducted, study design, age, sample size, and number of cases, perinatal risk factors (LBW or premature birth), odds ratio (OR), or hazard ratio (all results were presented as OR owing to this study designed as cohort) with 95% confidence interval (CI), definition of metabolic syndrome, adjustment for potential confounding factors, cutoff value of LBW and preterm, and Homeostasis Model Assessment of Insulin Resistance (HOMA-IR). For studies that reported several multivariate adjusted ORs, the effect estimate that was maximally adjusted for potential confounders was selected. The study quality was assessed using the Newcastle–Ottawa Scale (NOS), which was based on selection (four items), comparability (one item), and outcome (three items), with a total of 0–9 stars (29).
Statistical Analysis
The association of LBW or preterm with the risk of metabolic syndrome was assigned as categorical data, and OR with its 95% CI was calculated in an individual study before data pooling. Moreover, the potential association of LBW or preterm with HOMA-IR was assigned as a weighted mean difference (WMD) with 95% CI. All of the pooled analyses were carried out using the random-effects model because of underlying variations among included studies (30). The I2- and P-value for Q statistic were used to test the heterogeneity between the included studies (I2-values of 0, 25, 50, and 75% represented no, low, moderate, and high heterogeneity, respectively), and P < 0.10 was considered as significant heterogeneity (31). A univariate metaregression analysis was carried out to explore the potential sources of study heterogeneity (32). Subgroup analyses were performed by study design, sex, and continent where the studies were conducted, and the differences between subgroups were calculated using the interaction P-value, which was based on the t-test because of a lower number of included studies (33). An influence analysis was performed with one study removed at a time to assess the stability of the results (34). Publication bias was assessed with a visual inspection of the funnel plot and Egger test (35). The NOS was used to assess the quality of included studies (36).
All statistical analyses were performed using Stata 14.0 (StataCorp, College Station, TX, USA). All reported probabilities (P-values) were two-sided with a statistical significance level of 0.05.
Results
Literature Search and Study Characteristics
The search process is shown in Figure 1. A total of 4,821 articles were identified through the literature search. Three additional articles were found from the reference lists of the included articles. A total of 700 articles were excluded owing to duplicate topics. Moreover, 4,095 articles were excluded after reviewing the titles and abstracts. After reviewing full-text articles, seven articles without OR and/or 95% CI, four articles without relevant outcome, and three reviews were excluded. Finally, 15 published articles with 16 studies were included in this meta-analysis (13–27). All but two studies (20, 27) scored 7 points, and the remaining studies scored 5 or 6 points (Table 1).
Characteristics of Studies
For the association between LBW and the risk of metabolic syndrome, 10 articles (13–16, 18, 21–23, 25, 26) with 11 studies (six cohort studies and five cross-sectional studies) were included, involving 16,693 participants. Among these studies, three were conducted in Asia, five in Europe, and two in South America. Seven studies focused on women and men, two only on men, and two only on women. For the association between premature birth and the risk of metabolic syndrome, five articles (19, 20, 23, 24, 27) with five studies (four cohort studies and one cross-sectional study) were included, involving 7,295 participants. Among these studies, one was conducted in Europe, two in South America, one in North America, and one in Oceania. Four studies focused on women and men, and the remaining one study only on women. All of the included studies reported OR as an effect estimate, excluding the study conducted by Catov et al. (27). The detailed characteristics of the included studies are shown in Table 1.
Quantitative Synthesis
The pooled results of the association of LBW and premature birth with the risk of metabolic syndrome are summarized in Table 2.
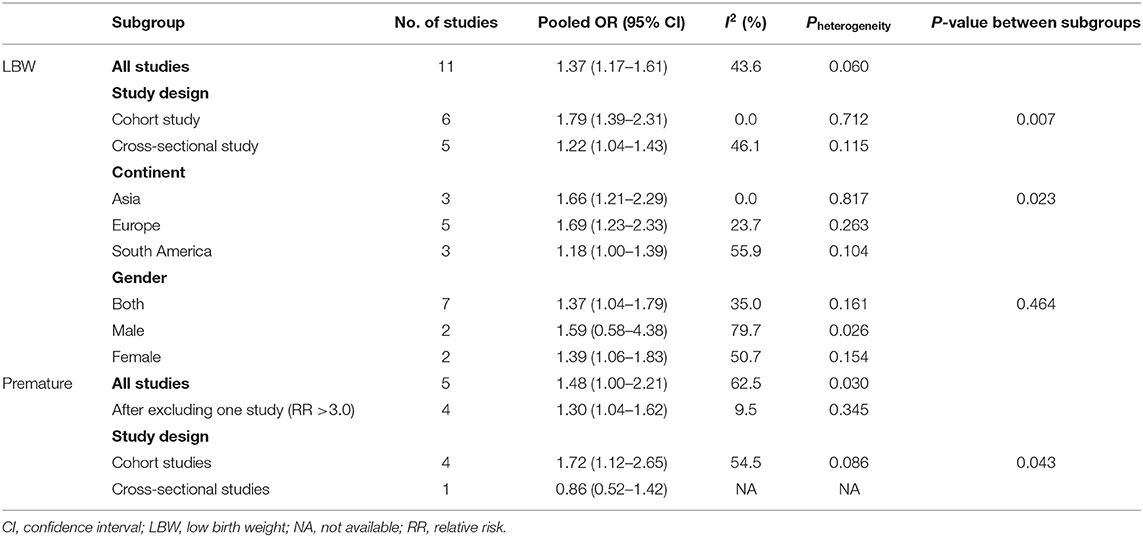
Table 2. Summary risk estimates of the association of LBW and premature with the risk of metabolic syndrome.
The pooled results suggested that LBW (OR = 1.37; 95% CI, 1.17–1.61; I2 = 43.6%; Pheterogeneity = 0.060, Figure 2) was significantly associated with the risk of metabolic syndrome. In a subgroup analysis stratified by the study design, the pooled OR of cohort studies was 1.79 (95% CI, 1.39–2.31), with no evidence of heterogeneity (I2 = 0.0%; Pheterogeneity = 0.712), and the pooled OR of cross-sectional studies was 1.22 (95% CI, 1.04–1.43; I2 = 46.1%; Pheterogeneity = 0.115) (Figure 2). In the subgroup analysis stratified by continent, a significant association was found in every continent: Asia (OR = 1.66; 95% CI, 1.21–2.29; I2 = 0.0%; Pheterogeneity = 0.817), Europe (OR = 1.69; 95% CI, 1.23–2.33; I2 = 23.7%; Pheterogeneity = 0.263), and South America (OR = 1.18; 95% CI, 1.00–1.39; I2 = 55.9%; Pheterogeneity = 0.104). Significant associations were found in studies including men and women (OR = 1.37; 95% CI, 1.04–1.79; I2 = 35.0%; Pheterogeneity = 0.161), as well as women only (OR = 1.39; 95% CI, 1.06–1.83; I2 = 50.7%; Pheterogeneity = 0.154), but not in men (OR = 1.59; 95% CI, 0.58–4.38; I2 = 79.7%; Pheterogeneity = 0.026). The interaction test suggested that the study design and continent biased the association between LBW and metabolic syndrome. The pooled OR showed a non-significant positive association between premature birth and metabolic syndrome (OR = 1.60; 95% CI, 1.00–2.21; I2 = 62.5%; Pheterogeneity = 0.030, Figure 3). In the subgroup analysis stratified by the study design, the pooled OR of cohort studies was 1.72 (95% CI, 1.12–2.65), with reduced heterogeneity (I2 = 54.5%; Pheterogeneity = 0.086). Moreover, the association between preterm and metabolic syndrome differed according to the study design. Finally, the pooled WMD indicated that LBW or preterm was associated with the higher level of HOMA-IR (WMD, 0.28; 95% CI = 0.19–0.36; I2 = 55.6%; Pheterogeneity = 0.061, Figure 4).
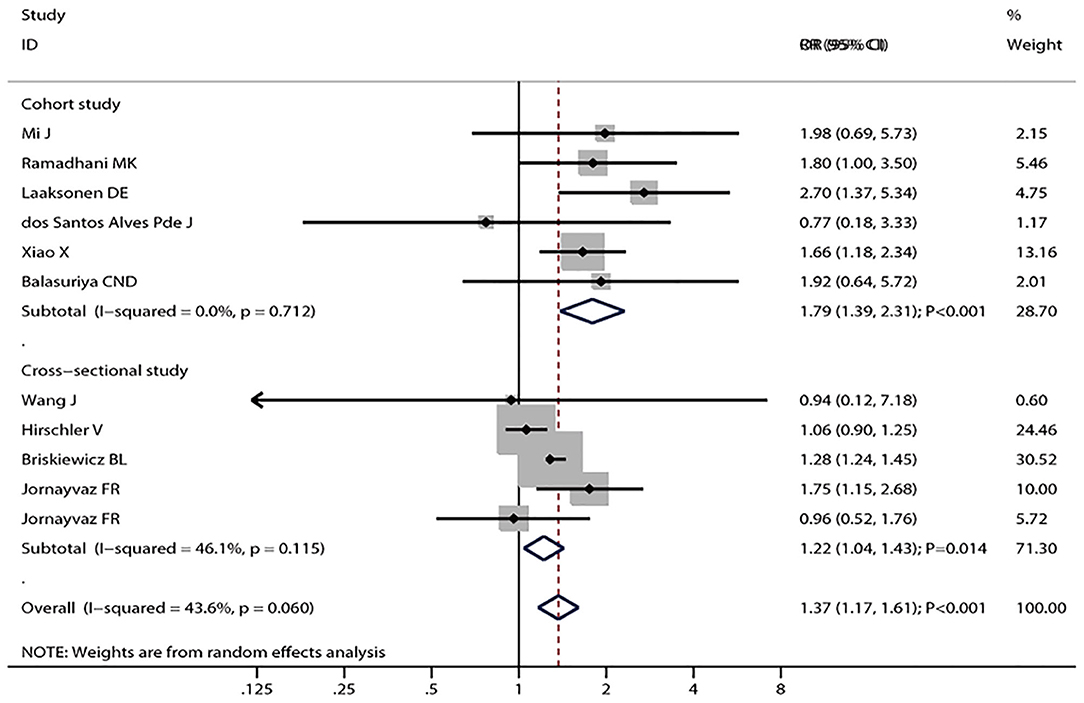
Figure 2. Forest plot of LBW and the risk of metabolic syndrome stratified by the study design. The size of the gray box is positively proportional to the weight assigned to each study, and horizontal lines represent 95% CIs.
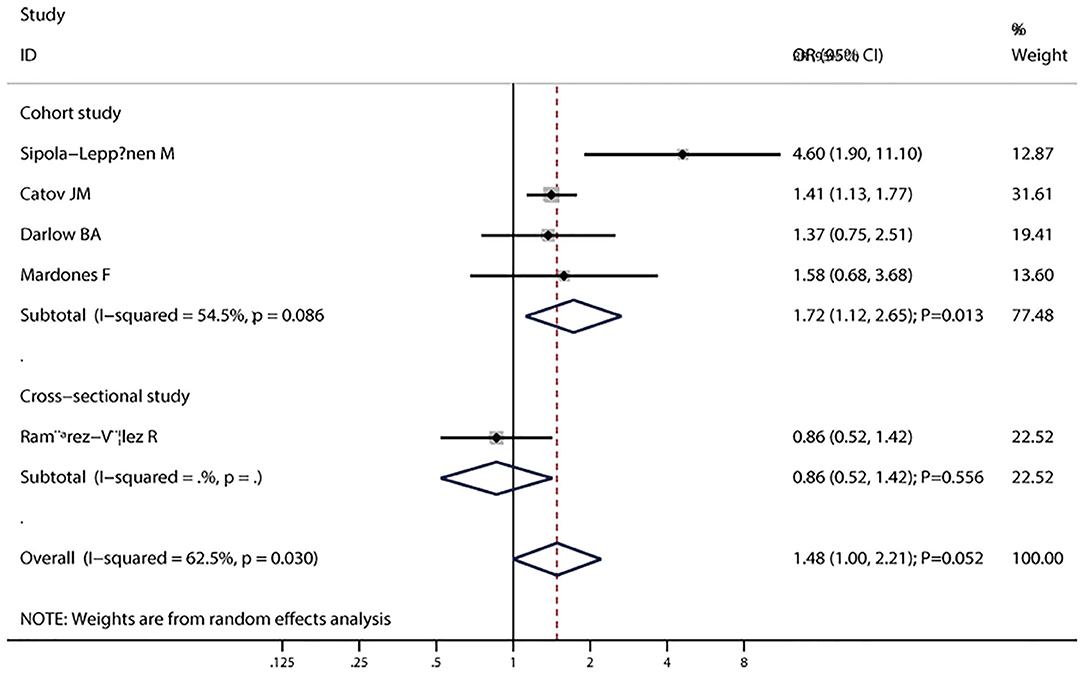
Figure 3. Forest plot of preterm and the risk of metabolic syndrome stratified by the study design. The size of the gray box is positively proportional to the weight assigned to each study, and the horizontal lines represent 95% CIs.
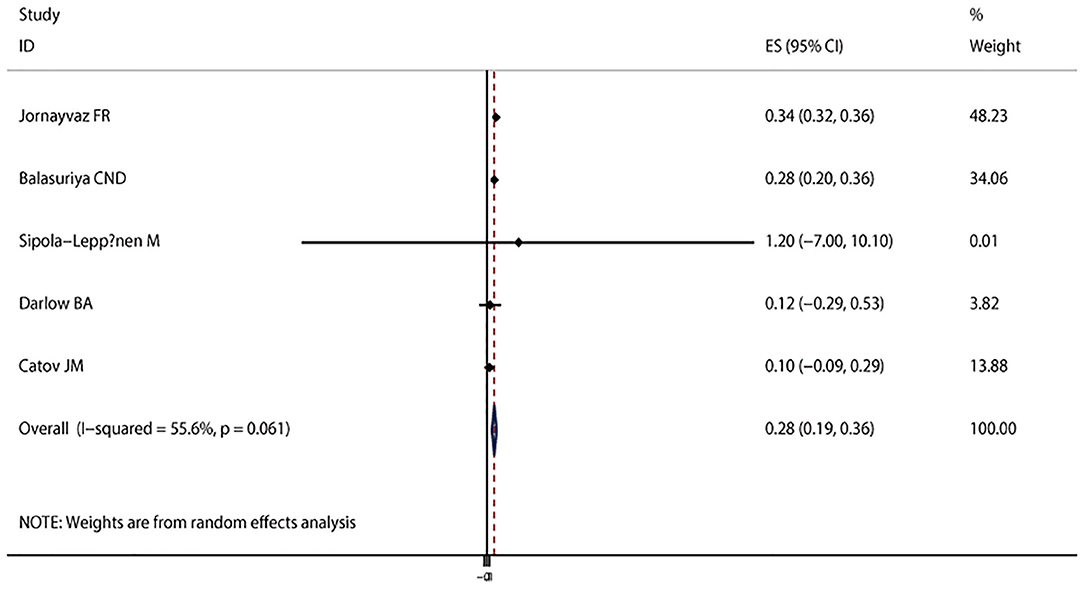
Figure 4. Forest plot of LBW or preterm with the insulin resistance level. The size of the gray box is positively proportional to the weight assigned to each study, and the horizontal lines represent 95% CIs.
Metaregression and Sensitivity Analysis
To explore the sources of between-study heterogeneity, the univariate metaregression analysis was performed with the covariates of study design, sex, and continent where the study was conducted. However, none of these covariates was a potential source of between-study heterogeneity. After excluding one study in the analysis of premature birth and metabolic syndrome (OR > 3.0) (22), which included relatively younger subjects, and few individuals met the definition of metabolic syndrome, the pooled result was changed to significant association (OR = 1.30; 95% CI, 1.04–1.62; I2 = 9.5%, Pheterogeneity = 0.345).
In the influence analysis, the pooled ORs (95% CIs) of the association between LBW and the risk of metabolic syndrome ranged from 1.33 (95% CI, 1.12–1.57) to 1.48 (95% CI, 1.24–1.76). The individual study did not have an excessive influence on the pooled ORs.
Small-Study Effect Evaluation
The visual inspection of the funnel plot (Figure 5) and Egger test (P = 0.286) showed no evidence of significant small-study effect for the association between LBW and the risk of metabolic syndrome. No evidence of significant small-study effect in terms of the association between premature birth and the risk of metabolic syndrome was found (Egger test: P = 0.693).
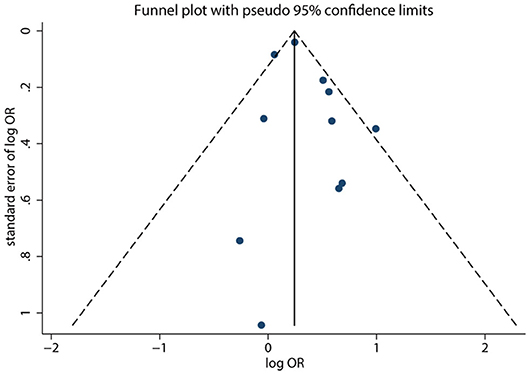
Figure 5. Funnel plot of LBW and the risk of metabolic syndrome. Each dot represents a different study.
Discussion
This meta-analysis assessed the association of LBW and premature birth with the risk of metabolic syndrome. The results of the meta-analysis indicated that LBW might increase the risk of metabolic syndrome. In the subgroup analysis by study design, a significantly positive association was found in both cohort and cross-sectional studies. The pooled ORs did not indicate a statistically significant association between preterm birth and the risk of metabolic syndrome. However, the subgroup analysis by study design indicated that premature birth might be associated with an increased risk of metabolic syndrome in the cohort studies. The pooled WMD suggested a significant association between LBW or preterm and higher HOMA-IR, which is widely used in clinical and epidemiological studies to evaluate insulin sensitivity. Insulin resistance is associated with impaired glucose metabolism, increased vascular resistance, atherogenic dyslipidemia, and adipose tissue dysfunction, even before the onset of type 2 diabetes, atherosclerosis, or hypertension.
A previous meta-analysis that included 27 studies found no significant differences between preterm and term-born for the majority of outcomes associated with the metabolic syndrome, whereas preterm birth was associated with higher blood pressure in adult life (37). Moreover, Markopoulou et al. (38) conducted a meta-analysis on 43 studies and suggested that preterm birth was strongly associated with several components of metabolic syndrome and cardiovascular disease in adult life. However, these two meta-analyses investigated the associations of preterm with the components of the metabolic syndrome (38). In addition, a meta-analysis conducted by Silveira and Horta (39) found LBW was associated with an increased risk of metabolic syndrome in adults, whereas the potential impact of premature on the risk of metabolic syndrome was not illustrated. Moreover, the definition of metabolic syndrome differs owing to this study being based on earlier studies. Therefore, the present meta-analysis was conducted from another angle to evaluate the association of preterm or LBW with the risk of metabolic syndrome.
Several biological mechanisms may explain the association of LBW and premature birth with the risk of metabolic syndrome. Low birth weight and premature infants experience in and ex utero growth restriction (40). The later neonatal overfeeding may lead to rapid weight gain, which may be positively related to overweight and elevated blood glucose level (41). The third trimester of pregnancy is the critical period for the kidneys. The development of kidneys after delivery is accelerated for premature infants, and the glomeruli are morphologically abnormal, leading to the development of hypertension later in life (42). The birth weight reflects the intrauterine nutritional status to some extent. Fetal undernutrition has some effect on liver growth. Impaired liver growth may lead to permanent changes in low-density lipoprotein cholesterol metabolism (43).
Between-study heterogeneity is common and needs to be explored in meta-analyses. Moderate between-study heterogeneities were found in this meta-analysis. However, metaregression with covariates of study design, sex, and continent where the study was conducted did not find the source of between-study heterogeneities. After excluding one study (19) (OR > 3.0) in the analysis of premature birth and the risk of metabolic syndrome, the I2 declined to 9.5%, and the conclusion was changed, suggesting that the conclusion was not robust and needed further verification. Moreover, the definition of LBW and preterm differed across included studies, affecting the net effect estimates between LBW or preterm and the risk of metabolic syndrome.
The present meta-analysis had several strengths. First, it was based on a large sample size, and the findings were more robust than those of any individual study. Second, the positive associations remained when cohort studies were pooled, indicating a potential causal relationship. Third, subgroup analysis was conducted with reduced between-study heterogeneity, suggesting that the results were stable.
However, this meta-analysis also had several limitations. First, the number of studies included was insufficient, especially for the analysis of premature birth. Second, the adjusted confounders differed across included studies, which might play an important role in the risk of metabolic syndrome. Third, the information about gestational age and birth weight was obtained through self-reported questionnaires, and it differed across included studies, thus affecting the progression of metabolic syndrome. Fourth, the data on the gestational age of infants in the LBW groups were not available. Hence, whether LBW in a preterm infant had different associations than LBW in a full-term infant could not be evaluated. Fifth, the prevalence of metabolic syndrome increased with age, and the outcome assessed at various ages might have biased the results. Sixth, the definition of metabolic syndrome differed across included studies, affecting the effect estimates for the association of LBW and preterm with the risk of metabolic syndrome. Finally, the analysis was at the study level, and individual patient data were not available, which restricted more detailed analysis, including the potential interaction impacts of LBW and preterm.
In conclusion, this meta-analysis suggests that LBW might be a risk factor for metabolic syndrome in childhood and adulthood. Further high-quality studies should be conducted to assess the potential interaction impacts of LBW and preterm on the risk of metabolic syndrome.
Data Availability Statement
The original contributions presented in the study are included in the article/supplementary material, further inquiries can be directed to the corresponding author.
Author Contributions
LL, YD, and DZ jointly wrote the article and approved the final. All authors contributed to the article and approved the submitted version.
Funding
This work was supported by the National Natural Science Foundation of China (No. 81300555) and Grants from the Fundamental Research Funds for the Central Universities (No. 2042018kf0082).
Conflict of Interest
The authors declare that the research was conducted in the absence of any commercial or financial relationships that could be construed as a potential conflict of interest.
References
1. Alberti KG, Zimmet P, Shaw J Group IDF Epidemiology Task Force Consensus Group. The metabolic syndrome–a new worldwide definition. Lancet. (2005) 366:1059–62. doi: 10.1016/S0140-6736(05)67402-8
2. Zimmet P, Alberti KG, Kaufman F, Tajima N, Silink M, Arslanian S, et al. The metabolic syndrome in children and adolescents - an IDF consensus report. Pediatr Diabetes. (2007) 8:299–306. doi: 10.1111/j.1399-5448.2007.00271.x
3. Expert Panel on Detection Evaluation Treatment of High Blood Cholesterol in Adults. Executive summary of the third report of the National cholesterol education program (NCEP) expert panel on detection, evaluation, and treatment of high blood Cholesterol in adults (adult treatment panel III). JAMA. (2001) 285:2486–97. doi: 10.1001/jama.285.19.2486
4. Keskin M, Kurtoglu S, Kendirci M, Atabek ME, Yazici C. Homeostasis model assessment is more reliable than the fasting glucose/insulin ratio and quantitative insulin sensitivity check index for assessing insulin resistance among obese children and adolescents. Pediatrics. (2005) 115:e500–3. doi: 10.1542/peds.2004-1921
5. Reaven GM. Banting lecture 1988. Role of insulin resistance in human disease. Diabetes. (1988) 37:1595–607. doi: 10.2337/diabetes.37.12.1595
6. Friend A, Craig L, Turner S. The prevalence of metabolic syndrome in children: a systematic review of the literature. Metab Syndr Relat Disord. (2013) 11:71–80. doi: 10.1089/met.2012.0122
7. Kassi E, Pervanidou P, Kaltsas G, Chrousos G. Metabolic syndrome: definitions and controversies. BMC Med. (2011) 9:48. doi: 10.1186/1741-7015-9-48
8. Wilson PW, D'Agostino RB, Parise H, Sullivan L, Meigs JB. Metabolic syndrome as a precursor of cardiovascular disease and type 2 diabetes mellitus. Circulation. (2005) 112:3066–72. doi: 10.1161/CIRCULATIONAHA.105.539528
9. Esposito K, Chiodini P, Colao A, Lenzi A, Giugliano D. Metabolic syndrome and risk of cancer: a systematic review and meta-analysis. Diabetes Care. (2012) 35:2402–11. doi: 10.2337/dc12-0336
10. Narain A, Kwok CS, Mamas MA. Soft drink intake and the risk of metabolic syndrome: a systematic review and meta-analysis. Int J Clin Pract. (2017) 71:2. doi: 10.1111/ijcp.12927
11. Oliveira RG, Guedes DP. Physical activity, sedentary behavior, cardiorespiratory fitness and metabolic syndrome in adolescents: systematic review and meta-analysis of observational evidence. PLoS ONE. (2016) 11:e0168503. doi: 10.1371/journal.pone.0168503
12. Tang F, Wang G, Lian Y. Association between anxiety and metabolic syndrome: A systematic review and meta-analysis of epidemiological studies. Psychoneuroendocrinology. (2017) 77:112–21. doi: 10.1016/j.psyneuen.2016.11.025
13. Darlow BA, Martin J, Horwood LJ. Metabolic syndrome in very low birth weight young adults and controls: the New Zealand 1986 VLBW study. J Pediatr. (2019) 206:128–33.e5. doi: 10.1016/j.jpeds.2018.10.060
14. de Jesus dos Santos Alves P, Henriques ACPT, Pinto LRM, Mota RMS, Alenchar CHM, Alves RS, et al. Endothelial and metabolic disorders in adolescence: low birth weight is not an isolated risk factor. J Pediatr Endocrinol Metab. (2015) 28:407–13. doi: 10.1515/jpem-2014-0146
15. Hirschler V, Bugna J, Roque M, Gilligan T, Gonzalez C. Does low birth weight predict obesity/overweight and metabolic syndrome in elementary school children? Arch Med Res. (2008) 39:796–802. doi: 10.1016/j.arcmed.2008.08.003
16. Mardones F, Arnaiz P, Pacheco P, Dominguez A, Villarroel L, Eriksson JG, et al. Associations of prenatal growth with metabolic syndrome, insulin resistance, and nutritional status in Chilean children. BioMed Res Int. (2014) 2014:472017. doi: 10.1155/2014/472017
17. Mi J, Cheng H, Zhao XY, Zhang ZK, Ding XY, Hou DQ, et al. Ponderal index at birth predicts metabolic syndrome in mid-aged Chinese. Zhonghua Yu Fang Yi Xue Za Zhi. (2004) 38:221–5.
18. Ramirez-Velez R, Correa-Bautista JE, Villa-Gonzalez E, Martinez-Torres J, Hackney AC, Garcia-Hermoso A. Effects of preterm birth and fetal growth retardation on life-course cardiovascular risk factors among schoolchildren from Colombia: the FUPRECOL study. Early Hum Dev. (2017) 106–7:53–8. doi: 10.1016/j.earlhumdev.2017.02.001
19. Wang J, Zhu Y, Cai L, Jing J, Chen Y, Mai J, et al. Metabolic syndrome and its associated early-life factors in children and adolescents: a cross-sectional study in Guangzhou, China. Public Health Nutr. (2016) 19:1147–54. doi: 10.1017/S1368980015002542
20. Briskiewicz BL, Barreto SM, do Amaral JF, Diniz M, Molina M, Matos SMA, et al. Early-life nutritional status and metabolic syndrome: gender-specific associations from a cross-sectional analysis of the Brazilian longitudinal study of adult health (ELSA-Brasil). Public Health Nutr. (2018) 21:1546–53. doi: 10.1017/S1368980017004256
21. Jornayvaz FR, Vollenweider P, Bochud M, Mooser V, Waeber G, Marques-Vidal P. Low birth weight leads to obesity, diabetes and increased leptin levels in adults: the CoLaus study. Cardiovasc Diabetol. (2016) 15:73. doi: 10.1186/s12933-016-0389-2
22. Laaksonen DE, Lakka HM, Lynch J, Lakka TA, Niskanen L, Rauramaa R, et al. Cardiorespiratory fitness and vigorous leisure-time physical activity modify the association of small size at birth with the metabolic syndrome. Diabetes Care. (2003) 26:2156–64. doi: 10.2337/diacare.26.7.2156
23. Ramadhani MK, Grobbee DE, Bots ML, Castro Cabezas M, Vos LE, Oren A, et al. Lower birth weight predicts metabolic syndrome in young adults: the atherosclerosis risk in young adults (ARYA)-study. Atherosclerosis. (2006) 184:21–7. doi: 10.1016/j.atherosclerosis.2005.03.022
24. Xiao X, Zhang ZX, Li WH, Feng K, Sun Q, Cohen HJ, et al. Low birth weight is associated with components of the metabolic syndrome. Metabolism. (2010) 59:1282–6. doi: 10.1016/j.metabol.2009.12.001
25. Sipola-Leppanen M, Vaarasmaki M, Tikanmaki M, Matinolli HM, Miettola S, Hovi P, et al. Cardiometabolic risk factors in young adults who were born preterm. Am J Epidemiol. (2015) 181:861–73. doi: 10.1093/aje/kwu443
26. Balasuriya CND, Stunes AK, Mosti MP, Schei B, Indredavik MS, Hals IK, et al. Metabolic outcomes in adults born preterm with very low birthweight or small for gestational age at term: a cohort study. J Clin Endocrinol Metab. (2018) 103:4437–46. doi: 10.1210/jc.2018-00464
27. Catov JM, Althouse AD, Lewis CE, Harville EW, Gunderson EP. Preterm delivery and metabolic syndrome in women followed from prepregnancy through 25 years later. Obstetr Gynecol. (2016) 127:1127–34. doi: 10.1097/AOG.0000000000001434
28. Moher D, Liberati A, Tetzlaff J, Altman DG, PRISMA Group. Preferred reporting items for systematic reviews and meta-analyses: the PRISMA statement. PLoS Med. (2009) 6:e1000097. doi: 10.1371/journal.pmed.1000097
29. Stang A. Critical evaluation of the Newcastle-Ottawa scale for the assessment of the quality of nonrandomized studies in meta-analyses. Eur J Epidemiol. (2010) 25:603–5. doi: 10.1007/s10654-010-9491-z
30. Ades AE, Lu G, Higgins JP. The interpretation of random-effects meta-analysis in decision models. Med Decis Making. (2005) 25:646–54. doi: 10.1177/0272989X05282643
31. Higgins JP, Thompson SG, Deeks JJ, Altman DG. Measuring inconsistency in meta-analyses. BMJ. (2003) 327:557–60. doi: 10.1136/bmj.327.7414.557
32. Thompson SG, Higgins JP. How should meta-regression analyses be undertaken and interpreted? Stat Med. (2002) 21:1559–73. doi: 10.1002/sim.1187
33. Altman DG, Bland JM. Interaction revisited: the difference between two estimates. BMJ. (2003) 326:219. doi: 10.1136/bmj.326.7382.219
34. Tobias A. Assessing the influence of a single study in meta-analysis. Stata Tech Bull. (1999) 47:15–7.
35. Egger M, Davey Smith G, Schneider M, Minder C. Bias in meta-analysis detected by a simple, graphical test. BMJ. (1997) 315:629–34. doi: 10.1136/bmj.315.7109.629
36. Wells G. The Newcastle-Ottawa Scale (NOS) for assessing the quality of nonrandomised studies in meta-analyses. (2019). Available online at: https://www.researchgate.net/publication/288802810_The_Newcastle-Ottawa_Scale_NOS_for_Assessing_The_Quality_of_Nonrandomised_Studies_in_Meta-analyses
37. Parkinson JR, Hyde MJ, Gale C, Santhakumaran S, Modi N. Preterm birth and the metabolic syndrome in adult life: a systematic review and meta-analysis. Pediatrics. (2013) 131:e1240–63. doi: 10.1542/peds.2012-2177
38. Markopoulou P, Papanikolaou E, Analytis A, Zoumakis E, Siahanidou T. Preterm birth as a risk factor for metabolic syndrome and cardiovascular disease in adult life: a systematic review and meta-analysis. J Pediatr. (2019) 210:69–80.e5. doi: 10.1016/j.jpeds.2019.02.041
39. Silveira VM, Horta BL. Birth weight and metabolic syndrome in adults: meta-analysis. Rev Saude Publ. (2008) 42:10–8. doi: 10.1590/S0034-89102008000100002
40. Tinnion R, Gillone J, Cheetham T, Embleton N. Preterm birth and subsequent insulin sensitivity: a systematic review. Arch Dis Childhood. (2014) 99:362–8. doi: 10.1136/archdischild-2013-304615
41. Stettler N, Stallings VA, Troxel AB, Zhao J, Schinnar R, Nelson SE, et al. Weight gain in the first week of life and overweight in adulthood: a cohort study of European American subjects fed infant formula. Circulation. (2005) 111:1897–903. doi: 10.1161/01.CIR.0000161797.67671.A7
42. Sutherland MR, Gubhaju L, Moore L, Kent AL, Dahlstrom JE, Horne RS, et al. Accelerated maturation and abnormal morphology in the preterm neonatal kidney. J Am Soc Nephrol. (2011) 22:1365–74. doi: 10.1681/ASN.2010121266
Keywords: low birth weight, meta-analysis, metabolic syndrome, premature birth, preterm
Citation: Liao L, Deng Y and Zhao D (2020) Association of Low Birth Weight and Premature Birth With the Risk of Metabolic Syndrome: A Meta-Analysis. Front. Pediatr. 8:405. doi: 10.3389/fped.2020.00405
Received: 18 March 2020; Accepted: 12 June 2020;
Published: 28 July 2020.
Edited by:
Offer Erez, Soroka Medical Center, IsraelReviewed by:
Kazumichi Fujioka, Kobe University, JapanMaryAnn Volpe, Tufts University School of Medicine, United States
Copyright © 2020 Liao, Deng and Zhao. This is an open-access article distributed under the terms of the Creative Commons Attribution License (CC BY). The use, distribution or reproduction in other forums is permitted, provided the original author(s) and the copyright owner(s) are credited and that the original publication in this journal is cited, in accordance with accepted academic practice. No use, distribution or reproduction is permitted which does not comply with these terms.
*Correspondence: Dongchi Zhao, zhaodongchi@znhospital.cn