- 1School and Graduate Institute of Physical Therapy, College of Medicine, National Taiwan University, Taipei, Taiwan
- 2Department of Pediatrics, National Taiwan University Hospital, Taipei, Taiwan
- 3Department of Pediatrics, National Cheng Kung University Hospital, Tainan, Taiwan
- 4Centers of Genomic and Precision Medicine, National Taiwan University, Taipei, Taiwan
- 5Institute of Epidemiology and Preventive Medicine, College of Public Health, National Taiwan University, Taipei, Taiwan
- 6Research Center for Genes, Environment and Human Health, National Taiwan University, Taipei, Taiwan
- 7Department of Psychiatry, National Taiwan University Hospital and College of Medicine, National Taiwan University, Taipei, Taiwan
- 8Physical Therapy Center, National Taiwan University, Taipei, Taiwan
This study aimed to examine the association of dopamine-related genes with mental and motor development and the gene-environment interaction in preterm and term children. A total of 201 preterm and 111 term children were examined for their development at 6, 12, 18, 24, and 36 months and were genotyped for 15 single-nucleotide polymorphisms (SNPs) in dopamine-related genes (DRD2, DRD3, DAT1, COMT, and MAOA). An independent sample of 256 preterm children was used for replication. Since the developmental age trends of preterm children differed from those of term children, the analyses were stratified by prematurity. Among the 8 SNPs on the MAOA gene examined in the whole learning sample, the results of linkage disequilibrium analysis indicated that they were located in one block (all D′ > 0.9), and rs2239448 was chosen as the tag (r2 > 0.85). In the analysis of individual SNPs in each dopamine-related gene, the tag SNP (rs2239448) in MAOA remained significantly associated with the mental scores of preterm children for the interaction with age trend (p < 0.0001; largest effect size of 0.65 at 24 months) after Bonferroni correction for multiple testing. Similar findings for rs2239448 were replicated in the independent sample (p = 0.026). However, none of the SNPs were associated with the motor scores of preterm children, and none were related to the mental or motor scores of term children. The genetic variants of the MAOA gene exert influence on mental development throughout early childhood for preterm, but not term, children.
Introduction
Preterm children with very low birth weight (VLBW, birth body weight <1,500 g) face an elevated risk of neurodevelopmental impairments throughout childhood (1–3). Both genetic and environmental factors may contribute to these developmental consequences (4, 5). Recent evidence has shown that the association of preterm birth with severe brain structural abnormalities, congenital disease (6, 7), and developmental problems (8) might be accounted for by a common genetic background. The dopamine system (DA) is a major neurotransmitter in the extrapyramidal system of the brain that involves motor control, endocrine function, cognition, reward system and behavior (9). Dopamine-related genes have long been implicated in child development (9, 10). Certain single nucleotide polymorphisms (SNPs) of dopamine-related genes (e.g., dopamine receptor 2 [DRD2] and DRD3 for DA release, dopamine transporter [DAT] for DA clearance, monoamine oxidase A [MAOA] and catechol-O-methyltransferase [COMT] for DA degradation) have been associated with neurodevelopmental disorders in children. Specifically, several SNPs in DAT1 (rs27072 and rs2550948) and MAOA (i.e., rs12843268, rs2072744, rs5905859, rs3027400, rs2235186, rs2235185, rs2239448, and rs3027407) have been associated with attention deficit hyperactivity disorder (ADHD) (11–16); some SNPs in DRD2 (rs1800497) and DRD3 (rs167771) have been related to child affective problems, obesity, working memory impairment, or autism spectrum disorders (ASD) (17–22); and some SNPs in COMT (rs4818, rs4680, and rs2075507) have been associated with ASD, aggressive behavior, verbal inhibition and poor working memory in school-aged children (23–25).
A challenge in research on child development is how to incorporate longitudinal data from repeated measurements (26). To date, few studies on the role of dopamine-related genes have adopted a longitudinal view that incorporates repeated measurements of development. Furthermore, existing studies have mainly been conducted among Caucasian populations on more severe developmental morbidities. Whether dopamine-related genes are involved in more common forms of developmental variation in preterm or term children, particularly among non-Caucasian populations, remains poorly understood.
To address the gap in the literature, we turned to a prospective follow-up study of preterm children and their term counterparts. The specific aims of this study were to (1) examine the association of dopamine-related genes with mental and motor development in preterm children with VLBW and term children with normal birth weight at 6, 12, 18, 24, and 36 months of age, (2) examine whether environmental factors (preterm/term birth) interact with dopamine-related genes in children's mental and motor development, and (3) replicate the findings in an independent sample of children.
Materials and Methods
Participants
The participants of the learning sample consisted of 201 preterm children with VLBW and 111 term children who were born in or admitted to three hospitals in northern Taiwan during the time periods of 1995–1997 (Cohort I), 2002–2004 (Cohort II), and 2006–2008 (Cohort III). Meanwhile, the replication sample had 256 preterm children with VLBW recruited during the time period of 2012–2014 (Cohort IV) from northern and southern Taiwan. More detailed descriptions of the recruitment are provided in the Supplementary Methods and Figure S1. Briefly, the inclusion criteria for VLBW preterm children from all cohorts were birth weight <1,500 g, gestational age <37 weeks, and the absence of congenital abnormalities and severe neonatal diseases. The selection criteria for term children included gestational age within 38–42 weeks, birth weight ≥ 2,500 g, and the absence of congenital abnormality and perinatal disease. All mothers were Taiwanese citizens, were over 18 years old, and had no history of psychiatric disorders or drug or alcohol abuse. All of the participants were from different families.
This study was approved by the Research Ethics Committee of National Taiwan University Hospital and the Institutional Review Board of National Cheng Kung University Hospital, and written informed consent was obtained from parents. Both the Cohort I and II studies were observational studies, while the Cohort III and IV studies were randomized controlled trials (URL: https://clinicaltrials.gov/, identifiers: NCT00173108 and NCT00946244 for Cohort III, and NCT01807533 for Cohort IV, National Taiwan University Hospital). Preterm children in Cohorts III and IV were randomly assigned to the intervention group [clinical-based intervention group and home-based intervention group in Cohort III (27); the family-centered intervention group in Cohort IV (28)] and the usual-care group (Cohorts III and IV). Children in the intervention group received 5 in-hospital and 7 after-discharge intervention sessions from hospitalization to 12 months of corrected age that emphasized environmental modulation, feeding support, massage, child developmental skills, parental support and education, and dyadic interaction activities. Children in the usual-care group received standard care. Randomizations were computer-generated and stratified by gestational age and hospital, with the sequence kept in a locked file and concealed from the parents, medical staff, and outcome examiners. However, the parents and the intervention providers were aware of group allocation. All methods were performed in accordance with the relevant guidelines and regulations.
Measurements
Children in Cohorts I to III had their perinatal and demographic data collected via chart review and parental interviews, and their developmental outcomes were evaluated at 6, 12, 18, 24, and 36 months of age using the Bayley Scales of Infant Development−2nd Edition (BSID-II) (29). Because the BSID-II was revised into the Bayley Scales of Infant and Toddler Development−3rd Edition (Bayley-III) (30) in 2006, both versions were administered to children in Cohort III at five time points (6, 12, 18, 24, and 36 months). For children in Cohort IV, the Bayley-III was administered at four time points (6, 12, 24, and 36 months), and the BSID-II was additionally administered at two time points (24 and 36 months). Because the replication sample had no BSID-II scores at 6 and 12 months, their Bayley-III scores at these ages were transformed into BSID-II scores using a linear regression procedure (31) (details in Supplementary Methods).
Genetic Analyses
A buccal cell sample was collected from the cheeks of each child using the Catch-All swabs of the BuccalAmp™ DNA Extraction Kit (Epicenter Biotechnologies, WI, USA). Genomic DNA was extracted from the buccal cell samples following the standard protocol of a commercial kit, the QIAamp Mini Kit (Qiagen, Chatsworth, CA, USA). A total of 15 SNP markers, including 1 in DRD2 (rs1800497) (19), 1 in DRD3 (rs167771) (17), 2 in DAT1 (rs27072 and rs2550948) (12), 3 in COMT (rs4818, rs4680; and rs2075507) (23, 24), and 8 in MAOA (i.e., rs12843268, rs2072744, rs5905859, rs3027400, rs2235186, rs2235185, rs2239448, and rs3027407) (13), were selected for genotyping using TaqMan analysis and a 7900HT Fast Real-Time PCR System (Applied Biosystems, Foster City, CA, USA). Quality control of the genotyping included duplications, a negative control, and a call rate > 95%. Although there is a well-known variant number of tandem repeats on MAOA (32), we did not include it in this study, since its genotyping requires different designs and sophisticated quality control.
None of the genotypes for any of the 15 markers showed significant deviation from Hardy-Weinberg equilibrium, as calculated using PLINK (33) (version 1.07, http://pngu.mgh.harvard.edu/purcell/plink/), with p > 0.001 defined as significant departure in either preterm or term children. The MAOA gene was examined in females only because males are hemizygous at X-linked loci.
Statistical Analysis
Both the Cohort III and IV were superiority randomized controlled trials. Based on the cognitive and motor development for preterm children for a statistical power of 80%, with an attrition rate of 20% and an alpha level of 0.05, the estimated sample size in the Cohort III study was 43 in each group of preterm children (34). For the Cohort IV study, for a power of 80% with a 20% attrition and an alpha level of 0.05, the estimated sample size in each group was 78 for the 24-month cognitive outcome and 115 for the 24-month motor outcome (27).
We used the QUANTO software (version 1.2.4, http://hydra.usc.edu/gxe) to estimate the overall sample size for the investigation of gene-environment interaction effects in both learning sample and replication sample. To achieve a power of 80%, with continuous outcome and independent individuals design, and an effect size as reported by Babineau et al. (35) for the interaction effect of 5-HTTLPR gene and environmental factors on child behavior scores (R2 = 0.04 to 0.06, from 3 to 36 months of age) as well as an attrition rate of 20%, an estimated sample size of 153 to 231 children were required.
The power analysis of our existing sample size of 111 term children and 201 preterm children with an alpha level at 0.05 using G*Power software (version 3.1.9.4, Germany) indicated a power of 60–100% for an effect size ranging from 0.11 to 0.65, derived from the standardized mean differences in mental score between the two genotype groups of rs2239448 at 6, 12, 18, 24, and 36 months of age.
Group comparisons were conducted using analysis of variance for continuous variables and chi-square tests for categorical variables. The alpha level was set at 0.05. Because some homozygous genotypes had a small number of individuals for certain SNPs, we merged two adjacent genotypes to adopt a dominant or recessive model as indicated. The pattern of linkage disequilibrium (LD) was examined using Haploview (36).
The relations of the genotypes of individual SNPs to mental and motor raw scores were first examined in the learning sample. We started with a PROC GLIMMIX model with main effects of preterm birth, genotype, and age trend (measured at 5 time points) and their pairwise two-way interactions and three-way interaction with a random intercept and adjustment for covariates, including cohort sources, intervention (yes or no), and sex. Analysis of preterm children's data was additionally adjusted for the effect of gestational age. The cohort differences in developmental scores are described in more detail in Table S1. An unstructured covariance structure was selected for the repeated observations based on the Akaike information criterion and the Bayesian information criterion (37). The significance level of the p-value obtained from the learning sample was adjusted according to the Bonferroni correction (38, 39). In correcting for multiple testing, we considered the p-values of the main effect variables and the interactions together. If the three-way interaction was not significant, the model was refitted by deleting the interaction term from the model. Then, the correction for multiple testing was conducted again for this refitted model without the three-way interaction. Under this circumstance, we conducted stratified analyses to examine the effect of age trend, genotype, and their interaction separately for preterm and term children, with correction for multiple testing within each stratum.
To examine the cumulative effect of significant genetic markers on developmental scores, we used the regression coefficients from mixed-effects models as weights to generate a summarized genetic risk score. The scores were compared using mixed-effects models of longitudinal data at five time points to examine whether the cumulative effects existed or not. Similar correction for multiple testing was conducted for this part of the analysis using the Bonferroni correction (38, 39) by considering the p-values of the main effect variables and the interactions together. All statistical analyses were conducted using Statistical Analysis Software (SAS, version 9.3, SAS Institute, Cary, NC, USA).
Results
The birth and demographic characteristics of the participating children are presented in Table 1. In the learning sample, preterm children had lower gestational age, birth body weight, parental education, and developmental scores and were more likely to be in Cohort III than were term children (all p < 0.05). The results of LD analysis for the 8 SNPs of the MAOA in the whole learning sample indicated that they were located in one block (all D′> 0.9) (Figure S2), and rs2239448 was therefore chosen as the tag (r2 > 0.85).
The distributions of the 8 SNPs (rs2239448 as the tag of MAOA) genotyped in this study are displayed in Table S2. We adopted a dominant model for the designated allele 1, which was determined by collapsing two adjacent genotypes if their distributions were closer than the remaining genotypes or one homozygous group having a very small number (one in DRD3 and two in DAT1).
Effects of Genetic Factors and Prematurity on Longitudinal Child Development
The results of the mixed-effects model analysis using a full model for the mental score with Bonferroni correction for 56 p-values are displayed in Table S3. Because the three-way interaction was not significant in each of the 8 SNPs, we then removed the three-way interaction and refitted the model (Table S4). Regarding the main effect, age trend and preterm birth were highly significant even after Bonferroni correction for 48 p-values, and genotype was not. In terms of interaction, the preterm × age trend was significant for all SNPs, but both the genotype × age trend and genotype × preterm interactions were significant only for the MAOA rs2239448, though the latter was not significant after Bonferroni correction. One possible reason for not reaching significance for the genotype × preterm interaction is the relatively small sample size for the term group (N = 111) vs. the preterm group (N = 201).
Because there was no three-way interaction and the sample was recruited separately for preterm and term children, we then conducted stratified analyses by preterm birth to examine the effect of age trend, genotype, and their interaction. For the stratum of preterm children's mental score (Table 2), the effect of age trend was significant for all SNPs, but the effect of genotype and genotype × age trend were significant only for the MAOA rs2239448 after Bonferroni correction for 24 p-values and adjustment for the effect of gestational age, cohort sources, intervention and sex. For illustration, the mean differences between two genotype groups at the five time points are also displayed for each SNP in Table 2 (more detailed mental score distributions are presented in Table S5). Of note, MAOA rs2239448 showed the smallest genotype effect (p = 0.0032) and genotype × age trend interaction effect (p < 0.0001), yet only the latter remained statistically significant after Bonferroni correction. The effect size for MAOA rs2239448 (i.e., mean differences between the two genotype groups divided by the standard deviation of preterm children) in relation to the mental score was 0.11, 0.27, 0.51, 0.65, and 0.39 at 6, 12, 18, 24, and 36 months of age, respectively.
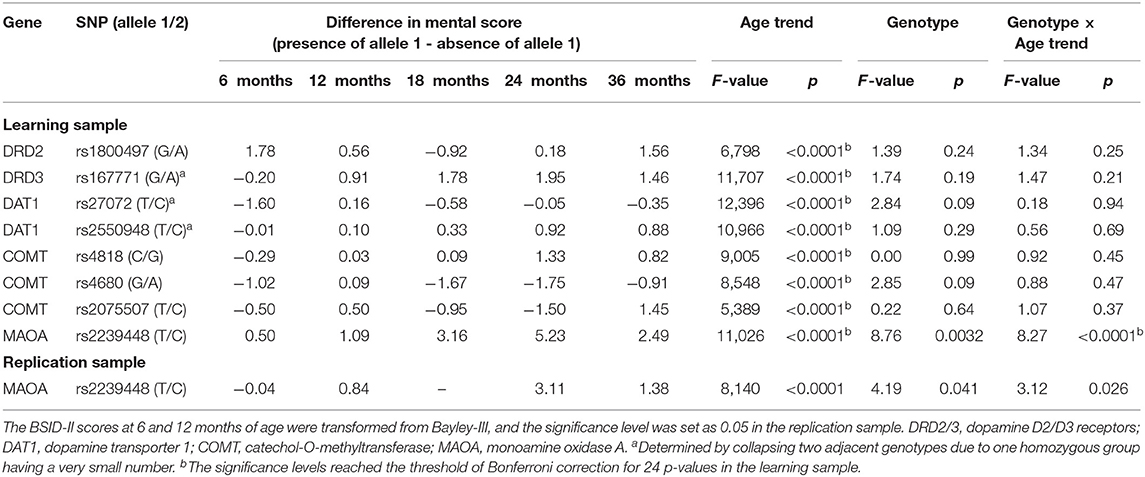
Table 2. Relations of genotypes with the mental raw scores at 6, 12, 18, 24, and 36 months of age in preterm children (cohort, intervention, sex, and gestational age are treated as covariates).
For the stratum of term children's mental scores (Table S6), the effect of age trend was significant for all SNPs, but the SNPs showed neither genotype nor genotype × age trend effects after Bonferroni correction for 24 p-values.
For the motor scores, we first used a full model containing the three main variables, all of their possible interactions, and covariates (Table S7). Regarding the main effect, age trend and preterm birth were significant, while genotype was not. In terms of interactions, the preterm × age trend was significant, which was also expected according to a previous study (26), but the genotype × age trend and genotype × preterm interactions and the three-way interaction were not significant. Even if we refitted the model without the three-way or two-way interactions, the results still indicated that only preterm, age trend, and preterm × age trend were significant.
Replicating the Finding in MAOA
To examine the robustness of the influence of MAOA rs2239448 and its interaction with age trend on the mental scores of preterm children, we further genotyped this SNP in an independent sample of 256 preterm children. MAOA rs2239448 had a significant main effect (p = 0.041) as well as a significant interaction effect with age trend (p = 0.026) (Table 2, bottom row). For MAOA rs2239448 in this replication sample, its effect size with the mental score was −0.01, 0.34, 0.43, and 0.18 at 6, 12, 24, and 36 months of age, respectively. Of note, the SD at 12 months of age (2.5) was derived from the Bayley-III, whereas the developmental data at 36 months of age (7.7) was derived from the BSID-II, resulting in a larger effect size for the former even though it had a smaller group difference than the latter.
To summarize the findings for MAOA rs2239448, the mean mental scores at five time points for the two genotype groups (presence of allele 1 vs. absence of allele 1) are depicted separately for the preterm children in the learning sample (Figure 1A), the term children in the learning sample (Figure 1B), and the preterm children in the replication sample (Figure 1C).
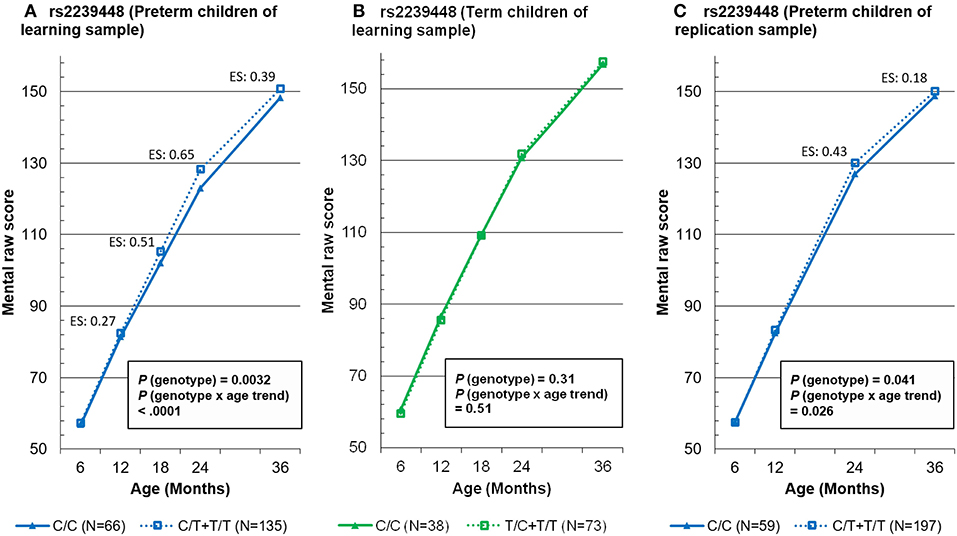
Figure 1. Mixed-effects model-based mental raw score at 5 time points by the genotypes of MAOA tag rs2239448 in preterm and term children in the learning sample (A,B, respectively) and in preterm children in the replication sample (C). ES, effect size.
Then, the most significant MAOA rs2239448 was combined sequentially with seven other markers according to the ascending order of p-value, and the cumulative genetic dose was subjected to mixed-effects model analysis after Bonferroni correction for 56 p-values (Table S8). Nevertheless, the combination of rs2239448 with other SNPs did not lead to any increase in the effect estimates regarding genotype, i.e., the main effect of genotype, genotype × age trend, genotype × preterm, and genotype × preterm × age trend.
Discussion
In this prospective study, we evaluated the potential influence of 15 dopamine-related genetic variants on developmental scores using mixed-effects models of longitudinal data to examine three main-effects variables (preterm, genotype, and age trend) and their possible interactions. After removing the three-way interaction due to its lack of statistical significance, the three two-way interactions were significant for the MAOA variant. After stratification by preterm birth, the effect of the genotype × age trend remained significant only for preterm children's mental scores for the variant of MAOA rs2239448 after Bonferroni correction. Furthermore, the influence of MAOA rs2239448 (genotype main effect and genotype × age trend interaction) in preterm children was successfully replicated in an independent sample. Nevertheless, a combination of MAOA rs2239448 with the SNPs of other dopamine-related genes failed to improve the association. These findings provide insightful information on the gene (MAOA rs2239448) × environment (preterm birth) interaction regarding the age trend of mental development.
As expected, preterm children demonstrated persistently lower mental and motor scores than their term peers did from 6 to 36 months of age. Such results were in line with previous findings indicating that preterm children showed poorer motor and mental development than their term counterparts did in their follow-up to 24 months of age (27).
Among the variants of dopamine-related genes examined, only those of MAOA exhibited an association with the mental scores of preterm children at different ages, with a small to medium effect size in the learning sample and a small effect size in the replication sample. For example, the preterm children with two genotypes on the MAOA rs2239448 had the largest difference in mental scores of 5.23 (i.e., a medium effect size of 0.65) in the learning sample and of 3.11 (i.e., a small effect size of 0.43) in the replication sample at 24 months of age. Although these MAOA variants have been found to be associated with some childhood-onset mental disorders, including ADHD (13) and ASD (40), this study is the first to demonstrate that the MAOA variants exert influence on the mental development of preterm children, providing further support for the role of MAOA implicated in prior animal studies (41).
On the other hand, our findings showed no association of dopamine-related genes with mental development in term children. One possibility is that the dopamine-related brain functions of term children have less variation than those of preterm children (42, 43). Hence, many previous studies have consistently reported no association between variants of dopamine-related genes and cognition in healthy children and adolescents (44, 45). In contrast, some prior studies found that the influence of dopamine-related genes could be detected only under certain environmental contexts, for example, the influence of MAOA on antisocial problems moderated by childhood maltreatment (46, 47), the influence of 5-HTT on dysregulation in children moderated by prenatal depression in mothers (35), and the influence of 5-HTT behavioral development in children moderated by child care quality (48). Therefore, the effect of dopamine-related genes on mental development may be very small unless the children underwent an adverse developmental environment, e.g., preterm birth in this study. Thus, our findings implied that preterm children are more vulnerable to the influence of MAOA on mental development.
A combination of the MAOA rs2239448 with the SNPs of other dopamine-related genes in this study did not lead to a stronger association with mental development in preterm children, as indicated in a previous study (49). One possibility is that our chosen genetic variants of other dopamine-related genes were mainly based on Caucasian populations and not on the most informative alleles in our study population.
Our failure to find any association of the variants of dopamine-related genes with motor development in preterm and term children is, to some extent, not surprising, given that our target markers were chosen based on studies focusing on childhood-onset diseases such as ADHD and ASDs. Future studies on child motor development need to consider genetic markers that may have associations with other motor-related diseases or functions.
Our findings indicate that prematurity in children with the absence of the T allele of MAOA rs2239448 is associated with slower mental development. In practice, the MAOA rs2239448 may be used to identify preterm children who are vulnerable and can benefit from early intervention to help them overcome the slowing pace of mental development. It is also warranted to investigate whether the MAOA variants moderate the effect of intervention in preterm children and help develop personalized interventions for preterm children.
This study has the following strengths: (1) the incorporation of a longitudinal design with follow-ups at 6, 12, 18, 24, and 36 months on both the mental and motor development of preterm and term children and (2) the successful replication of the robust results in an independent sample of preterm children. However, several limitations are important to note. First, because preterm children with severe brain damage or neonatal diseases were excluded from this study, our findings may not be generalizable to those with more severe developmental disorders. Second, this study consisted of participants from several cohorts established in different years. While clinical practice and environmental exposure might vary across time, we treated cohort membership as a covariate to control for potential confounding effects of birth year. Some information was not collected for some cohorts, such as socioeconomic status for Cohort I and Cohort II. The failure to include socioeconomic status as a covariate in our analysis might render our adjustment for potential confounders inadequate. Third, our study focused only on certain candidate SNPs selected from previous genome-wide association studies. Future research may explore the MAOA variable number of tandem repeats for more genetic information. Finally, the BSID-II scores at both 6 and 12 months of age in the replication sample of Cohort IV were transformed from the Bayley-III scores via regression modeling. This might not overcome the difference between the two, e.g., a smaller standard deviation of the Bayley-III scores at the age of 12 months than its counterparts of BSID-II scores.
In conclusion, this prospective study of preterm and term children demonstrated that the MAOA rs2239448 variants were significantly associated with the mental scores of preterm children for the main effect and its interaction with the age trend. Similar findings for MAOA rs2239448 were replicated in an independent sample of preterm children. However, none of the SNPs were associated with the motor scores of preterm children, and none were related to the mental or motor scores of term children. Our results have shed new light on the genetic influences of MAOA on mental development in preterm children and have implications for intervention.
Data Availability Statement
The raw data supporting the conclusions of this article will be made available by the authors, without undue reservation, to any qualified researcher.
Ethics Statement
The studies involving human participants were reviewed and approved by the Ethics Committee of National Taiwan University Hospital (Identifier on ClinicalTrials.gov: NCT00173108, NCT00946244, and NCT01807533). Written informed consent to participate in this study was provided by the participants' legal guardian/next of kin.
Author Contributions
N-JY conceptualized and designed the medical data collection and analysis procedures, contributed to child developmental data collection, carried out the genetic data collection and genotyping experiment, and drafted the initial manuscript. W-SH and C-HL supervised case enrollment and medical data collection in two different hospitals. C-IT carried out the DNA extraction and assisted genotyping experiment. W-YL and P-HK contributed to the statistical analysis and interpretation of the data. Y-TY helped with child developmental data collection. WC supervised the genotyping experiment and statistical analysis, contributed to the interpretation of data, and revised the manuscript critically. S-FJ designed the study, procured the grant support, coordinated all data collection of all the hospitals, supervised the child developmental data collection, and critically revised the manuscript. All authors reviewed and approved the manuscript.
Funding
All phases of this study were supported by the National Health Research Institute (Grant NHRI-EX101-10106PI); the Ministry of Science and Technology (Grants 98-2314-B-002-010-MY3, 105-2314-B-002-017, and 107-2314-B-002-042-MY3); and the National Taiwan University Hospital (Grant NTUH-98-S-1085) in Taiwan.
Conflict of Interest
The authors declare that the research was conducted in the absence of any commercial or financial relationships that could be construed as a potential conflict of interest.
Acknowledgments
We thank the children and their parents for participating in the study, and we thank the staff of the neonatal intensive care unit at the National Taiwan University Hospital, MacKay Memorial Hospital, National Cheng Kung University Hospital, and Taipei City Hospital Branch for Women and Children for their assistance with patient recruitment.
Supplementary Material
The Supplementary Material for this article can be found online at: https://www.frontiersin.org/articles/10.3389/fped.2020.00092/full#supplementary-material
References
1. Mulder H, Pitchford NJ, Hagger MS, Marlow N. Development of executive function and attention in preterm children: a systematic review. Dev Neuropsychol. (2009) 34:393–421. doi: 10.1080/87565640902964524
2. Williams J, Lee KJ, Anderson PJ. Prevalence of motor-skill impairment in preterm children who do not develop cerebral palsy: a systematic review. Dev Med Child Neurol. (2010) 52:232–7. doi: 10.1111/j.1469-8749.2009.03544.x
3. Rogers CE, Lean RE, Wheelock MD, Smyser CD. Aberrant structural and functional connectivity and neurodevelopmental impairment in preterm children. J Neurodev Disord. (2018) 10:38. doi: 10.1186/s11689-018-9253-x
4. Belsky J, Pluess M. The nature (and nurture?) of plasticity in early human development. Perspect Psychol Sci. (2009) 4:345–51. doi: 10.1111/j.1745-6924.2009.01136.x
5. Burnett AC, Cheong JLY, Doyle LW. Biological and Social Influences on the Neurodevelopmental Outcomes of Preterm Infants. Clin Perinatol. (2018) 45:485–500. doi: 10.1016/j.clp.2018.05.005
6. Hallman M. Premature birth and diseases in premature infants: common genetic background? J Matern Fetal Neonatal Med. (2012) 25 (Suppl 1):21–4. doi: 10.3109/14767058.2012.667600
7. Poggi C, Giusti B, Gozzini E, Sereni A, Romagnuolo I, Kura A, et al. Genetic contributions to the development of complications in preterm newborns. PLoS One. (2015) 10:e0131741. doi: 10.1371/journal.pone.0131741
8. Blair LM, Pickler RH, Anderson C. Integrative review of genetic factors influencing neurodevelopmental outcomes in preterm infants. Biol Res Nurs. (2016) 18:127–37. doi: 10.1177/1099800415605379
9. Nemoda Z, Szekely A, Sasvari-Szekely M. Psychopathological aspects of dopaminergic gene polymorphisms in adolescence and young adulthood. Neurosci Biobehav Rev. (2011) 35:1665–86. doi: 10.1016/j.neubiorev.2011.04.002
10. Nguyen M, Roth A, Kyzar EJ, Poudel MK, Wong K, Stewart AM, et al. Decoding the contribution of dopaminergic genes and pathways to autism spectrum disorder (ASD). Neurochem Int. (2014) 66:15–26. doi: 10.1016/j.neuint.2014.01.002
11. Genro JP, Polanczyk GV, Zeni C, Oliveira AS, Roman T, Rohde LA, et al. A common haplotype at the dopamine transporter gene 5' region is associated with attention-deficit/hyperactivity disorder. Am J Med Genet B Neuropsychiatr Genet. (2008) 147B:1568–75. doi: 10.1002/ajmg.b.30863
12. Gizer IR, Ficks C, Waldman ID. Candidate gene studies of ADHD: a meta-analytic review. Hum Genet. (2009) 126:51–90. doi: 10.1007/s00439-009-0694-x
13. Guan L, Wang B, Chen Y. A high-density single-nucleotide polymorphism screen of 23 candidate genes in attention deficit hyperactivity disorder: suggesting multiple susceptibility genes among Chinese Han population. Mol Psychiatry. (2009) 14:546–54. doi: 10.1038/sj.mp.4002139
14. Gomez-Sanchez CI, Carballo JJ, Riveiro-Alvarez R, Soto-Insuga V, Rodrigo M, Mahillo-Fernandez I, et al. Pharmacogenetics of methylphenidate in childhood attention-deficit/hyperactivity disorder: long-term effects. Sci Rep. (2017) 7:10391. doi: 10.1038/s41598-017-10912-y
15. Davies P, Cicchetti D, Hentges RF. Maternal unresponsiveness and child disruptive problems: the interplay of uninhibited temperament and dopamine transporter genes. Child Dev. (2015) 86:63–79. doi: 10.1111/cdev.12281
16. Rommelse NN, Altink ME, Arias-Vasquez A, Buschgens CJ, Fliers E, Faraone SV, et al. Differential association between MAOA, ADHD and neuropsychological functioning in boys and girls. Am J Med Genet B Neuropsychiatr Genet. (2008) 147b:1524–30. doi: 10.1002/ajmg.b.30845
17. de Krom M, Staal WG, Ophoff RA. A common variant in DRD3 receptor is associated with autism spectrum disorder. Biol Psychiatry. (2009) 65:625–30. doi: 10.1016/j.biopsych.2008.09.035
18. Mills-Koonce WR, Propper CB, Gariepy JL, Blair C, Garrett-Peters P, Cox MJ. Bidirectional genetic and environmental influences on mother and child behavior: the family system as the unit of analyses. Dev Psychopathol. (2007) 19:1073–87. doi: 10.1017/S0954579407000545
19. Soderqvist S, Matsson H, Peyrard-Janvid M, Kere J, Klingberg T. Polymorphisms in the dopamine receptor 2 gene region influence improvements during working memory training in children and adolescents. J Cogn Neurosci. (2014) 26:54–62. doi: 10.1162/jocn_a_00478
20. Nymberg C, Banaschewski T, Bokde AL, Buchel C, Conrod P, Flor H, et al. DRD2/ANKK1 polymorphism modulates the effect of ventral striatal activation on working memory performance. Neuropsychopharmacology. (2014) 39:2357–65. doi: 10.1038/npp.2014.83
21. Cardel MI, Lemas DJ, Lee AM, Miller DR, Huo T, Klimentidis YC, et al. Taq1a polymorphism (rs1800497) is associated with obesity-related outcomes and dietary intake in a multi-ethnic sample of children. Pediatr Obes. (2019) 14:e12470. doi: 10.1111/ijpo.12470
22. Staal WG. Autism, DRD3 and repetitive and stereotyped behavior, an overview of the current knowledge. Eur Neuropsychopharmacol. (2015) 25:1421–6. doi: 10.1016/j.euroneuro.2014.08.011
23. Albaugh MD, Harder VS, Althoff RR, Rettew DC, Ehli EA, Lengyel-Nelson T, et al. COMT Val158Met genotype as a risk factor for problem behaviors in youth. J Am Acad Child Adolesc Psychiatry. (2010) 49:841–9. doi: 10.1016/j.jaac.2010.05.015
24. Barnett JH, Heron J, Goldman D, Jones PB, Xu K. Effects of catechol-O-methyltransferase on normal variation in the cognitive function of children. Am J Psychiatry. (2009) 166:909–16. doi: 10.1176/appi.ajp.2009.08081251
25. Esmaiel NN, Ashaat EA, Mosaad R, Fayez A, Ibrahim M, Abdallah ZY, et al. The potential impact of COMT gene variants on dopamine regulation and phenotypic traits of ASD patients. Behav Brain Res. (2020) 378:112272. doi: 10.1016/j.bbr.2019.112272
26. Su YH, Jeng SF, Hsieh WS, Tu YK, Wu YT, Chen LC. Gross motor trajectories during the first year of life for preterm infants with very low birth weight. Phys Ther. (2017) 97:365–73. doi: 10.1093/ptj/pzx007
27. Wu YC, Leng CH, Hsieh WS, Hsu CH, Chen WJ, Gau SS, et al. A randomized controlled trial of clinic-based and home-based interventions in comparison with usual care for preterm infants: effects and mediators. Res Dev Disabil. (2014) 35:2384–93. doi: 10.1016/j.ridd.2014.06.009
28. Yu YT, Hsieh WS, Hsu CH, Lin YJ, Lin CH, Hsieh S, et al. Family-centered care improved neonatal medical and neurobehavioral outcomes in preterm infants: randomized controlled trial. Phys Ther. (2017) 97:1158–68. doi: 10.1093/ptj/pzx089
29. Bayley N. Bayley Scales of Infant Development. San Antonio, TX: The Psychological Corporation, Harcourt Brace & Company (1993).
30. Bayley N, Reuner G. Bayley Scales of Infant and Toddler Development: Bayley-III. San Antonio, TX: Harcourt Assessment Inc. (2006). doi: 10.1037/t14978-000
31. Wu YT, Tsou KI, Hsu CH, Fang LJ, Yao G, Jeng SF. Taiwanese infants' mental and motor development −6-24 months. J Pediatr Psychol. (2008) 33:102–8. doi: 10.1093/jpepsy/jsm067
32. Manca M, Pessoa V, Lopez AI, Harrison PT, Miyajima F, Sharp H, et al. The Regulation of monoamine oxidase A gene expression by distinct variable number tandem repeats. J Mol Neurosci. (2018) 64:459–70. doi: 10.1007/s12031-018-1044-z
33. Purcell S, Neale B, Todd-Brown K, Thomas L, Ferreira MA, Bender D, et al. PLINK: a tool set for whole-genome association and population-based linkage analyses. Am J Hum Genet. (2007) 81:559–75. doi: 10.1086/519795
34. Resnick MB, Eyler FD, Nelson RM, Eitzman DV, Bucciarelli RL. Developmental intervention for low birth weight infants: Improved early development outcome. Pediatrics. (1987) 80:68–74.
35. Babineau V, Green CG, Jolicoeur-Martineau A, Bouvette-Turcot AA, Minde K, Sassi R, et al. Prenatal depression and 5-HTTLPR interact to predict dysregulation from 3 to 36 months–a differential susceptibility model. J Child Psychol Psyc. (2015) 56:21–9. doi: 10.1111/jcpp.12246
36. Barrett JC. Haploview: Visualization and analysis of SNP genotype data. Cold Spring Harb Protoc. (2009) 2009:pdb.ip71. doi: 10.1101/pdb.ip71
37. Walker GA, Shostak J. Common Statistical Methods for Clinical Research with SAS Examples. Cary, NC: SAS Institute Inc. (2010).
38. Armstrong RA. When to use the Bonferroni correction. Ophthalmic Physiol Opt. (2014) 34:502–8. doi: 10.1111/opo.12131
39. Neyman J, Pearson ES. On the use and interpretation of certain test criteria for purposes of statistical inference. Biometrika. (1928) 20A:175–240. doi: 10.1093/biomet/20A.1-2.175
40. Park Y, Won S, Nam M, Chung JH, Kwack K. Interaction between MAOA and FOXP2 in association with autism and verbal communication in a Korean population. J Child Neurol. (2013) 29:207–11. doi: 10.1177/0883073813511301
41. Shih JC, Thompson RF. Monoamine oxidase in neuropsychiatry and behavior. Am J Hum Genet. (1999) 65:593–8. doi: 10.1086/302562
42. Arpino C, D'Argenzio L, Ticconi C, Di Paolo A, Stellin V, Lopez L, et al. Brain damage in preterm infants: etiological pathways. Ann Ist Super Sanita. (2005) 41:229–37.
43. Bhutta AT, Anand KJ. Vulnerability of the developing brain. Neuronal mechanisms. Clin Perinatol. (2002) 29:357–72. doi: 10.1016/S0095-5108(02)00011-8
44. Wardle MC, de Wit H, Penton-Voak I, Lewis G, Munafo MR. Lack of association between COMT and working memory in a population-based cohort of healthy young adults. Neuropsychopharmacology. (2013) 38:1253–63. doi: 10.1038/npp.2013.24
45. Dumontheil I, Roggeman C, Ziermans T, Peyrard-Janvid M, Matsson H, Kere J, et al. Influence of the COMT genotype on working memory and brain activity changes during development. Biol Psychiatry. (2011) 70:222–9. doi: 10.1016/j.biopsych.2011.02.027
46. Caspi A, McClay J, Moffitt TE, Mill J, Martin J, Craig IW, et al. Role of genotype in the cycle of violence in maltreated children. Science. (2002) 297:851–4. doi: 10.1126/science.1072290
47. Maglione D, Caputi M, Moretti B, Scaini S. Psychopathological consequences of maltreatment among children and adolescents: a systematic review of the GxE literature. Res Dev Disabil. (2018) 82:53–66. doi: 10.1016/j.ridd.2018.06.005
48. Belsky J, Pluess M, Widaman KF. Confirmatory and competitive evaluation of alternative gene-environment interaction hypotheses. J Child Psychol Psyc. (2013) 54:1135–43. doi: 10.1111/jcpp.12075
Keywords: dopamine, genetics, child development, cognitive development, prematurity, longitudinal analysis
Citation: Yao N-J, Hsieh W-S, Lin C-H, Tseng C-I, Lin W-Y, Kuo P-H, Yu Y-T, Chen WJ and Jeng S-F (2020) Interaction Between Prematurity and the MAOA Gene on Mental Development in Children: A Longitudinal View. Front. Pediatr. 8:92. doi: 10.3389/fped.2020.00092
Received: 05 September 2019; Accepted: 21 February 2020;
Published: 09 March 2020.
Edited by:
Nicole Letourneau, University of Calgary, CanadaReviewed by:
Jim Kennedy, Centre for Addiction and Mental Health (CAMH), CanadaNihar Ranjan Mishra, Veer Surendra Sai Medical College and Hospital, India
Copyright © 2020 Yao, Hsieh, Lin, Tseng, Lin, Kuo, Yu, Chen and Jeng. This is an open-access article distributed under the terms of the Creative Commons Attribution License (CC BY). The use, distribution or reproduction in other forums is permitted, provided the original author(s) and the copyright owner(s) are credited and that the original publication in this journal is cited, in accordance with accepted academic practice. No use, distribution or reproduction is permitted which does not comply with these terms.
*Correspondence: Wei J. Chen, d2pjaGVuQG50dS5lZHUudHc=; Suh-Fang Jeng, amVuZ0BudHUuZWR1LnR3