- 1Department of Radiation Oncology, Yonsei Cancer Center, Yonsei University College of Medicine, Seoul, South Korea
- 2Division of Medical Oncology, Department of Internal Medicine, Yonsei Cancer Center, Songdang Institute for Cancer Research, Yonsei University College of Medicine, Seoul, South Korea
- 3Graduate School of Medical Science, Brain Korea 21 Project, Yonsei University College of Medicine, Seoul, South Korea
- 4Division of Biomedical Informatics, Center for Genome Science, National Institute of Health, KCDC, Cheongju, South Korea
Background: Despite the important role of radiotherapy in cancer treatment, a subset of patients responds poorly to treatment majorly due to radioresistance. Particularly the role of radiotherapy has not been established in gastric cancer (GC). Herein, we aimed to identify a radiosensitivity gene signature and to discover relevant targets to enhance radiosensitivity in GC cells.
Methods: An oligonucleotide microarray (containing 22,740 probes) was performed in 12 GC cell lines prior to radiation. A clonogenic assay was performed to evaluate the survival fraction at 2 Gy (SF2) as a surrogate marker for radiosensitivity. Genes differentially expressed (fold change > 6, q-value < 0.025) were identified between radiosensitive and radioresistant cell lines, and quantitative reverse transcriptase-polymerase chain reaction (qRT-PCR) was performed for validation. Gene set and pathway analyses were performed using Ingenuity Pathway Analysis (IPA).
Results: Radiosensitive (SF2 < 0.4) and radioresistant cell lines (SF2 ≥ 0.6) exhibited a marked difference in gene expression. We identified 68 genes that are differentially expressed between radiosensitive and radioresistant cell lines. The identified genes showed interactions via AKT, HIF1A, TGFB1, and TP53, and their functions were associated with the genetic networks associated with cellular growth and proliferation, cellular movement, and cell cycle. The Akt signaling pathway exhibited the highest association with radiosensitivity. Combinatorial treatment with MK-2206, an allosteric Akt inhibitor, and radiotherapy significantly increased cell death compared with radiotherapy alone in two radioresistant cell lines (YCC-2 and YCC-16).
Conclusion: We identified a GC-specific radiosensitivity gene signature and suggest that the Akt signaling pathway could serve as a therapeutic target for GC radiosensitization.
Introduction
Gastric cancer (GC) is the third leading cause of cancer-related death worldwide (1, 2). In operable cases, surgery and chemotherapy form the mainstay of treatment. However, 5-year disease-free survival rates of patients with stage III GC ranges from 50 to 60% with standard treatments. Therefore, treatment intensification in patients with locally-advanced GC may be needed to improve the treatment outcomes.
A combination of radiotherapy and chemotherapy has been widely investigated in preoperative as well as postoperative settings as a strategy to improve GC treatment (3–5). However, recent trials suggest that the survival benefit of adding radiotherapy to the treatment regimen is modest (4). The limited efficacy of radiotherapy in GC can be explained by the intrinsic radiation resistance and suboptimal dose delivered due to the low radiation tolerance of organs at risk that surround the stomach. A variety of radiation resistance mechanisms have been studied, such as the DNA repair system, increased receptor tyrosine kinase signaling, decreased radiation-induced apoptosis, tumor hypoxia, and angiogenesis (6). The most widely used agents for radiosensitization are cytotoxic chemotherapeutics, such as 5-fluorouracil, and cisplatin (7). Combinations of radiotherapy with these agents have already been investigated with marginal effects and not negligible toxicities. Therefore, new classes of radiosensitizing agents are needed to improve the treatment outcomes in GC patients. One of the candidates can be relevant to a radiosensitivity mechanism.
Gene expression profile analysis to predict response to radiation therapy has been performed in various types of cancers (8–14). It has been shown that a gene expression-based model can predict patient-specific radiation sensitivity (15). These gene expression data can not only be used in predicting patient radiosensitivity but also in screening for new possible targets in addition to previously suggested predictive markers (16).
In this study, we measured the radiosensitivity, analyzed the mRNA expression profile of GC cells before radiotherapy, and identified differentially-expressed genes and relevant signaling pathways involved in the development of radiosensitivity by comparing radiosensitive and radioresistant GC cell lines. Especially, the Akt pathway was evaluated as a crucial pathway in determining radioresistance of GC cells, and an Akt inhibitor markedly increased radiosensitivity in radioresistant GC cell lines.
Materials and Methods
Cell Lines and Culture
Twelve GC cell lines (AGS, MKN-1, MKN-74, SNU-216, SNU-484, SNU-638, YCC-1, YCC-16, YCC-2, YCC-3, YCC-6, and YCC-7) were used. The SNU-series of cell lines were obtained from the Korean cell line bank, and the YCC-series represented cell lines that were established using samples from Korean GC patients at the Songdang Institute for Cancer Research (SICR, Yonsei University College of Medicine, Seoul, South Korea). Cells were incubated in RPMI supplemented with 10% heat inactivated fetal bovine serum, 1% penicillin/streptomycin at 37°C in a 5% CO2 humidified atmosphere, following the institutional protocol (17).
Clonogenic Assay
Radiosensitivity was defined as the survival fraction at 2 Gy of radiation (SF2). To evaluate radiation sensitivity in GC cells, cells were seeded in triplicate and were incubated overnight at 37°C to enable them to adhere to the wells. Cells were then irradiated with X-rays (2 Gy), and cell viability was evaluated 7–10 days post-irradiation. After fixation, colonies containing over 50 cells were calculated. Experiments were replicated three times independently, and the average number of colonies was used. SF2 was determined by the following formula:
where seedingefficiency is defined as the number of colonies formed divided by expected colony number. SF2 ranged from 0 to 1, wherein a lower SF2 represents higher radiosensitivity.
RNA Preparation and Oligonucleotide Microarrays
Microarray data were obtained from GC cells in the unirradiated condition. The total RNA was extracted from each cell line using the TRIzol reagent (Invitrogen, Carlsbad, CA, United States) according to the manufacturer’s instructions. The Yonsei reference RNA was prepared as previously described (18). The quantity and quality of RNA were confirmed using an ND-1000 spectrophotometer (NanoDrop Technologies, United States) and gel electrophoresis. An oligonucleotide microarray was performed using a human oligo chip (SICR-GT, Seoul, South Korea) containing 22,740 oligonucleotide probes (70 bases each with a reference design). The test samples (RNA from each GC cell line) were labeled with Cy5 and individually co-hybridized with the Cy3-labeled reference RNA (SICR, Seoul, South Korea). Gene expression data were deposited in the Gene Expression Omnibus (GEO) database (accession number: GSE39747).
Gene Expression Analysis
Microarray data extraction and analysis were performed using the BRB-ArrayTools1 for gene identification and gene set analysis. For normalization, the linear models for the microarray data (LIMMA) package were applied using R (version 3.6.1). Genes for which less than 20% of expression data exhibited at least a 1.5-fold change in either direction from the median value of the gene were excluded in the filtering process. Genes differentially expressed between radiosensitive and radioresistant cells were identified by a two-sample t-test using a random-variance model (q-value < 0.025 and fold change more than 2-fold). The P value was adjusted for multiple hypothesis testing using the q-value as suggested by Story (19).
The genetic network was generated through the use of Ingenuity Pathways Analysis (IPA, Ingenuity Systems, www.ingenuity.com). Differentially expressed genes (DEGs) were overlaid onto a global molecular network developed based on the information contained in the Ingenuity Knowledge Base. Networks of network-eligible molecules were then algorithmically generated based on their connectivity. In the genetic network, molecules were represented as nodes, and the biological relationship between two nodes was represented as an edge. All edges were supported by at least one reference from the literature, a textbook, or canonical information stored in the Ingenuity Pathways Knowledge Base.
A heatmap was generated using the BRB-ArrayTools. Principal component analysis was performed for data reduction and simplifying datasets to three dimensions for plotting purposes. Principal component analysis was conducted using R statistical software2, using the “princomp()” function and default options.
Quantitative RT-PCR
DIRAS3, CDKN2B, POF1B, ALDH1A1, and ANTXR2 were selected for the validation of the microarray data by quantitative RT-PCR (qRT-PCR) performed on the 12 GC cell lines. In brief, 4 μg of total RNA from each sample was reversely transcribed using the SuperScript II Reverse Transcriptase (Invitrogen, Carlsbad, CA, United States). Two hundred nanograms of synthesized cDNA were PCR-amplified using QuantiTect SYBR Green PCR (QIAGEN, Valencia, CA, United States). Each reaction was run in a Stratagene MX3005P (Stratagene, La Jolla, CA, United States). Expression values for each gene were determined using a standard curve constructed from Human Genomic DNA (Promega, Madison, WI, United States). The house-keeping gene hypoxanthine phosphoribosyltransferase (HGPRT, HPRT1) was selected for the normalization and construction of the standard curve. Non-template control wells without cDNA were included as negative controls. The primer sets designed for PCR amplification are shown in Supplementary Table S3.
Whole Exome Sequencing
To identify somatic mutations in TP53, KRAS, and PIK3CA, whole exome sequencing (WES) data of the 12 GC cell lines were obtained from the genome database of the SICR and the Yonsei University College of Medicine (Seoul, South Korea). Briefly, single nucleotide variants (SNVs) were evaluated using WES data as previously described (17).
AKT Inhibitor Treatment
A highly selective AKT inhibitor, MK-2206 was purchased from Selleckchem (Houston, TX, United States). To assess the radiosensitizing effect of AKT pathway inhibition in combination with radiation, clonogenic assays with or without the AKT pathway inhibitor MK-2206 were performed using radioresistant YCC-16 cells, which harbor the PIK3CA mutation, and YCC-2 cells, which harbor the KRAS mutation. Clonogenic assays were performed as described previously. Cells were seeded in triplicate for 24 h to allow attachment to wells. Cells were treated with MK-2206 (1.0 μM) or the vehicle control for 1 h, followed by 2 Gy or mock irradiation. Immediate growth medium exchange was not performed after radiation. Plates were incubated to allow for colony formation (7 d). Colonies containing over 50 cells were then calculated and experiments were repeated three times.
Statistical Analysis
Statistical analyses were performed using the GraphPad Prism 7 and R software (v.3.5.1). For continuous variables, student’s t-test was applied when comparing two groups which are non-paired. When comparing continuous variables among more than two groups, analysis of variance (ANOVA) was applied and Tukey’s post hoc analysis was performed to identify which groups exhibit significant difference among the multiple groups. The statistical tests used are specified in the figure legends. A Pearson’s correlation analysis was used to evaluate the correlations between parameters. P values < 0.05 indicated statistical significance.
Results
Association of SF2 With Genetic Variation in GC Cells
The study design is summarized in Figure 1. First, to determine the radiosensitivity of gastric cells, twelve GC cell lines were irradiated at 2 Gy and a clonogenic assay was performed to calculate the SF2 (Table 1 and Supplementary Figure S1). SNU-638 and MKN-1 cells were highly radiosensitive with an SF2 < 0.4 (0.127 and 0.143, respectively). The YCC-2, YCC-16, and YCC-7 cell lines were radioresistant with an SF2 ≥ 0.6 (0.609, 0.620, and 0.667, respectively). No significant difference in SF2 was observed according to genetic mutations in TP53, PIK3CA, and KRAS (Supplementary Figure S2A). In addition, no significant correlation between doubling time and SF2 was observed (Supplementary Figure S2B).
Differential Expression of Genes Between Radiosensitive and Radioresistant GC Cells
To identify individual genes and functions relevant to radiosensitivity, gene expression profiling was performed. Two-sample t-tests between radiosensitive (SF2 < 0.4) and radioresistant cell lines (SF2 ≥ 0.6) identified 613 genes showing expression levels with differences greater than 2-fold and q-value of less than 0.025 (Figure 2A). Cell lines with intermediate radiosensitivity were excluded from DEG-analysis to drive a more radiosensitivity specific gene set. Of these genes, 68 showed differences in expression with more than a 6-fold change (Supplementary Table S1). To validate the microarray results, we selected five genes (DIRAS3, CDKN2B, POF1B, ALDH1A1, and ANTXR2) for analysis by qRT-PCR. Similar to the microarray results, these genes exhibited a greater than 6-fold difference between radiosensitive and radioresistant GC cells (Figure 2B). Moreover, we confirmed that the cell lines with intermediate radiosensitivity exhibited an intermediate gene signature between sensitive and resistant cell lines (Supplementary Figure S3). In addition, principal component analysis (PCA) was performed to assess the transcriptional landscape of the GC cell lines. The PCA plot well distinguished the radiosensitive cell lines (SNU-638 and MKN-1) from radioresistant cell lines (YCC-2, YCC-16, and YCC-7) while the intermediate cell lines (YCC-3, YCC-1, AGS, YCC-6, SNU-216, SNU-484, and MKN-74) were placed between the radiosensitive and radioresistant cell lines (Figure 2C).
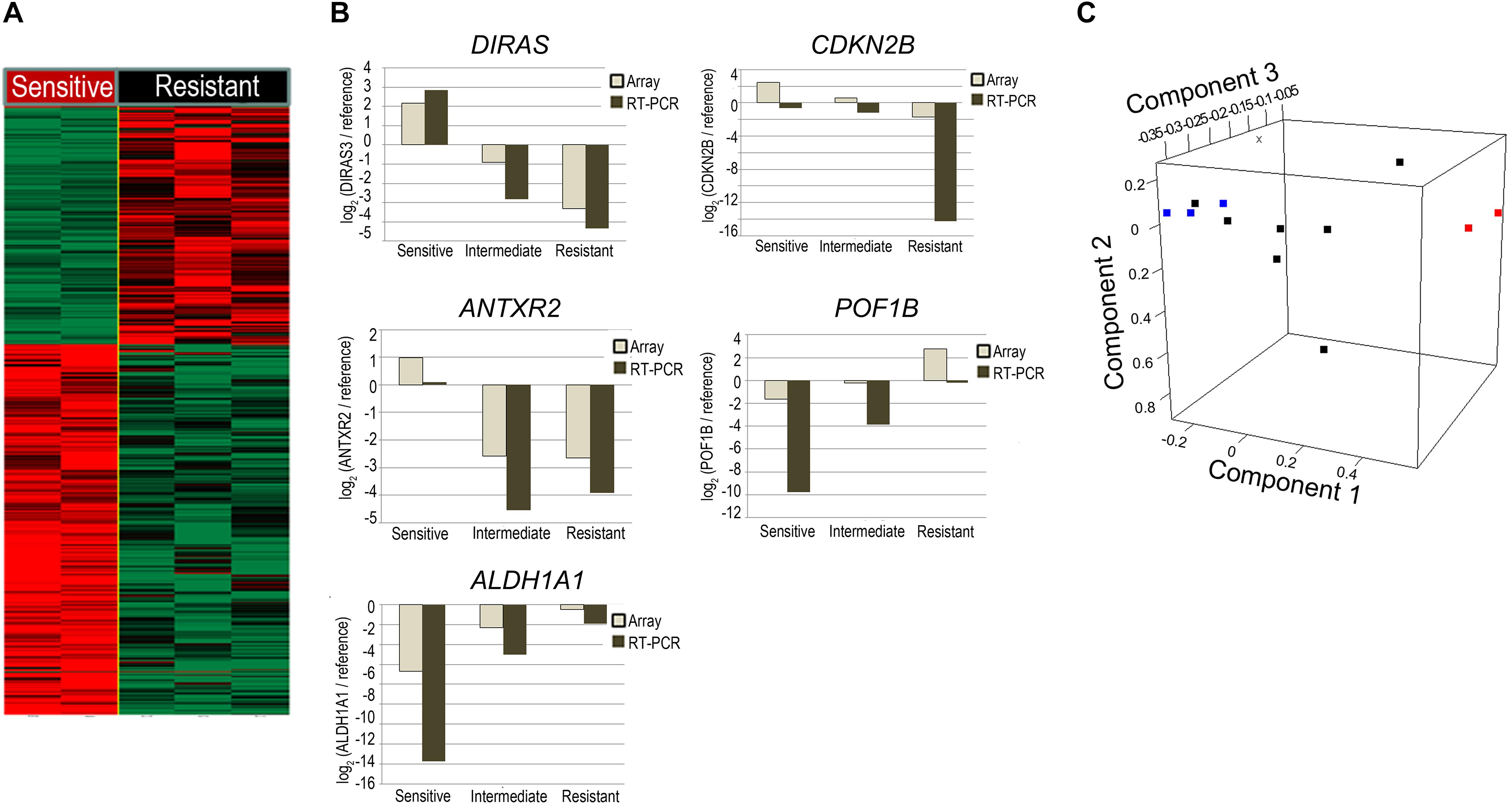
Figure 2. Comparison of the gene expression profile between radiosensitive and radioresistant gastric cancer (GC) cell lines. (A) Microarray was performed in radiosensitive (SF2 < 0.4; SNU-638; and MKN-1) and radioresistant cells (SF2 ≥ 0.6; YCC-2, YCC-16, and YCC-7). A heatmap showing differences in gene expression levels greater than 2-fold change. Genes whose expression levels increased more than 2-fold are shown in red, whereas those whose expression decreased more than 2-fold are shown in green. (B) Comparison of qRT-PCR with microarray-based gene expression levels among radiosensitive (SF2 < 0.4; SNU-638; and MKN-1), intermediate (0.4 ≤ SF2 < 0.6; YCC-3, YCC-1, AGS, YCC-6, SNU-216, SNU-484, and MKN-74), and radioresistant cells (SF2 ≥ 0.6; YCC-2, YCC-16, and YCC-7) of DIRAS3, CDKN2B, ANTXR2, and ALDH1A1. (C) Principal component analysis with gene expression profiles of the GC cell lines. Each cell line is represented as a radiosensitive group (SF2 < 0.4; red), an intermediate group (SF2 between 0.4 and 0.6; black), or a radioresistant group (SF2 ≥ 0.6; blue).
Akt Pathway as a Candidate for Regulating Radiosensitivity in GC Cells
Functional annotation and pathway analysis of the identified 68-gene signature was performed using Ingenuity Pathway Analysis (IPA) to assess which pathways are involved in the development of radiosensitivity in GC cells. Figure 3 displays the top four genetic networks found to be enriched in IPA. Each genetic network showed interactions via major signaling pathway molecules, including AKT, HIF1A, TGFB1, and TP53 (Figure 3D). Functions associated with the genetic networks included cellular growth and proliferation, cellular movement, and cell cycle (Table 2). The Akt-centered network exhibited the highest IPA score, and nine genes of the 68 identified genes were related to the Akt signaling pathway (Supplementary Table S2).
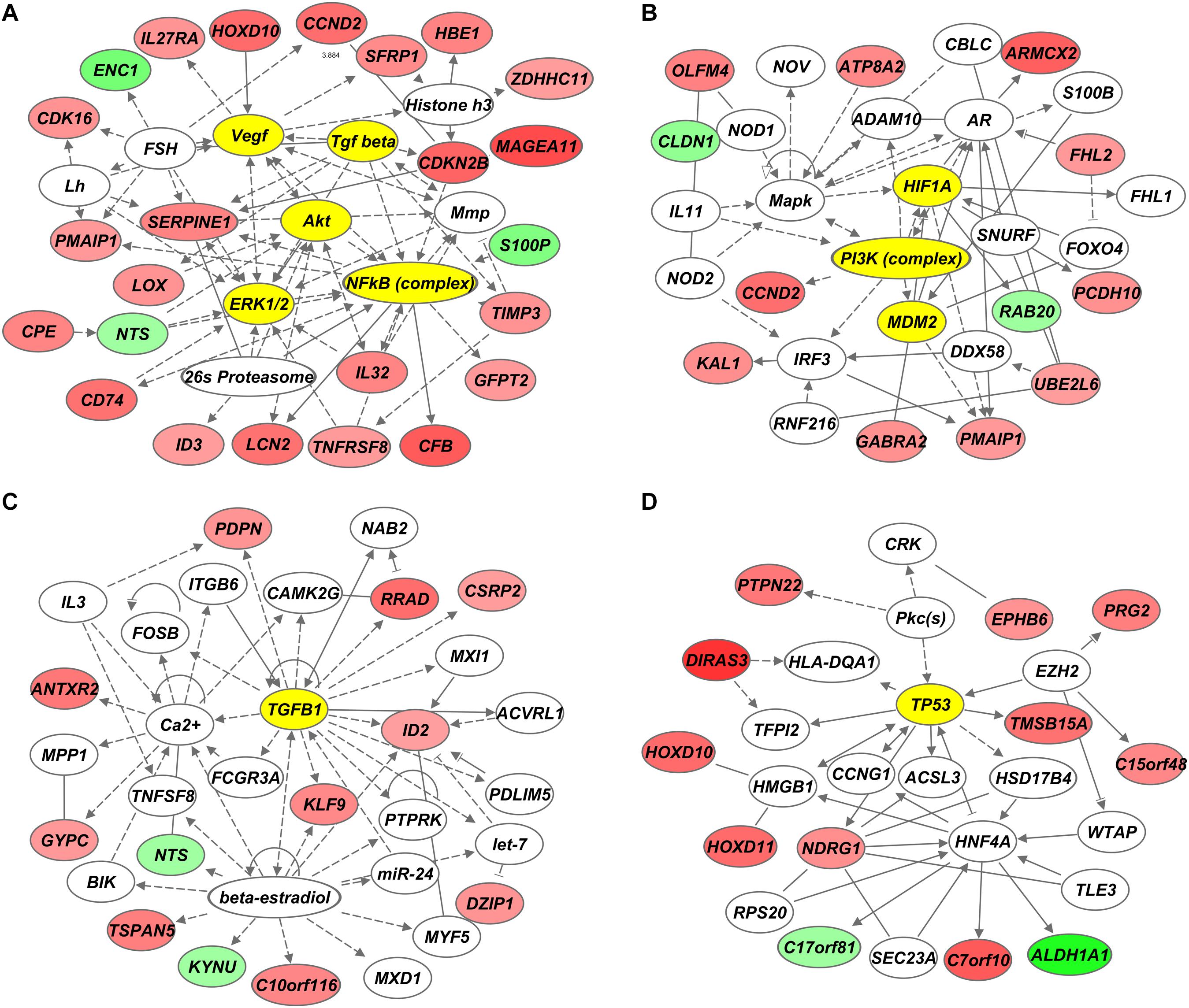
Figure 3. Genetic networks associated with genes differentially expressed between radiosensitive and radioresistant gastric cancer (GC) cell lines. (A–D) Ingenuity Pathway Analysis (IPA) revealed AKT (A), HIF1A (B), TGFB1 (C), and TP53 (D) as core genetic networks associated with radiosensitivity.
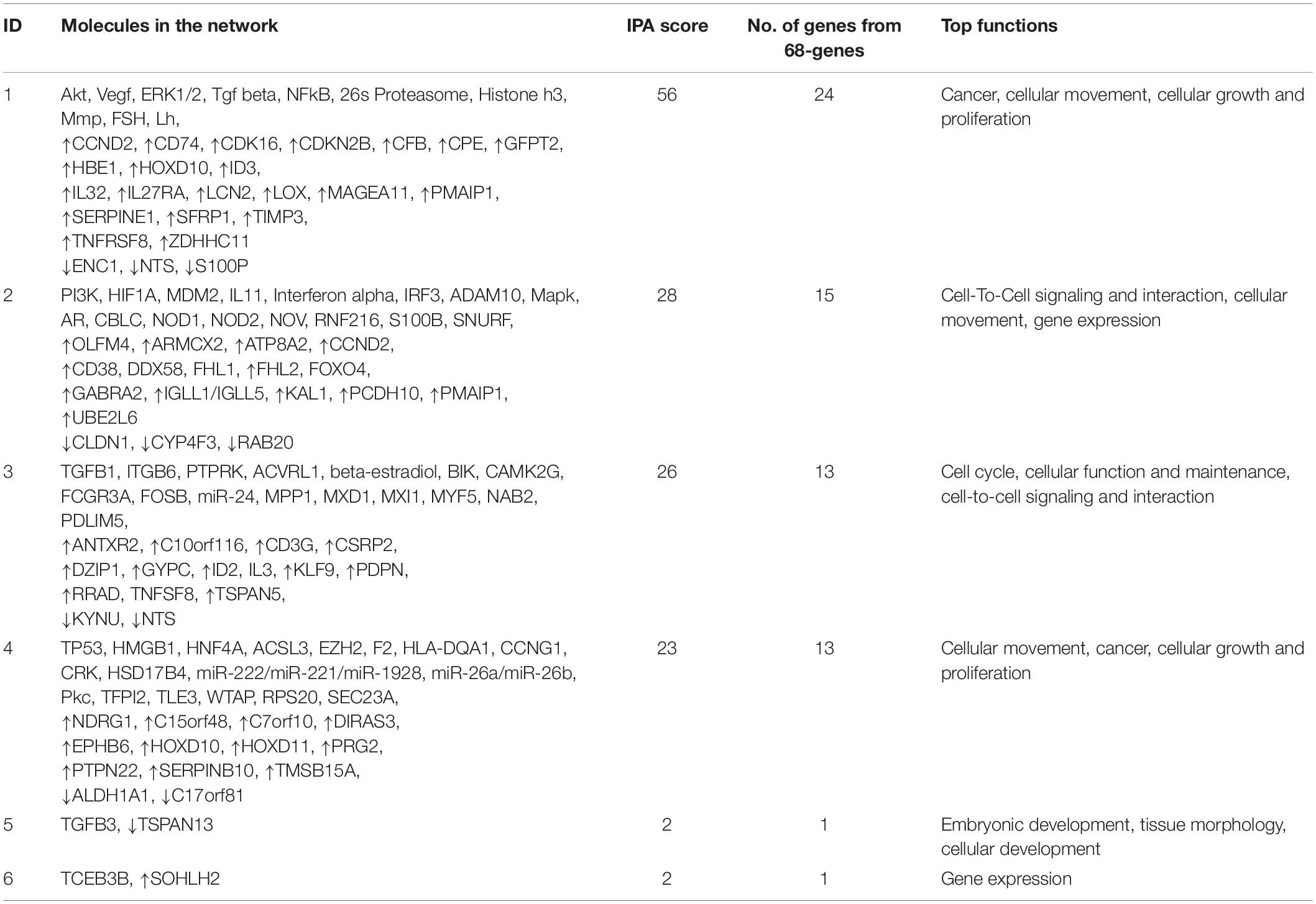
Table 2. Results of the Ingenuity pathway network analysis (IPA) using the 68 differentially-expressed genes
Increase in Radiosensitivity as a Result of Akt Pathway Inhibition
Considering the potential role of the Akt pathway in determining the radiosensitivity based on gene network analyses, we further investigated whether the Akt pathway could serve as a druggable target to decrease radiosensitivity in GC cells. The radioresistant cell lines, YCC-2 and YCC-16, were subjected to combinatorial treatment with 2 Gy of radiation and allosteric Akt inhibitor, MK-2206. Although a decrease in SF2 was observed after monotherapy with either MK-2206 or radiotherapy, a combinatorial treatment of MK-2206 and radiotherapy resulted in a significant decrease in SF2 compared to that observed on using either treatment alone (Figure 4).
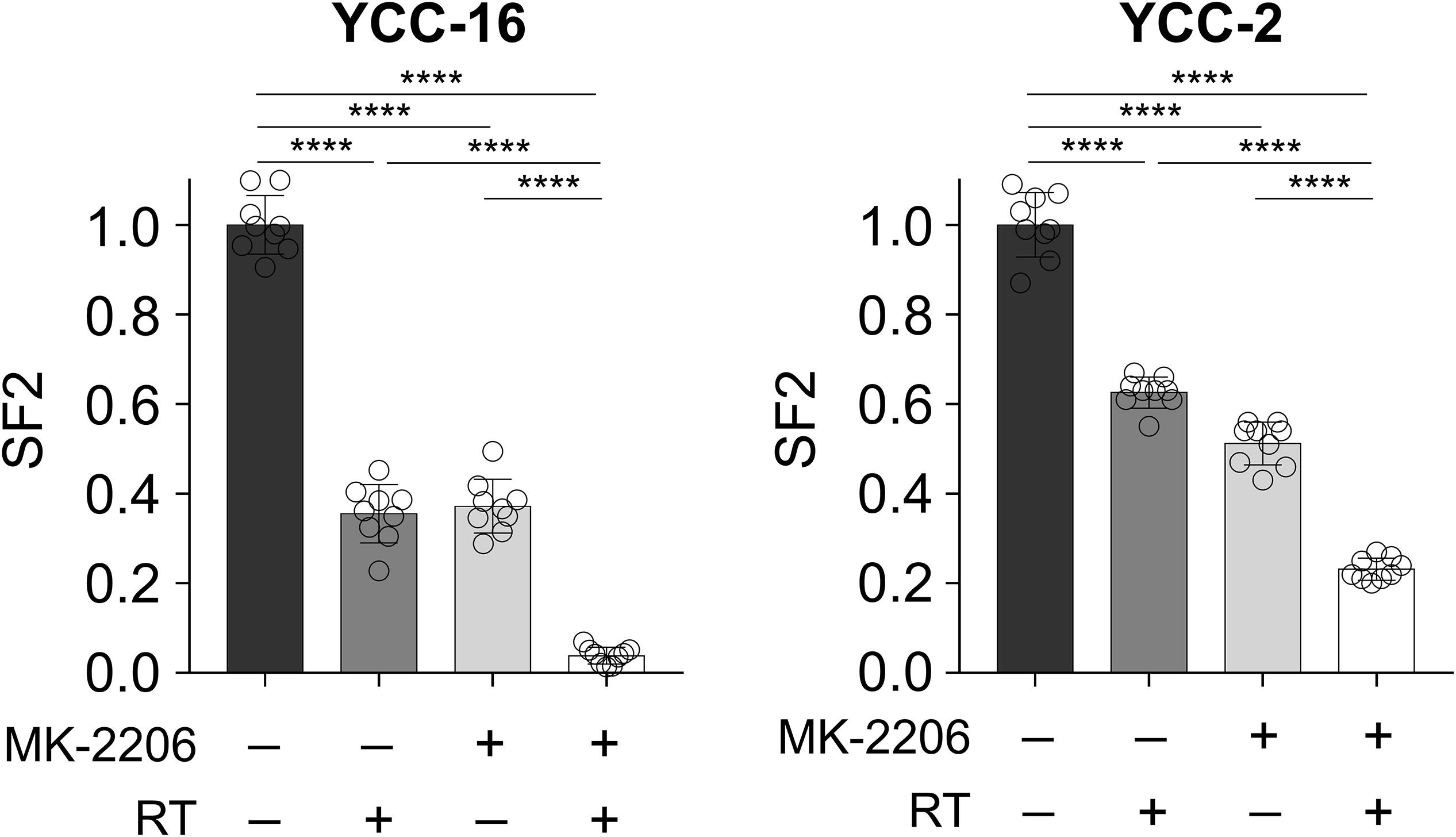
Figure 4. AKT signaling inhibitor MK-2206 enhances the effect of radiotherapy. YCC-16 and YCC-2 cell lines were treated with MK-2206 (1.5 μM) for 1 h prior to radiation, and the clonogenic assay was performed to measure SF2 in each treatment condition (n = 9 for each experiment). Statistical analysis by ANOVA with post-hoc Tukey’s multiple comparison test. ****P < 0.0001.
Discussion
Herein, we describe the gene signature of radiosensitive and radioresistant GC cells, and demonstrate the role of the Akt pathway in determining the radiosensitivity of GC cells. We used 12 GC cell lines and found that radioresistant GC cell lines exhibit contrasting gene expression profiles compared with radiosensitive GC cell lines. Further gene network analyses revealed Akt as a central pathway regulating radiosensitivity. Blocking the Akt signaling pathway through a clinically available Akt inhibitor, MK-2206, significantly enhanced radiosensitivity in radioresistant GC cell lines, which implies that Akt pathway could serve as a potential therapeutic target.
This is the first study to describe the SF2 of multiple GC cell lines and correlate gene expression profiles with radiosensitivity. From our gene network analyses, we discovered major signaling pathways, including molecules such as Akt, VEGF, HIF-1α, TGF-β, and p53. These gene networks are known to regulate cellular growth, proliferation, movement, and cell cycle. We further focused on the role of Akt as the Akt-centered network exhibited the highest IPA score. Akt is a serine/threonine protein kinase and a major signaling molecule of the phosphatidylinositol 3-kinase (PI3K)/AKT/mTOR pathway, which activates downstream molecules involved in cell survival, cell cycle, and proliferation (20). Amplification of AKT1 and a somatic mutation of AKT2 have been previously reported in GC, and about 80% of tumors have been found to have elevated levels of Akt and phosphorylated Akt, which showed a statistically significant correlation with poor patient outcomes in GC (21). Akt-mediated radioresistance has been suggested to occur via the activation of the DNA-dependent protein kinase catalytic subunit (DNA-PKcs), which is a major enzyme involved in DNA-double strand break repair, and is responsible for decreased degradation of cyclin D1 (which is crucial for cell cycle progression), and the vial up-regulation of miR-214 (22–24).
In our study, although we observed anti-tumor effects of MK-2206 as monotherapy, the anti-tumor effect was significantly enhanced when combined with radiotherapy. In clinical settings, the safety and therapeutic efficacy of the MK-2206 monotherapy as a second-line agent were evaluated in GC patients who progressed after first-line treatment (25). Although the treatment was well tolerated, the therapeutic effect was modest with a response rate of 1%. This is in contrast to the in vitro data from our study, where MK-2206 treated as a single agent induced similar tumor cell killing effects as radiotherapy. The discrepancy may come from the lower efficacy of in vivo drug delivery to tumor compared to in vitro treatment, which may result in a suboptimal drug dose in tumor to kill cancer cells. This implies that although Akt itself may be a therapeutic target in GC, development of combination treatments is needed considering the poor therapeutic efficacy as monotherapy. The combination of radiotherapy and Akt inhibitors has mostly been tested in preclinical models, with Akt inhibitors having a radiosensitizing effect (26, 27). However, such combinations have been rarely tested in clinical studies. Recently, several phase I trials were conducted to evaluate the safety of combining an Akt inhibitor with chemoradiation or stereotactic body radiotherapy in solid tumors (28, 29). Combination treatments of Akt and radiotherapy were well tolerated in these trials. Future studies are needed to evaluate the therapeutic efficacy of this novel combination.
Along with Akt, we also found other major signaling pathways that may be involved in radiosensitization, including VEGF, HIF-1α, TGF-β, and p53. Although p53 is not druggable, target agents blocking VEGF, or TGF-β pathway have been developed. The radiosensitizing effect of anti-angiogenic therapy has been demonstrated from many studies where the radiosensitizing effect is mediated through normalization of tumor vasculature leading to increase in oxygenation of the hypoxic tumor (30). Not only through tumor vasculature, but VEGF may also induce cancer cell intrinsic radioresistance through VEGFR2 expressed on cancer cells, which can be reversed by interfering VEGF/VEGFR2 signaling (31). TGF-β has also been suggested to exhibit synergistic therapeutic effects with radiotherapy (32, 33). TGF-β can mediate radioresistance by inducing a mesenchymal phenotype in cancer cells (33) or by inhibiting radiotherapy-induced anti-tumor immunity (32). Therefore, combination of TGF-β inhibitors with radiotherapy could also be a promising combination. In a clinical trial, fresolimumab, a TGF-β blocking antibody, in combination with radiotherapy was tested in breast cancer patients who failed at least one line of treatment (34). A marked increase in CD8 central memory pool was observed, although the therapeutic response was modest. Other classes of TGF-β inhibitors, such as galunisertib and vactosertib, are also being tested in combination with chemotherapy (35) or immunotherapy (NCT03724851). Taken together, it would be of great interest to investigate the effects of VEGF, HIF-1α, TGF-β, and p53 pathways on radiosensitization. Future trials should also consider the possible radiosensitizing effect of drugs targeting these pathways and test the combination with radiotherapy.
Using gene expression analyses on the 12 GC cell lines, we identified 68 genes that were differentially expressed between radioresistant and radiosensitive cell lines. Expression of selected genes in cell lines according to radiosensitivity were also validated by qRT-PCR (DIRAS3, CDKN2B, POF1B, ALDH1A1, and ANTXR2). We identified other candidates responsible for the development of radiosensitization among the 68 genes. Expression of DIRAS3 and CDKN2B was markedly increased in radiosensitive cell lines. DIRAS3 expression has been shown to be associated with chemosensitization to paclitaxel via cell cycle arrest at the G2/M stage in breast cancer cells (36). CDKN2B encodes a cyclin-dependent kinase inhibitor and controls cell cycle G1 progression, which has a radiosensitizing potential since cells at late G1 stage are known to be radiosensitive (37). Similarly, agents that selectively block CDK4/6 have been reported to enhance radiosensitivity in cancer cells (38). In contrast, expression of ALDH1A1 and POF1B were significantly increased in radioresistant cell lines. ALDH1A1 (aldehyde dehydrogenase 1 family member A1) has been used as a cancer stem cell marker and is associated with chemoresistance in ovarian cancer (39). Moreover, a previous study found that ALDH1A1-silencing sensitized ovarian cancer cells to chemotherapy (40). POF1B has a function in actin binding, and this adhesion-related molecule has been suggested to be important for radioresistance through an interaction with the extracellular matrix (41). Although we only focused on the Akt pathway, other molecules may also serve as promising targets for enhancing radiosensitivity and could be investigated in further studies.
The 68 DEGs may also provide an opportunity to evaluate the radiosensitivity of tumors by gene expression analysis. Although further validation in human tumor samples is required, the 68 DEGs may be used to develop a radiosensitivity scoring platform for predicting response to radiotherapy in GC patients. Oncotype DX is already being widely used in breast cancer patients to properly identify candidates for chemotherapy (42). Scott et al. have reported that a gene-expression-based radiation-sensitivity index derived from the NCI-60 database can predict treatment outcome in patients with solid tumors (15, 43). Selecting the proper candidates and radiation dose for patients based on their gene expression data may improve the therapeutic efficacy of radiotherapy. Future trials exploring the role of radiotherapy in GC patients may need to integrate the gene signature for radiosensitivity to predict tumor-intrinsic radiosensitivity in order to aid in selecting the right patients for enrollment.
Limitations were also present in this study. The number of cell lines used in this study was limited to 12 cell lines which may be inadequate to draw clear conclusions. In addition, we did not perform in vivo studies to evaluate the effect of Akt inhibition on radiosensitivity. Further investigations utilizing a larger number of cell lines along with in vivo studies are required for adequate conclusions.
In conclusion, we report a unique radiosensitivity gene signature in GC cells and describe the role of the Akt pathway in radiosensitizing GC cells. Our findings suggest Akt pathway as a potential therapeutic target to enhance radiosensitivity in GC. The predictive power of our radiosensitivity gene signature and the therapeutic efficacy of combining Akt inhibitors to radiotherapy should be validated in future clinical studies.
Data Availability Statement
The datasets presented in this study can be found in online repositories. The names of the repository/repositories and accession number(s) can be found below: Gene Expression Omnibus (GEO) database (accession number: GSE39747).
Author Contributions
KK, HK, SK, and SR contributed conception and design of the study. HK, SK, and DK collected the data. KK, HK, and SK performed the statistical analysis. KK, HK, and SR wrote the manuscript. YK and HC discussed the hypothesis and contributed to data interpretation. All authors contributed to manuscript revision, read and approved the submitted version.
Funding
This work was supported by the National Research Foundation of Korea (NRF) grant funded by the Korea Government (MSIT; 2020R1A2B5B02001452 to SR; No. 2019R1C1C1006709; and No. 2018R1A5A2025079 to HK), a fund (2017-NI72002-00) by the Research of Korea Centers for Disease Control and Prevention.
Conflict of Interest
The authors declare that the research was conducted in the absence of any commercial or financial relationships that could be construed as a potential conflict of interest.
Supplementary Material
The Supplementary Material for this article can be found online at: https://www.frontiersin.org/articles/10.3389/fonc.2020.562284/full#supplementary-material
Footnotes
References
1. Bray F, Ferlay J, Soerjomataram I, Siegel RL, Torre LA, Jemal A. Global cancer statistics 2018: GLOBOCAN estimates of incidence and mortality worldwide for 36 cancers in 185 countries. CA Cancer J Clin. (2018) 68:394–424. doi: 10.3322/caac.21492
2. Hong S, Won YJ, Park YR, Jung KW, Kong HJ, Lee ES, et al. Cancer statistics in korea: incidence mortality, survival, and prevalence in 2017. Cancer Res Treat. (2020) 52:335–50. doi: 10.4143/crt.2020.206
3. Kim HS, Koom WS, Baek SE, Kim HI, Jung M, Beom SH, et al. Phase II trial of preoperative sequential chemotherapy followed by chemoradiotherapy for high-risk gastric cancer. Radiother Oncol. (2019) 140:143–9. doi: 10.1016/j.radonc.2019.06.029
4. Park SH, Sohn TS, Lee J, Lim DH, Hong ME, Kim KM, et al. Phase III trial to compare adjuvant chemotherapy with capecitabine and cisplatin versus concurrent chemoradiotherapy in gastric cancer: final report of the adjuvant chemoradiotherapy in stomach tumors trial, including survival and subset analyses. J Clin Oncol. (2015) 33:3130–6. doi: 10.1200/JCO.2014.58.3930
5. Kim Y, Kim KM, Choi MG, Lee JH, Sohn TS, Bae JM, et al. Adjuvant chemotherapy with or without concurrent radiotherapy for patients with stage IB gastric cancer: a subgroup analysis of the adjuvant chemoradiotherapy in stomach tumors (ARTIST) phase III trial. J Gastric Cancer. (2018) 18:348–55. doi: 10.5230/jgc.2018.18.e34
6. Hirst DG, Robson T. Molecular biology: the key to personalised treatment in radiation oncology? Br J Radiol. (2010) 83:723–8. doi: 10.1259/bjr/91488645
7. Lawrence TS, Blackstock AW, McGinn C. The mechanism of action of radiosensitization of conventional chemotherapeutic agents. Semin Radiat Oncol. (2003) 13:13–21. doi: 10.1053/srao.2003.50002
8. Spitzner M, Emons G, Kramer F, Gaedcke J, Rave-Frank M, Scharf JG, et al. A gene expression signature for chemoradiosensitivity of colorectal cancer cells. Int J Radiat Oncol Biol Phys. (2010) 78:1184–92. doi: 10.1016/j.ijrobp.2010.06.023
9. Harima Y, Togashi A, Horikoshi K, Imamura M, Sougawa M, Sawada S, et al. Prediction of outcome of advanced cervical cancer to thermoradiotherapy according to expression profiles of 35 genes selected by cDNA microarray analysis. Int J Radiat Oncol Biol Phys. (2004) 60:237–48. doi: 10.1016/j.ijrobp.2004.02.047
10. Kim HS, Kim SC, Kim SJ, Park CH, Jeung HC, Kim YB, et al. Identification of a radiosensitivity signature using integrative metaanalysis of published microarray data for NCI-60 cancer cells. BMC Genomics. (2012) 13:348. doi: 10.1186/1471-2164-13-348
11. Eschrich SA, Pramana J, Zhang H, Zhao H, Boulware D, Lee JH, et al. A gene expression model of intrinsic tumor radiosensitivity: prediction of response and prognosis after chemoradiation. Int J Radiat Oncol Biol Phys. (2009) 75:489–96. doi: 10.1016/j.ijrobp.2009.06.014
12. Strom T, Hoffe SE, Fulp W, Frakes J, Coppola D, Springett GM, et al. Radiosensitivity index predicts for survival with adjuvant radiation in resectable pancreatic cancer. Radiother Oncol. (2015) 117:159–64. doi: 10.1016/j.radonc.2015.07.018
13. Manem VS, Lambie M, Smith I, Smirnov P, Kofia V, Freeman M, et al. Modeling cellular response in large-scale radiogenomic databases to advance precision radiotherapy. Cancer Res. (2019) 79:6227–37. doi: 10.1158/0008-5472.CAN-19-0179
14. Speers C, Zhao S, Liu M, Bartelink H, Pierce LJ, Feng FY. Development and validation of a novel radiosensitivity signature in human breast cancer. Clin Cancer Res. (2015) 21:3667–77. doi: 10.1158/1078-0432.CCR-14-2898
15. Scott JG, Berglund A, Schell MJ, Mihaylov I, Fulp WJ, Yue B, et al. A genome-based model for adjusting radiotherapy dose (GARD): a retrospective, cohort-based study. Lancet Oncol. (2017) 18:202–11. doi: 10.1016/S1470-2045(16)30648-9
16. Pavlopoulou A, Bagos PG, Koutsandrea V, Georgakilas AG. Molecular determinants of radiosensitivity in normal and tumor tissue: a bioinformatic approach. Cancer Lett. (2017) 403:37–47. doi: 10.1016/j.canlet.2017.05.023
17. Kim HJ, Kang SK, Kwon WS, Kim TS, Jeong I, Jeung HC, et al. Forty-nine gastric cancer cell lines with integrative genomic profiling for development of c-MET inhibitor. Int J Cancer. (2018) 143:151–9. doi: 10.1002/ijc.31304
18. Jung JJ, Jeung HC, Chung HC, Lee JO, Kim TS, Kim YT, et al. In vitro pharmacogenomic database and chemosensitivity predictive genes in gastric cancer. Genomics. (2009) 93:52–61. doi: 10.1016/j.ygeno.2008.08.002
19. Storey, JD. The positive false discovery rate: a bayesian interpretation and the q-Value. Ann Statist. (2003) 31:2013–35. doi: 10.1214/aos/1074290335
20. Engelman, JA. Targeting PI3K signalling in cancer: opportunities, challenges and limitations. Nat Rev Cancer. (2009) 9:550–62. doi: 10.1038/nrc2664
21. Almhanna K, Strosberg J, Malafa M. Targeting AKT protein kinase in gastric cancer. Anticancer Res. (2011) 31:4387–92.
22. Shimura T, Kakuda S, Ochiai Y, Nakagawa H, Kuwahara Y, Takai Y, et al. Acquired radioresistance of human tumor cells by DNA-PK/AKT/GSK3beta-mediated cyclin D1 overexpression. Oncogene. (2010) 29:4826–37. doi: 10.1038/onc.2010.238
23. Schuurbiers OC, Kaanders JH, van der Heijden HF, Dekhuijzen RP, Oyen WJ, Bussink J. The PI3-K/AKT-pathway and radiation resistance mechanisms in non-small cell lung cancer. J Thorac Oncol. (2009) 4:761–7. doi: 10.1097/JTO.0b013e3181a1084f
24. Li Y, Song X, Liu Z, Li Q, Huang M, Su B, et al. Upregulation of miR-214 induced radioresistance of osteosarcoma by targeting PHLDA2 via PI3K/Akt signaling. Front Oncol. (2019) 9:298. doi: 10.3389/fonc.2019.00298
25. Ramanathan RK, McDonough SL, Kennecke HF, Iqbal S, Baranda JC, Seery TE, et al. Phase 2 study of MK-2206, an allosteric inhibitor of AKT, as second-line therapy for advanced gastric and gastroesophageal junction cancer: a SWOG cooperative group trial (S1005). Cancer. (2015) 121:2193–7. doi: 10.1002/cncr.29363
26. Shimura T, Kakuda S, Ochiai Y, Kuwahara Y, Takai Y, Fukumoto M. Targeting the AKT/GSK3beta/cyclin D1/Cdk4 survival signaling pathway for eradication of tumor radioresistance acquired by fractionated radiotherapy. Int J Radiat Oncol Biol Phys. (2011) 80:540–8. doi: 10.1016/j.ijrobp.2010.12.065
27. Narayan RS, Fedrigo CA, Brands E, Dik R, Stalpers LJ, Baumert BG, et al. The allosteric AKT inhibitor MK2206 shows a synergistic interaction with chemotherapy and radiotherapy in glioblastoma spheroid cultures. BMC Cancer. (2017) 17:204. doi: 10.1186/s12885-017-3193-9
28. Buijsen J, Lammering G, Jansen RL, Beets GL, Wals J, Sosef M, et al. Phase I trial of the combination of the Akt inhibitor nelfinavir and chemoradiation for locally advanced rectal cancer. Radiother Oncol. (2013) 107:184–8. doi: 10.1016/j.radonc.2013.03.023
29. Lin C, Verma V, Ly QP, Lazenby A, Sasson A, Schwarz JK, et al. Phase I trial of concurrent stereotactic body radiotherapy and nelfinavir for locally advanced borderline or unresectable pancreatic adenocarcinoma. Radiother Oncol. (2019) 132:55–62. doi: 10.1016/j.radonc.2018.11.002
30. Wachsberger P, Burd R, Dicker AP. Tumor response to ionizing radiation combined with antiangiogenesis or vascular targeting agents: exploring mechanisms of interaction. Clin Cancer Res. (2003) 9:1957–71.
31. Knizetova P, Ehrmann J, Hlobilkova A, Vancova I, Kalita O, Kolar Z, et al. Autocrine regulation of glioblastoma cell cycle progression, viability and radioresistance through the VEGF-VEGFR2 (KDR) interplay. Cell Cycle. (2008) 7:2553–61. doi: 10.4161/cc.7.16.6442
32. Vanpouille-Box C, Diamond JM, Pilones KA, Zavadil J, Babb JS, Formenti SC, et al. TGFbeta is a master regulator of radiation therapy-induced antitumor immunity. Cancer Res. (2015) 75:2232–42. doi: 10.1158/0008-5472.CAN-14-3511
33. Yadav P, Shankar BS. Radio resistance in breast cancer cells is mediated through TGF-beta signalling, hybrid epithelial-mesenchymal phenotype and cancer stem cells. Biomed Pharmacother. (2019) 111:119–30. doi: 10.1016/j.biopha.2018.12.055
34. Formenti SC, Lee P, Adams S, Goldberg JD, Li X, Xie MW, et al. Focal irradiation and systemic TGFbeta blockade in metastatic breast cancer. Clin Cancer Res. (2018) 24:2493–504. doi: 10.1158/1078-0432.CCR-17-3322
35. Melisi D, Garcia-Carbonero R, Macarulla T, Pezet D, Deplanque G, Fuchs M, et al. Galunisertib plus gemcitabine vs. gemcitabine for first-line treatment of patients with unresectable pancreatic cancer. Br J Cancer. (2018) 119:1208–14. doi: 10.1038/s41416-018-0246-z
36. Zou CF, Jia L, Jin H, Yao M, Zhao N, Huan J, et al. Re-expression of ARHI (DIRAS3) induces autophagy in breast cancer cells and enhances the inhibitory effect of paclitaxel. BMC Cancer (2011) 11:22. doi: 10.1186/1471-2407-11-22
37. Sherr CJ, Roberts JM. CDK inhibitors: positive and negative regulators of G1-phase progression. Genes Dev. (1999) 13:1501–12.
38. Naz S, Sowers A, Choudhuri R, Wissler M, Gamson J, Mathias A, et al. Abemaciclib, a selective CDK4/6 inhibitor, enhances the radiosensitivity of non-small cell lung cancer in vitro and in vivo. Clin Cancer Res. (2018) 24:3994–4005. doi: 10.1158/1078-0432.CCR-17-3575
39. Deng S, Yang X, Lassus H, Liang S, Kaur S, Ye Q, et al. Distinct expression levels and patterns of stem cell marker, aldehyde dehydrogenase isoform 1 (ALDH1), in human epithelial cancers. PLoS One (2010) 5:e10277. doi: 10.1371/journal.pone.0010277
40. Landen, CN Jr., Goodman B, Katre AA, Steg AD, Nick AM, Stone RL, et al. Targeting aldehyde dehydrogenase cancer stem cells in ovarian cancer. Mol Cancer Ther. (2010) 9:3186–99. doi: 10.1158/1535-7163.MCT-10-0563
41. Sandfort V, Koch U, Cordes N. Cell adhesion-mediated radioresistance revisited. Int J Radiat Biol. (2007) 83:727–32. doi: 10.1080/09553000701694335
42. Sparano JA, Gray RJ, Makower DF, Pritchard KI, Albain KS, Hayes DF, et al. Prospective validation of a 21-gene expression assay in breast cancer. N Engl J Med. (2015) 373:2005–14. doi: 10.1056/NEJMoa1510764
Keywords: radiosensitivity, gastric cancer, gene signature, Akt, MK-2206
Citation: Kim KH, Kim HS, Kim SC, Kim D, Kim YB, Chung HC and Rha SY (2020) Gene Expression Profiling Identifies Akt as a Target for Radiosensitization in Gastric Cancer Cells. Front. Oncol. 10:562284. doi: 10.3389/fonc.2020.562284
Received: 15 May 2020; Accepted: 18 August 2020;
Published: 11 September 2020.
Edited by:
Fenglin Liu, Fudan University, ChinaReviewed by:
Zsolt Kovács, University of Medicine and Pharmacy of Târgu Mureş, RomaniaCorey Speers, University of Michigan, United States
Copyright © 2020 Kim, Kim, Kim, Kim, Kim, Chung and Rha. This is an open-access article distributed under the terms of the Creative Commons Attribution License (CC BY). The use, distribution or reproduction in other forums is permitted, provided the original author(s) and the copyright owner(s) are credited and that the original publication in this journal is cited, in accordance with accepted academic practice. No use, distribution or reproduction is permitted which does not comply with these terms.
*Correspondence: Sun Young Rha, cmhhNzY1NUB5dWhzLmFj
†These authors have contributed equally to this work and share first authorship