- 1Department of Radiology, Netherlands Cancer Institute, Antoni van Leeuwenhoek, Amsterdam, Netherlands
- 2Department of Surgery, Netherlands Cancer Institute, Antoni van Leeuwenhoek, Amsterdam, Netherlands
- 3GROW School for Oncology and Developmental Biology, Maastricht University, Maastricht, Netherlands
- 4Department of Regional Health Research, University of Southern Denmark, Odense, Denmark
MR imaging (MRI) is now part of the standard work up of patients with rectal cancer. Restaging MRI has been traditionally used to plan the surgical approach. Its role has recently increased and been adopted as a valuable tool to assist the clinical selection of clinical (near) complete responders for organ preserving treatment. Recently several studies have addressed new imaging biomarkers that combined with morphological provides a comprehensive picture of the tumor. Diffusion-weighted MRI (DWI) has entered the clinics and proven useful for response assessment after chemoradiotherapy. Other functional (quantitative) MRI technologies are on the horizon including artificial intelligence modeling. This narrative review provides an overview of recent advances in rectal cancer (re)staging by imaging with a specific focus on response prediction and evaluation of neoadjuvant treatment response. Furthermore, directions are given for future research.
Introduction
In the past, rectal cancer surgery was associated with a local recurrence rate of up to 30% (1). Due to the introduction of total mesorectal excision (TME) in combination with neoadjuvant (chemo-) radiotherapy and optimal staging by MR imaging (MRI), this rate is now down to less than 3% (2). MRI plays a pivotal role in primary staging to stratify the patient to the right treatment according to the risk for local recurrence. Restaging MRI after neoadjuvant treatment can accurately show downsizing and downstaging of the tumor and plan the surgical approach. In approximately 15–20% of the patients neoadjuvant chemoradiation (CRT) leads to (near) complete response. Organ preserving approach such as a watch-and-wait (W&W) has shown to be a safe alternative for surgery provided that the patient is managed in expert centers, equipped with modern imaging and endoscopic technology and a dedicated multidisciplinary team. To be able to safely apply W&W, selection of patients with a (near) complete response is key. Functional MRI techniques capture changes in tumor perfusion and microstructure before morphological changes become apparent (3). A well-established functional MRI is diffusion-weighted MRI (DWI) which analyses the movement or “diffusion” of water molecules in which tissues with high cellularity (i.e., tumors and lymph nodes) have restricted diffusion (high signal), while normal tissue and fibrosis will lead to free diffusion (low signal). DWI is now part of the standard restaging MRI. In addition, other techniques such as perfusion MRI [dynamic contrast enhanced (DCE) MRI] and artificial intelligence modeling are being explored. This narrative review provides an overview of recent advances in rectal cancer imaging with a specific focus on response prediction and evaluation of neoadjuvant treatment response. Furthermore, directions are given for future research.
Baseline Imaging
MR imaging and endorectal ultrasound (EUS) are the established modalities for rectal cancer imaging. MRI is the most accurate modality to assess the tumor extent, nodal involvement, and guide treatment planning. For the distinction between T1 and T2 tumors, MRI is not accurate, therefore EUS is used for this specific purpose (4). However, morphological imaging does not provide information on tumor biology. Martens et al. reviewed the available literature on different volumetric methods and showed that whole-volume measurements on pre-CRT imaging reaches the highest accuracy of 71–73% for prediction of response to chemoradiotherapy (CRT) (5). Studies which focused on both MR and DWI volumetry at baseline, MRI showed low to moderate performances for predicting the response to CRT as compared to volumetric changes at post-CRT imaging (AUC of 0.57–0.73% vs. 0.63–0.77) (6, 7). The largest evidence is for DWI. A low pre-CRT apparent diffusion coefficient (ADC) at baseline DWI has by several studies shown to be significantly related with pathological complete response (pCR) and good response (6, 8–11). A possible explanation is that tumors with a high ADC value have more areas of necrosis which makes them less sensitive for radio- and chemotherapy (12). Despite some initial promising results for ADC subsequent literature showed conflicting results and considerable variability in reported cut off ADC values (13). Hence ADC measurements has not gained a significant role in response evaluation of rectal cancer treatment. Intravoxel incoherent motion (IVIM) uses low-b-values of DW-images to extract the perfusion fraction hence providing information on the tumor microvasculature without administration of intravenous contrast. Several studies show that IVIM is promising in prediction of response (14, 15). Diffusion kurtosis uses very high b-values and reflects intratumoral heterogeneity. The first few studies have not shown superiority of kurtosis imaging above ADC parameters in predicting the treatment response (16, 17). Two studies did show some potential for kurtosis imaging to predict response (18, 19). However, due to the lack of standardization and lack of strong evidence both IVIM and kurtosis imaging are currently only explored in a research setting (20).
DCE-MRI is a method which measures the inflow of intravenously injected contrast agents into the tumor and leakage of contrast into the extracellular space on T1W-MRI and provides direct information of the tissue perfusion. DCE-MRI can be analyzed quantitatively (by measurement of the perfusion of a voxel-by-voxel basis) or by semiquantitative analyses (in which a signal intensity time curve is plotted to assess parameters such as time to peak enhancement or area under the curve). DCE-MRI can provide valuable information on tumor biology (aggressiveness and the degree of angiogenesis) and initial studies have shown promise in the prediction of response (19, 21–25). Several studies showed that patients who achieved a pCR had significantly higher perfusion parameters [Ktrans, Kep (volume of extracellular space), and Ve (constant of flow rate)] than those who did not (21, 22, 25). Another group showed that the “late slope” of the signal enhancement curve after administration of contrast on baseline DCE-MRI was able to differentiate between good and poor responders with an AUC of 0.90 (23), although this study used a macromolecular blood pool contrast agent “gadofosveset” instead of the in clinics routinely applied micromolecular contrast “Gadolinium DTPA.” So far, DCE-MRI has not found its way to clinical practice due to the relatively high intra- and inter-tumor variation, need for intravenous contrast agents and lack of robustness of the technique. Research for optimization as well as standardization of the technique is much needed (4, 26). Table 1 provides an overview of the accuracy and predictive values of the different MRI techniques in a primary setting before neoadjuvant treatment.
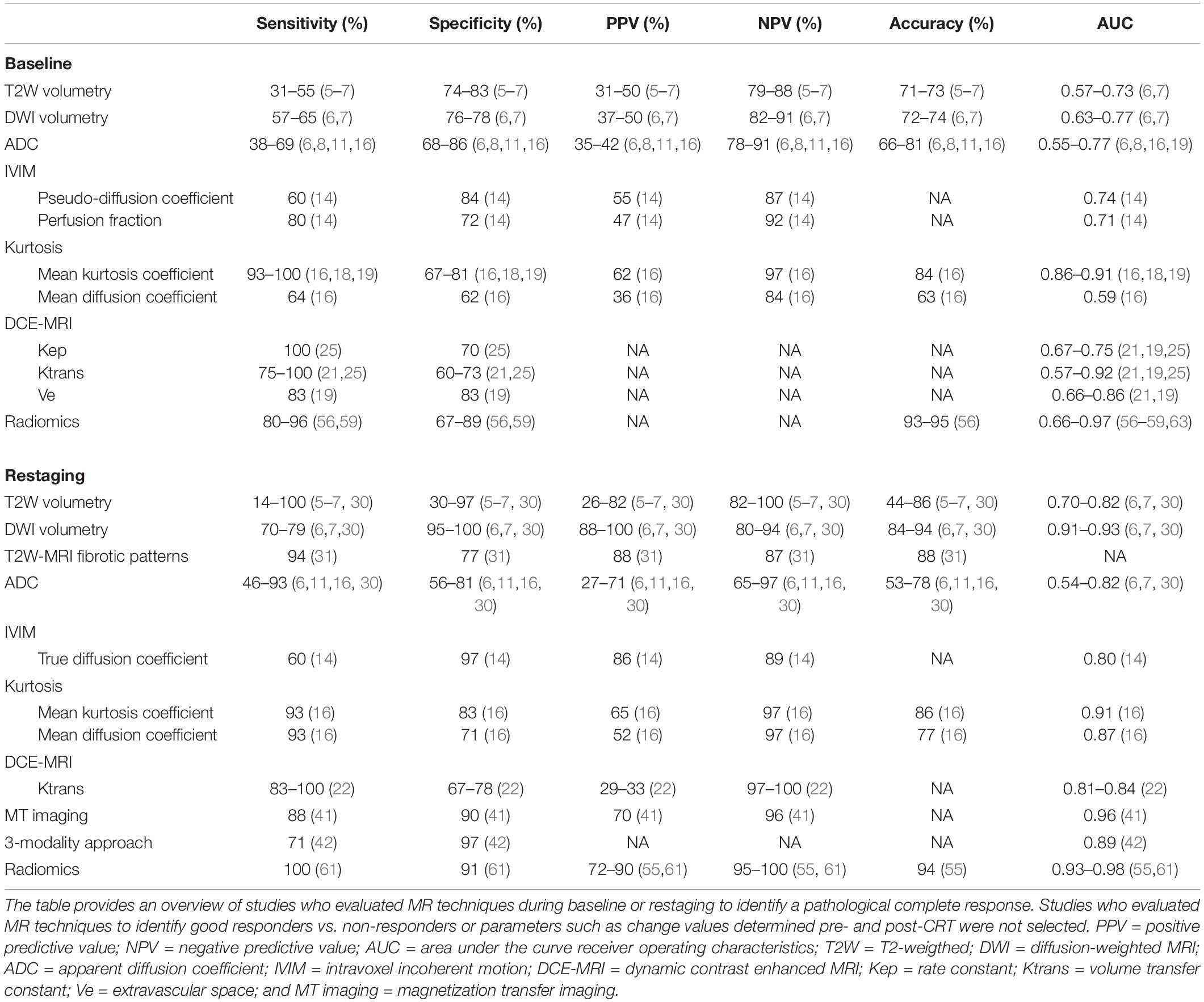
Table 1. Overview of the accuracy and predictive values of the different MRI techniques during baseline and restaging to identify pathological complete response.
Response Evaluation
As for primary staging, morphological MRI is also the main imaging modality to evaluate the luminal response and to identify extraluminal findings or remaining malignant nodes after neoadjuvant treatment. However, standard T2-weighted MRI lacks the ability to accurately evaluate response to neoadjuvant therapy because of the difficult distinction between fibrosis with and without viable tumor. Reported accuracies are 43–60% for detection of residual tumor after CRT (27–29). Studies addressing T2W and DWI volumetry have shown that the decrease in volume and absolute volume after CRT were correlated with response T2W volumetry and showed accuracies over 80% for the assessment of complete responders (6, 7). DWI volumetry using whole tumor volume manual delineation outperformed T2 volumetry (AUCs up to 0.93) in several studies (6, 7, 30). However, manual volumetry is time-consuming making it less useful to apply in clinical practice (13). Lambregts et al. proposed a method to qualitatively assess the fibrotic pattern that appears after CRT and showed that the exact type of fibrotic pattern on restaging T2W-MRI helps to evaluate the response after CRT (31). They found that the fibrotic pattern follows the pattern of the primary tumor. For example, a polypoid, or (semi)circular tumor shows a sharply demarcated semicircular fibrotic wall after CRT, and an irregular or spiculated tumor often shows irregular fibrotic thickening of the wall on restaging MRI. Only 25% of the patterns were easy to interpret, i.e., a normalized wall in the complete responders and bulky tumoral mass in the patients with residual disease. In the majority of the irradiated patients, however, different fibrotic patterns were seen (i.e., a mixed signal or irregular aspect on T2) which were difficult to interpret (31, 32). The magnetic resonance tumor regression grade (mrTRG), adapted from a similar TRG classification used in histopathology (33) categorized response into a scale from TRG-1 (only fibrosis, probably complete response) to TRG-5 (no fibrosis, probably residual disease) (34, 35). Siddiqui et al. showed that this metric has a good interobserver agreement and in 90% the radiologists correctly identified poor responders. However, in only 66% of the cases the radiologists correctly selected good responders (35). An on-going randomized controlled trial aims to assess the ability of mrTRG to direct management (36). The results of this trial will show whether mrTRG based stratification will impact outcome.
The value of functional MR parameters for response evaluation after CRT have been explored. Several studies showed that a higher value of ADC and a larger increase in ADC are both associated with a good response to CRT (10, 37, 38). A meta-analysis described pooled sensitivities and specificities of 68% and 69% for pretreatment ADC for the prediction of pCR after CRT, and of 72 and 78%, respectively, for the increase in ADC after CRT (11). One study showed that an increase of an IVIM coefficient was seen after CRT with a significantly higher value in good vs. poor responders (39). Another study showed that diffusion kurtosis imaging was feasible to assess response and superior to mrTRG (17). However, both techniques are far from ready to be implemented in clinics and remain in research setting (16, 17, 39).
A repeated finding in multiple DCE-MRI studies is that a large decrease of Ktrans after CRT is predictive for (complete) response (26). For most other (semi-) quantitative DCE parameters after CRT no robust conclusions can be drawn (26) which is the reason why DCE-MRI is not routinely applied in clinical practice. A less studied functional imaging technique is magnetization transfer (MT) imaging (traditionally applied in brain imaging). MT imaging explores differences in the magnetization interaction. The transfer of magnetization (MT ratio) between protons bound to macromolecules (collagen) and free/unbound water protons is high in case of collagen rich tissue (fibrosis) and may be useful to discriminate residual disease from post-CRT fibrosis (40, 41). Yet evidence is limited and MT imaging is only explored in research setting.
The highest accuracy to identify complete responders after CRT has been reached with the use of a three-modality approach (42) including digital rectal exam (DRE) with endoscopy, T2W-MRI, and DWI. Maas et al. described that when the combination of DRE, endoscopy, and T2W-MRI and DWI all indicate a complete response, this diagnosis is correct in 98% of the patients (42) Figure 1. Endoscopy was shown to be an invaluable tool for response evaluation, with MRI being an important adjunct to assess the extramural parts of the tumor and nodes. This method has been adopted globally in the selection of patients for organ preservation (43). Despite the good results, up to 15% of complete responders are still missed, due to the fact that many complete responding patients may show some findings that are often associated with residual tumor (e.g., ulcers at endoscopy, focal diffusion signal on DWI, irregular nodes on T2W-MRI among others) (44–46). Unfortunately, biopsies are of limited clinical value in this setting, because of the risk for sampling error and a risk for false positive findings (e.g., dysplasia in biopsy, but complete response in the TME specimen) (44, 46–48). An overview of the accuracy and predictive values of the different MRI techniques during restaging is given in Table 1.
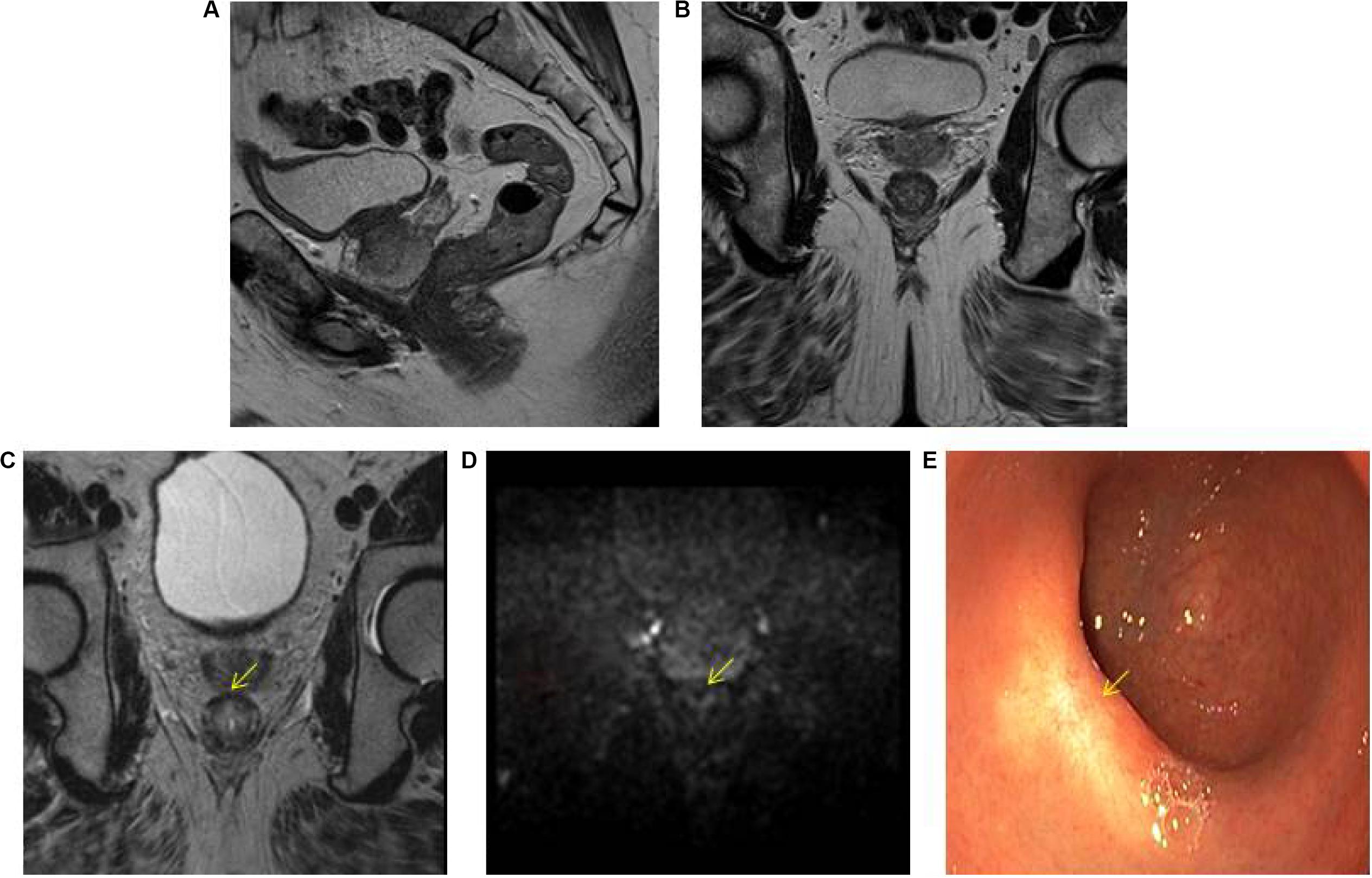
Figure 1. Three-modality approach with combination of T2W-MRI, DWI, and endoscopy of the selection of a patient with a clinical complete response. A low rectal tumor is seen on MRI (A, B). On restaging MRI 8 weeks after completion of chemoradiotherapy (C) only minimal fibrosis (yellow arrow) is seen anteriorly in the rectal wall. On restaging DWI (D) there is absence of high diffusion signal (yellow arrow). Clinical assessment by endoscopy (E) reveals a white scar with telangiectasia (yellow arrow).
Artificial Intelligence
Artificial intelligence models hold promise in cancer imaging. These approaches aim to use computer algorithms to find associations between quantitative imaging features and clinical outcome. This procedure is termed Radiomics, and it can be carried out in a variety of different ways. The most common approach is to use pre-defined, general purpose quantitative imaging features that describe intensity distribution, tumor shape, and heterogeneity. More modern technique, such as deep learning, allows the computer to learn problem-specific imaging features leading to more robust models (49). Independently from the approach chosen, radiomics features can be combined with clinical and pathological data (possibly also extracted in the same fashion, i.e., pathomics) to predict clinical outcome, such as response to therapy (50). Across most radiomics studies, it is noted how non-visual information relating to tumor heterogeneity is an important biomarker for response prediction (51, 52). So far, radiomics has been applied to many tumor types (liver, lung, head and neck, and brain) using varying modalities (CT, MRI, 18F-FDG PET/CT) with promising results (50, 53, 54). Many studies have evaluated MR-based radiomics signatures (55–61), with a main focus on response evaluation. It is important to consider the technical challenges when applying radiomics on MR-images: problems with standardization, normalization and regularization of images may hamper the generalizability of radiomics models (62). Despite these difficulties, so far promising results have been found in response prediction (56–59, 63) and response evaluation (55, 60, 61, 64). Cui et al., for example, showed a favorable prognostic performance to predict pCR with radiomics on pre-CRT MR-images (AUCs of 0.94–0.97) (56), but other studies reported lower accuracies (AUCs of 0.69–0.79) (57–59, 63) (Table 1). Van Griethuysen et al. showed that radiomics on pre-CRT MR-images could predict response to therapy on image segmentation with comparable diagnostic performance as expert radiologists, regardless of their experience on image segmentation (63). On post-CRT MR T2W-images, Horvat et al. showed that radiomics had a better classification performance compared to the combination of DWI and T2W-MRI to identify pCR, with a significant higher specificity and PPV (91% vs. 56%; 72% vs 30%) (61). However, sensitivity and NPV were not significantly different. Another study concluded that radiomics could be used as an additional tool for clinical decision making on post-CRT imaging (64). Until now, only single center studies using a heterogeneous methodology have been performed. Additionally, external validation of findings in radiomics research is often lacking, which is an important prerequisite to eventually apply developed predictive radiomics models in clinical practice. Currently, initiatives are being taken to deal with standardization of radiomics analyses and start up large datasets in order to facilitate external validation by international collaborations.
Discussion
During the past decades advances in MR imaging technology and in image analysis and post processing methods have opened new windows of opportunity for research that will foster further personalization of treatment. Patients with smaller tumors will undergo neoadjuvant treatment with the aim to achieve a complete response and to offer organ preserving treatment. Main clinical questions concern the ability of a non-invasive imaging tool to accurately select before the onset of treatment those patients who are likely to achieve a (near) complete response. It is expected that functional parametric MRI will perform superior to conventional MRI because the combination of morphological and functional data provides a comprehensive information on the tumor. More advanced metrics derived from DWI perfusion and kurtosis imaging as well as artificial intelligence modeling are promising but currently only the subject of research.
Author Contributions
HH drafted the manuscript. ST helped to draft the artificial intelligence section. MM and RB-T helped to draft and review the manuscript. All authors read and approved the final manuscript.
Conflict of Interest
The authors declare that the research was conducted in the absence of any commercial or financial relationships that could be construed as a potential conflict of interest.
References
1. Hermanek P, Wiebelt H, Staimmer D, Riedl S. Prognostic factors of rectum carcinoma–experience of the German multicentre study SGCRC. German study group colo-rectal carcinoma. Tumori. (1995) 81(3 Suppl.):60–4.
2. Maas M, Nelemans PJ, Valentini V, Das P, Rodel C, Kuo LJ, et al. Long-term outcome in patients with a pathological complete response after chemoradiation for rectal cancer: a pooled analysis of individual patient data. Lancet Oncol. (2010) 11:835–44. doi: 10.1016/s1470-2045(10)70172-8
3. Joye I, Debucquoy A, Deroose CM, Vandecaveye V, Cutsem EV, Wolthuis A, et al. Quantitative imaging outperforms molecular markers when predicting response to chemoradiotherapy for rectal cancer. Radiother Oncol. (2017) 124:104–9. doi: 10.1016/j.radonc.2017.06.013
4. Beets-Tan RGH, Lambregts DMJ, Maas M, Bipat S, Barbaro B, Curvo-Semedo L, et al. Magnetic resonance imaging for clinical management of rectal cancer: updated recommendations from the 2016 European society of gastrointestinal and abdominal radiology (ESGAR) consensus meeting. Eur Radiol. (2018) 28:1465–75. doi: 10.1007/s00330-017-5026-2
5. Martens MH, Van Heeswijk MM, Van Den Broek JJ, Rao S-X, Vandecaveye V, Vliegen RA, et al. Prospective, multicenter validation study of magnetic resonance volumetry for response assessment after preoperative chemoradiation in rectal cancer: can the results in the literature be reproduced? Int J Radiat Oncol Biol Phys. (2015) 93:1005–14. doi: 10.1016/j.ijrobp.2015.09.008
6. Curvo-Semedo L, Lambregts DMJ, Maas M, Thywissen T, Mehsen RT, Lammering G, et al. Rectal cancer: assessment of complete response to preoperative combined radiation therapy with chemotherapy—conventional MR volumetry versus diffusion-weighted MR imaging. Radiology. (2011) 260:734–43. doi: 10.1148/radiol.11102467
7. Lambregts DM, Rao SX, Sassen S, Martens MH, Heijnen LA, Buijsen J, et al. MRI and diffusion-weighted MRI Volumetry for identification of complete tumor responders after preoperative chemoradiotherapy in patients with rectal cancer: a Bi-institutional validation study. Ann Surg. (2015) 262:1034–9. doi: 10.1097/sla.0000000000000909
8. Intven M, Reerink O, Philippens ME. Diffusion-weighted MRI in locally advanced rectal cancer: pathological response prediction after neo-adjuvant radiochemotherapy. Strahlenther Onkol. (2013) 189:117–22. doi: 10.1007/s00066-012-0270-5
9. Sun Y-S, Zhang X-P, Tang L, Ji J-F, Gu J, Cai Y, et al. Locally advanced rectal carcinoma treated with preoperative chemotherapy and radiation therapy: preliminary analysis of diffusion-weighted MR imaging for early detection of tumor histopathologic downstaging. Radiology. (2010) 254:170–8.
10. Jung SH, Heo SH, Kim JW, Jeong YY, Shin SS, Soung M-G, et al. Predicting response to neoadjuvant chemoradiation therapy in locally advanced rectal cancer: diffusion-weighted 3 tesla MR imaging. J Magn Reson Imaging. (2012) 35:110–6. doi: 10.1002/jmri.22749
11. Joye I, Deroose CM, Vandecaveye V, Haustermans K. The role of diffusion-weighted MRI and 18F-FDG PET/CT in the prediction of pathologic complete response after radiochemotherapy for rectal cancer: a systematic review. Radiother Oncol. (2014) 113:158–65. doi: 10.1016/j.radonc.2014.11.026
12. Herneth AM, Guccione S, Bednarski M. Apparent diffusion coefficient: a quantitative parameter for in vivo tumor characterization. Eur J Radiol. (2003) 45:208–13. doi: 10.1016/s0720-048x(02)00310-8
13. Lambregts DMJ, Boellaard TN, Beets-Tan RGH. Response evaluation after neoadjuvant treatment for rectal cancer using modern MR imaging: a pictorial review. Insights Imaging. (2019) 10:15.
14. Lu W, Jing H, Ju-Mei Z, Shao-Lin N, Fang C, Xiao-Ping Y, et al. Intravoxel incoherent motion diffusion-weighted imaging for discriminating the pathological response to neoadjuvant chemoradiotherapy in locally advanced rectal cancer. Sci Rep. (2017) 7:8496.
15. Bakke KM, Hole KH, Dueland S, Grøholt KK, Flatmark K, Ree AH, et al. Diffusion-weighted magnetic resonance imaging of rectal cancer: tumour volume and perfusion fraction predict chemoradiotherapy response and survival. Acta Oncol. (2017) 56:813–8. doi: 10.1080/0284186x.2017.1287951
16. Hu F, Tang W, Sun Y, Wan D, Cai S, Zhang Z, et al. The value of diffusion kurtosis imaging in assessing pathological complete response to neoadjuvant chemoradiation therapy in rectal cancer: a comparison with conventional diffusion-weighted imaging. Oncotarget. (2017) 8:75597–606. doi: 10.18632/oncotarget.17491
17. Yu J, Xu Q, Song JC, Li Y, Dai X, Huang DY, et al. The value of diffusion kurtosis magnetic resonance imaging for assessing treatment response of neoadjuvant chemoradiotherapy in locally advanced rectal cancer. Eur Radiol. (2017) 27:1848–57. doi: 10.1007/s00330-016-4529-6
18. De Cecco CN, Ganeshan B, Ciolina M, Rengo M, Meinel FG, Musio D, et al. Texture analysis as imaging biomarker of tumoral response to neoadjuvant chemoradiotherapy in rectal cancer patients studied with 3-T magnetic resonance. Invest Radiol. (2015) 50:239–45. doi: 10.1097/rli.0000000000000116
19. De Cecco CN, Ciolina M, Caruso D, Rengo M, Ganeshan B, Meinel FG, et al. Performance of diffusion-weighted imaging, perfusion imaging, and texture analysis in predicting tumoral response to neoadjuvant chemoradiotherapy in rectal cancer patients studied with 3T MR: initial experience. Abdom Radiol. (2016) 41:1728–35. doi: 10.1007/s00261-016-0733-8
20. Schurink NW, Lambregts DMJ, Beets-Tan RGH. Diffusion-weighted imaging in rectal cancer: current applications and future perspectives. Br J Radiol. (2019) 92:20180655. doi: 10.1259/bjr.20180655
21. Tong T, Sun Y, Gollub MJ, Peng W, Cai S, Zhang Z, et al. Dynamic contrast-enhanced MRI: use in predicting pathological complete response to neoadjuvant chemoradiation in locally advanced rectal cancer. J Magn Reson Imaging. (2015) 42:673–80. doi: 10.1002/jmri.24835
22. Intven M, Reerink O, Philippens ME. Dynamic contrast enhanced MR imaging for rectal cancer response assessment after neo-adjuvant chemoradiation. J Magn Reson Imaging. (2015) 41:1646–53. doi: 10.1002/jmri.24718
23. Martens MH, Subhani S, Heijnen LA, Lambregts DM, Buijsen J, Maas M, et al. Can perfusion MRI predict response to preoperative treatment in rectal cancer? Radiother Oncol. (2015) 114:218–23. doi: 10.1016/j.radonc.2014.11.044
24. Dijkhoff RAP, Maas M, Martens MH, Papanikolaou N, Lambregts DMJ, Beets GL, et al. Correlation between quantitative and semiquantitative parameters in DCE-MRI with a blood pool agent in rectal cancer: can semiquantitative parameters be used as a surrogate for quantitative parameters? Abdom Radiol. (2017) 42:1342–9. doi: 10.1007/s00261-016-1024-0
25. Ciolina M, Caruso D, De Santis D, Zerunian M, Rengo M, Alfieri N, et al. Dynamic contrast-enhanced magnetic resonance imaging in locally advanced rectal cancer: role of perfusion parameters in the assessment of response to treatment. Radiol Med. (2019) 124:331–8. doi: 10.1007/s11547-018-0978-0
26. Dijkhoff RAP, Beets-Tan RGH, Lambregts DMJ, Beets GL, Maas M. Value of DCE-MRI for staging and response evaluation in rectal cancer: a systematic review. Eur J Radiol. (2017) 95:155–68. doi: 10.1016/j.ejrad.2017.08.009
27. Kulkarni T, Gollins S, Maw A, Hobson P, Byrne R, Widdowson D. Magnetic resonance imaging in rectal cancer downstaged using neoadjuvant chemoradiation: accuracy of prediction of tumour stage and circumferential resection margin status. Colorectal Dis. (2008) 10:479–89. doi: 10.1111/j.1463-1318.2007.01451.x
28. Dresen RC, Beets GL, Rutten HJ, Engelen SM, Lahaye MJ, Vliegen RF, et al. Locally advanced rectal cancer: MR imaging for restaging after neoadjuvant radiation therapy with concomitant chemotherapy. Part I. Are we able to predict tumor confined to the rectal wall? Radiology. (2009) 252:71–80. doi: 10.1148/radiol.2521081200
29. Allen SD, Padhani AR, Dzik-Jurasz AS, Glynne-Jones R. Rectal carcinoma: MRI with histologic correlation before and after chemoradiation therapy. AJR Am J Roentgenol. (2007) 188:442–51. doi: 10.2214/ajr.05.1967
30. Ha HI, Kim AY, Yu CS, Park SH, Ha HK. Locally advanced rectal cancer: diffusion-weighted MR tumour volumetry and the apparent diffusion coefficient for evaluating complete remission after preoperative chemoradiation therapy. Eur Radiol. (2013) 23:3345–53. doi: 10.1007/s00330-013-2936-5
31. Lambregts DMJ, Delli Pizzi A, Lahaye MJ, van Griethuysen JJM, Maas M, Beets GL, et al. A pattern-based approach combining tumor morphology on MRI with distinct signal patterns on diffusion-weighted imaging to assess response of rectal tumors after chemoradiotherapy. Dis Colon Rectum. (2018) 61:328–37.
32. Lambregts DMJ, Maas M, Boellaard TN, Pizzi AD, Van Der Sande ME, Hupkens BJP, et al. Long-term imaging characteristics of clinical complete responders during watch-and-wait for rectal cancer—an evaluation of over 1500 MRIs. Eur Radiol. (2020) 30:272–80. doi: 10.1007/s00330-019-06396-1
33. Mandard AM, Dalibard F, Mandard JC, Marnay J, Henry-Amar M, Petiot JF, et al. Pathologic assessment of tumor regression after preoperative chemoradiotherapy of esophageal carcinoma. Clinicopathologic correlations. Cancer. (1994) 73:2680–6. doi: 10.1002/1097-0142(19940601)73:11<2680::aid-cncr2820731105>3.0.co;2-c
34. Patel UB, Taylor F, Blomqvist L, George C, Evans H, Tekkis P, et al. Magnetic resonance imaging-detected tumor response for locally advanced rectal cancer predicts survival outcomes: MERCURY experience. J Clin Oncol. (2011) 29:3753–60. doi: 10.1200/jco.2011.34.9068
35. Siddiqui MR, Gormly KL, Bhoday J, Balyansikova S, Battersby NJ, Chand M, et al. Interobserver agreement of radiologists assessing the response of rectal cancers to preoperative chemoradiation using the MRI tumour regression grading (mrTRG). Clin Radiol. (2016) 71:854–62. doi: 10.1016/j.crad.2016.05.005
36. Battersby NJ, Dattani M, Rao S, Cunningham D, Tait D, Adams R, et al. A rectal cancer feasibility study with an embedded phase III trial design assessing magnetic resonance tumour regression grade (mrTRG) as a novel biomarker to stratify management by good and poor response to chemoradiotherapy (TRIGGER): study protocol for a randomised controlled trial. Trials. (2017) 18:394.
37. Monguzzi L, Ippolito D, Bernasconi DP, Trattenero C, Galimberti S, Sironi S. Locally advanced rectal cancer: value of ADC mapping in prediction of tumor response to radiochemotherapy. Eur J Radiol. (2013) 82:234–40. doi: 10.1016/j.ejrad.2012.09.027
38. Ippolito D, Monguzzi L, Guerra L, Deponti E, Gardani G, Messa C, et al. Response to neoadjuvant therapy in locally advanced rectal cancer: assessment with diffusion-weighted MR imaging and 18FDG PET/CT. Abdom Imaging. (2012) 37:1032–40. doi: 10.1007/s00261-011-9839-1
39. Nougaret S, Vargas HA, Lakhman Y, Sudre R, Do RKG, Bibeau F, et al. Intravoxel incoherent motion–derived histogram metrics for assessment of response after combined chemotherapy and radiation therapy in rectal cancer: initial experience and comparison between single-section and volumetric analyses. Radiology. (2016) 280:446–54. doi: 10.1148/radiol.2016150702
40. Martens MH, Lambregts DMJ, Papanikolaou N, Alefantinou S, Maas M, Manikis GC, et al. Magnetization transfer imaging to assess tumour response after chemoradiotherapy in rectal cancer. Eur Radiol. (2016) 26:390–7. doi: 10.1007/s00330-015-3856-3
41. Martens MH, Lambregts DM, Papanikolaou N, Heijnen LA, Riedl RG, zur Hausen A, et al. Magnetization transfer ratio: a potential biomarker for the assessment of postradiation fibrosis in patients with rectal cancer. Invest Radiol. (2014) 49:29–34. doi: 10.1097/rli.0b013e3182a3459b
42. Maas M, Lambregts DM, Nelemans PJ, Heijnen LA, Martens MH, Leijtens JW, et al. Assessment of clinical complete response after chemoradiation for rectal cancer with digital rectal examination, endoscopy, and MRI: selection for organ-saving treatment. Ann Surg Oncol. (2015) 22:3873–80. doi: 10.1245/s10434-015-4687-9
43. van der Valk MJM, Hilling DE, Bastiaannet E, Meershoek-Klein Kranenbarg E, Beets GL, Figueiredo NL, et al. Long-term outcomes of clinical complete responders after neoadjuvant treatment for rectal cancer in the International Watch & Wait Database (IWWD): an international multicentre registry study. Lancet. (2018) 391:2537–45.
44. van der Sande ME, Beets GL, Hupkens BJ, Breukink SO, Melenhorst J, Bakers FC, et al. Response assessment after (chemo)radiotherapy for rectal cancer: Why are we missing complete responses with MRI and endoscopy? Eur J Surg Oncol. (2018) 45:1011–7. doi: 10.1016/j.ejso.2018.11.019
45. Nahas SC, Rizkallah Nahas CS, Sparapan Marques CF, Ribeiro U Jr., Cotti GC, Imperiale AR, et al. Pathologic complete response in rectal cancer: can we detect it? Lessons learned from a proposed randomized trial of watch-and-wait treatment of rectal cancer. Dis Colon Rectum. (2016) 59:255–63. doi: 10.1097/dcr.0000000000000558
46. Smith FM, Wiland H, Mace A, Pai RK, Kalady MF. Clinical criteria underestimate complete pathological response in rectal cancer treated with neoadjuvant chemoradiotherapy. Dis Colon Rectum. (2014) 57:311–5. doi: 10.1097/dcr.0b013e3182a84eba
47. Perez RO, Habr-Gama A, Pereira GV, Lynn PB, Alves PA, Proscurshim I, et al. Role of biopsies in patients with residual rectal cancer following neoadjuvant chemoradiation after downsizing: can they rule out persisting cancer? Colorectal Dis. (2012) 14:714–20. doi: 10.1111/j.1463-1318.2011.02761.x
48. Beets GL, Figueiredo NF, Beets-Tan RG. Management of rectal cancer without radical resection. Annu Rev Med. (2017) 68:169–82. doi: 10.1146/annurev-med-062915-021419
49. Trebeschi S, Van Griethuysen JJM, Lambregts DMJ, Lahaye MJ, Parmar C, Bakers FCH, et al. Deep learning for fully-automated localization and segmentation of rectal cancer on multiparametric MR. Sci Rep. (2017) 7:5301.
50. Aerts HJ, Velazquez ER, Leijenaar RT, Parmar C, Grossmann P, Carvalho S, et al. Decoding tumour phenotype by non-invasive imaging using a quantitative radiomics approach. Nat Commun. (2014) 5:4006.
51. Gillies RJ, Kinahan PE, Hricak H. Radiomics: images are more than pictures, they are data. Radiology. (2016) 278:563–77. doi: 10.1148/radiol.2015151169
52. Kiessling F. The changing face of cancer diagnosis: from computational image analysis to systems biology. Eur Radiol. (2018) 28:3160–4. doi: 10.1007/s00330-018-5347-9
53. Bakr S, Echegaray S, Shah R, Kamaya A, Louie J, Napel S, et al. Non-invasive radiomics signature based on quantitative analysis of computed tomography images as a surrogate for microvascular invasion in hepatocellular carcinoma: a pilot study. J Med Imaging. (2017) 4:041303.
54. Zhou M, Scott J, Chaudhury B, Hall L, Goldgof D, Yeom KW, et al. Radiomics in brain tumor: image assessment, quantitative feature descriptors, and machine-learning approaches. AJNR Am J Neuroradiol. (2018) 39:208–16. doi: 10.3174/ajnr.a5391
55. Liu Z, Zhang X-Y, Shi Y-J, Wang L, Zhu H-T, Tang Z, et al. Radiomics analysis for evaluation of pathological complete response to neoadjuvant chemoradiotherapy in locally advanced rectal cancer. Clin Cancer Res. (2017) 23:7253–62. doi: 10.1158/1078-0432.ccr-17-1038
56. Cui Y, Yang X, Shi Z, Yang Z, Du X, Zhao Z, et al. Radiomics analysis of multiparametric MRI for prediction of pathological complete response to neoadjuvant chemoradiotherapy in locally advanced rectal cancer. Eur Radiol. (2019) 29:1211–20. doi: 10.1007/s00330-018-5683-9
57. Cusumano D, Dinapoli N, Boldrini L, Chiloiro G, Gatta R, Masciocchi C, et al. Fractal-based radiomic approach to predict complete pathological response after chemo-radiotherapy in rectal cancer. Radiol Med. (2018) 123:286–95. doi: 10.1007/s11547-017-0838-3
58. Dinapoli N, Barbaro B, Gatta R, Chiloiro G, Casà C, Masciocchi C, et al. Magnetic resonance, vendor-independent, intensity histogram analysis predicting pathologic complete response after radiochemotherapy of rectal cancer. Int J Radiat Oncol Biol Phys. (2018) 102:765–74. doi: 10.1016/j.ijrobp.2018.04.065
59. Meng Y, Zhang C, Zou S, Zhao X, Xu K, Zhang H, et al. MRI texture analysis in predicting treatment response to neoadjuvant chemoradiotherapy in rectal cancer. Oncotarget. (2018) 9:11999–2008. doi: 10.18632/oncotarget.23813
60. Bulens P, Couwenberg A, Haustermans K, Debucquoy A, Vandecaveye V, Philippens M, et al. Development and validation of an MRI-based model to predict response to chemoradiotherapy for rectal cancer. Radiother Oncol. (2018) 126:437–42. doi: 10.1016/j.radonc.2018.01.008
61. Horvat N, Veeraraghavan H, Khan M, Blazic I, Zheng J, Capanu M, et al. MR imaging of rectal cancer: radiomics analysis to assess treatment response after neoadjuvant therapy. Radiology. (2018) 287:833–43. doi: 10.1148/radiol.2018172300
62. Collewet G, Strzelecki M, Mariette F. Influence of MRI acquisition protocols and image intensity normalization methods on texture classification. Magn Reson Imaging. (2004) 22:81–91. doi: 10.1016/j.mri.2003.09.001
63. van Griethuysen JJM, Lambregts DMJ, Trebeschi S, Lahaye MJ, Bakers FCH, Vliegen RFA, et al. Radiomics performs comparable to morphologic assessment by expert radiologists for prediction of response to neoadjuvant chemoradiotherapy on baseline staging MRI in rectal cancer. Abdom Radiol. (2020) 45:632–43. doi: 10.1007/s00261-019-02321-8
Keywords: rectal cancer, MR imaging, organ preservation, functional imaging, artificial intelligence
Citation: Haak HE, Maas M, Trebeschi S and Beets-Tan RGH (2020) Modern MR Imaging Technology in Rectal Cancer; There Is More Than Meets the Eye. Front. Oncol. 10:537532. doi: 10.3389/fonc.2020.537532
Received: 24 February 2020; Accepted: 02 September 2020;
Published: 08 October 2020.
Edited by:
Alexandra Zaborowski, St. Vincent’s University Hospital, IrelandReviewed by:
Francesca De Felice, Sapienza University of Rome, ItalyAlexander Heriot, Peter MacCallum Cancer Centre, Australia
Copyright © 2020 Haak, Maas, Trebeschi and Beets-Tan. This is an open-access article distributed under the terms of the Creative Commons Attribution License (CC BY). The use, distribution or reproduction in other forums is permitted, provided the original author(s) and the copyright owner(s) are credited and that the original publication in this journal is cited, in accordance with accepted academic practice. No use, distribution or reproduction is permitted which does not comply with these terms.
*Correspondence: Regina G. H. Beets-Tan, r.beetstan@nki.nl; Hester E. Haak, h.haak@nki.nl