- 1Institute of Bioinformatics, International Technology Park, Bangalore, India
- 2Amrita School of Biotechnology, Amrita Vishwa Vidyapeetham, Kollam, India
- 3Medgenome Labs Pvt. Ltd., Bangalore, India
- 4Manipal Academy of Higher Education, Manipal, India
- 5Department of Pathology, Kidwai Memorial Institute of Oncology, Bangalore, India
- 6Center for Systems Biology and Molecular Medicine, Yenepoya Research Centre, Yenepoya (Deemed to be University), Mangalore, India
- 7Department of Laboratory Medicine and Pathology, Mayo Clinic, Rochester, MN, United States
- 8Center for Individualized Medicine, Mayo Clinic, Rochester, MN, United States
- 9Center for Molecular Medicine, National Institute of Mental Health and Neurosciences, Bangalore, India
- 10Genetics and Computational Biology, QIMR Berghofer Medical Research Institute, Brisbane, QLD, Australia
Esophageal squamous cell carcinoma (ESCC) is the most common histological subtype of esophageal cancer in India. Cigarette smoking and chewing tobacco are known risk factors associated with ESCC. However, genomic alterations associated with ESCC in India are not well-characterized. In this study, we carried out exome sequencing to characterize the mutational landscape of ESCC tumors from subjects with a varied history of tobacco usage. Whole exome sequence analysis of ESCC from an Indian cohort revealed several genes that were mutated or had copy number changes. ESCC from tobacco chewers had a higher frequency of C:G > A:T transversions and 2-fold enrichment for mutation signature 4 compared to smokers and non-users of tobacco. Genes, such as TP53, CSMD3, SYNE1, PIK3CA, and NOTCH1 were found to be frequently mutated in Indian cohort. Mutually exclusive mutation patterns were observed in PIK3CA–NOTCH1, DNAH5–ZFHX4, MUC16–FAT1, and ZFHX4–NOTCH1 gene pairs. Recurrent amplifications were observed in 3q22-3q29, 11q13.3-q13.4, 7q22.1-q31.1, and 8q24 regions. Approximately 53% of tumors had genomic alterations in PIK3CA making this pathway a promising candidate for targeted therapy. In conclusion, we observe enrichment of mutation signature 4 in ESCC tumors from patients with a history of tobacco chewing. This is likely due to direct exposure of esophagus to tobacco carcinogens when it is chewed and swallowed. Genomic alterations were frequently observed in PIK3CA-AKT pathway members independent of the history of tobacco usage. PIK3CA pathway can be potentially targeted in ESCC which currently has no effective targeted therapeutic options.
Introduction
Esophageal cancer is the eighth most common cancer in the world and the sixth most common cause of cancer-related mortality (1). Histologically, there are two major types of esophageal cancer—Esophageal Squamous Cell Carcinoma (ESCC) and Esophageal Adenocarcinoma (EAC) with distinct geographical patterns of incidences across the globe (2). China has the highest burden of esophageal cancer in the world. The incidence and mortality rate is projected to increase in the future, especially in regions extending from Northeast China to Middle East China which is termed as esophageal cancer belt (3, 4). ESCC is generally diagnosed at advanced stages and hence its prognosis is poor. The overall 5-years survival rate of patients with advanced-stage cancer is lower than 15% (5). Surgery remains a major therapeutic option for ESCC and the standard chemotherapy regimen includes cisplatin and 5-fluorouracil (6). Thus, identification of novel therapeutic targets is necessary for better treatment strategies in ESCC.
In India, cancers associated with tobacco consumption accounts for 42% of total cancer-related deaths (7). Tobacco is consumed in different forms including cigarette smoking and smokeless tobacco products, such as khaini, gutka, betel quid, etc. As per the Global Adult Tobacco Survey (GATS), 34.6% of the adult Indian population consumes tobacco as cigarette and/or smokeless form by chewing and swallowing the extract. Cigarette smoking is a known risk factor for ESCC. Smoking is associated with poor prognosis as overall survival among ESCC patients is poor in smokers compared to non-smokers (8–11). However, molecular alterations associated with varied tobacco consumption in ESCC have not been well-studied.
Multiple studies from Chinese and Japanese cohort have characterized genomic anomalies in ESCC tumors including those from cigarette smokers to investigate mutational landscape and elucidation of potential driver genes (12–15). For instance, Song et al. studied ESCC using whole genome sequencing (WGS) of 17 and whole exome sequencing (WES) of 71 ESCC samples and reported ADAM29 and FAM1335B as two significantly mutated genes along with frequently mutated genes, such as TP53, PIK3CA, NOTCH1, and NFE2L2 (13). Lin et al. carried out WES analysis of 139 ESCC samples and identified ZNF750 and FAT1 as tumor suppressors which are frequently disrupted in ESCC (12). A study on ESCC from the Japanese cohort has reported an enrichment of drinking and smoking-related mutation signature which are not observed in the Chinese cohort (14). Despite the high incidence of ESCC in India and known association of tobacco consumption in both smoke and smokeless form, there are no reports on the mutation landscape of ESCC tumors from an Indian cohort. Hence, we employed whole exome sequencing analysis of 28 ESCC samples from an Indian cohort to characterize genomic alterations from patients with history of smoking or chewing tobacco and those with no history of tobacco consumption.
Materials and Methods
Study Cohort
ESCC tumor and paired adjacent normal tissue samples were collected from Kidwai Memorial Institute of Oncology, Bangalore, India. Informed consent from all the patients and ethical clearance were obtained from Kidwai Memorial Institute of Oncology, Bangalore. History of smoking and/or chewing tobacco was determined based on self-reporting by patients. Patients in the non-users group had no history of tobacco consumption. Details of 28 ESCC patients enrolled in this study and their history of tobacco consumption are provided in Supplementary Table 1. All the samples were stored at −80°C until further processing. The project was approved by institutional biosafety committee at the Institute of Bioinformatics.
Whole Exome Sequence Analysis
DNA library for whole exome sequencing was prepared using Agilent SureSelectXT Human All Exon V5 kit using genomic DNA extracted from tissue samples. Genomic DNA was subjected to sonication using M220 Focused-ultrasonicator™ to produce sheared fragments of 150–200 bp length. Sheared DNA fragments were end-repaired and phosphorylated, adenylated at 3′ ends followed by ligation of standard paired-end adaptors. Hybridization reaction was carried out at 65°C for 16 h using a DNA library with the addition of biotin-labeled RNA probe sets. Resulting DNA-RNA duplexes were captured using Dynabeads® MyOne™ Streptavidin T1 beads. Amplification of the specific libraries was done using indexed primers and Herculase II Fusion DNA Polymerase (Agilent Technologies Inc.). Subsequently, cluster amplification was performed according to the manufacturer's protocol (Illumina Inc.). Paired-end sequencing was performed on Illumina HiSeq 2500 platform with a read length of 100 bp. Raw reads acquired in FASTQ format were assessed for Phred score using FastQC (16). To increase the accuracy and specificity of read mapping, bases with Phred score <20 were removed and trimmed reads with length ≥35 bps were retained for further analysis. Reads were aligned against reference genome hg19 (GRCh37) using BWA (Burrows-Wheeler Aligner)-MEM (Maximal Exact Matches) (17) with default parameters. Binary alignment map (BAM) files were further processed using GATK tool suite (Genome Analysis Toolkit, Broad Institute) which includes removal of duplicates using MarkDuplicates of Picard tools, indel realignment using IndelRealigner, and base recalibration using BaseRecalibrator. High confidence somatic single nucleotide variants (SNVs) were filtered using Strelka (18) and annotated using VariMAT. Identification of genes with mutually exclusive variants, oncoprints, and lollipop plots of somatic SNVs were generated using cBioPortal (19, 20).
Mutational signatures were deduced using R package SomaticSignatures (21). Somatic mutational signatures for each cohort were further deconstructed using Mutalisk to determine the presence of different COSMIC signatures in each cohort (22). Copy number alterations (CNAs) were inferred using OncoCNV and amplification with fold change >3 was considered CNA gain and <1 was considered CNA loss with p-value threshold 1 × 10−5 (23). Genes recurrently affected by CNAs in at least 5 samples were queried against DGIdb 3.0 database to identify potential druggable targets (24). Clinically actionable therapeutic targets were predicted using The Drug Gene Interaction Database (DGIdb). The database has curated list of drug-gene interactions compiled from various resources. A list of genes with coding mutations and/or amplified in at least five samples were queried against the database by choosing “Clinically actionable” gene category and keeping default source databases of DGIdb as background. “Druggable Genome” category was used to identify potentially druggable targets in ESCC. The Integrative Genomics Viewer (IGV) was used to visualize WES datasets (25) and boxplots were generated using R. Genes affected by copy number alteration were further analyzed for their gene expression pattern in TCGA dataset using “TCGA analysis” module of UALCAN (http://ualcan.path.uab.edu/analysis.html). Genes were queried against “Esophageal carcinoma” database and explored for expression and survival analysis with p-value threshold of 0.05 (26).
Neoantigen Prediction
Single nucleotide somatic variants were used to predict potential neoantigens using tumor-specific neoantigen detector (TSNAD) (27). The tool is based on NetMHCpan (28) which is designed using an artificial neural network approach. It is specifically designed to handle somatic SNVs identified using WGS or WES analysis for prediction of potential neoantigens that can bind to major histocompatibility complex (MHC) class I molecule. Human leukocyte antigen (HLA) allele subtype is a mandatory input of TSNAD to predict compatible neoantigen peptide. HLA subtypes for each patient were inferred using HLAScan which is specifically designed for whole exome datasets (29). Clinically relevant four-digit HLA allele predicted using HLAScan was used to restrict neoantigen prediction compatible for each patient.
Gene Ontology and Pathway Enrichment Analysis
Gene ontology overrepresentation analysis was carried out using FunRich with default Human database with p-value threshold of 0.05 (30). Pathway enrichment analysis was done using recurrently mutated genes to identify the most affected pathways. Genes affected by non-synonymous SNVs in at least two samples and/or genes with CNV in at least four samples were queried using DAVID (31). Pathway enrichment was carried out using Human backend database with a p-value threshold of 0.05 (Fisher's exact test).
Statistical Analysis
Statistical significance of the differences in mutation load between cohorts in each study was determined using unpaired t-test with assumption of similar standard deviation with 95% confidence level. Statistical significance of transition and transversion frequencies between cohorts was determined using unpaired two-tailed Mann-Whitney test and within cohort was determined using paired two tailed Wilcoxon test. P-values for gene expression differences were obtained from UALCAN platform. Estimation of gene expression difference was performed using t-test module of Comprehensive Perl Archive Network (CPAN) “Statistics::TTest” in UALCAN (26). Differences with p-values ≤ 0.05 were considered statistically significant. Kaplan-Meier curve was generated using the PROGgeneV2 platform by grouping the samples in TCGA-Esophageal Carcinoma dataset based on median gene expression.
Results
Whole Exome Sequence Analysis
We performed whole exome sequence analysis of 28 ESCC tumor samples and germline DNA from adjacent normal tissue to characterize mutation landscape. Approximately 100× target depth with ~99% target coverage was achieved with an average of 90 million reads for all samples (Supplementary Table 2). WES analysis led to the identification of 9,524 SNVs in 6,774 genes. Among 9,524 SNVs, 1,500 were synonymous, 3,944 were non-synonymous, and others were non-exonic variants. Non-synonymous exonic variants include 3,630 missense, 217 nonsense, 84 splice site mutations, 7 stop loss, and 6 start loss SNVs. We observed a median of 296 variants per sample with a mutation burden of 5.81/Mb (2.28 ns-SNVs/Mb) (Supplementary Table 3).
Somatic Mutation Spectrum of Esophageal Squamous Cell Carcinoma
TP53 (71%), TTN (64%), CSMD3 (43%), SYNE1 (28%), LRP1B (25%), PIK3CA (25%), DNAH5 (21%), and NOTCH1 (14%) were recurrently mutated genes in our ESCC cohort (Figure 1). TP53 mutations were identified in 71% of the samples which is in concordance with the reported mutation frequency of ~80% in previous studies (12, 13). NF1 is a well-known tumor suppressor gene and it was mutated in 11% of the samples and ZFHX4 in 21% of the samples. We identified SNVs in the cadherin repeat domains of FAT family genes, i.e., FAT1 and FAT4 in 21% samples, which are functionally characterized as tumor suppressor genes in multiple cancers including ESCC (13, 32–34). In concordance with ESCC genomic profiles from Chinese and Japanese cohorts, we identified several previously reported recurrently mutated genes (Supplementary Table 4). Gene ontology analysis revealed that most of the mutated genes are involved in cell communication, cell growth and maintenance (Supplementary Figure 1).
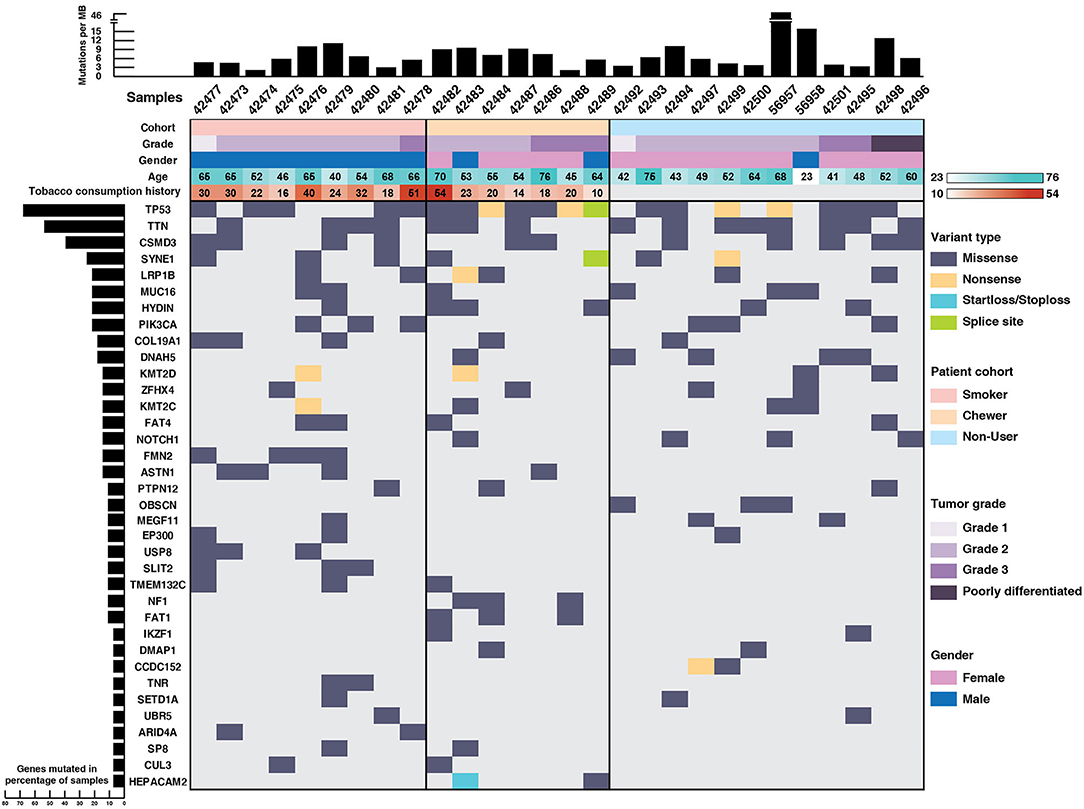
Figure 1. Mutational landscape of esophageal squamous cell carcinoma. Top panel depicts mutation load per Mb, middle panel depicts sample details including tumor grade, gender, age, and tobacco consumption history. Each column represents a sample and each row depicts a gene. Somatic mutations are colored based on mutation type and left panel depicts percentage of samples that harbor mutations.
Notably, genes involved in epigenetic mechanisms, such as SETD1A, CUL3, IKZF1, UBR5, EP300, and ARID4A were recurrently mutated. SETD1A, also known as KMT2F, is a histone methyltransferase which methylates Lys4 of histone H3 and regulates transcriptional activation (35). Other genes from same family, i.e., KMT2D and KMT2C were also recurrently mutated. Gene expression analysis of SETD1A in EBI Expression atlas (36) revealed that SETD1A is downregulated in many cancers including triple-negative breast cancer, colorectal carcinoma and pancreatic adenocarcinoma. Also, its downregulation is significantly associated with poor overall survival in TCGA-Esophageal Carcinoma (ESCA) dataset (p = 0.019) as per the PROGeneV2 platform (Supplementary Figure 2). EP300 and CUL3 are well-known genes commonly mutated in ESCC (14, 37, 38). IKZF1 is known to play a key role in enhancing the susceptibility of solid tumors to immunotherapy (39). Interestingly, we observed SNVs in epigenetic modulator DMAP1 and mitotic kinetics regulator HEPACAM2 that are not well-studied in ESCC. DMAP1 (DNA methyltransferase 1 associated protein 1) forms a transcriptional repressive complex by directly interacting with DNMT1, a key enzyme responsible for epigenetic methylation patterns and transcriptional silencing process (40). Together with DNMT1, it plays an important role in DNA repair process (41–43). Mutations in various genes involved in epigenetic mechanisms suggest that epigenetic signatures could serve as a major hallmark of disease progression in ESCC. Apart from epigenetic modulators, mitotic kinetics regulator or HEPACAM family member 2 (HEPACAM2) was mutated in chewers cohort with a start loss mutation and a missense mutation. It is a cell adhesion molecule which belongs to Immunoglobulin superfamily and variants are observed in proximity to Immunoglobulin-like domain. HEPACAM2 is involved in centrosome maturation during the mitotic cell division. Its expression is significantly downregulated in progressive manner from normal mucosa to adenoma to carcinoma in colorectal cancer and its higher expression is significantly associated with better survival (44).
Mutually Exclusive Mutations in ESCC
Large-scale sequencing studies have shown several mutated genes exist in mutually exclusive manner across different tumors (45). Two major hypothesis associated with mutually exclusive mutated genes in cancers is functional redundancy in downstream pathways or synthetic lethality (46). We observed a mutually exclusive mutation pattern in PIK3CA–NOTCH1, DNAH5–ZFHX4, MUC16–FAT1, and ZFHX4–NOTCH1 gene pairs in ESCC (Figure 2). A study by Song et al. has investigated mutually exclusive mutations of PIK3CA and NOTCH1. Based on clinicopathological parameters, they reported a better response to chemotherapy in patients with PIK3CA mutation compared to those with NOTCH1 mutations. PIK3CA mutations are associated with longer overall survival and progression free survival compared to NOTCH1 mutations (47). Mutations in ZFHX4 and DNAH5 were also associated with poor survival among ESCC patients. Knockdown of ZFHX4 decreased migratory and invasive abilities of ESCC cell lines indicating a tumor-promoting ability in ESCC (48). FAT1 is a significantly mutated gene in ESCC. Knockdown of FAT1 leads to increased proliferation rate suggesting potential tumor suppressor function. (12). Few studies have investigated the role of MUC16 in ESCC, and its overexpression is reported to have prognostic value in ESCC (49). A similar mutually exclusive pattern between these gene pairs is observed in cBioPortal, GDC (Supplementary Figure 3), and published Chinese and Japanese cohorts (Supplementary Figures 4–7). One of the genes in these gene pairs is often a known oncogene or tumor suppressor gene.
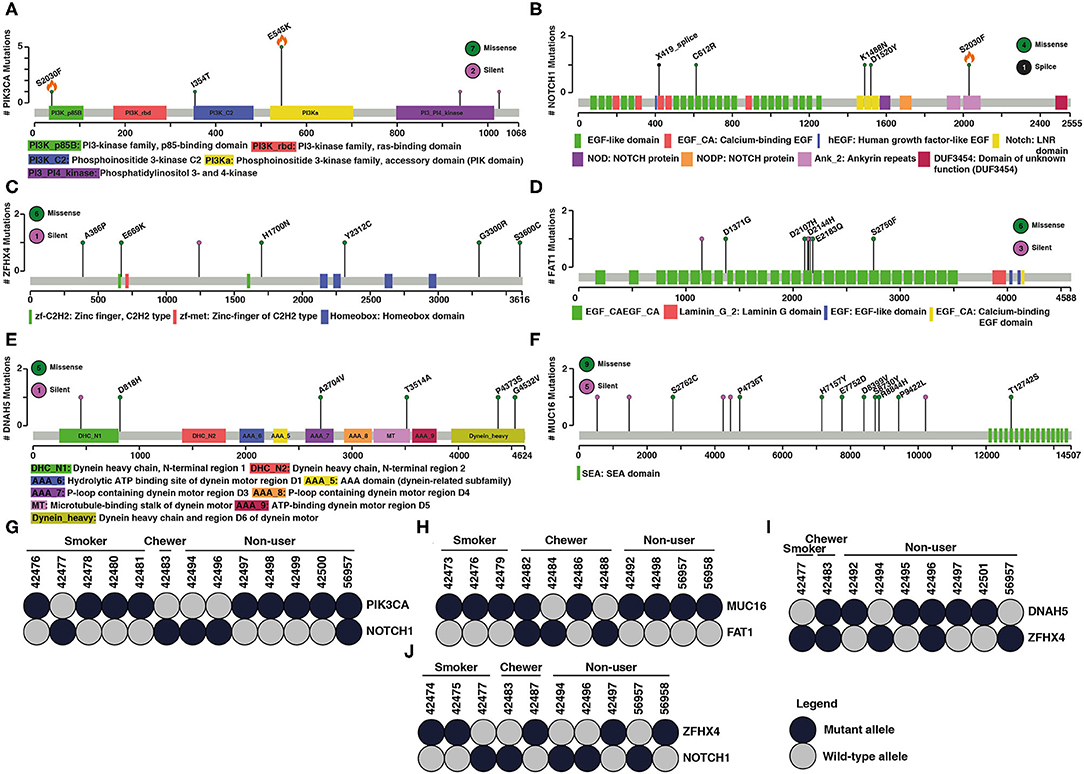
Figure 2. Examples of mutated genes that showed mutual exclusivity. Schematic representation of coding variant distribution on proteins (A) PIK3CA, (B) NOTCH1, (C) ZFHX4, (D) FAT1, (E) DNAH5, and (F) MUC16. Green indicates missense mutation, black indicates splice site and purple indicates silent mutation. Total number of different type of mutations for each gene is given within the circle on right side. X-axis represents length of the protein (amino acid) and y-axis represents number of samples. Orange flame indicates COSMIC hotspot mutations. Instances of gene pairs with mutually exclusive mutation pattern in ESCC (G–J) PIK3CA and NOTCH1, MUC16 and FAT1, DNAH5 and ZFHX4, ZFHX4 and NOTCH1. Column represents samples and row represents genes. Dark shaded circles indicate the mutant allele and the gray circle indicates wild type allele.
Mutation Signatures Associated With Tobacco Usage
In the present study, we sequenced 28 ESCC samples consisting of nine samples with tobacco smoking history, seven samples with tobacco chewing history and 12 samples from patients with no known history of tobacco consumption. The average mutation burden was 5.35 SNVs per Mb in smokers, 7.22 SNVs per Mb in chewers, and 5.8 SNVs per Mb in patients with no history of tobacco usage. We compared the mutation load in our cohort to published ESCC genomic profiling studies from Chinese and Japanese cohorts. We did not observe a significant difference in mutation load between smokers and non-smokers in Chinese as well as Japanese cohorts which was in line with observations from our cohort (12–14, 37, 50, 51). This is unlike lung cancers where lung squamous cell carcinoma and lung adenocarcinoma exhibit a high mutation load in smokers compared to non-smokers (5, 52–54) (Figure 3, Supplementary Table 5). Few studies have investigated the carcinogenic effects of chewing tobacco. Higher mutation load in tobacco chewers is reported in oral squamous cell carcinoma compared to non-chewers (55–57). We speculate that direct and prolonged exposure of chewing tobacco to esophagus and oral cavity may result in higher mutation burden compared to tobacco smoking.
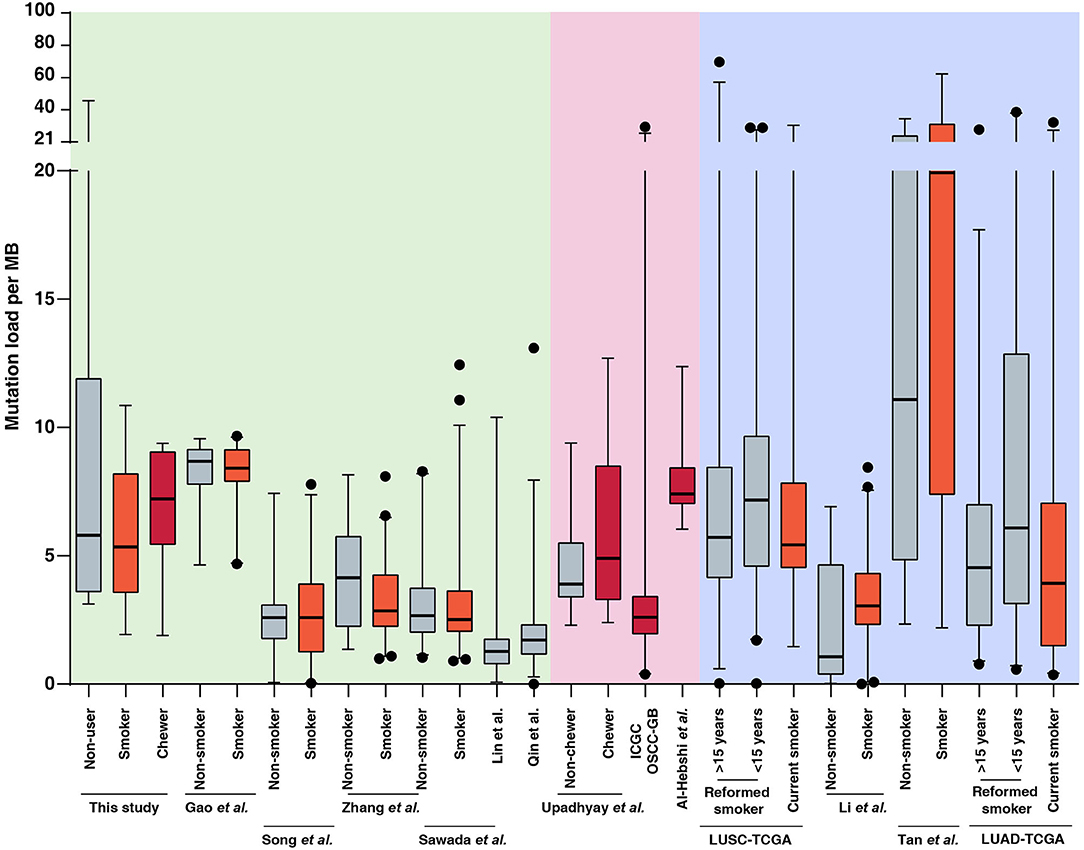
Figure 3. Mutation load observed in tumors from tobacco users and non-users in this study and publicly available datasets of esophageal squamous cell carcinoma (ESCC), oral squamous cell carcinoma (OSCC), lung squamous cell carcinoma (LUSC), and lung adenocarcinoma (LUAD). Black dots represents outliers identified using 97.5 percentile interval. Mutation burden for each sample in ESCC, OSCC, LUSC, and LUAD datasets is provided in Supplementary Table 5 and plotted as per tobacco consumption history.
We further investigated mutational signatures in tumors from patients with and without history of tobacco usage. In concordance with previous ESCC studies, we observe a high frequency of C:G > T:A transitions regardless of tobacco usage history (13, 58) and higher frequency of C > A in chewer cohort (Figure 4A). C > A transversions were observed at a frequency of 0.75 SNVs/Mb in chewer cohort whereas 0.37 SNVs/Mb and 0.36 SNVs/Mb in smoker and non-user cohort, respectively (Figure 4B). Further, deconstruction of mutation signature for each cohort using Mutalisk revealed enrichment of COSMIC mutation signature 4 (38.3%) in chewer cohort whereas 14.9% in smoker and non-user cohort (Figure 4C). Signature 4 is enriched with C > A transversion and is associated with exposure to tobacco derived carcinogens, such as benzo[a]pyrene (58). Chronic treatment of mouse embryonic fibroblasts with tobacco carcinogen benzo[a]pyrene is reported to produce a mutation pattern with high C > A transversions (59). Higher frequency of C > A transversion due to direct exposure to tobacco mutagens is reported in multiple cigarette smoking associated cancers, such as lung and larynx cancer (58). Mutation signature of individual samples from three different cohorts is depicted in Supplementary Figure 8. In congruence with previous reports (58, 59), we propose that higher proportion of C > A transversions in chewers cohort could be attributed to direct exposure of esophageal tissue to tobacco mutagens whereas lack of enrichment of this signature in smoker's cohort may be due to passive exposure to cigarette smoke.
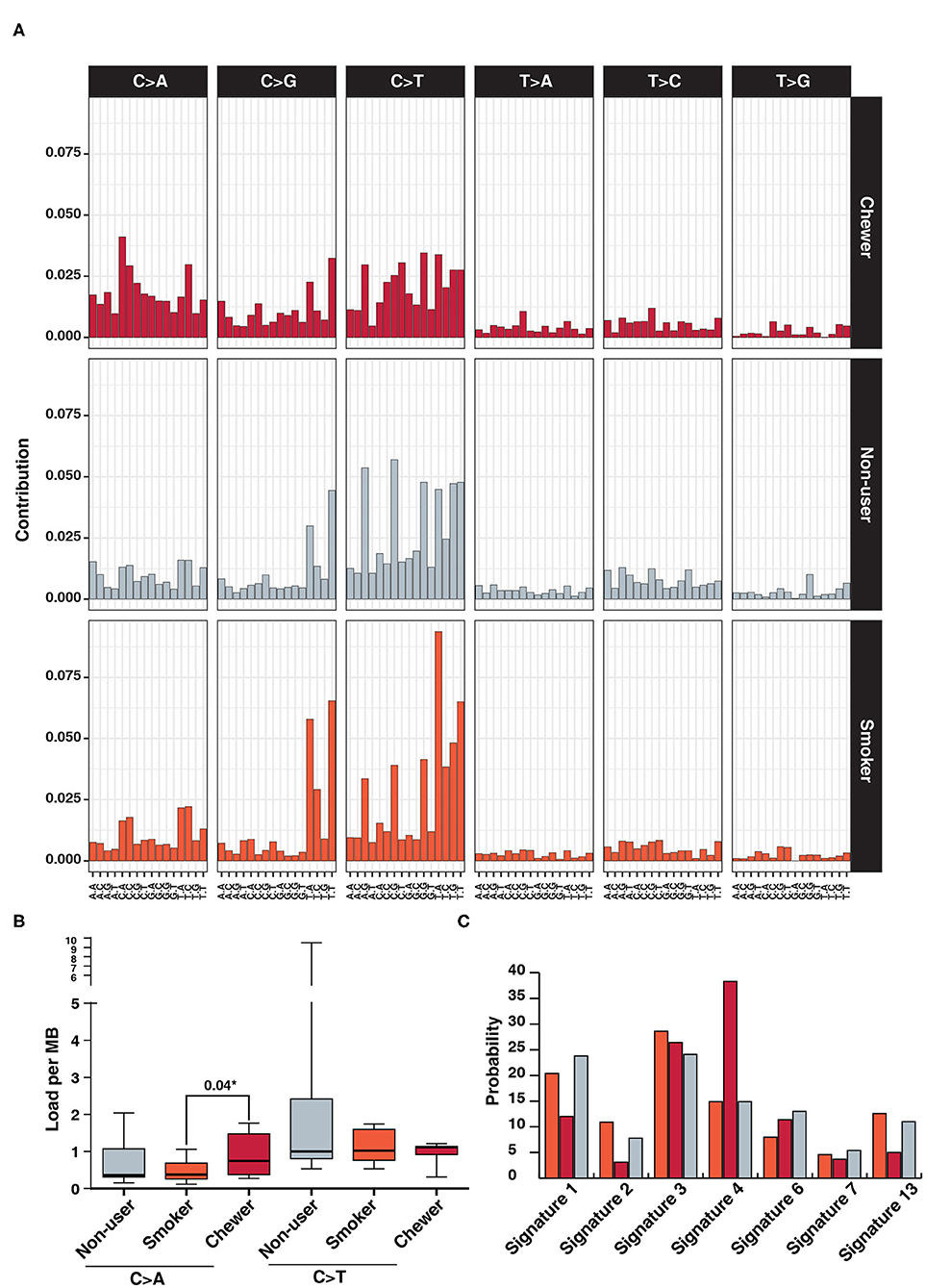
Figure 4. Analysis of mutation signatures enriched in ESCC from smokers, chewers and tobacco non-users. (A) Mutation signatures associated with ESCC from smokers, chewers and tobacco non-users. Height of the bar represents contribution of the base substitution across different trinucleotide contexts. (B) Mutation load of C > A transversions and C > T transitions in smokers, chewers and tobacco non-users. (C) Results of mutation signature decomposition in ESCC samples from chewers, smokers and tobacco non-users. (Red) Chewers, (Gray) Non-users, and (Orange) Smokers.
Potential Neoantigens in ESCC
Cancer neoantigens derived from coding variants can be valuable candidates for developing cancer vaccines. T-cells can recognize neoantigens presented by cancer cells and eliminate them; which makes them valuable candidates for cancer immunotherapy. Efficacy of immune checkpoint inhibitors in treating melanoma and lung cancer is attributed to higher mutation load that potentially contributes to higher neoantigen load in these tumor types (60–62). Somatic non-synonymous single nucleotide variants identified using GATK pipeline were screened for such neoantigenic peptides using HLA-Scan and TSNAD. Elicitation of the immune response depends on the binding affinity of neoantigen with the MHC complex. A high confidence set of 91 neoantigens with wild type peptide binding affinity of ≥500 nM and corresponding mutant peptide binding affinity of ≤100 nM were predicted (63) (Supplementary Table 6). These 91 potential neoantigens were predicted based on 24 coding variants in chewers, 16 coding variants in smokers, and 51 coding variants in non-user cohort. Thirteen genes affected by this include ATF6, DOCK5, IARS, KIAA0922, KIFAP3, MAN2B2, MBTPS1, MXRA8, NSD1, SKI, SLC12A9, TP53, and ZNF277. Expression pattern of 6 out of 13 genes (KIFAP3, MBTPS1, NSD1, SLC12A9, TP53, and ZNF277) in the TCGA-Esophageal Carcinoma (ESCA) dataset indicates overexpression in ESCC samples (n = 95) (26). There are four predicted neoantigens harboring mutation p.Asp153Tyr in sample 42,482 that is compatible with predicted HLA-subtype of the patient, although with weak binding affinity. Similarly, two neoantigens with mutation p.Val320Phe were predicted in sample 42,483. As none of the variants in predicted neoantigens were positionally recurrent in our cohort, identifying neoantigens that can be targeted across multiple patients may prove challenging for immunotherapy. Validation of neoantigens in larger cohorts is warranted to determine their prevalence and potential use in immunotherapy.
Copy Number Alterations in ESCC
Copy number alteration analysis led to the identification of 2,368 affected genes in at least two samples (Supplementary Table 7). Surprisingly, we observed high copy number alterations in tumors from non-tobacco users compared to smokers or chewers. In non-users, 1,540 genes were affected compared to 358 in chewers and 102 in smokers. In concordance with previously published reports, we observed frequent amplifications in 3q24-26, 8q24, 11q13, 5p15, 9q34, 7q22, 16p13, and 20q11 regions in our cohort (Supplementary Figure 9) (51, 64). 11q13.3 region harbors key genes involved in cell growth, such as fibroblast growth factors (FGF3, FGF4, and FGF19) and recently reported oncogenes in ESCC including ANO1 and SHANK2 (65). CNV gains in 3q22-29 region are most frequently observed in ESCC and it contains several key oncogenes, such as PIK3CA, TP63, and SOX2 (Figure 5). Out of 307 genes, 121 (40%) genes in 3q22-29 region are overexpressed in TCGA-Esophageal Carcinoma (ESCA) dataset as well as in ESCC vs. EAC based on the UALCAN database. This includes genes, such as PFN2 and CLSTN2 (26). Higher gene expression of CLSTN2 is associated with poor overall survival in the TCGA-Esophageal Carcinoma dataset (p-value = 0.05) (Supplementary Figure 10). Genes in 3q26.33 (n = 15), 3q26.32 (n = 5) which is also referred to as OncoCassette (66) and 3q27.1 (n = 30) had copy number gain in ≥ 9 samples. Notably, KCNMB2 and KCNMB3 genes in 3q26.32 were amplified in 11 out of 28 samples. Overexpression of these genes is associated with overall poor survival in TCGA-Esophageal Carcinoma dataset (p-value ≤ 0.05). Genomic alterations, such as gain in 8q24 and 11q13 have been associated with poor prognosis in ESCC (67). Co-amplification of SOX2 and TP63 is often observed in squamous cell carcinomas which further validate our findings (68). Overall, our results indicate that amplification of 3q region could be a major driver in the development of ESCC.
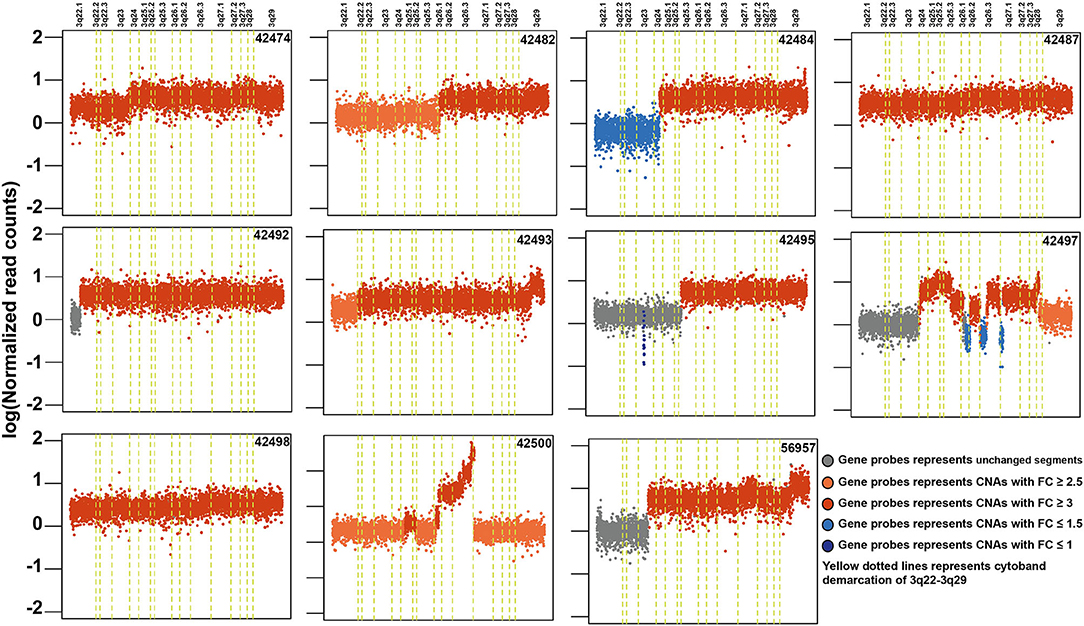
Figure 5. Schematic depicting recurrent genomic amplification in cytoband 3q22-3q29 in esophageal squamous cell carcinoma samples.
Identification of Potentially Druggable Genes
To identify potential druggable targets, we combined genes bearing somatic variants and genes amplified in at least 5 out of 28 samples and screened them against FDA approved anti-neoplastic drugs database DGIdb (24) (Supplementary Table 8). Analysis revealed PIK3CA as a potential therapeutic target. In our cohort, PIK3CA was recurrently mutated (Glu545Lys) in five samples and amplified in 11 samples. Pathway analysis of genes affected by genomic anomalies also revealed enrichment of PIK3CA-AKT pathway which suggests potential therapeutic benefit of targeting PIK3CA in ESCC (Figure 6). PIK3CA gene amplification is associated with advanced stage of tumor and overall poor disease-free survival (69). Other genes which have targeted drugs include NOTCH1, NF1, FBXW7, PRKAA1, MYC, ATR (Supplementary Table 9). Potential druggable targets include USP13, LAMP3, BRD9, TNK2, PAK2, and PRKDC which are overexpressed in ESCC based on RNA-Seq datasets from TCGA (Supplementary Figure 11) (26).
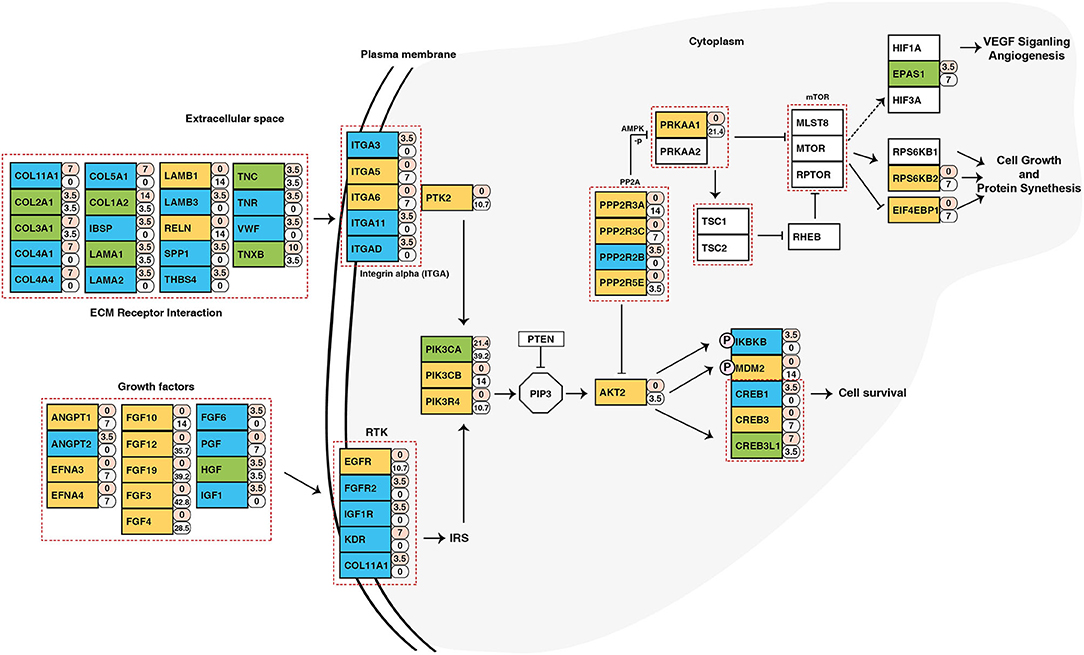
Figure 6. Schematic representation of PIK3CA/AKT pathway depicting genes altered in ESCC. The numbers beside rectangles indicate percentage of samples that carry mutations (pink) and/or copy number variations (white) in respective genes. Green color represents genes with both mutations and CNVs, yellow color represents genes with only CNVs, and blue color are genes with only mutations.
Discussion
Esophageal squamous cell carcinoma is one of the deadliest cancers. Higher incidence of ESCC is observed in Asian countries including China, Japan and India. In Western countries, esophageal adenocarcinoma is more common. The reason for this difference is not well-understood, however, its complex etiology is frequently associated with environmental factors and food habits. Tobacco usage is a known risk factor for ESCC. It is predominantly consumed in the form of cigarette smoking in most countries. In India, it is consumed in different forms, such as gutka and betel nut quid which is a form of smokeless tobacco and cigarettes with/without filter. Epidemiological studies by Dar NA et al. in Kashmir, India and Nasrollahzadeh et al. in Iran have reported association of smokeless tobacco usage with increased risk of ESCC with an odds ratio of 2.88 and 2.91, respectively (70, 71). Multiple Chinese and Japanese studies have investigated genomic alterations in ESCC from patients with a history of cigarette smoking. However, a detailed investigation in an Indian cohort where tobacco is consumed in both smoking and chewing form has not been done.
In this study, we investigated mutational landscape of ESCC from an Indian cohort which included tobacco smokers, chewers and those with no history of tobacco usage. We identified several known and novel genomic alterations in ESCC. The observed non-synonymous mutation load (2.28 mutations per Mb) was comparable to reported mutation load of 1.9–3 mutations per Mb (12–14, 37, 64). We identified several frequently mutated genes, such as TP53, PIK3CA, NOTCH1, FAT1, CUL3, and SLIT2 that have been previously reported in ESCC. Mutation and copy number alterations in PIK3CA are observed at a frequency of 4–45% in published studies. In our study, we observed PIK3CA alteration in 53% of the samples (64, 72). We observed mutually exclusive mutation patterns among samples with PIK3CA and NOTCH1 variants as previously reported by Song et al. Knockdown of NOTCH1 is known to enhance proliferation rate but not migration and invasive ability of KYSE150 and KYSE140 cells (47). We speculate that the concomitant presence of PIK3CA and NOTCH1 mutation might result in synthetic lethality. However, functional validation of this hypothesis is warranted. We observed several genes that were recurrently mutated in patients with history of tobacco usage. This includes USP8, FAT1, SLIT2, TMEM132C, NF1, ARID4A, CUL3, and HEPACAM2. FAT1 was mutated in 3 samples from patients with history of chewing tobacco. A previous study has shown that FAT1 knockdown upregulates MAPK/ERK pathway and promotes epithelial-mesenchymal transition, a hallmark of cancer (73). CUL3 was mutated in two samples with a history of tobacco consumption. It is known to have tumor suppressive property in ESCC and not in EAC (74). Knockdown of CUL3, a core member of BTB-CUL3-RBX1 E3 ubiquitin-protein ligase complex increased the proliferative capacity, migration and invasiveness in ESCC cell lines (38). SLIT2 was mutated in 3 smoker samples and its lower expression is known to promote metastasis by activation of srGAP-Cdc42 pathway and is significantly correlated with poor overall survival and disease-free survival in ESCC patients (75). We did not identify mutations in genes AJUBA and ZNF750 in our cohort which are reported to be commonly mutated in Chinese cohorts (50, 76).
We evaluated genomic signatures associated with ESCC based on the history of tobacco consumption. High frequency of C:G > T:A transition was observed in ESCC regardless of tobacco usage history. This transition is a result of oxidative damage to cytosine residues in DNA. Oxidatively modified cytosines are deaminated by cytidine deaminases leading to C > T change (77). We also observed 2-fold enrichment of signature 4 which is a known mutation signature associated with tobacco exposure in chewer cohort compared to smoker and non-user cohort. We did not observe enrichment of smoking associated signature in smokers cohort and non-user cohort as previously reported in the Chinese cohort (50). Overall, our findings suggest that exposure to chewing tobacco may damage DNA and increase the risk of ESCC.
Copy number alteration analysis showed genomic regions affected across different cohorts. Many amplified regions contain key driver genes, such as PIK3CA, SOX2 in 3q26.32-33, MYC in 8q24.21, FGF3/FGF4/FGF19 in 11q13.3, and FGF12 in 3q28. These could be potentially associated with disease progression in ESCC. Combining mutations and copy number alterations, PIK3CA was affected in 53% of samples. PIK3CA hotspot mutations were not observed in chewer cohort (Supplementary Figure 12A). PIK3CA is overexpressed in ESCC compared to adenocarcinoma or normal esophagus (Supplementary Figure 12B) in TCGA-ESCA dataset (78). It is highly expressed in esophageal cancer in Asians compared to Caucasians and African-Americans (Supplementary Figure 12C). Comparing expression levels across TCGA datasets revealed that PIK3CA is relatively highly expressed in squamous cell carcinomas compared to adenocarcinomas across various cancer types (Supplementary Figure 12D). We also predicted potentially druggable targets among genes that were frequently mutated or amplified in ESCC using FDA approved anti-neoplastic drugs database DGIdb. For example, USP13 (Ubiquitin specific peptidase 13) is a deubiquitinase enzyme which plays an important role in autophagy and endoplasmic reticulum-associated degradation. USP13 is co-amplified with PIK3CA in high-grade serous ovarian cancer. Knockdown of USP13 sensitized ovarian xenograft tumors to pan-AKT inhibitor MK-2206 (79). In a separate study, it was shown that inhibition of USP13 also sensitizes the tumor cells to BH3 mimetic inhibitor, ABT-263 (80). Thus, USP13 is a potential therapeutic candidate in ESCC. TNK2, also known as ACK1 is a non-receptor tyrosine kinase and amplification of TNK2 is widely reported in multiple cancers (81–83). Knockdown of TNK2 in xenograft model reduced the aggressiveness of triple negative breast cancer which further demonstrates the significance of developing an effective targeted therapy against TNK2 (84). Our study provides significant insights into molecular alterations in ESCC and reveals potential candidates for therapeutic targeting.
Conclusions
ESCC is one of the prevalent cancers in India. Although genomic alterations associated with ESCC were previously reported in Chinese and Japanese cohorts, no data was available from Indian cohorts. Our study provides the first report of mutational landscape of ESCC in an Indian cohort. Tobacco usage is a well-known risk factor of ESCC. By carrying out exome sequencing of ESCC samples from cigarette smokers, tobacco chewers and non-users, we show that mutation signature 4 is enriched in patients with a history of tobacco chewing. We did not observe distinct signatures associated with ESCC from patients with a history of smoking. Unlike what is reported in lung cancers (85), high copy number alterations were observed in patients with no history of tobacco usage in our cohort. In addition to mutated genes known in ESCC, we identified several novel genes that have not been reported before. Alterations in the PIK3CA pathway were observed in half of the ESCC samples from Indian cohort. This was independent of the tobacco consumption history of the patients. Mutations in PIK3CA and NOTCH1 were mutually exclusive potentially due to synthetic lethality. Inhibition of these pathways could be explored as a targeted therapeutic strategy to treat ESCC. We did not find specific gene targets based on the history of tobacco usage. Due to low sample numbers, we are not sufficiently powered to identify such gene targets. Further studies are warranted with larger sample size to validate these findings. We predicted potential neoantigens and novel drug targets that can be explored in ESCC.
Data Availability Statement
The datasets generated for this study can be found in the Sequence Read Archive hosted by National Center for Biotechnology Information Search database (NCBI) with accession number PRJNA507919.
Ethics Statement
The studies involving human participants were reviewed and approved by Kidwai Memorial Institute of Oncology, Bangalore. The patients/participants provided their written informed consent to participate in this study.
Author Contributions
HG conceived and designed this study. KM and AK were involved in the sample collection. CK and SM were involved in DNA extraction, quality control, and next generation sequencing. KM, KP, MM, RaG, RoG, and AK-G were involved in the genomic data analyses and interpretation. KM and KP prepared the manuscript and manuscript figures. AChau, PK, BN, RK, AK-G, TP, AChat, AP, and HG edited, critically read, and revised the manuscript. All authors have read and approved the final manuscript.
Funding
This work was supported by the Wellcome Trust/DBT India Alliance Margdarshi Fellowship (grant number IA/M/15/1/502023) awarded to AP. We thank the Department of Biotechnology (DBT), Government of India for research support to the Institute of Bioinformatics (IOB), Bangalore. IOB was supported by DBT Program Support on Neuroproteomics and infrastructure for proteomic data analysis (BT/01/COE/08/05).
Conflict of Interest
MM, CK, SM, RaG, RoG, AK-G, and AChau were employed by the company Medgenome Labs Pvt. Ltd.
The remaining authors declare that the research was conducted in the absence of any commercial or financial relationships that could be construed as a potential conflict of interest.
Acknowledgments
KM was a recipient of Junior Research Fellowship from University Grants Commission (UGC). KP was the recipient of Senior Research Fellow from Council of Scientific and Industrial Research (CSIR). HG was supported by career development fellowship from NHMRC (APP1148551).
Supplementary Material
The Supplementary Material for this article can be found online at: https://www.frontiersin.org/articles/10.3389/fonc.2020.01457/full#supplementary-material
Supplementary Figure 1. Gene ontology enrichment analysis of frequently mutated genes using FunRich keeping default human as background database.
Supplementary Figure 2. Kaplan–Meier curve for overall survival as a function of SETD1A expression using PROGgeneV2 platform [TCGA-Esophageal Carcinoma (ESCA) dataset].
Supplementary Figure 3. Schematic representation of gene pairs PIK3CA and NOTCH1, MUC16 and FAT1, DNAH5 and ZFHX4, ZFHX4 and NOTCH1 with mutually exclusive genomic alteration pattern derived using publicly available TCGA-Esophageal Carcinoma (ESCA) datasets hosted on cBioPortal and NCI GDC Data platform. Column represents examined samples and row represents genes.
Supplementary Figure 4. Schematic representation of mutual exclusivity of mutant PIK3CA and NOTCH1 in published ESCC studies. (A) Zhang et al. (50), (B) Sawada et al. (14), (C) Gao et al. (37), and (D) Qin et al. (51). Blue circles indicate the presence of mutation in respective tumor sample and gray circle indicates presence of WT allele. Column represents examined samples and row represents genes.
Supplementary Figure 5. Schematic representation of mutual exclusivity of mutant MUC16 and FAT1 in published ESCC studies. (A) Zhang et al. (50), (B) Sawada et al. (14), (C) Gao et al. (37), and (D) Qin et al. (51). Blue circles indicate the presence of mutation in respective tumor sample and gray circle indicates presence of WT allele. Column represents examined samples and row represents genes.
Supplementary Figure 6. Schematic representation of mutual exclusivity of DNAH5 and ZFHX4 in published ESCC studies. (A) Zhang et al. (50), (B) Sawada et al. (14), (C) Gao et al. (37), and (D) Qin et al. (51). Blue circles indicate the presence of mutation in respective tumor sample and gray circle indicates presence of WT allele. Column represents examined samples and row represents genes.
Supplementary Figure 7. Schematic representation of mutual exclusivity of NOTCH1 and ZFHX4 in published ESCC studies. (A) Zhang et al. (50), (B) Sawada et al. (14), (C) Gao et al. (37), and (D) Qin et al. (51). Blue circles indicate the presence of mutation in respective tumor sample and gray circle indicates presence of WT allele. Column represents examined samples and row represents genes.
Supplementary Figure 8. Mutation signatures deduced by linear decomposition method using Mutalisk in each ESCC sample.
Supplementary Figure 9. Landscape of genomic amplifications and deletions in each esophageal squamous cell carcinoma sample investigated in the study. Green one-point-outlier, Dark gray surroundings: frequent one-point-outlier, Brown >1 level gain, Brown surroundings: 1-level gain, Blue: >1 level loss, Blue surroundings: 1-level loss.
Supplementary Figure 10. (A) Relative expression level of CLSTN2 in normal esophagus, esophageal adenocarcinoma and esophageal squamous cell carcinoma. (B) Kaplan-Meier survival curve as a function of CLSTN2 expression generated based on TCGA-Esophageal Carcinoma (ESCA) dataset using UALCAN platform.
Supplementary Figure 11. Relative expression level of (A) USP13, (B) LAMP3, (C) BRD9, (D) TNK2, (E) PAK2, (F) PRKDC in normal esophagus and ESCC (TCGA-Esophageal Carcinoma dataset) derived from UALCAN platform.
Supplementary Figure 12. PIK3CA is a recurrently altered and overexpressed gene in esophageal squamous cell carcinoma. (A) Schematic representation of ESCC samples with somatic variants or copy number alteration in PIK3CA. (B) PIK3CA expression levels in normal esophageal tissues, esophageal adenocarcinoma and esophageal squamous cell carcinoma based on UALCAN analysis (***p-value ≤ 0.001). (C) PIK3CA expression level in ESCC tumors from Asian, African-American and Caucasian cohorts based on UALCAN analysis (***p-value ≤ 0.001). (D) Pan-cancer expression levels of PIK3CA across different squamous cell carcinomas and adenocarcinomas compared to adjacent normal tissue samples. BLCA, Bladder Urothelial Carcinoma; BRCA, Breast Invasive Carcinoma; CESC, Cervical Squamous Cell Carcinoma; CHOL, Cholangiocarcinoma; ESCA, Esophageal Carcinoma; GBM, Glioblastoma Multiforme; HNSC, Head and Neck Squamous cell Carcinoma; KICH, Kidney Chromophobe; KIRC, Kidney Real Clear Cell Carcinoma; KIRP, Kidney Renal Papillary Cell Carcinoma; LIHC, Liver Hepatocellular Carcinoma; LUAD, Lung Adenocarcinoma; LUSC, Lung Squamous Cell Carcinoma; PAAD, Pancreatic Adenocarcinoma; PRAD, Prostate Adenocarcinoma; PCPG, Pheochromocytoma and Paraganglioma; READ, Rectum Adenocarcinoma; SARC, Sarcoma; SKCM, Skin Cutaneous Melanoma; THCA, Thyroid Carcinoma; THYM, Thymoma; STAD, Stomach Adenocarcinoma; UCEC, Uterine Corpus Endometrial Carcinoma.
Supplementary Table 1. Clinical characteristics of patients with ESCC that were subjected to whole exome sequencing.
Supplementary Table 2. Read statistics and QC details of whole exome sequencing for each sample.
Supplementary Table 3. List of different types of variants identified in ESCC samples.
Supplementary Table 4. List of somatic single nucleotide variants identified using whole exome sequencing of ESCC patients.
Supplementary Table 5. Mutation load in esophageal squamous cell carcinoma (ESCC), oral squamous cell carcinoma (OSCC), lung squamous cell carcinoma (LUSC), and Lung adenocarcinoma (LUAD).
Supplementary Table 6. List of neoantigens predicted using TSNAD.
Supplementary Table 7. (A) List of copy number alterations and affected genes in ESCC patients. (B) List of cytobands and number of genes affected by Copy number alterations (CNA).
Supplementary Table 8. List of genes screened for potential druggability using DGIdb resource.
Supplementary Table 9. List of identified genes with an available FDA-approved anti-neoplastic drug using DGIdb resource.
Abbreviations
BAM, binary alignment map; BWA, Burrows-Wheeler Aligner; CAN, copy number alterations; EAC, esophageal adenocarcinoma; ESCA, esophageal carcinoma; ESCC, esophageal squamous cell carcinoma; FDR, false discovery rate; HLA, human leukocyte antigen; HNSCC, head and neck squamous cell carcinoma (HNSCC); LUAD, lung adenocarcinoma; LUSC, lung squamous cell carcinoma; MEM, maximal exact matches; MHC, major histocompatibility complex; SNV, somatic single nucleotide variants; TSNAD, tumor-specific neoantigen detector; WES, whole exome sequence; WGS, whole genome sequence.
References
1. Ferlay J, Soerjomataram I, Dikshit R, Eser S, Mathers C, Rebelo M, et al. Cancer incidence and mortality worldwide: sources, methods and major patterns in GLOBOCAN 2012. Int J Cancer. (2015) 136:E359–386. doi: 10.1002/ijc.29210
2. Fitzmaurice C, Allen C, Barber RM, Barregard L, Bhutta ZA, Brenner H, et al. Global, regional, and national cancer incidence, mortality, years of life lost, years lived with disability, and disability-adjusted life-years for 32 cancer groups, 1990 to 2015: a systematic analysis for the global burden of disease study. JAMA Oncol. (2017) 3:524–48. doi: 10.1001/jamaoncol.2016.5688
3. Gupta B, Kumar N. Worldwide incidence, mortality and time trends for cancer of the oesophagus. Eur J Cancer Prev. (2017) 26:107–18. doi: 10.1097/CEJ.0000000000000249
4. Malhotra GK, Yanala U, Ravipati A, Follet M, Vijayakumar M, Are C. Global trends in esophageal cancer. J Surg Oncol. (2017) 115:564–79. doi: 10.1002/jso.24592
5. Li C, Gao Z, Li F, Li X, Sun Y, Wang M, et al. Whole exome sequencing identifies frequent somatic mutations in cell-cell adhesion genes in chinese patients with lung squamous cell carcinoma. Sci Rep. (2015) 5:14237. doi: 10.1038/srep14237
6. Ohashi S, Miyamoto S, Kikuchi O, Goto T, Amanuma Y, Muto M. Recent advances from basic and clinical studies of esophageal squamous cell carcinoma. Gastroenterology. (2015) 149:1700–15. doi: 10.1053/j.gastro.2015.08.054
7. Dikshit R, Gupta PC, Ramasundarahettige C, Gajalakshmi V, Aleksandrowicz L, Badwe R, et al. Cancer mortality in India: a nationally representative survey. Lancet. (2012) 379:1807–16. doi: 10.1016/S0140-6736(12)60358-4
8. Freedman ND, Abnet CC, Leitzmann MF, Mouw T, Subar AF, Hollenbeck AR, et al. A prospective study of tobacco, alcohol, and the risk of esophageal and gastric cancer subtypes. Am J Epidemiol. (2007) 165:1424–33. doi: 10.1093/aje/kwm051
9. Wu IC, Wu CC, Lu CY, Hsu WH, Wu MC, Lee JY, et al. Substance use (alcohol, areca nut and cigarette) is associated with poor prognosis of esophageal squamous cell carcinoma. PLoS ONE. (2013) 8:e55834. doi: 10.1371/journal.pone.0055834
10. Zheng Y, Cao X, Wen J, Yang H, Luo K, Liu Q, et al. Smoking affects treatment outcome in patients with resected esophageal squamous cell carcinoma who received chemotherapy. PLoS ONE. (2015) 10:e0123246. doi: 10.1371/journal.pone.0123246
11. Yang X, Chen X, Zhuang M, Yuan Z, Nie S, Lu M, et al. Smoking and alcohol drinking in relation to the risk of esophageal squamous cell carcinoma: a population-based case-control study in China. Sci Rep. (2017) 7:17249. doi: 10.1038/s41598-017-17617-2
12. Lin DC, Hao JJ, Nagata Y, Xu L, Shang L, Meng X, et al. Genomic and molecular characterization of esophageal squamous cell carcinoma. Nat Genet. (2014) 46:467–73. doi: 10.1038/ng.2935
13. Song Y, Li L, Ou Y, Gao Z, Li E, Li X, et al. Identification of genomic alterations in oesophageal squamous cell cancer. Nature. (2014) 509:91–5. doi: 10.1038/nature13176
14. Sawada G, Niida A, Uchi R, Hirata H, Shimamura T, Suzuki Y, et al. Genomic landscape of esophageal squamous cell carcinoma in a Japanese population. Gastroenterology. (2016) 150:1171–82. doi: 10.1053/j.gastro.2016.01.035
15. Dai W, Ko JMY, Choi SSA, Yu Z, Ning L, Zheng H, et al. Whole-exome sequencing reveals critical genes underlying metastasis in oesophageal squamous cell carcinoma. J Pathol. (2017) 242:500–10. doi: 10.1002/path.4925
16. Andrews S. (2010). FastQC: A Quality Control Tool for High Throughput Sequence Data. Available online at: https://www.bioinformatics.babraham.ac.uk/projects/fastqc/
17. Li H, Durbin R. Fast and accurate short read alignment with Burrows-Wheeler transform. Bioinformatics. (2009) 25:1754–60. doi: 10.1093/bioinformatics/btp324
18. Saunders CT, Wong WS, Swamy S, Becq J, Murray LJ, Cheetham RK. Strelka: accurate somatic small-variant calling from sequenced tumor-normal sample pairs. Bioinformatics. (2012) 28:1811–7. doi: 10.1093/bioinformatics/bts271
19. Cerami E, Gao J, Dogrusoz U, Gross BE, Sumer SO, Aksoy BA, et al. The cBio cancer genomics portal: an open platform for exploring multidimensional cancer genomics data. Cancer Discov. (2012) 2:401–4. doi: 10.1158/2159-8290.CD-12-0095
20. Gao J, Aksoy BA, Dogrusoz U, Dresdner G, Gross B, Sumer SO, et al. Integrative analysis of complex cancer genomics and clinical profiles using the cBioPortal. Sci Signal. (2013) 6:pl1. doi: 10.1126/scisignal.2004088
21. Gehring JS, Fischer B, Lawrence M, Huber W. SomaticSignatures: inferring mutational signatures from single-nucleotide variants. Bioinformatics. (2015) 31:3673–5. doi: 10.1093/bioinformatics/btv408
22. Lee J, Lee AJ, Lee JK, Park J, Kwon Y, Park S, et al. Mutalisk: a web-based somatic MUTation AnaLyIS toolKit for genomic, transcriptional and epigenomic signatures. Nucleic Acids Res. (2018) 46:W102–8. doi: 10.1093/nar/gky406
23. Boeva V, Popova T, Lienard M, Toffoli S, Kamal M, Le Tourneau C, et al. Multi-factor data normalization enables the detection of copy number aberrations in amplicon sequencing data. Bioinformatics. (2014) 30:3443–50. doi: 10.1093/bioinformatics/btu436
24. Cotto KC, Wagner AH, Feng YY, Kiwala S, Coffman AC, Spies G, et al. DGIdb 3.0: a redesign and expansion of the drug-gene interaction database. Nucleic Acids Res. (2018) 46:D1068–73. doi: 10.1093/nar/gkx1143
25. Thorvaldsdottir H, Robinson JT, Mesirov JP. Integrative genomics viewer (IGV): high-performance genomics data visualization and exploration. Brief Bioinform. (2013) 14:178–92. doi: 10.1093/bib/bbs017
26. Chandrashekar DS, Bashel B, Balasubramanya SAH, Creighton CJ, Ponce-Rodriguez I, Chakravarthi B, et al. UALCAN: a portal for facilitating tumor subgroup gene expression and survival analyses. Neoplasia. (2017) 19:649–58. doi: 10.1016/j.neo.2017.05.002
27. Zhou Z, Lyu X, Wu J, Yang X, Wu S, Zhou J, et al. TSNAD: an integrated software for cancer somatic mutation and tumour-specific neoantigen detection. R Soc Open Sci. (2017) 4:170050. doi: 10.1098/rsos.170050
28. Hoof I, Peters B, Sidney J, Pedersen LE, Sette A, Lund O, et al. NetMHCpan, a method for MHC class I binding prediction beyond humans. Immunogenetics. (2009) 61:1–13. doi: 10.1007/s00251-008-0341-z
29. Ka S, Lee S, Hong J, Cho Y, Sung J, Kim HN, et al. HLAscan: genotyping of the HLA region using next-generation sequencing data. BMC Bioinformatics. (2017) 18:258. doi: 10.1186/s12859-017-1671-3
30. Pathan M, Keerthikumar S, Ang CS, Gangoda L, Quek CY, Williamson NA, et al. FunRich: An open access standalone functional enrichment and interaction network analysis tool. Proteomics. (2015) 15:2597–601. doi: 10.1002/pmic.201400515
31. Huang da W, Sherman BT, Lempicki RA. Systematic and integrative analysis of large gene lists using DAVID bioinformatics resources. Nat Protoc. (2009) 4:44–57. doi: 10.1038/nprot.2008.211
32. Morris LG, Kaufman AM, Gong Y, Ramaswami D, Walsh LA, Turcan S, et al. Recurrent somatic mutation of FAT1 in multiple human cancers leads to aberrant Wnt activation. Nat Genet. (2013) 45:253–61. doi: 10.1038/ng.2538
33. Cai J, Feng D, Hu L, Chen H, Yang G, Cai Q, et al. FAT4 functions as a tumour suppressor in gastric cancer by modulating Wnt/beta-catenin signalling. Br J Cancer. (2015) 113:1720–9. doi: 10.1038/bjc.2015.367
34. Hou L, Chen M, Zhao X, Li J, Deng S, Hu J, et al. FAT4 functions as a tumor suppressor in triple-negative breast cancer. Tumour Biol. (2016) 37:16337–43. doi: 10.1007/s13277-016-5421-3
35. Rao RC, Dou Y. Hijacked in cancer: the KMT2 (MLL) family of methyltransferases. Nat Rev Cancer. (2015) 15:334–46. doi: 10.1038/nrc3929
36. Papatheodorou I, Moreno P, Manning J, Fuentes AM, George N, Fexova S, et al. Expression Atlas update: from tissues to single cells. Nucleic Acids Res. (2020) 48:D77–83. doi: 10.1093/nar/gkz947
37. Gao YB, Chen ZL, Li JG, Hu XD, Shi XJ, Sun ZM, et al. Genetic landscape of esophageal squamous cell carcinoma. Nat Genet. (2014) 46:1097–102. doi: 10.1038/ng.3076
38. Chang J, Tan W, Ling Z, Xi R, Shao M, Chen M, et al. Genomic analysis of oesophageal squamous-cell carcinoma identifies alcohol drinking-related mutation signature and genomic alterations. Nat Commun. (2017) 8:15290. doi: 10.1038/ncomms15290
39. Chen JC, Perez-Lorenzo R, Saenger YM, Drake CG, Christiano AM. IKZF1 enhances immune infiltrate recruitment in solid tumors and susceptibility to immunotherapy. Cell Syst. (2018) 7:92–103 e104. doi: 10.1016/j.cels.2018.05.020
40. Rountree MR, Bachman KE, Baylin SB. DNMT1 binds HDAC2 and a new co-repressor, DMAP1, to form a complex at replication foci. Nat Genet. (2000) 25:269–77. doi: 10.1038/77023
41. Negishi M, Chiba T, Saraya A, Miyagi S, Iwama A. Dmap1 plays an essential role in the maintenance of genome integrity through the DNA repair process. Genes Cells. (2009) 14:1347–57. doi: 10.1111/j.1365-2443.2009.01352.x
42. Lee GE, Kim JH, Taylor M, Muller MT. DNA methyltransferase 1-associated protein (DMAP1) is a co-repressor that stimulates DNA methylation globally and locally at sites of double strand break repair. J Biol Chem. (2010) 285:37630–40. doi: 10.1074/jbc.M110.148536
43. Penicud K, Behrens A. DMAP1 is an essential regulator of ATM activity and function. Oncogene. (2014) 33:525–31. doi: 10.1038/onc.2012.597
44. Wu Z, Liu Z, Ge W, Shou J, You L, Pan H, et al. Analysis of potential genes and pathways associated with the colorectal normal mucosa-adenoma-carcinoma sequence. Cancer Med. (2018) 7:2555–66. doi: 10.1002/cam4.1484
45. Kandoth C, McLellan MD, Vandin F, Ye K, Niu B, Lu C, et al. Mutational landscape and significance across 12 major cancer types. Nature. (2013) 502:333–9. doi: 10.1038/nature12634
46. Deng Y, Luo S, Deng C, Luo T, Yin W, Zhang H, et al. Identifying mutual exclusivity across cancer genomes: computational approaches to discover genetic interaction and reveal tumor vulnerability. Brief Bioinform. (2019) 20:254–66. doi: 10.1093/bib/bbx109
47. Song B, Cui H, Li Y, Cheng C, Yang B, Wang F, et al. Mutually exclusive mutations in NOTCH1 and PIK3CA associated with clinical prognosis and chemotherapy responses of esophageal squamous cell carcinoma in China. Oncotarget. (2016) 7:3599–613. doi: 10.18632/oncotarget.6120
48. Qing T, Zhu S, Suo C, Zhang L, Zheng Y, Shi L. Somatic mutations in ZFHX4 gene are associated with poor overall survival of Chinese esophageal squamous cell carcinoma patients. Sci Rep. (2017) 7:4951. doi: 10.1038/s41598-017-04221-7
49. Zhao H, Chen W, Wu J, Wang L, Mao W. Clinical significance of preoperative serum tumor markers in esophageal squamous cell carcinoma. J Cancer Res Ther. (2014) 10:C179–85. doi: 10.4103/0973-1482.145863
50. Zhang L, Zhou Y, Cheng C, Cui H, Cheng L, Kong P, et al. Genomic analyses reveal mutational signatures and frequently altered genes in esophageal squamous cell carcinoma. Am J Hum Genet. (2015) 96:597–611. doi: 10.1016/j.ajhg.2015.02.017
51. Qin HD, Liao XY, Chen YB, Huang SY, Xue WQ, Li FF, et al. Genomic characterization of esophageal squamous cell carcinoma reveals critical genes underlying tumorigenesis and poor prognosis. Am J Hum Genet. (2016) 98:709–27. doi: 10.1016/j.ajhg.2016.02.021
52. Cancer Genome Atlas Research Network. Comprehensive genomic characterization of squamous cell lung cancers. Nature. (2012) 489:519–25. doi: 10.1038/nature11404
53. Cancer Genome Atlas Research Network. Comprehensive molecular profiling of lung adenocarcinoma. Nature. (2014) 511:543–50. doi: 10.1038/nature13385
54. Tan Q, Li F, Wang G, Xia W, Li Z, Niu X, et al. Identification of FGF19 as a prognostic marker and potential driver gene of lung squamous cell carcinomas in Chinese smoking patients. Oncotarget. (2016) 7:18394–402. doi: 10.18632/oncotarget.7817
55. India Project Team of the International Cancer Genome Consortium. Mutational landscape of gingivo-buccal oral squamous cell carcinoma reveals new recurrently-mutated genes and molecular subgroups. Nat Commun. (2013) 4:2873. doi: 10.1038/ncomms3873
56. Al-Hebshi NN, Li S, Nasher AT, El-Setouhy M, Alsanosi R, Blancato J, et al. Exome sequencing of oral squamous cell carcinoma in users of Arabian snuff reveals novel candidates for driver genes. Int J Cancer. (2016) 139:363–72. doi: 10.1002/ijc.30068
57. Upadhyay P, Gardi N, Desai S, Chandrani P, Joshi A, Dharavath B, et al. Genomic characterization of tobacco/nut chewing HPV-negative early stage tongue tumors identify MMP10 asa candidate to predict metastases. Oral Oncol. (2017) 73:56–64. doi: 10.1016/j.oraloncology.2017.08.003
58. Alexandrov LB, Ju YS, Haase K, Van Loo P, Martincorena I, Nik-Zainal S, et al. Mutational signatures associated with tobacco smoking in human cancer. Science. (2016) 354:618–22. doi: 10.1126/science.aag0299
59. Nik-Zainal S, Kucab JE, Morganella S, Glodzik D, Alexandrov LB, Arlt VM, et al. The genome as a record of environmental exposure. Mutagenesis. (2015) 30:763–70. doi: 10.1093/mutage/gev073
60. Rizvi NA, Hellmann MD, Snyder A, Kvistborg P, Makarov V, Havel JJ, et al. Cancer immunology. Mutational landscape determines sensitivity to PD-1 blockade in non-small cell lung cancer. Science. (2015) 348:124–8. doi: 10.1126/science.aaa1348
61. Van Allen EM, Miao D, Schilling B, Shukla SA, Blank C, Zimmer L, et al. Genomic correlates of response to CTLA-4 blockade in metastatic melanoma. Science. (2015) 350:207–11. doi: 10.1126/science.aad0095
62. Miller A, Asmann Y, Cattaneo L, Braggio E, Keats J, Auclair D, et al. High somatic mutation and neoantigen burden are correlated with decreased progression-free survival in multiple myeloma. Blood Cancer J. (2017) 7:e612. doi: 10.1038/bcj.2017.94
63. Fritsch EF, Rajasagi M, Ott PA, Brusic V, Hacohen N, Wu CJ. HLA-binding properties of tumor neoepitopes in humans. Cancer Immunol Res. (2014) 2:522–9. doi: 10.1158/2326-6066.CIR-13-0227
64. Cheng C, Cui H, Zhang L, Jia Z, Song B, Wang F, et al. Genomic analyses reveal FAM84B and the NOTCH pathway are associated with the progression of esophageal squamous cell carcinoma. Gigascience. (2016) 5:1. doi: 10.1186/s13742-015-0107-0
65. Yu Y, Cao J, Wu W, Zhu Q, Tang Y, Zhu C, et al. Genome-wide copy number variation analysis identified ANO1 as a novel oncogene and prognostic biomarker in esophageal squamous cell cancer. Carcinogenesis. (2019) 40:1198–208. doi: 10.1093/carcin/bgz077
66. Fields AP, Justilien V, Murray NR. The chromosome 3q26 OncCassette: a multigenic driver of human cancer. Adv Biol Regul. (2016) 60:47–63. doi: 10.1016/j.jbior.2015.10.009
67. Carneiro A, Isinger A, Karlsson A, Johansson J, Jonsson G, Bendahl PO, et al. Prognostic impact of array-based genomic profiles in esophageal squamous cell cancer. BMC Cancer. (2008) 8:98. doi: 10.1186/1471-2407-8-98
68. Watanabe H, Ma Q, Peng S, Adelmant G, Swain D, Song W, et al. SOX2 and p63 colocalize at genetic loci in squamous cell carcinomas. J Clin Invest. (2014) 124:1636–45. doi: 10.1172/JCI71545
69. Kim HS, Lee SE, Bae YS, Kim DJ, Lee CG, Hur J, et al. PIK3CA amplification is associated with poor prognosis among patients with curatively resected esophageal squamous cell carcinoma. Oncotarget. (2016) 7:30691–701. doi: 10.18632/oncotarget.8749
70. Nasrollahzadeh D, Kamangar F, Aghcheli K, Sotoudeh M, Islami F, Abnet CC, et al. Opium, tobacco, and alcohol use in relation to oesophageal squamous cell carcinoma in a high-risk area of Iran. Br J Cancer. (2008) 98:1857–63. doi: 10.1038/sj.bjc.6604369
71. Dar NA, Bhat GA, Shah IA, Iqbal B, Makhdoomi MA, Nisar I, et al. Hookah smoking, nass chewing, and oesophageal squamous cell carcinoma in Kashmir, India. Br J Cancer. (2012) 107:1618–23. doi: 10.1038/bjc.2012.449
72. Phillips WA, Russell SE, Ciavarella ML, Choong DY, Montgomery KG, Smith K, et al. Mutation analysis of PIK3CA and PIK3CB in esophageal cancer and Barrett's esophagus. Int J Cancer. (2006) 118:2644–6. doi: 10.1002/ijc.21706
73. Hu X, Zhai Y, Kong P, Cui H, Yan T, Yang J, et al. FAT1 prevents epithelial mesenchymal transition (EMT) via MAPK/ERK signaling pathway in esophageal squamous cell cancer. Cancer Lett. (2017) 397:83–93. doi: 10.1016/j.canlet.2017.03.033
74. Lin DC, Dinh HQ, Xie JJ, Mayakonda A, Silva TC, Jiang YY, et al. Identification of distinct mutational patterns and new driver genes in oesophageal squamous cell carcinomas and adenocarcinomas. Gut. (2018) 67:1769–79. doi: 10.1136/gutjnl-2017-314607
75. Tseng RC, Chang JM, Chen JH, Huang WR, Tang YA, Kuo IY, et al. Deregulation of SLIT2-mediated Cdc42 activity is associated with esophageal cancer metastasis and poor prognosis. J Thorac Oncol. (2015) 10:189–98. doi: 10.1097/JTO.0000000000000369
76. Du P, Huang P, Huang X, Li X, Feng Z, Li F, et al. Comprehensive genomic analysis of oesophageal squamous cell carcinoma reveals clinical relevance. Sci Rep. (2017) 7:15324. doi: 10.1038/s41598-017-14909-5
77. Kreutzer DA, Essigmann JM. Oxidized, deaminated cytosines are a source of C –> T transitions in vivo. Proc Natl Acad Sci USA. (1998) 95:3578–82. doi: 10.1073/pnas.95.7.3578
78. Cancer Genome Atlas Research Network, Analysis Working Group, Asan University, BCC Agency, Brigham and Women's Hospital, Broad Institute, et al. Integrated genomic characterization of oesophageal carcinoma. Nature. (2017) 541:169–75. doi: 10.1038/nature20805
79. Han C, Yang L, Choi HH, Baddour J, Achreja A, Liu Y, et al. Amplification of USP13 drives ovarian cancer metabolism. Nat Commun. (2016) 7:13525. doi: 10.1038/ncomms13525
80. Zhang S, Zhang M, Jing Y, Yin X, Ma P, Zhang Z, et al. Deubiquitinase USP13 dictates MCL1 stability and sensitivity to BH3 mimetic inhibitors. Nat Commun. (2018) 9:215. doi: 10.1038/s41467-017-02693-9
81. Mahajan K, Coppola D, Chen YA, Zhu W, Lawrence HR, Lawrence NJ, et al. Ack1 tyrosine kinase activation correlates with pancreatic cancer progression. Am J Pathol. (2012) 180:1386–93. doi: 10.1016/j.ajpath.2011.12.028
82. Lei X, Li YF, Chen GD, Ou DP, Qiu XX, Zuo CH, et al. Ack1 overexpression promotes metastasis and indicates poor prognosis of hepatocellular carcinoma. Oncotarget. (2015) 6:40622–41. doi: 10.18632/oncotarget.5872
83. Xu SH, Huang JZ, Xu ML, Yu G, Yin XF, Chen D, et al. ACK1 promotes gastric cancer epithelial-mesenchymal transition and metastasis through AKT-POU2F1-ECD signalling. J Pathol. (2015) 236:175–85. doi: 10.1002/path.4515
84. Wu X, Zahari MS, Renuse S, Kelkar DS, Barbhuiya MA, Rojas PL, et al. The non-receptor tyrosine kinase TNK2/ACK1 is a novel therapeutic target in triple negative breast cancer. Oncotarget. (2017) 8:2971–83. doi: 10.18632/oncotarget.13579
Keywords: tobacco, mutation signatures, squamous cell carcinoma, esophageal cancer, whole exome sequencing
Citation: Mangalaparthi KK, Patel K, Khan AA, Manoharan M, Karunakaran C, Murugan S, Gupta R, Gupta R, Khanna-Gupta A, Chaudhuri A, Kumar P, Nair B, Kumar RV, Prasad TSK, Chatterjee A, Pandey A and Gowda H (2020) Mutational Landscape of Esophageal Squamous Cell Carcinoma in an Indian Cohort. Front. Oncol. 10:1457. doi: 10.3389/fonc.2020.01457
Received: 04 April 2020; Accepted: 09 July 2020;
Published: 20 August 2020.
Edited by:
Xiao Zhu, Guangdong Medical University, ChinaReviewed by:
Qingyu Wang, Agilent Technologies, United StatesM. Iqbal Parker, University of Cape Town, South Africa
Copyright © 2020 Mangalaparthi, Patel, Khan, Manoharan, Karunakaran, Murugan, Gupta, Gupta, Khanna-Gupta, Chaudhuri, Kumar, Nair, Kumar, Prasad, Chatterjee, Pandey and Gowda. This is an open-access article distributed under the terms of the Creative Commons Attribution License (CC BY). The use, distribution or reproduction in other forums is permitted, provided the original author(s) and the copyright owner(s) are credited and that the original publication in this journal is cited, in accordance with accepted academic practice. No use, distribution or reproduction is permitted which does not comply with these terms.
*Correspondence: Harsha Gowda, aGFyc2hhLmdvd2RhJiN4MDAwNDA7cWltcmJlcmdob2Zlci5lZHUuYXU=
†These authors have contributed equally to this work