- 1Department of Urology, The First Hospital of Jilin University, Changchun, China
- 2Key Laboratory of Pathobiology, Ministry of Education, Jilin University, Changchun, China
The tumor microenvironment (TME) is a complex system that plays an important role in tumor development and progression, but the current knowledge about its effect on bladder cancer (BC) is scarce. In this study, we performed a comprehensive analysis of the relationship between the TME and gene expression profiles to identify prognostic biomarkers for BC. The ESTIMATE algorithm was used to calculate immune and stromal scores of BC patients who were obtained from the Gene Expression Omnibus database. We found that the immune and stromal scores were associated with clinical characteristics and the prognosis of BC patients. Based on these scores, 104 immune-related differentially expressed genes were identified. Further, functional enrichment analysis revealed that these genes were mainly involved in the immune-related biological processes and signaling pathways. Three prognostic genes were then identified and used to establish a risk prediction model using Cox regression analyses. Kaplan–Meier survival analysis showed that the expression levels of COL1A1, COMP, and SERPINE2 significantly correlated with cancer-specific survival and overall survival of BC patients. Additionally, we validated the prognostic values of these genes using two independent cohorts from The Cancer Genome Atlas and Gene Expression Omnibus databases. Finally, the relationships between the three prognostic genes and several immune cells were evaluated using Tumor Immune Estimation Resource, indicating that the expression levels of COL1A1, COMP, and SERPINE2 correlated positively with the tumor infiltration levels of CD4+ T cells and macrophages. In conclusion, the current study comprehensively analyzed the TME and presented immune-related prognostic genes for BC, providing new insights into immunotherapeutic strategies for BC patients.
Introduction
Bladder cancer (BC) is the 10th most common malignancy worldwide with 549,000 new cases and 200,000 deaths in 2018 (1). It is a heterogeneous disease, which is divisible into non-muscle-invasive BC (NMIBC) and muscle-invasive BC (MIBC) based on the invasion of lamina propria (2). At initial diagnosis, 70–75% of patients are diagnosed as NMIBC while the remaining 25–30% suffer from MIBC (3). Unfortunately, it is estimated that 60–70% of NMIBCs will relapse and 10–30% of these cases will progress to an advanced crippling morphology despite seasonable intensive therapy (4). When the lesions are confined to the mucosal or sub-mucosal connective tissues, BC patients have a relatively favorable prognosis with a 5-year cancer-specific survival (CSS) rate ranging from 88.5 to 99.1% (5). However, once the tumor progresses to MIBC, the 5-year CSS rate decreases to 40% (6). The lack of understanding about the molecular mechanism behind BC development leads to the deficiency of effective therapy for BC, especially MIBC. Thus, it is still important to identify novel biomarkers for the early diagnosis and develop effective therapeutic strategies for BC patients.
Tumor progression is a complex process that depends on the heterogeneous components of the tumor microenvironment (TME), which includes stromal, endothelial, and tumor-infiltrating immune cells (7). Recently, the TME has increasingly become a compelling topic due to its significant impact on tumor progression, therapeutic resistance, and clinical outcomes. Tumor-infiltrating stromal and immune cells, which are two major components of the TME, have been deemed to be of potential value in the diagnosis and prognosis of cancer (8, 9). For example, infiltration of macrophages in BC was associated with T cell tolerance and could affect the outcome of patients with MIBC (10, 11). In addition, the CC-chemokine receptor (CCR8) has been identifed as an important marker expressed on intratumoral regulatory T cells (Tregs). Wang et al. (12) confirmed that the heavy CCR8+Tregs accumulation in MIBC was associated with the immune tolerance and contributed to chemotherapy resistance and poor prognosis of MIBC. Therefore, it is necessary to study the diverse components of the microenvironment and their complex communications for identifying novel therapeutic targets.
ESTIMATE algorithm is a newly developed method, which is used to predict the infiltration levels of non-tumor cells by analyzing their specific gene expression signature (13). Using this algorithm, stromal score and immune score can be generated based on the single sample Gene Set Enrichment Analysis. Previous studies have applied this algorithm to calculate the immune scores and stromal scores of BC samples to identify the TME-related genes with poor prognosis (14, 15). Besides, the ESTIMATE algorithm has been also applied to clear cell renal cell carcinoma (16), breast cancer (17), and lung cancer (18), confirming its feasibility and effectiveness.
In this study, we calculated immune and stromal scores of BC samples from the Gene Expression Omnibus (GEO) database using the ESTIMATE algorithm and correlated these scores to clinical characteristics and prognosis of BC patients. Based on these scores, the immune-related differentially expressed genes (DEGs) were identified. Then we applied Cox regression analyses to identify immune-related prognostic genes and establish prognostic gene signature. To further investigate the molecular and infiltrating immune cells in the TME, we evaluated the associations between the prognostic genes and several immune cells based on Tumor Immune Estimation Resource (TIMER). Moreover, we verified the availability and reliability of the prognostic genes using two independent cohorts from The Cancer Genome Atlas (TCGA) and GEO databases.
Materials and Methods
Patients and Dataset
The gene expression series matrix and clinical characteristics of BC patients were downloaded from the GEO dataset GSE13507 (https://www.ncbi.nlm.nih.gov/geo/). One hundred and sixty-five eligible patients with complete clinical information were included in this study. In terms of the gene expression profile, we downloaded the unprocessed raw data for calculating the immune and stromal scores. Both gene expression data and corresponding clinical characteristics were publicly available. Therefore, there was no requirement for ethics committee approval.
Calculation of Immune and Stromal Scores
The ESTIMATE algorithm was applied to evaluate the infiltrating levels of the immune and stromal cells in the BC tissues. Based on the gene expression data, the immune and stromal scores were calculated using the “estimate” R package. Furthermore, the best cutoff values generated using X-tile plots (19) were used to divide the samples into high and low score groups. The clinical characteristics between the high and low score groups were compared using a chi-square test. The survival curves were drawn using GraphPad Prism 8 (GraphPad Software Inc., United States) and the log-rank test was employed to test the statistic difference with the significance level p < 0.05.
Identification of DEGs and Functional Enrichment Analyses
The “limma” R package was applied to analyze the matrix data of gene expression levels for identifying DEGs between the high and low score groups. Log2 (fold change) >1 or < −1 and p < 0.05 were set as the threshold for filtering DEGs. Moreover, an immune-related gene list was obtained from the ImmPort platform (https://www.immport.org/) (20), and utilized to identify immune-related DEGs by the Venny online tool (Venny 2.1, https://bioinfogp.cnb.csic.es/tools/venny/). The DAVID database was then employed to perform the functional enrichment analyses of Gene Ontology (GO) and Kyoto Encyclopedia of Genes and Genomes (KEGG) pathway. P < 0.05 was considered to be statistical significant.
Establishment of a Risk Prediction Model
Next, we established a risk model and identified the immune-related prognostic genes for predicting the prognosis of patients with BC. First, we assessed the relationships between the expression levels of the selected DEGs and CSS of patients by univariate Cox regression analysis using the “survival” R package. The significant genes with p < 0.05 were screened out for further analysis. Subsequently, using the “glmnet” R package, we performed the Least Absolute Shrinkage and Selector Operation (LASSO) analysis that could reduce the estimation variance while providing an explicable final model (21), and identified the key genes affecting patients' prognosis. Finally, we conducted a multivariate Cox regression analysis to establish a risk prediction model. The risk scores for each patient were generated using the Equation: Risk score = Exp1 * β1 + Exp2 * β2 + ···Expn * βn, where “Exp” represented the expression level of key gene while “β” is the regression coefficient acquired from the multivariate Cox regression analysis. The best cutoff value was generated using X-tile plots and was set as the threshold to divide patients into high- and low-risk groups.
Evaluation of the Risk Prediction Model and Survival Analysis
Further, a receiver operating characteristic (ROC) curve was utilized to evaluate the efficiency of the risk prediction model. The ROC curves with the area under the curve (AUC) values were visualized using the “timeROC” package. Kaplan–Meier curves were drawn to exhibit the associations of risk score and potential prognostic genes with survivals of patients. The log-rank test was employed to test the statistic difference with the significance level p < 0.05.
Validation of Prognostic Value
TCGA is a publicly collaborative project that includes lots of clinical information and gene expression profiles across 33 cancer types (22, 23). To confirm the reliability of the identified prognostic genes from the GEO data, BC data from TCGA were utilized to perform validation with the Kaplan–Meier Plotter (http://kmplot.com/analysis/) and Gene Expression Profiling Interactive Analysis (GEPIA) database (http://GEPIA.cancer-pku.cn/). In addition, BC data from the GEO dataset GSE32548 were also utilized to perform validation using the online survival analysis tool OSblca (http://bioinfo.henu.edu.cn/BLCA/BLCAList.jsp) (24).
Analysis of Immune Cell Infiltration
TIMER is also a publicly available resource that can be used to assess the abundances of six infiltrating immune cells, including B cells, CD4+ T cells, CD8+ T cells, neutrophils, macrophages, and dendritic cells (DCs). We investigated the correlation between the prognostic genes in BC and the abundances of the six immune cells using the online tool TIMER (https://cistrome.shinyapps.io/timer/).
Results
Immune and Stromal Scores Correlate With BC Clinical Characteristics and Prognosis
Gene expression profiles and corresponding clinical information of 165 BC samples were obtained from the dataset GSE13507. After normalizing, 10,180 messenger RNAs were extracted from gene expression profiles. The immune and stromal scores of these samples were calculated by the ESTIMATE algorithm using the “estimate” R package (Supplementary Table 1). Using X-tile plots, the best cutoff value was generated to divide patients into high and low score groups. Table 1 displayed the different distributions of clinical characteristics with respect to scores. Further, the statistical analyses showed that both immune and stromal scores of MIBC, high-grade, and later stage BC were significantly higher compared to NMIBC, low-grade, and earlier stage groups (Figures 1A–F; p < 0.05). But the immune and stromal scores were not associated with BC recurrence (p = 0.13 and p = 0.22, respectively) and progression (p = 0.72 and p = 0.91, respectively) (Supplementary Figures 1A–D).
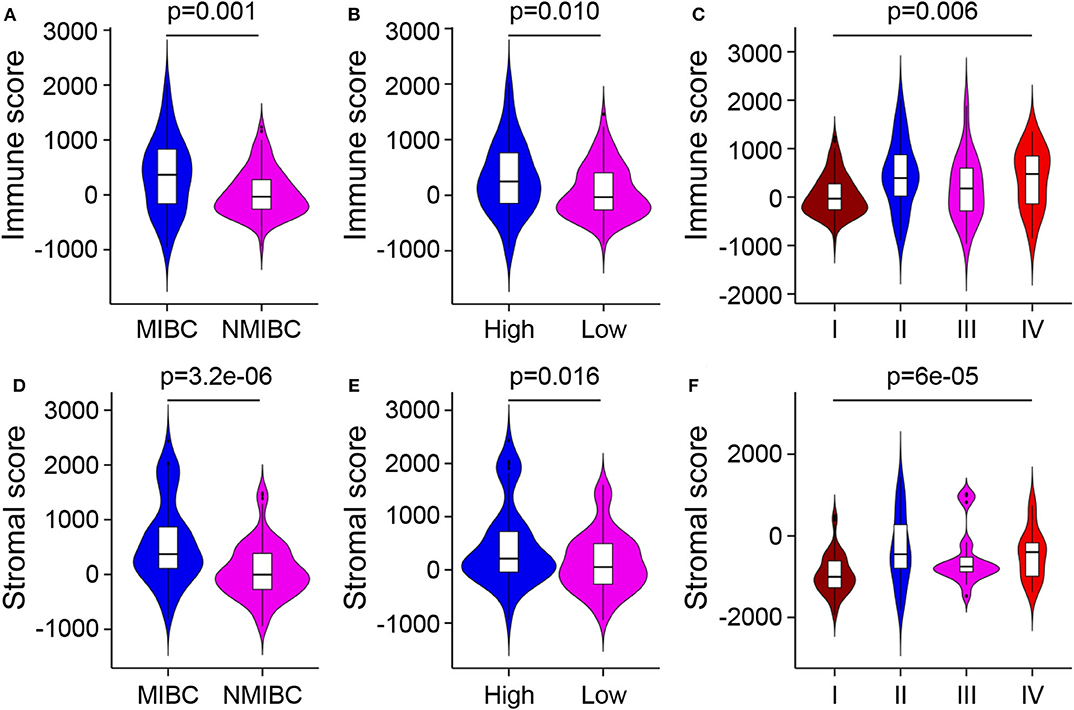
Figure 1. Relationship between immune and stromal scores and BC clinical characteristics. (A–C) Distributions of immune scores among different BC invasions, differentiation grades, and stages. (D–F) Distributions of stromal scores among different BC invasions, differentiation grades, and stages. MIBC, muscle-invasive bladder cancer; NMIBC, non-muscle-invasive bladder cancer.
To explore whether the immune and stromal scores were related to CSS and overall survival (OS), BC patients were divided into two groups based on the best cutoff value generated using X-tile plots. Kaplan–Meier survival curves were performed to analyze the correlation, revealing that the high immune and stromal scores negatively correlated with CSS of BC patients (Figures 2A,B; p = 0.011 and 0.003, respectively). Whereas, no significant correlation was found between the immune/stromal scores and OS of BC patients (Figures 2C,D; p = 0.118 and 0.097, respectively).
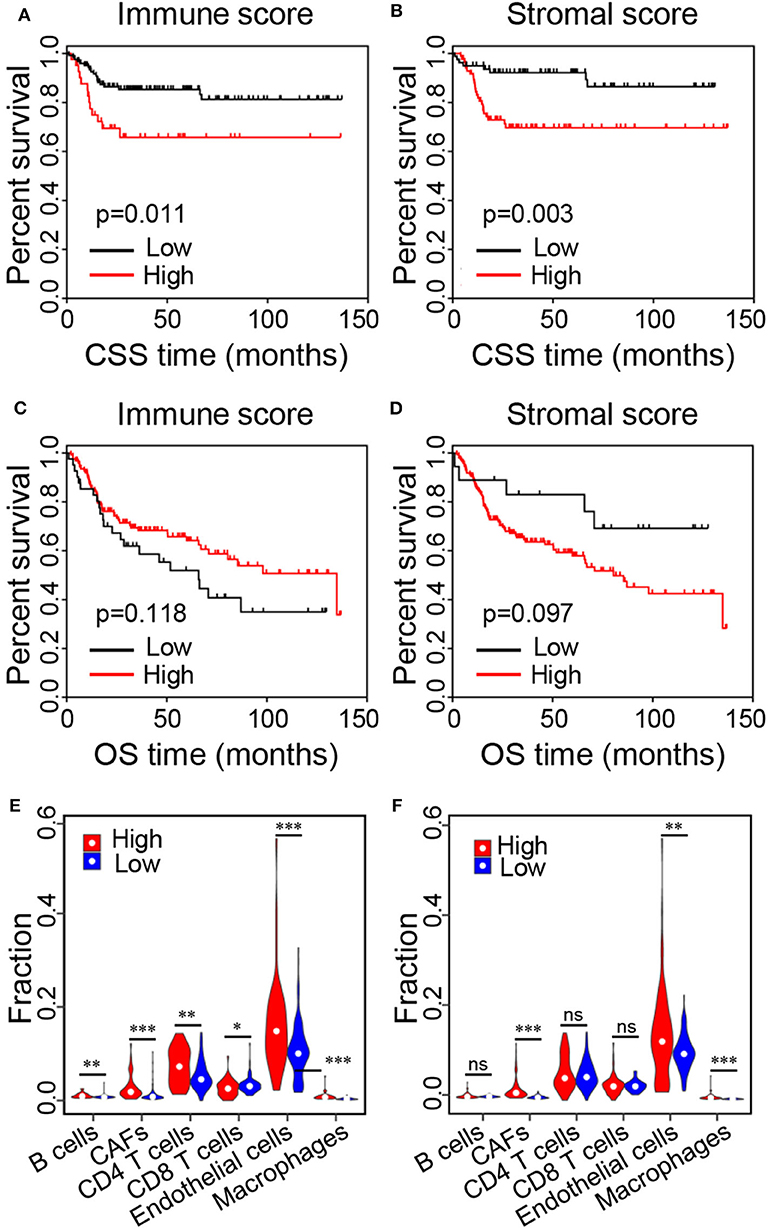
Figure 2. Kaplan–Meier survival curves of BC patients and relative proportions of immune cells in the high and low score groups. (A,B) Kaplan–Meier curves of CSS for patients with low vs. high immune and stromal scores. (C,D) Kaplan–Meier curves of OS for patients with low vs. high immune and stromal scores. (D–F) Relative proportions of immune cells in the low vs. high immune and stromal score groups (*p < 0.05, **p < 0.01, ***p < 0.001, ns, no significance). CSS, cancer-specific survival; OS, overall survival.
To further analyze the reasons for the poor outcomes of the patients with higher scores, we assessed the proportions of immune cells in the TME of the high and low score groups using the online tool Estimate the Proportion of Immune and Cancer cells (EPIC) (25). Specifically, the proportions of B cells (p = 0.002), cancer-associated fibroblasts (CAFs, p = 1.2e-08), CD4+ T cells (p = 0.003), endothelial cells (p = 2.9e-04), and macrophages (p = 2.0e-12) were significantly higher in the high immune score group compared with the low immune score group, while the proportions of CD8+ T cells were significantly lower (Figure 2E; p = 0.024). In addition, the proportions of CAFs (p < 2.0e-16), endothelial cells (p = 0.005), and macrophages (p = 2.1e-13) were significantly higher in the high stromal score group compared with the low stromal score group (Figure 2F). These results indicated that high immune and stromal scores were related to adverse prognosis in BC patients.
Identification of DEGs and Functional Enrichment Analyses
To explore the association of the gene expression levels with the stromal/immune scores, we compared the gene microarray data of all 165 samples obtained in the dataset GSE13507. Using “limma” package, we identified 329 genes with differential expression between the high and low immune score group. Similarly, 421 genes were identified by comparison of the high and low stromal score groups. The volcano plots showed that 304 and 412 genes were up-regulated in the high immune and stromal score groups, respectively (Figure 3A), while 25 and 9 genes were down-regulated (Figure 3B). Subsequently, we identified the overlapping genes among immune score-related DEGs, stromal score-related DEGs, and immune function-related genes obtained from the ImmPort database (Figure 3C). The 104 overlapping genes showed in the Venn diagrams were selected for further analysis.
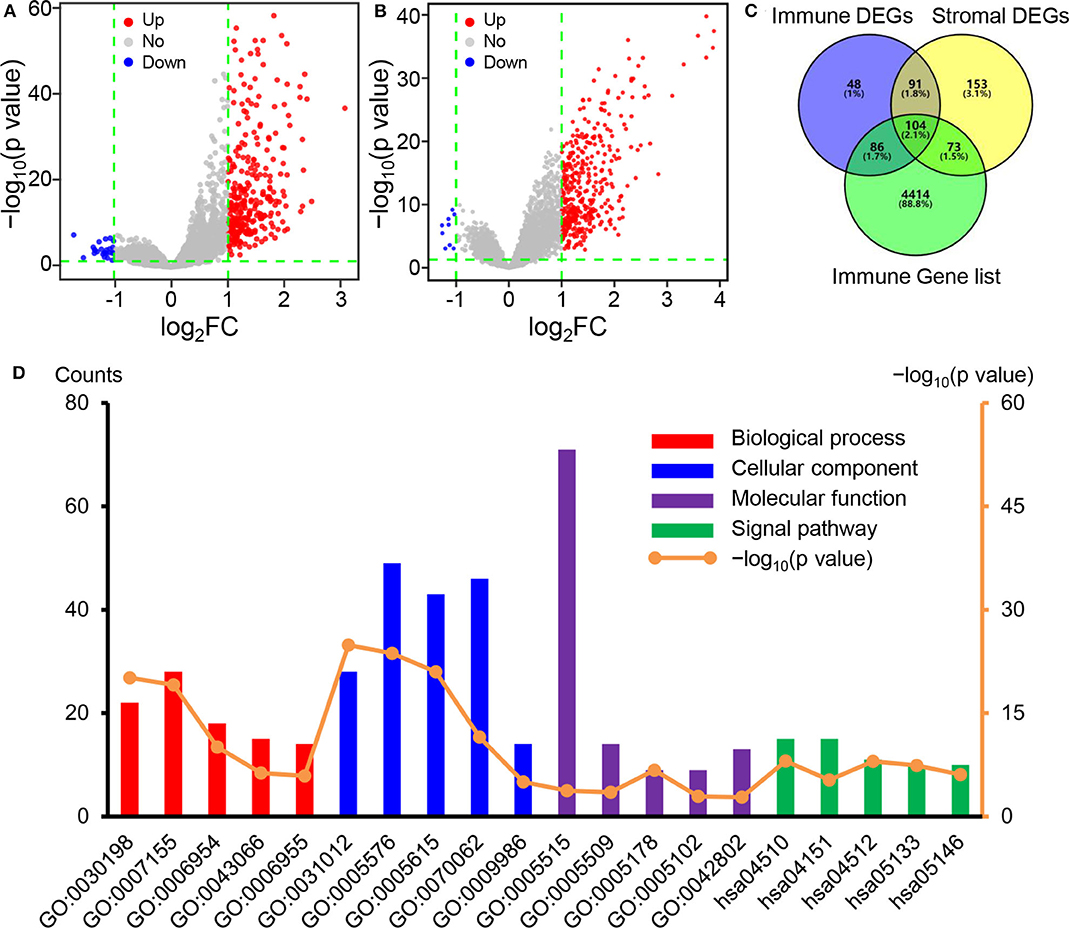
Figure 3. Identification of DEGs and functional enrichment analyses. (A) Volcano plot of DEGs based on immune score in BC samples. (B) Volcano plot of DEGs based on stromal score in BC samples. (C) Venn diagrams showing the overlapping genes among immune score-related DEGs, stromal score-related DEGs, and immune function-related genes. (D) The top five GO and KEGG pathway terms of the overlapping immune-related DEGs. DEGs, differentially expressed genes; BC, bladder cancer; GO, Gene Ontology; KEGG, Kyoto Encyclopedia of Genes and Genomes.
Later, we performed functional enrichment analyses to comprehend the functional properties of the 104 differential immune-related genes. Using the DAVID tool, we identified 173 GO terms and 28 KEGG terms in which the immune-related DEGs enriched significantly (p < 0.05). Figure 3D showed the top five KEGG pathway terms and GO terms, including biological processes, cellular component, and molecular function. The top GO terms, including extracellular matrix organization, inflammatory response, and immune response (Table 2), were strongly associated with the immune microenvironment of tumors. Moreover, the KEGG pathways mainly focused on focal adhesion and PI3K-Akt signaling pathway (Table 2), which were also closely related to the immune microenvironment.
Identification of Genes Associated With Prognosis and Establishment of the Risk Prediction Model
To further explore the prognostic significance of the immune-related DEGs, we performed the Cox regression analyses. The univariate regression analysis revealed that 45 immune-related genes were significantly associated with CSS of BC patients (Supplementary Table 2). Then, the 45 significant genes from the result of the univariate regression were utilized for the multiple LASSO regression (Figure 4A), and three key genes were identified (Figure 4B). Finally, the risk prediction model was built with the three immune-related genes, namely, COL1A1, COMP, and SERPINE2.
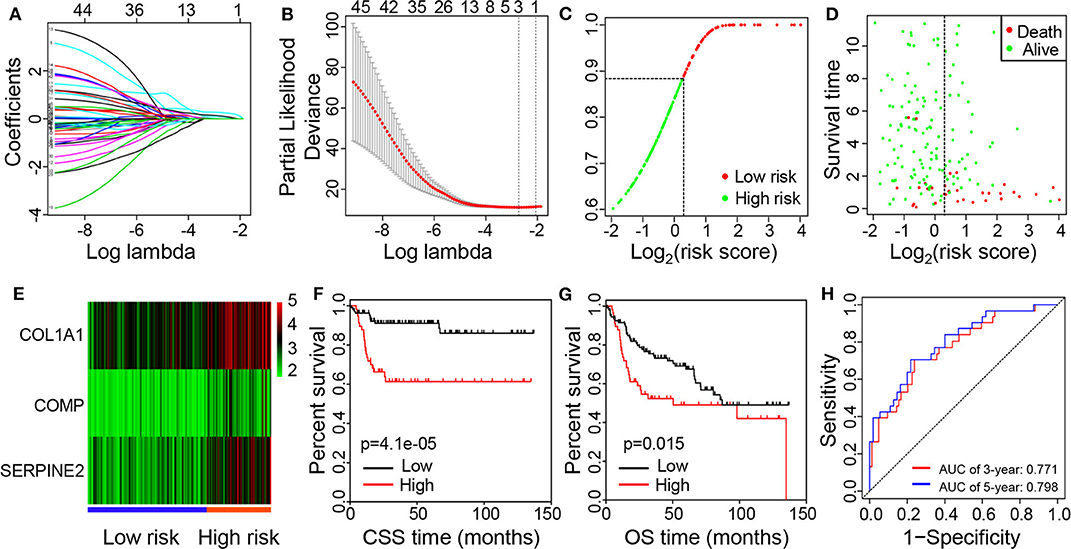
Figure 4. Identification of prognostic genes and establishment of the risk prediction model. (A) LASSO coefficient profiles of the 45 significant genes from the result of the univariate regression. (B) Feature selection for prognostic biomarkers using the LASSO method. (C) Distributions of risk score. (D) The survival time of patients in high- and low-risk groups. (E) Heat map of expression levels of the three prognostic genes in the high- and low-risk groups. (F) Kaplan–Meier curves of CSS for patients with low vs. high risk scores. (G) Kaplan–Meier curves of OS for patients with low vs. high risk scores. (H) ROC curves of the risk model for predicting 3- and 5-year survival rates. LASSO, Least Absolute Shrinkage and Selector Operation; CSS, cancer-specific survival; OS, overall survival; ROC, receiver operating characteristic.
Based on relative coefficients in the multivariate regression analysis, the risk scores were calculated according to the formula: (0.187 * COL1A1 expression level) + (0.293 * COMP expression level) + (0.243 * SERPINE2 expression level). 165 patients were divided into high- and low-risk groups according to the best cutoff value generated using X-tile plots (Figure 4C). The survival times of BC patients were significantly shorter while the number of deaths was higher in the high-risk group compared with the low-risk group (Figure 4D). Moreover, higher expression levels of COL1A1, COMP, and SERPINE2 were observed in the high-risk group compared with the low-risk group (Figure 4E). The Kaplan-Meier survival analysis revealed that a high-risk score was significantly associated with poor CSS (Figure 4F; p = 4.1e-05) and OS (Figure 4G; p = 0.015). As presented graphically in Figure 4H, according to the ROC curve, the AUC for the risk model in predicting 3- and 5-year survival rates were 0.771 and 0.798, respectively.
Survival Analysis and Validation of the Prognostic Value
To further assess the potential prognostic value, we conducted survival analysis concerning the three immune-related genes and patients' prognosis. The results showed that the high expression levels of COL1A1, COMP, and SERPINE2 were associated with poor CSS (Figures 5A–C; p < 0.001). Similarly, the high expression levels of COL1A1 (p = 0.034), COMP (p = 0.011), and SERPINE2 (p = 6.31e-05) negatively correlated with OS (Figures 5D–F).
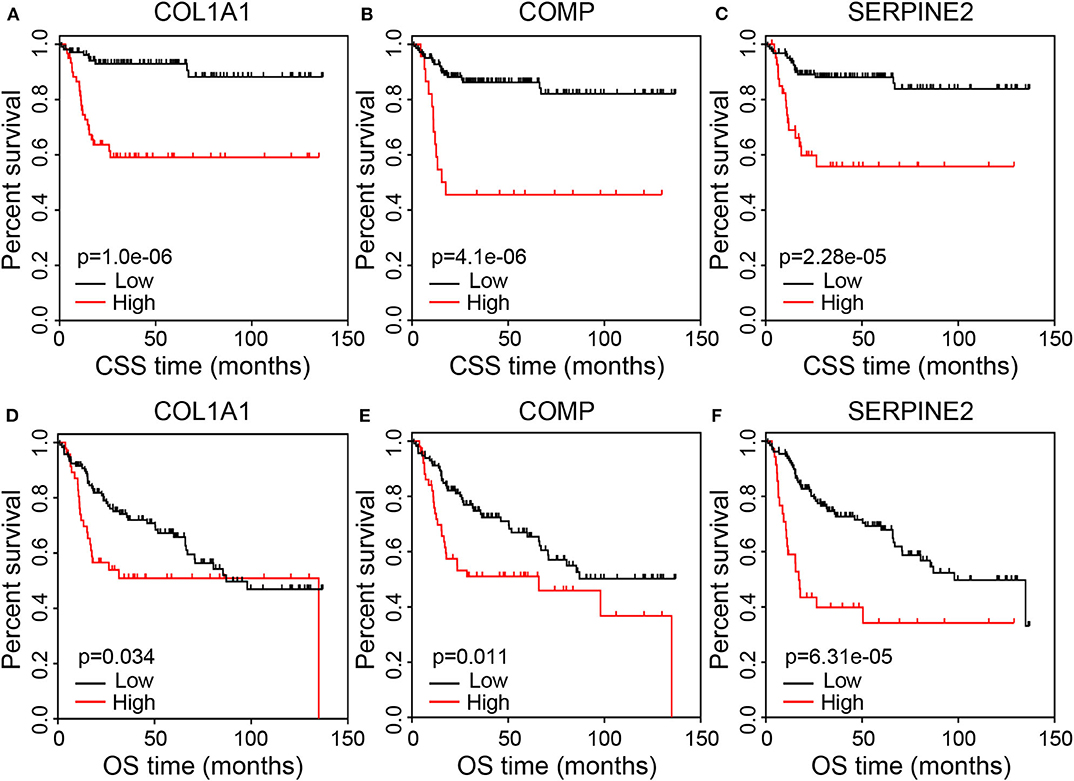
Figure 5. Correlation of the prognostic genes with survival of BC patients. (A–C) Kaplan–Meier curves of CSS for patients grouped by expression levels of COL1A1, COMP, and SERPINE2. (D–F) Kaplan–Meier curves of OS for patients grouped by expression levels of COL1A1, COMP, and SERPINE2. BC, bladder cancer; CSS, cancer-specific survival; OS, overall survival.
To verify whether the prognostic value of the three immune-related genes identified by GEO analyses was reliable and available in other cases of BC, we selected two independent cohorts of BC cases from the TCGA and GEO databases. Kaplan–Meier curves generated using the Kaplan-Meier Plotter database revealed that BC patients in the high COL1A1 (p = 0.003) and COMP (p = 0.003) expression groups suffered a significantly shorter OS than those in the low expression groups (Supplementary Figures 2A,B). BC patients in the high SERPINE2 expression group had shorter OS compared to the low expression group, although this did not reach a statistical significance (Supplementary Figure 2C; p = 0.066). The median OS was 28.8 months in the high expression group and 88.03 months in the low expression group. Furthermore, Kaplan–Meier curves generated using the GEPIA database revealed that the high expression levels of COMP and SERPINE2 were significantly associated with poor prognosis for OS (p = 0.005 and 8.1e-05, respectively; Supplementary Figures 2E,F). But there was no obvious relationship between the COL1A1 expression level and OS (Supplementary Figure 2D; p = 0.11). Finally, we used the OSblca tool to verify the prognostic value of the three genes. As shown in Supplementary Figures 3A–C, the high expression levels of COL1A1 (p = 6e-04), COMP (p = 0.026), and SERPINE2 (p = 0.013) negatively correlated with OS. These data suggested that COL1A1, COMP, and SERPINE2 could be valuable prognostic factors in BC.
Correlations of the Prognostic Genes With Infiltrating Immune Cells
To investigate the associations between the prognostic genes and infiltrating immune cells in BC, the abundances of six immune cells were analyzed using TIMER. As presented graphically in Figure 6, the expression of COL1A1 have a positive correlation with the infiltrating levels of CD8+ T cells (p = 1.27e-03), CD4+ T cells (p = 6.67e-04), macrophages (p = 7.41e-22), neutrophils (p = 8.76e-05), and dendritic cells (p = 5.89e-06), while having a negative correlation with that of B cells (p = 1.99e-03). COMP was also positively related with infiltrating levels of CD4+ T cells (p = 3.64e-03) and macrophages (p = 2.72e-21; Figure 6). Moreover, the expression of SERPINE2 was positively related with the infiltrating levels of CD8+ T cells (p = 1.86e-09), CD4+ T cells (p = 2.25e-05), macrophages (p = 8.64e-09), neutrophils (p = 2.12e-08), and dendritic cells (p = 2.01e-08; Figure 6). These data suggested that COL1A1, COMP, and SERPINE2 might play important roles in the activation and recruitment of immune cells in BC.
Discussion
TME is a complex system that contains fibroblasts, epithelial cells, and infiltrating immune cells, as well as extracellular matrix, which all support neoplastic proliferation, invasion, transformation, and immune tolerance. Tumor cells and infiltrating cells in the TME can produce high levels of immunosuppressive cytokines to inhibit T cell proliferation and effector function while promoting tumor development (26, 27). In addition, tumor-expressing specific proteins can suffice to induce immune cell deactivation and facilitate immune evasion (28). As the most common malignancy of the urinary system, BC has been intensely studied, but the relationship between immune microenvironment and prognosis of BC remains poorly understood. Therefore, it will be highly necessary to understand the underlying mechanism.
In this study, we first calculated immune and stromal scores of BC samples using the ESTIMATE algorithm and analyzed the relationships between these scores and clinical characteristics and prognosis of BC patients. We found that the stromal and immune scores were significantly associated with the clinical pathologic characteristics of BC (e.g., invasiveness, histological grade, and stage) and the patients' prognosis. Both immune and stromal scores of MIBC, high-grade, and later stage BC were significantly higher compared to NMIBC, low-grade, and earlier stage groups, while the immune and stromal scores were not associated with BC recurrence and progression. In addition, higher immune and stromal scores showed close associations with poorer CSS. However, there was no significant correlation between the immune/stromal scores and OS of BC patients. These results are generally consistent with previous studies (15). Our findings indicated that the TME composition could affect the clinical outcomes of BC patients.
In the TME, CAFs are able to express and secret multiple soluble factors, such as growth factors, chemokines, and extracellular matrix-related proteins. Increased secretion of these soluble factors has been proven to restrain anti-tumor immunity by blocking T cells infiltration (29) and be closely connected with poor prognosis in clinical oncology (30). Moreover, increasing research suggests that CAFs are predictors for poor prognosis in various malignancies, including BC (31). Tumor-associated endothelial cells are essential angiogenic regulators for tumor growth and are regulated by various signals from nearby infiltrating immune cells, tumor cells, and stromal cells (32). Previous researchers have found that increased macrophage presence in the TME is correlated with poor prognosis of BC patients (11). In this study, we analyzed the proportions of immune cells in the TME of the high and low score groups to explain why the outcomes of the patients with higher scores were poorer. We found that the relative abundances of CAFs, endothelial cells, and macrophages were significantly increased in both high immune and stromal score groups compared to the low score groups, whereas that of CD8+ T cells was significantly decreased in the high immune score group. These results suggested that the infiltrations of immune cells displaying immunosuppressive functions were increased in BC.
Next, we identified immune-related DEGs between the high and low immune/stromal score groups. A total of 104 overlapping genes were obtained for further analysis. The GO enrichment analysis showed that the immune-related DEGs were strongly involved in extracellular matrix organization, inflammatory response, and immune response that were associated with the immune microenvironment of tumors. Previous research shown that focal adhesion kinase was a crucial signaling molecule in regulating fibrotic and immunosuppressive TME and its expression was negatively associated with the infiltration of T cells (33). PI3K-Akt signaling pathway is a well-known transduction pathway involved in tumorigenesis, proliferation, and immune microenvironment (34). In this study, KEGG pathway analysis showed that enrichments of the immune-related DEGs clustered observably in focal adhesion and PI3K-Akt signaling pathway. These results indicated that the immune-related DEGs were involved in the proliferation and progression of BC by affecting the immune microenvironment.
Since LASSO is recognized as a type of penalized regression, we used LASSO regression analysis to screen covariates. With the combination of using univariate analysis and regression coefficient, we identified three prognostic immune-related genes and subsequently constructed a risk prediction model showing a great capacity for predicting CSS and OS. Patients with BC were stratified to high- and low-risk groups based on the best cutoff value of the risk score. Higher expression levels of COL1A1, COMP, and SERPINE2 were observed in the high-risk group compared with the low-risk group. Unfortunately, ~55.3% (21/38) of high-risk patients died of BC within 3 years of diagnosis, while less than 21% (9/43) died in the low-risk group. Therefore, we suggest that BC patients in the high-risk group should receive more aggressive therapy and more frequent follow-up after diagnosis.
Further survival analysis indicated that the high expression levels of COL1A1, COMP, and SERPINE2 were associated with poor CSS and OS in BC patients. These genes have been proven to be involved in proliferation, migration, development, and progression of various cancers. COL1A1 has been considered as an oncogene and promoted cancer migration and invasion by inducing epithelial-mesenchymal transition (35). Brooks et al. (36) found that the high COL1A1 expression level was associated with poor survival in patients with NMIBC, which was in line with our results. In addiation, increasing evidence confirmed that inhibition of COL1A1 could significantly suppress cancer cells proliferation, clonogenicity, and invasion, indicating that COL1A1 is a putative therapeutic target for cancer (35, 37, 38). COMP, encoding a non-collagenous extracellular matrix protein, is up-regulated in various cancers and involved in cancer cell proliferation, tumorigenesis, epithelial-mesenchymal transition, and stemness features (39–41). It has been suggested as an independent prognostic marker for breast cancer (41). Liu et al. (42) established COMP-knockout cells to detect the effects of COMP on colon cancer cells and found that knockout of COMP could suppress cells proliferation, clonogenicity, and tumor growth, while increasing sensitivity to chemotherapy. These findings indicate that COMP may be also a potential therapeutic target for cancer. Moreover, SERPINE2 contributes to enhanced invasion, metastasis, and stemness of cancer cells by remodeling the tumor matrix, and has been identified as a poor biomarker for endometrial cancer (43). SERPINE2 knockdown has been proven to inhibite tumor cell invasion and metastasis, indicating that SERPINE2 likewise can serve as a potential therapeutic target (44, 45). Nonetheless, these identified genes received less research and attention in BC. Our findings indicated that they could serve as potential prognostic markers and therapeutic target for BC.
As the primary components of the TME, immune cells play an important part in modulating tumor behavior and response to treatment (46, 47). Finally, we used the TIMER database to investigate the associations between the prognostic genes and infiltrating immune cells in BC. Our findings indicated that there is a significantly positive correlation between the expression levels of COL1A1 and SERPINE2 and the infiltrating levels of CD8+ T cells, CD4+ T cells, macrophages, neutrophils, and dendritic cells in BC. COMP was positively associated with infiltrating levels of CD4+ T cells and macrophages. These correlations suggested that COL1A1, COMP, and SERPINE2 might play important roles in the activation and recruitment of immune cells in BC.
Data Availability Statement
The datasets presented in this study can be found in online repositories. The names of the repository/repositories and accession number(s) can be found in the article/Supplementary Material.
Author Contributions
FL and YW developed the idea and designed the study. FL, HT, and ML conducted the study, collected, and analyzed the data. BL, DZ, and HZ interpreted the data. FL wrote this article. FL and ZX accomplished the interpretation of data and participated in the revision. HZ and YW were responsible for editing and submitting this manuscript. All authors approved the final version and submission.
Conflict of Interest
The authors declare that the research was conducted in the absence of any commercial or financial relationships that could be construed as a potential conflict of interest.
Supplementary Material
The Supplementary Material for this article can be found online at: https://www.frontiersin.org/articles/10.3389/fonc.2020.01302/full#supplementary-material
References
1. Bray F, Ferlay J, Soerjomataram I, Siegel RL, Torre LA, Jemal A. Global cancer statistics 2018: GLOBOCAN estimates of incidence and mortality worldwide for 36 cancers in 185 countries. CA Cancer J Clin. (2018) 68:394–424. doi: 10.3322/caac.21492
2. Gao F, Wang X, Chen S, Xu T, Wang X, Shen Y, et al. CIP2A depletion potentiates the chemosensitivity of cisplatin by inducing increased apoptosis in bladder cancer cells. Oncol Rep. (2018) 40:2445–54. doi: 10.3892/or.2018.6641
3. Chen J, Wang L, Tang Y, Gong G, Liu L, Chen M, et al. Maspin enhances cisplatin chemosensitivity in bladder cancer T24 and 5637 cells and correlates with prognosis of muscle-invasive bladder cancer patients receiving cisplatin based neoadjuvant chemotherapy. J Exp Clin Cancer Res. (2016) 35:2. doi: 10.1186/s13046-015-0282-y
4. Knowles MA, Hurst CD. Molecular biology of bladder cancer: new insights into pathogenesis and clinical diversity. Nat Rev Cancer. (2015) 15:25–41. doi: 10.1038/nrc3817
5. Cambier S, Sylvester RJ, Collette L, Gontero P, Brausi MA, van Andel G, et al. EORTC nomograms and risk groups for predicting recurrence, progression, and disease-specific and overall survival in non-muscle-invasive stage Ta-T1 urothelial bladder cancer patients treated with 1-3 years of maintenance bacillus calmette-guerin. Eur Urol. (2016) 69:60–9. doi: 10.1016/j.eururo.2016.01.055
6. van den Bosch S, Alfred Witjes J. Long-term cancer-specific survival in patients with high-risk, non-muscle-invasive bladder cancer and tumour progression: a systematic review. Eur Urol. (2011) 60:493–500. doi: 10.1016/j.eururo.2011.05.045
7. Keren L, Bosse M, Marquez D, Angoshtari R, Jain S, Varma S, et al. A structured tumor-immune microenvironment in triple negative breast cancer revealed by multiplexed ion beam imaging. Cell. (2018) 174:1373–87.e19. doi: 10.1016/j.cell.2018.08.039
8. Chen B, Chen W, Jin J, Wang X, Cao Y, He Y. Data mining of prognostic microenvironment-related genes in clear cell renal cell carcinoma: a study with TCGA database. Dis Markers. (2019) 2019:8901649. doi: 10.1155/2019/8901649
9. Joseph M, Enting D. Immune responses in bladder cancer-role of immune cell populations, prognostic factors and therapeutic implications. Front Oncol. (2019) 9:1270. doi: 10.3389/fonc.2019.01270
10. Sjodahl G, Lovgren K, Lauss M, Chebil G, Patschan O, Gudjonsson S, et al. Infiltration of CD3(+) and CD68(+) cells in bladder cancer is subtype specific and affects the outcome of patients with muscle-invasive tumors. Urol Oncol. (2014) 32:791–7. doi: 10.1016/S1569-9056(14)60778-8
11. Hu B, Wang Z, Zeng H, Qi Y, Chen Y, Wang T, et al. Blockade of DC-SIGN(+) tumor-associated macrophages reactivates antitumor immunity and improves immunotherapy in muscle-invasive bladder cancer. Cancer Res. (2020) 80:1707–19. doi: 10.1158/0008-5472.CAN-19-2254
12. Wang T, Zhou Q, Zeng H, Zhang H, Liu Z, Shao J, et al. CCR8 blockade primes anti-tumor immunity through intratumoral regulatory T cells destabilization in muscle-invasive bladder cancer. Cancer Immunol Immunother. (2020). doi: 10.1007/s00262-020-02583-y. [Epub ahead of print].
13. Yoshihara K, Shahmoradgoli M, Martinez E, Vegesna R, Kim H, Torres-Garcia W, et al. Inferring tumour purity and stromal and immune cell admixture from expression data. Nat Commun. (2013) 4:2612. doi: 10.1038/ncomms3612
14. Li F, Guo H, Wang Y, Liu B, Zhou H. Profiles of tumor-infiltrating immune cells and prognostic genes associated with the microenvironment of bladder cancer. Int Immunopharmacol. (2020) 85:106641. doi: 10.1016/j.intimp.2020.106641
15. Luo Y, Zeng G, Wu S. Identification of microenvironment-related prognostic genes in bladder cancer based on gene expression profile. Front Genet. (2019) 10:1187. doi: 10.3389/fgene.2019.01187
16. Luo J, Xie Y, Zheng Y, Wang C, Qi F, Hu J, et al. Comprehensive insights on pivotal prognostic signature involved in clear cell renal cell carcinoma microenvironment using the ESTIMATE algorithm. Cancer Med. (2020) 9:4310–23. doi: 10.1002/cam4.2983
17. Li B, Geng R, Wu Q, Yang Q, Sun S, Zhu S, et al. Alterations in immune-related genes as potential marker of prognosis in breast cancer. Front Oncol. (2020) 10:333. doi: 10.3389/fonc.2020.00333
18. Qu Y, Cheng B, Shao N, Jia Y, Song Q, Tan B, et al. Prognostic value of immune-related genes in the tumor microenvironment of lung adenocarcinoma and lung squamous cell carcinoma. Aging. (2020) 12:4757–77. doi: 10.18632/aging.102871
19. Camp RL, Dolled-Filhart M, Rimm DL. X-tile: A new bioinformatics tool for biomarker assessment and outcomebased cut-point optimization. Clin Cancer Res. (2004) 10:7252–9. doi: 10.1158/1078-0432.CCR-04-0713
20. Bhattacharya S, Andorf S, Gomes L, Dunn P, Schaefer H, Pontius J, et al. ImmPort: disseminating data to the public for the future of immunology. Immunol Res. (2014) 58:234–9. doi: 10.1007/s12026-014-8516-1
21. Yuan Y, Xu Y, Xu J, Ball RL, Liang H. Predicting the lethal phenotype of the knockout mouse by integrating comprehensive genomic data. Bioinformatics. (2012) 28:1246–52. doi: 10.1093/bioinformatics/bts120
22. Bailey MH, Tokheim C, Porta-Pardo E, Sengupta S, Bertrand D, Weerasinghe A, et al. Comprehensive characterization of cancer driver genes and mutations. Cell. (2018) 173:371–85.e18. doi: 10.1016/j.cell.2018.02.060
23. Tomczak K, Czerwinska P, Wiznerowicz M. The cancer genome atlas (TCGA): an immeasurable source of knowledge. Contemp Oncol (Pozn). (2015) 19:A68–A77. doi: 10.5114/wo.2014.47136
24. Zhang G, Wang Q, Yang M, Yuan Q, Dang Y, Sun X, et al. OSblca: A web server for investigating prognostic biomarkers of bladder cancer patients. Front Oncol. (2019) 9:466. doi: 10.3389/fonc.2019.00466
25. Racle J, de Jonge K, Baumgaertner P, Speiser DE, Gfeller D. Simultaneous enumeration of cancer and immune cell types from bulk tumor gene expression data. Elife. (2017) 6:e26476. doi: 10.7554/eLife.26476
26. Chakraborty S, Panda AK, Bose S, Roy D, Kajal K, Guha D, et al. Transcriptional regulation of FOXP3 requires integrated activation of both promoter and CNS regions in tumor-induced CD8(+) Treg cells. Sci Rep. (2017) 7:1628. doi: 10.1038/s41598-017-01788-z
27. Chen J, Guo XZ, Li HY, Zhao JJ, Xu WD. Dendritic cells engineered to secrete anti-DcR3 antibody augment cytotoxic T lymphocyte response against pancreatic cancer in vitro. World J Gastroenterol. (2017) 23:817–29. doi: 10.3748/wjg.v23.i5.817
28. Medina PJ, Adams VR. PD-1 pathway inhibitors: immuno-oncology agents for restoring antitumor immune responses. Pharmacotherapy. (2016) 36:317–34. doi: 10.1002/phar.1714
29. Mariathasan S, Turley SJ, Nickles D, Castiglioni A, Yuen K, Wang Y, et al. TGFβ attenuates tumour response to PD-L1 blockade by contributing to exclusion of T cells. Nature. (2018) 554:544–8. doi: 10.1038/nature25501
30. Sun Y. Translational horizons in the tumor microenvironment: harnessing breakthroughs and targeting cures. Med Res Rev. (2015) 35:408–36. doi: 10.1002/med.21338
31. Calvete J, Larrinaga G, Errarte P, Martin AM, Dotor A, Esquinas C, et al. The coexpression of fibroblast activation protein (FAP) and basal-type markers (CK 5/6 and CD44) predicts prognosis in high-grade invasive urothelial carcinoma of the bladder. Hum Pathol. (2019) 91:61–8. doi: 10.1016/j.humpath.2019.07.002
32. Chandler KB, Costello CE, Rahimi N. Glycosylation in the tumor microenvironment: implications for tumor angiogenesis and metastasis. Cells. (2019) 8:544. doi: 10.3390/cells8060544
33. Jiang H, Hegde S, Knolhoff BL, Zhu Y, Herndon JM, Meyer MA, et al. Targeting focal adhesion kinase renders pancreatic cancers responsive to checkpoint immunotherapy. Nat Med. (2016) 22:851–60. doi: 10.1038/nm.4123
34. Jiang N, Dai Q, Su X, Fu J, Feng X, Peng J. Role of PI3K/AKT pathway in cancer: the framework of malignant behavior. Mol Biol Rep. (2020) 47:4587–629. doi: 10.1007/s11033-020-05435-1
35. Zhu H, Chen H, Wang J, Zhou L, Liu S. Collagen stiffness promoted non-muscle-invasive bladder cancer progression to muscle-invasive bladder cancer. Onco Targets Ther. (2019) 12:3441–57. doi: 10.2147/OTT.S194568
36. Brooks M, Mo Q, Krasnow R, Ho PL, Lee YC, Xiao J, et al. Positive association of collagen type I with non-muscle invasive bladder cancer progression. Oncotarget. (2016) 7:82609–19. doi: 10.18632/oncotarget.12089
37. Ma HP, Chang HL, Bamodu OA, Yadav VK, Huang TY, Wu ATH, et al. Collagen 1A1 (COL1A1) Is a reliable biomarker and putative therapeutic target for hepatocellular carcinogenesis and metastasis. Cancers (Basel). (2019) 11:786. doi: 10.3390/cancers11060786
38. Zhang Z, Fang C, Wang Y, Zhang J, Yu J, Zhang Y, et al. COL1A1: A potential therapeutic target for colorectal cancer expressing wild-type or mutant KRAS. Int J Oncol. (2018) 53:1869–80. doi: 10.3892/ijo.2018.4536
39. Nfonsam VN, Jecius HC, Janda J, Omesiete PN, Elquza E, Scott AJ, et al. Cartilage oligomeric matrix protein (COMP) promotes cell proliferation in early-onset colon cancer tumorigenesis. Surg Endosc. (2019). doi: 10.1007/s00464-019-07185-z. [Epub ahead of print].
40. Sun L, Wang Y, Wang L, Yao B, Chen T, Li Q, et al. Resolvin D1 prevents epithelial-mesenchymal transition and reduces the stemness features of hepatocellular carcinoma by inhibiting paracrine of cancer-associated fibroblast-derived COMP. J Exp Clin Cancer Res. (2019) 38:170. doi: 10.1186/s13046-019-1163-6
41. Papadakos KS, Darlix A, Jacot W, Blom AM. High levels of cartilage oligomeric matrix protein in the serum of breast cancer patients can serve as an independent prognostic marker. Front Oncol. (2019) 9:1141. doi: 10.3389/fonc.2019.01141
42. Liu TT, Liu XS, Zhang M, Liu XN, Zhu FX, Zhu FM, et al. Cartilage oligomeric matrix protein is a prognostic factor and biomarker of colon cancer and promotes cell proliferation by activating the Akt pathway. J Cancer Res Clin Oncol. (2018) 144:1049–63. doi: 10.1007/s00432-018-2626-4
43. Shen Y, Wang X, Xu J, Lu L. SerpinE2, a poor biomarker of endometrial cancer, promotes the proliferation and mobility of EC cells. Cancer Biomark. (2017) 19:271–8. doi: 10.3233/CBM-160442
44. Zhang J, Luo A, Huang F, Gong T, Liu Z. SERPINE2 promotes esophageal squamous cell carcinoma metastasis by activating BMP4. Cancer Lett. (2020) 469:390–8. doi: 10.1016/j.canlet.2019.11.011
45. Wu QW. Serpine2, a potential novel target for combating melanoma metastasis. Am J Transl Res. (2016) 8:1985–97.
46. Guo L, Cao C, Goswami S, Huang X, Ma L, Guo Y, et al. Tumoral PD-1hiCD8+ T cells are partially exhausted and predict favorable outcome in triple-negative breast cancer. Clin Sci (Lond). (2020) 134:711–26. doi: 10.1042/CS20191261
Keywords: bladder cancer, tumor microenvironment, prognosis, immune score, stromal score, overall survival, cancer-specific survival
Citation: Li F, Teng H, Liu M, Liu B, Zhang D, Xu Z, Wang Y and Zhou H (2020) Prognostic Value of Immune-Related Genes in the Tumor Microenvironment of Bladder Cancer. Front. Oncol. 10:1302. doi: 10.3389/fonc.2020.01302
Received: 06 May 2020; Accepted: 23 June 2020;
Published: 28 July 2020.
Edited by:
Ja Hyeon Ku, Seoul National University, South KoreaReviewed by:
Guru Sonpavde, Dana–Farber Cancer Institute, United StatesXiangqian Guo, Henan University, China
Copyright © 2020 Li, Teng, Liu, Liu, Zhang, Xu, Wang and Zhou. This is an open-access article distributed under the terms of the Creative Commons Attribution License (CC BY). The use, distribution or reproduction in other forums is permitted, provided the original author(s) and the copyright owner(s) are credited and that the original publication in this journal is cited, in accordance with accepted academic practice. No use, distribution or reproduction is permitted which does not comply with these terms.
*Correspondence: Yishu Wang, d2FuZ3lzJiN4MDAwNDA7amx1LmVkdS5jbg==; Honglan Zhou, d2Fsa2VyemhvdWhsJiN4MDAwNDA7MTYzLmNvbQ==