- 1Department of Oncology, The Second Affiliated Hospital of Zunyi Medical University, Zunyi, China
- 2Department of Radiation Oncology, Universitätsklinikum Erlangen, Erlangen, Germany
- 3Comprehensive Cancer Center Erlangen-EMN, Erlangen, Germany
- 4Guangdong Lung Cancer Institute, Guangdong Provincial People's Hospital, Guangdong Academy of Medical Sciences, School of Medicine, South China University of Technology, Guangzhou, China
- 5Department of Orthodontics, Affiliated Stomatological Hospital of Zunyi Medical University, Zunyi, China
- 6Department of Clinical Laboratory, The Sixth Affiliated Hospital of Sun Yat-sen University, Guangzhou, China
Background: Testicular germ cell tumors (TGCTs) are commonly diagnosed tumors in young men. However, a satisfactory approach to predict relapse of stage I TGCTs is still lacking. Therefore, this study aimed to develop a robust risk score model for stage I TGCTs.
Method: RNA-sequence data of stage I TGCTs and normal testis samples were downloaded and analyzed to identify different expression genes. Gene-based prognostic model was constructed in The Cancer Genome Atlas (TCGA) using least absolute shrinkage and selection operator (LASSO) regression analysis and validated in GSE99420 dataset. Potential biological functions of the genes in prognostic model were determined via Gene Set Enrichment Analysis (GSEA) between high-risk and low-risk patients.
Results: A total of 9,391 differentially expressed genes and 84 prognosis-related genes were identified. An eight-gene-based risk score model was constructed to divide patients into high or low risk of relapse. The low-risk patients had a significantly better relapse-free survival (RFS) than high-risk patients in both training and validation cohorts (HR = 0.129, 95% CI = 0.059–0.284, P < 0.001; HR = 0.277, 95% CI = 0.116–0.661, P = 0.004, respectively). The area under the receiver operating characteristic curve (AUC) values at 5 years was 0.805 and 0.724 in the training and validation cohorts, respectively. Functional enrichment analyses showed that DNA replication, ribosome, cell cycle, and TGF-beta signaling pathway may contribute to the relapse process.
Conclusion: In summary, our analysis provided a novel eight-gene signature that could predict RFS in stage I TGCT patients.
Introduction
Testicular germ cell tumors (TGCTs) are the most commonly diagnosed tumors in young men (1–3). Most patients with TGCTs have a good prognosis; stage I patients who receive only surgical resection have an 85.5–95% 5 year survival rate, and even patients with metastasis still have a cure rate of ~80%, because TGCTs are sensitive to radiotherapy and chemotherapy, such as cisplatin and other cytostatic agents (1, 4–6). Previous studies have shown that advanced TNM stage, high serum tumor marker level (7–9), non-seminoma or predominantly embryonal carcinoma histology (10), lymph vascular invasion (11–13), rete testis invasion (14, 15), and large tumor size (15, 16) are risk factors of poor prognosis or relapse. However, based on conventional clinical or pathological characteristics, the prediction of recurrence is still not sufficiently accurate and remains controversial in TGCTs (1, 15), especially in stage I patients who are subjected to surveillance after radical orchiectomy (5). The lack of ideal prognostic biomarkers makes individualized therapy difficult. Therefore, the development of a novel discriminatory signature to identify the high-risk relapse subset of stage I TGCTs is urgently required.
The Cancer Genome Atlas (TCGA) database provides large-scale samples of TGCTs with abundant gene expression profiles and clinical information that enable a comprehensive analysis of TGCTs. In addition, another public database, the Genotype-Tissue Expression (GTEx) Project, contains the expression data of normal tissue (17, 18) that enable genomics analysis between TGCTs and normal testis.
By analyzing the clinical and expression data from TCGA and GTEx, we aimed to develop a risk score model based on gene expression in TGCTs and to explore the underlying mechanism. The results from this study may provide a new tool to guide treatment decisions for TGCT patients.
Methods
Overall Workflow and Data Downloading
Gene expression data for TGCTs, clinical information, and mutation data were downloaded from TCGA data portal (dated to February 18, 2019) by TCGAbiolinks (version 2.14.0) (19). Data of follow-up information were also downloaded from TCGA Pan-Cancer Clinical Data Resource (TCGA-CDR) to ensure data consistency from TCGA data portal (20). Human normal testis tissue gene expression profiles were downloaded from the GTEx Portal as previously described (17, 21, 22). Raw read counts were used for differential expression analysis. Transcripts per kilobase million (TPM) were used for survival analysis and model development. The GSE99420 dataset was downloaded from the Gene Expression Omnibus (GEO), which contains 60 stage I TGCTs from Princess Margaret Cancer Centre, Toronto. This dataset was based on GPL14951 platform (Illumina HumanHT-12 WG-DASL V4.0 R2 expression beadchip). Follow-up information was obtained from the contributor of GSE99420. Stage I TGCT samples in TCGA-TGCT cohort were set as training cohort for prognostic risk model construction and GSE99420 cohort as validation cohort. Figure 1 shows the overall design of this study.
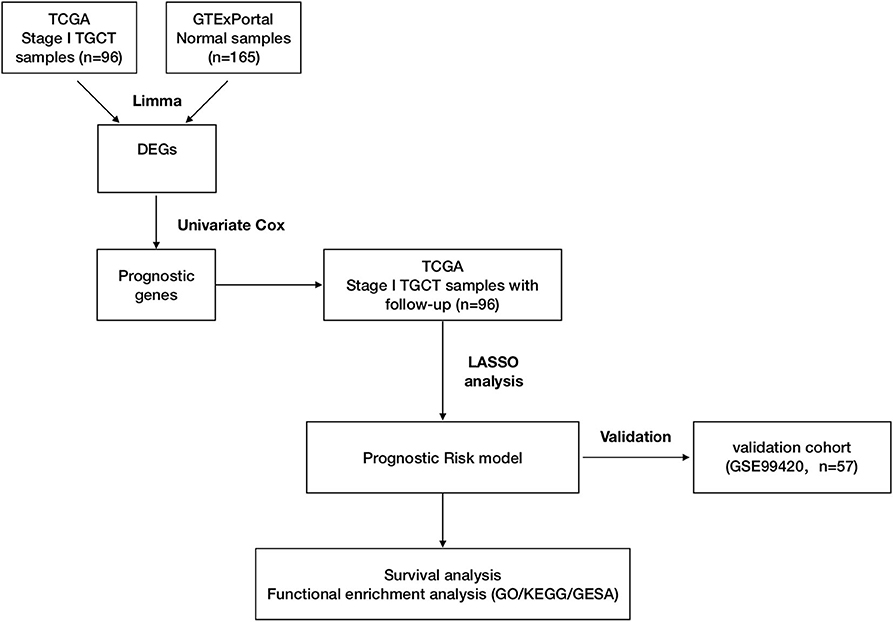
Figure 1. Workflow of construction and validation of the risk score model. The figure shows the overall workflow of the prognostic model construction and validation.
Differentially Expressed Genes and Prognostic Gene Selection
Identification of differentially expressed genes (DEGs) were using the Limma package in R (23). Genes with more than 2-fold expression were regarded as differentially expressed (adjust P < 0.05). All gene expression data from different datasets were separately log2 transformed and normalized by “limma” package in R to eliminate biological deviation. Then the genes, which were correlated with relapse-free survival (RFS), analyzed by univariate Cox proportional hazards regression (survival, version 2.43-3), were identified as prognostic genes (P < 0.05) for the subsequent construction of the prediction model (24, 25).
Risk Score Model Construction and Validation
Previously selected genes were further screened and confirmed by least absolute shrinkage and selection operator (LASSO) regression using the R package (glmnet, version 3.0-2) (26). The prognostic risk score was calculated with the following formula: risk score = β1 * (expression of RNA1) + β2 * (expression of RNA2) + ··· + βn * (expression of RNAn). We randomly repeated the procedure 200,000 times to construct the best risk score model by LASSO regression. Risk scores for each patient were calculated using the formula. Time-dependent receiver operating characteristic (ROC) curves were constructed to evaluate the optimal cutoff for risk scores in the training and validation cohort using the R package (survivalROC, version 1.0.3) (27). On the basis of their cut-off, patients were classified into either the high-risk group or low-risk group, and then survival analysis was compared between high- and low-risk patients to test the predictive power in the validation cohort.
Functional Enrichment Analysis
DEGs between high- and low-risk patients were considered hub genes for relapse in TGCT patients. Functional enrichment analyses and Gene Set Enrichment Analysis (GSEA)-based (28) Kyoto Encyclopedia of Genes and Genomes (KEGG) and Gene Ontology (GO) analyses were conducted by the R package (clusterProfiler, version 3.14.3) (29) to explore different molecular mechanisms and involved pathways between high- and low-risk patients. Normalized enrichment score was acquired using gene set permutations with 1,000 times and the cutoff P-value was 0.05 to filter the significant enrichment results.
Statistical Analysis
All statistical analyses were performed using the software R (version 3.5.2) with corresponding packages. Continuous variables were presented as means ± SD and categorical variables were displayed as percentage. With the use of an R package (survival, version 2.43.3) (24, 25), Kaplan–Meier survival analysis and the log-rank test were employed to compare RFS between the high-risk and low-risk groups in the training and validation cohorts. The area under the ROC curve (AUC) was calculated using R package (survivalROC) (27) to estimate the prognostic power in two groups. Univariate and multivariate Cox regression analyses were performed between high-risk and low-risk groups in all stage I TGCTs and different subgroups.
Results
Baseline Characteristics of Patients
After relapse time of <30 days was excluded, 96 stage I TGCT patients with relapse information were selected from TCGA-TGCT dataset as training cohort. GSE99420 included 57 stage I TGCT patients, which had relapse information, but without detail clinical information. The detailed baseline characteristics of the training and validation cohort are listed in Table 1.
Identification of Differentially Expressed Genes
As expected, the tumor and normal tissues showed significantly different clusters in principal component analysis (PCA) (Figure 2A). Differential expression analyses identified 9,391 genes differentially expressed between the stage I TGCT samples and testis tissues (3,059 upregulated and 6,332 downregulated genes, Figures 2B,C). The details of differential expression genes are shown in Supplementary Table 1.
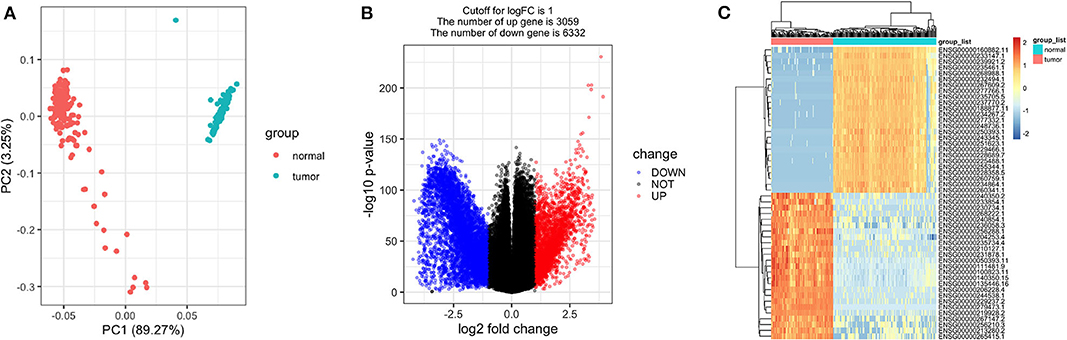
Figure 2. Differentially expressed genes between stage I testicular germ cell tumors (TGCTs) and normal testis tissues. Principal component analysis plot (A), volcano plot (B), and heatmap (C) demonstrating differentially expressed genes between stage I TGCT and normal tissues.
Development and Validation of the Risk Score Model Based on Gene Expression
Among the 9,391 candidate DEGs, 84 were identified as being independently associated with RFS in univariate Cox regression analysis (Supplementary Table 2). Based on the results of LASSO Cox regression analysis, a risk formula was developed. The risk score was calculated as follows: [Expression of GPR174 * (−1.6762644) + Expression of TCTEX1D1 * (−1.4554797) + Expression of TMEM89 * (−1.0862306) + Expression of CST2 * (−0.8966736) + Expression of SRARP * (−0.6258739) + Expression level of GSC * (−0.3162878) + Expression of PLEKHS1 * (−0.2249954) + Expression of FLG2 * (1.2350859)]. Table 2 summarized the information of the eights genes.
In this risk score model, seven genes were positively associated with RFS, and one was negatively associated with RFS. Risk scores for each patient were calculated with this formula (Supplementary Table 3).
Based on the results of time-dependent ROC curve analysis, −17.519 and −16.832 were chosen as the optimal cut-off value for the training and validation cohorts, respectively. All patients were classified into either a high-risk group or a low-risk group according to their cutoffs. Patients with a high-risk score had a shorter RFS in both the training cohort (P < 0.001, Figure 3A) and validation cohorts (P = 0.021, Figure 3B). The distribution relapse status and relapse time for each patients in training (Figure 3C) and validation cohort (Figure 3D) were plotted with a division line by risk score cutoffs, which showed more relapse events in high-risk patients.
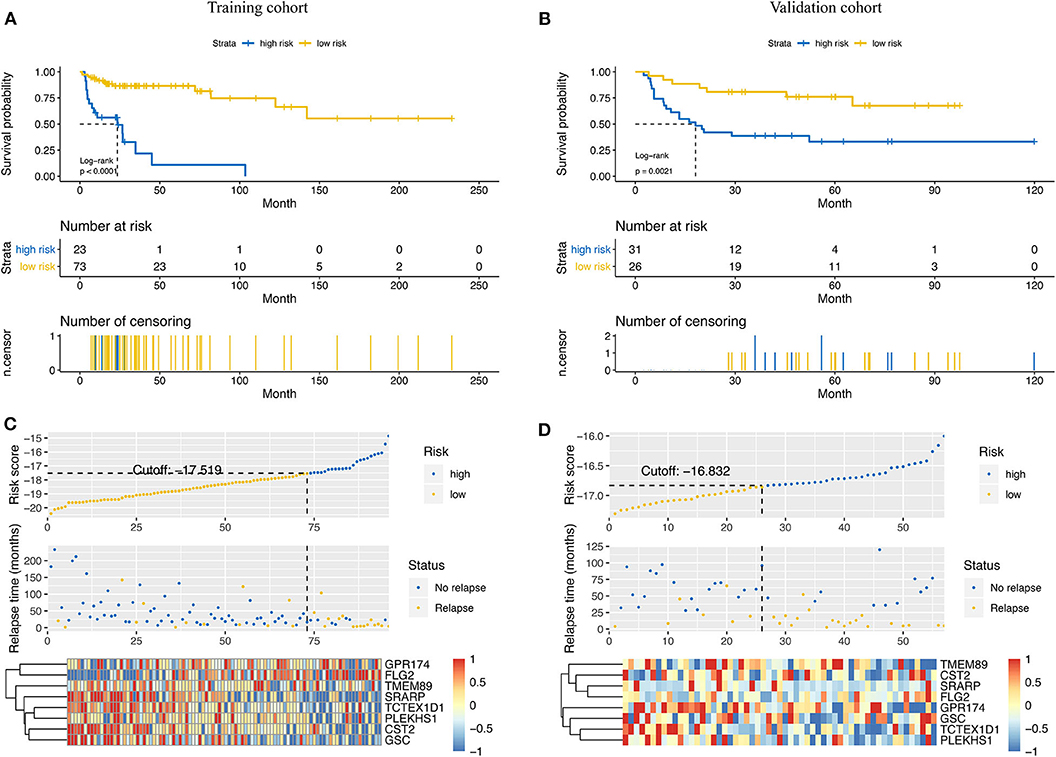
Figure 3. Prediction power of the risk score model in the training cohort and the validation cohort. Kaplan–Meier curves of relapse-free survival in the training cohort (A) and the validation cohort (B) stratified by risk scores. Rank of risk scores and relapse status for each patient in the training cohort (C) and the validation cohort (D). Heatmaps show the eight prognostic genes in the training cohort (C) and the validation cohort (D) between the high- and low-risk groups.
We assessed the prognostic accuracy of the risk score model with time-dependent ROC curve analysis at 1, 3, and 5 years in the training cohort and validation cohort (Figure 4). The AUC values of the eight-gene-based risk score model at 1, 3, and 5 years were 0.771, 0.774, and 0.805 in the training cohort, respectively. The AUC values of the risk score model at 1, 3, and 5 years were 0.715, 0.733, and 0.724 in the validation cohort, respectively.
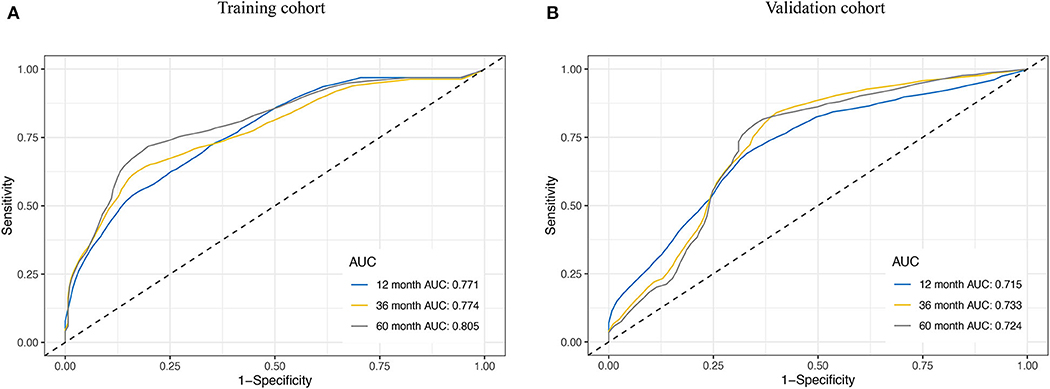
Figure 4. Receiver operating characteristic (ROC) curve analysis for the survival prediction model. The time-dependent ROC curves for relapse-free survival (RFS) were plotted at 1, 3, and 5 years to evaluate the prognostic performance of the eight-RNA risk score model in the training cohort (A) and the validation cohort (B).
As validation cohort had not enough clinical information, we only performed univariate and multivariate Cox regression analyses in TCGA-TGCT cohort to test the prognostic value of risk score. Univariate Cox regression revealed that serum markers stage, histological type, and risk score were prognostic factors for relapse in stage I TGCTs. Additionally, these significant prognostic factors were then investigated in multivariate Cox-regression analyses, and only risk score and histological type were the independent risk factors of TGCT relapse (Table 3). In different subgroups, the prognostic model also showed good performance in stratification (Table 4).
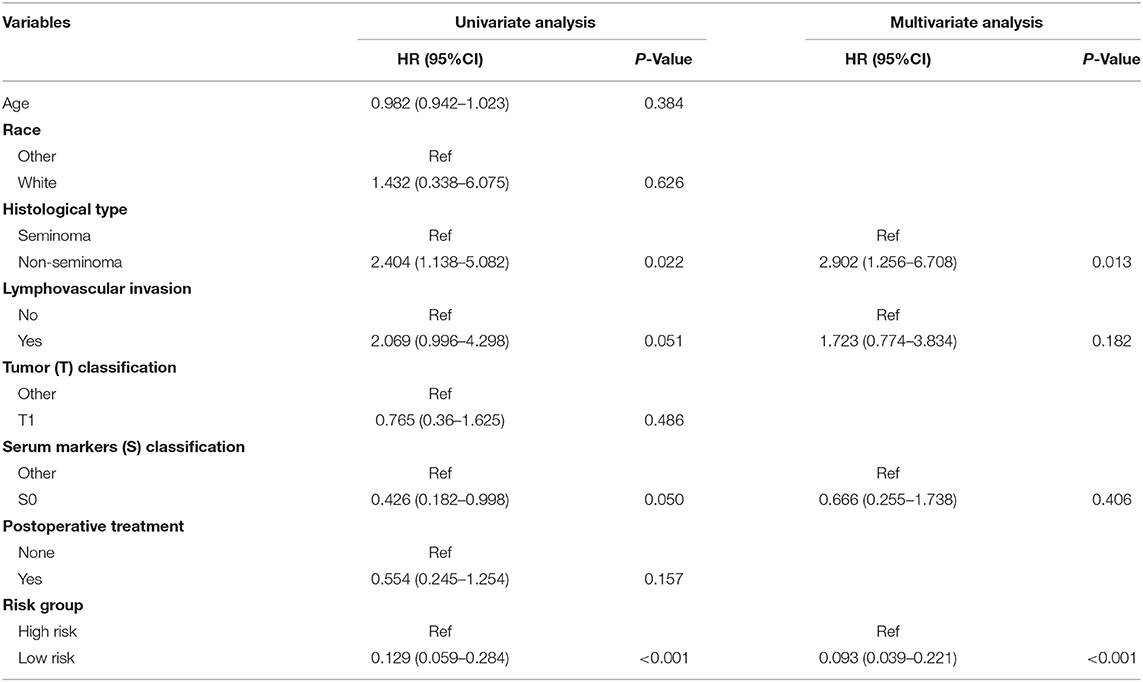
Table 3. Univariate and multivariate Cox regression analyses of risk factors associated with Relapse free Survival.
Functional Enrichment Analysis Between High- and Low-Risk Patients
To evaluate potential key molecules and pathways contributing to relapse, we performed GO analysis and GSEA between high-risk and low-risk patients. Top enriched GO terms in biological processes (BP), cellular components (CC), and molecular function (MF) are shown in Supplementary Tables 4–6, respectively. GO analysis revealed that axon genesis, regulation of cell morphogenesis, proteasomal protein catabolic process, and Ras protein signal transduction were the main terms involved in BP; cell–substrate junction, endosome membrane, and mitochondrial matrix were significantly enriched in CC; cell adhesion molecule binding, cytoskeletal protein binding, ATPase activity, and ubiquitin-like protein transferase activity were top enriched in MF (Figure 5A). Results of the KEGG pathway analysis showed that 27 pathways were enriched in high-risk TGCTs group (P < 0.05), among which the DNA replication, ribosome, cell cycle, and cytokine–cytokine receptor interaction signaling pathway may highly correlate with tumor relapse (Figure 5B, Supplementary Table 7). GSEA showed the significantly enriched hallmark terms associated with the relapse included DNA repair, MYC targets, and WNT β-catenin signaling (Figures 5C,D).
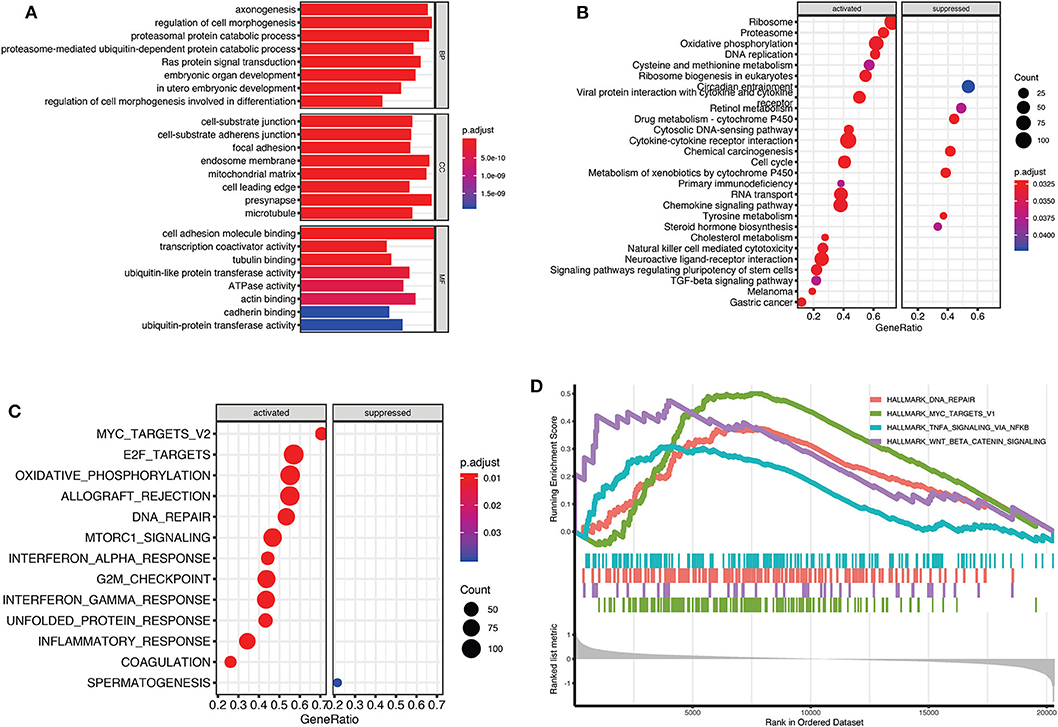
Figure 5. Gene Set Enrichment Analysis (GSEA) for Gene Ontology (GO) and Kyoto Encyclopedia of Genes and Genomes (KEGG) enrichment between high-risk and low-risk groups. (A) The top terms of molecular functions (MF), biological processes (BP), and cellular components (CC) in GO enrichment analysis. (B) GSEA-based KEGG analysis revealed the 27 significantly enriched pathways. (C) Activated and suppressed enrichment hallmarks terms in high-risk patients. (D) The visualization of some important enrichment hallmark terms.
Discussion
In the present study, we conducted a comprehensive genomic analysis of TGCTs and provided a prognostic risk model based on the expression of eight genes to predict relapse in patients with stage I TGCTs. Based on this model, the risk score was an independent prognostic factor for relapse in patients with stage I TGCTs.
Currently, TGCT patients have a 95% cure rate at first diagnosis and an expected 80% cure rate at the metastatic stage (4). However, the optimal management strategy for stage I TGCTs is one of the most controversial aspects of postoperative care (1, 5, 30, 31). Relapses are observed among 20–28% of TGCT patients who undergo surveillance after orchiectomy. Lack of satisfactory stratification that prevents overtreatment in low-risk patients and selection of patients with a high risk of relapse are difficult in clinical practice.
Classic clinical characteristics such as tumor type, serum tumor markers [alpha-fetoprotein (AFP), human chorionic gonadotropin (HCG), and lactate dehydrogenase (LDH)] and TNM stage can act as risk factors for relapse, but these clinical characteristics have low accuracy and low sensitivity for predicting relapse and are unable to meet the needs of clinical guidance. Terbuch et al. reported that serum miR-371a-3p is increased during TGCT recurrence in patients (32). The serum miRNA marker displays a diagnostic value for recurrence; however, it cannot be used for risk stratification in TGCT patients.
Gilbert et al. reported that CXCL12 expression can act as a prognostic index for stage I non-seminoma (33). Nevertheless, a meta-analysis summarized that no single risk factor could predict relapse in stage I seminoma (15). Korkola et al. identified an eight-gene model that can predict overall survival in patients with TGCTs in 2009, but without validation of RFS (34). Lewin et al. also reported a discriminatory gene expression profile between relapsed and non-relapsed cases on the basis of 10- and 30-gene signatures (35). However, the prediction score by Lewin et al. was limited to a cohort with single histology and lacked validation in large external sets. Our eight-gene-based risk score model had the ability to identify patients with a high risk of relapse and may suggest a risk-adapted adjuvant approach for TGCTs. The predictability was validated in an independent external cohort.
In this prognostic signature, seven had a protective role in TGCT relapse, and one was a risk factor for relapse in TGCT patients. By searching previous publications of those genes, results showed that all were newly reported associated with the prognosis of TGCTs. Some genes also play important biological roles in cancer development and progression. For example, SRARP (Steroid Receptor Associated And Regulated Protein) is a protein coding gene, which could function as a tumor suppressors and predict clinical outcome in malignancies (36). PLEKHS1 (pleckstrin homology domain containing S1) promoter mutations are a poor prognosis genetic marker for thyroid cancer (37).
GO analysis indicated that cell adhesion, cytoskeleton, Ras protein signal transduction, and autophagy most likely contribute to the relapse of TGCTs. From the results of GSEA-based KEGG pathway analysis, cell cycle, DNA replication, and TGF-beta signaling pathway were famous pathways of tumorigenesis; these pathways may be associated with metastatic behavior (38). Activated hallmark terms, such as MYC targets and WNT β-catenin signaling, also indicated the possible mechanisms of relapse in high-risk patients. For example, the activity of the MYC oncogene regulates tumor metastasis through specific effects on cancer cell invasion and migration (39). Wnt/β-catenin signaling could promote tumor metastasis via maintain epithelial-to-mesenchymal transition (EMT) or cancer stemness (40, 41). These pathways and detailed mechanisms that affect the relapse of TGCT require further investigation.
To the best of our knowledge, this is the first validated prognostic signature for stage I TGCT, and the present study included a large number of normal testis tissues and TGCT samples. The previous similar prognostic model study conducted by Korkola was based on microarrays and a small number of normal tissues (34). Our results provide novel biomarker candidates for TGCT studies and potential targets for treating TGCTs.
There are some limitations in the present study. The constructed risk score system was based on expression results, without consideration of the mutations, methylation status, or other genetic events that may be more important drivers of TGCTs. Further research on biological processes is still required to better understand the biology of TGCTs.
Conclusions
In the present study, we developed a novel eight-gene-based risk model for predicting the RFS of stage I TGCT patients. The relapse risk prediction model provides an approach to individualize treatment decisions for stage I TGCT patients.
Data Availability Statement
The datasets generated for this study can be found in the TCGA and GSE99420.
Author Contributions
J-GZ, JY, S-HJ, and HM conceived, designed, or planned the study. JY and J-GZ analyzed the data. T-YZ and S-HJ acquired the data. J-GZ, S-HJ, LS, and HM helped interpret the results. J-GZ and JY provided study materials or patient data. J-GZ, JY, SX, S-HJ, UG, and HM drafted the manuscript. All authors revised and reviewed this work and gave their final approval of the submitted manuscript.
Funding
This research was funded by the National Natural Science Foundation of China (Grant no. 81660512), Research Programs of Science and Technology Commission Foundation of Zunyi City (Grant nos. HZ2019-11 and HZ2019-07), Research Programs of Health Commission Foundation of Guizhou Province (Grant nos. gzwjkj2019-1-073 and gzwjkj2019-1-172). The study funders had no role in the design of the study; the collection, analysis, or interpretation of the data; the writing of the manuscript; or the decision to submit the manuscript for publication.
Conflict of Interest
The authors declare that the research was conducted in the absence of any commercial or financial relationships that could be construed as a potential conflict of interest.
Acknowledgments
The results shown in the present study are in whole or part based on data generated by the TCGA Research Network (https://www.cancer.gov/tcga) and the Genotype-Tissue Expression (GTEx) Project (http://www.GTExportal.org). We thank Dr. Aaron Hansen from University Health Network (Hansen-Zunyi-2020-0783) for providing survival and clinical information of GSE99420.
Supplementary Material
The Supplementary Material for this article can be found online at: https://www.frontiersin.org/articles/10.3389/fonc.2020.01147/full#supplementary-material
Abbreviations
TCGA, The Cancer Genome Atlas; GTEx, Genotype-Tissue Expression Project; GEO, Gene Expression Omnibus; TGCTs, testicular germ cell tumors; FDR, false discovery rate; ROC, receiver operating characteristic; AUC, area under the ROC curve; GO, Gene Ontology; KEGG, Kyoto Encyclopedia of Genes and Genomes; MF, molecular functions; BP, biological processes; CC, cellular components; TPM, transcripts per kilobase million; CI, confidence interval; HR, hazard ratio; DEGs, differentially expressed genes; RFS, relapse-free survival; PCA, principal component analysis; LASSO, least absolute shrinkage and selection operator.
References
1. Cheng L, Albers P, Berney DM, Feldman DR, Daugaard G, Gilligan T, et al. Testicular cancer. Nat Rev Dis Primers. (2018) 4:29. doi: 10.1038/s41572-018-0029-0
2. Chovanec M, Cheng L. Molecular characterization of testicular germ cell tumors: chasing the underlying pathways. Fut Oncol. (2019) 15:227–9. doi: 10.2217/fon-2018-0617
3. Sheikine Y, Genega E, Melamed J, Lee P, Reuter VE, Ye H. Molecular genetics of testicular germ cell tumors. Am J Cancer Res. (2012) 2:153–67.
4. Hanna NH, Einhorn LH. Testicular cancer–discoveries and updates. N Engl J Med. (2014) 371:2005–16. doi: 10.1056/NEJMra1407550
5. Tandstad T, Smaaland R, Solberg A, Bremnes RM, Langberg CW, Laurell A, et al. Management of seminomatous testicular cancer: a binational prospective population-based study from the Swedish norwegian testicular cancer study group. J Clin Oncol. (2011) 29:719–25. doi: 10.1200/JCO.2010.30.1044
6. Einhorn LH. Treatment of testicular cancer: a new and improved model. J Clin Oncol. (1990) 8:1777–81. doi: 10.1200/JCO.1990.8.11.1777
7. von Eyben FE. Laboratory markers and germ cell tumors. Crit Rev Clin Lab Sci. (2003) 40:377–427. doi: 10.1080/10408360390247814
8. Amin MB, Edge S, Greene S, Byrd F, Brookland RK, Washington MK, et al. AJCC Cancer Staging Manual, 8th ed. New York, NY: Springer (2017).
9. Murray MJ, Huddart RA, Coleman N. The present and future of serum diagnostic tests for testicular germ cell tumours. Nat Rev Urol. (2016) 13:715–25. doi: 10.1038/nrurol.2016.170
10. Atsu N, Eskicorapci S, Uner A, Ekici S, Gungen Y, Erkan I, et al. A novel surveillance protocol for stage I nonseminomatous germ cell testicular tumours. BJU Int. (2003) 92:32–5. doi: 10.1046/j.1464-410X.2003.04270.x
11. Fossa SD, Jacobsen AB, Aass N, Heilo A, Stenwig AE, Kummen O, et al. How safe is surveillance in patients with histologically low-risk non-seminomatous testicular cancer in a geographically extended country with limited computerised tomographic resources? Br J Cancer. (1994) 70:1156–60. doi: 10.1038/bjc.1994.464
12. Daugaard G, Gundgaard MG, Mortensen MS, Agerbaek M, Holm NV, Rorth MR, et al. Surgery after relapse in stage I nonseminomatous testicular cancer. J Clin Oncol. (2015) 33:2322. doi: 10.1200/JCO.2014.60.2177
13. Albers P, Siener R, Kliesch S, Weissbach L, Krege S, Sparwasser C, et al. Risk factors for relapse in clinical stage I nonseminomatous testicular germ cell tumors: results of the German testicular cancer study group trial. J Clin Oncol. (2003) 21:1505–12. doi: 10.1200/JCO.2003.07.169
14. Chung P, Daugaard G, Tyldesley S, Atenafu EG, Panzarella T, Kollmannsberger C, et al. Evaluation of a prognostic model for risk of relapse in stage I seminoma surveillance. Cancer Med. (2015) 4:155–60. doi: 10.1002/cam4.324
15. Boormans JL, de Castro JM, Marconi L, Yuan Y, Laguna Pes MP, Bokemeyer C, et al. Testicular tumour size and rete testis invasion as prognostic factors for the risk of relapse of clinical stage I Seminoma testis patients under surveillance: a systematic review by the testicular cancer guidelines panel. Eur Urol. (2017) 73:394–405. doi: 10.1016/S1569-9056(18)32055-4
16. Warde P, Specht L, Horwich A, Oliver T, Panzarella T, Gospodarowicz M, et al. Prognostic factors for relapse in stage I seminoma managed by surveillance: a pooled analysis. J Clin Oncol. (2002) 20:4448–52. doi: 10.1200/JCO.2002.01.038
17. Carithers LJ, Moore HM. The Genotype-Tissue Expression (GTEx) project. Biopreserv Biobank. (2015) 13:307–8. doi: 10.1089/bio.2015.29031.hmm
18. Lonsdale J, Thomas J, Salvatore M, Phillips R, Lo E, Shad S, et al. The Genotype-Tissue Expression (GTEx) project. Nat Genet. (2013) 45:580–5. doi: 10.1038/ng.2653
19. Colaprico A, Silva TC, Olsen C, Garofano L, Cava C, Garolini D, et al. TCGAbiolinks: an R/Bioconductor package for integrative analysis of TCGA data. Nucleic Acids Res. (2016) 44:e71. doi: 10.1093/nar/gkv1507
20. Liu J, Lichtenberg T, Hoadley KA, Poisson LM, Lazar AJ, Cherniack AD, et al. An integrated TCGA pan-cancer clinical data resource to drive high-quality survival outcome analytics. Cell. (2018) 173:400–16.e11. doi: 10.1158/1538-7445.AM2018-3287
21. Kosti I, Jain N, Aran D, Butte AJ, Sirota M. Cross-tissue analysis of gene and protein expression in normal and cancer tissues. Sci Rep. (2016) 6:24799. doi: 10.1038/srep24799
22. Ardlie KG, deLuca DS, Segre AV, Sullivan TJ, Young TR, Gelfand ET, et al. The Genotype-Tissue Expression (GTEx) pilot analysis: multitissue gene regulation in humans. Science. (2015) 348:648–60. doi: 10.1126/science.1262110
23. Ritchie ME, Phipson B, Wu D, Hu YF, Law CW, Shi W, et al. limma powers differential expression analyses for RNA-sequencing and microarray studies. Nucleic Acids Res. (2015) 43:e47. doi: 10.1093/nar/gkv007
24. Therneau TM, Grambsch PM, Fleming T. A Package for Survival Analysis in S. version 2.38. (2015). Available online at: https://CRAN.R-project.org/package=survival (accessed April 16, 2020).
25. Therneau TM, Grambsch PM. Modeling Survival Data: Extending the Cox Model. New York, NY: Springer (2000).
26. Friedman J, Hastie T, Tibshirani R. Regularization paths for generalized linear models via coordinate descent. J Stat Softw. (2010) 33:1–22. doi: 10.18637/jss.v033.i01
27. Heagerty PJ, Lumley T, Pepe MS. Time-dependent ROC curves for censored survival data and a diagnostic marker. Biometrics. (2000) 56:337–44. doi: 10.1111/j.0006-341X.2000.00337.x
28. Varemo L, Nielsen J, Nookaew I. Enriching the gene set analysis of genome-wide data by incorporating directionality of gene expression and combining statistical hypotheses and methods. Nucleic Acids Res. (2013) 41:4378–91. doi: 10.1093/nar/gkt111
29. Yu G, Wang LG, Han Y, He QY. clusterProfiler: an R package for comparing biological themes among gene clusters. Omics. (2012) 16:284–7. doi: 10.1089/omi.2011.0118
30. Oldenburg J, Aparicio J, Beyer J, Cohn-Cedermark G, Cullen M, Gilligan T, et al. Personalizing, not patronizing: the case for patient autonomy by unbiased presentation of management options in stage I testicular cancer. Ann Oncol. (2015) 26:833–8. doi: 10.1093/annonc/mdu514
31. Feldman DR. Treatment options for stage I nonseminoma. J Clin Oncol. (2014) 32:3797–800. doi: 10.1200/JCO.2014.56.8006
32. Terbuch A, Adiprasito JB, Stiegelbauer V, Seles M, Klec C, Pichler GP, et al. MiR-371a-3p serum levels are increased in recurrence of testicular germ cell tumor patients. Int J Mol Sci. (2018) 19:3130. doi: 10.3390/ijms19103130
33. Gilbert DC, Al-Saadi R, Thway K, Chandler I, Berney D, Gabe R, et al. Defining a new prognostic index for stage I nonseminomatous germ cell tumors using CXCL12 expression and proportion of embryonal Carcinoma. Clin Cancer Res. (2016) 22:1265–73. doi: 10.1158/1078-0432.CCR-15-1186
34. Korkola JE, Houldsworth J, Feldman DR, Olshen AB, Qin LX, Patil S, et al. Identification and validation of a gene expression signature that predicts outcome in adult men with germ cell tumors. J Clin Oncol. (2009) 27:5240–7. doi: 10.1200/JCO.2008.20.0386
35. Lewin J, Soltan Ghoraie L, Bedard PL, Hamilton RJ, Chung P, Moore M, et al. Gene expression signatures prognostic for relapse in stage I testicular germ cell tumours. BJU Int. (2018) 122:814–22. doi: 10.1111/bju.14372
36. Naderi A. SRARP and HSPB7 are epigenetically regulated gene pairs that function as tumor suppressors and predict clinical outcome in malignancies. Mol Oncol. (2018) 12:724–55. doi: 10.1002/1878-0261.12195
37. Jung CK, Jung SH, Jeon S, Jeong YM, Kim Y, Lee S, et al. Risk stratification using a novel genetic classifier including PLEKHS1 promoter mutations for differentiated thyroid cancer with distant metastasis. Thyroid. (2020). doi: 10.1089/thy.2019.0459. [Epub ahead of print].
38. Hanahan D, Weinberg RA. Hallmarks of cancer: the next generation. Cell. (2011) 144:646–74. doi: 10.1016/j.cell.2011.02.013
39. Wolfer A, Ramaswamy S. MYC and metastasis. Cancer Res. (2011) 71:2034–7. doi: 10.1158/0008-5472.CAN-10-3776
40. Zhang Q, Bai X, Chen W, Ma T, Hu Q, Liang C, et al. Wnt/β-catenin signaling enhances hypoxia-induced epithelial-mesenchymal transition in hepatocellular carcinoma via crosstalk with hif-1α signaling. Carcinogenesis. (2013) 34:962–73. doi: 10.1093/carcin/bgt027
Keywords: testicular germ cell tumors, The Cancer Genome Atlas, risk score model, relapse, GEO
Citation: Zhou J-G, Yang J, Jin S-H, Xiao S, Shi L, Zhang T-Y, Ma H and Gaipl US (2020) Development and Validation of a Gene Signature for Prediction of Relapse in Stage I Testicular Germ Cell Tumors. Front. Oncol. 10:1147. doi: 10.3389/fonc.2020.01147
Received: 18 November 2019; Accepted: 08 June 2020;
Published: 30 July 2020.
Edited by:
Haitao Wang, National Cancer Centre Singapore, SingaporeCopyright © 2020 Zhou, Yang, Jin, Xiao, Shi, Zhang, Ma and Gaipl. This is an open-access article distributed under the terms of the Creative Commons Attribution License (CC BY). The use, distribution or reproduction in other forums is permitted, provided the original author(s) and the copyright owner(s) are credited and that the original publication in this journal is cited, in accordance with accepted academic practice. No use, distribution or reproduction is permitted which does not comply with these terms.
*Correspondence: Hu Ma, bWFodWFiQDE2My5jb20=; Udo S. Gaipl, VWRvLkdhaXBsQHVrLWVybGFuZ2VuLmRl
†These authors have contributed equally to this work and share first authorship