- 1Southern Marine Science and Engineering Guangdong Laboratory (Zhanjiang), The Marine Biomedical Research Institute, Guangdong Medical University, Zhanjiang, China
- 2Cancer Center, The Affiliated Hospital, Guangdong Medical University, Zhanjiang, China
- 3School of Foreign Languages, Guangdong Medical University, Dongguan, China
Background: Patients with glioblastoma have a poor prognosis. We want to develop and validate nomograms for predicting overall survival in patients with glioblastoma.
Methods: Data of patients with glioblastoma diagnosed pathologically in the SEER database from 2007 to 2016 were collected by SEER*Stat software. After eliminating invalid and missing clinical information, 3,635 patients (total group) were finally identified and randomly divided into the training group (2,183 cases) and the verification group (1,452 cases). Cox proportional risk regression model was used in the training group, the verification group and the total group to analyze the prognostic factors of patients in the training group, and then the nomogram was constructed. C-indexes and calibration curves were used to evaluate the predictive value of nomogram by internal (training group data) and external validation (verification group data).
Results: Cox proportional risk regression model in the training group showed that age, year of diagnosis, laterality, radiation, chemotherapy were all influential factors for prognosis of patients with glioblastoma (P < 0.05) and were all used to construct nomogram as well. The internal and external validation results of nomogram showed that the C-index of the training group was 0.729 [95% CI was (0.715, 0.743)], and the verification group was 0.734 [95% CI was (0.718, 0.750)]. The calibration curves of both groups showed good consistency.
Conclusions: The proposed nomogram resulted in accurate prognostic prediction for patients with glioblastoma.
Introduction
Glioma is the most common primary central nervous system malignancy in adults, with an annual incidence of 5.26 per 100,000 people (1). In the WHO classification, grade IV glioma is glioblastoma (GBM) (2), which is the highest level (3) in the WHO classification of brain tumors. It is highly malignant (4) and patients have poor prognosis. It is a kind of cancer that is difficult to treat. Conventional treatments for glioblastoma include surgery, alkylation chemotherapy, and radiotherapy. Traditional treatments are often ineffective, not only because glioblastoma is highly invasive, but also because the blood-brain barrier prevents drugs from killing tumor cells completely. Within 2 cm of the primary site, 77% of GBM will recur (5). 72% of the cases will recur in field of radiotherapy (6). These factors are also associated with poor prognosis in patients with GBM.
SEER database (surveillance epidemiology and end results) (https://seer.cancer.gov/) is from the National Cancer Institute (NCI). This database records in detail the demographic information, tumor site and morphology, diagnosis stage, treatment and prognosis of millions of patients with malignant tumors and carcinoma in situ in some states since 1973 (7), which provides good data for clinical studies of tumors. Nomogram includes a variety of cancer-related risk factors and presents their impacts on patients' survival in a visual way. It can personally predict the survival rate of patients and it is a common tool for prognosis assessment of cancer patients (8–10). In this study, we extracted the cases of glioblastoma in SEER database from 2007 to 2015, and constructed a nomogram to predict the survival rate of patients and guide clinical prognosis and treatment decisions.
Patients and Methods
We used SEER*Stat (version 8.3.4) to collect 128,554 cases of nervous system tumors diagnosed pathologically. Then Excel 2016 was used to perform data cleaning to eliminate invalid data and select patients with glioblastoma from 2009 to 2015 (Tables 1, 2).
The data set partitioning function [createDataPartition] in the “caret” package of R version 3.5.3 was used for random grouping, so the cleaned data of patients were randomly divided into training group and verification group. In the training group, “survival” package was used for univariate and multivariate Cox proportional risk regression model analysis to screen prognostic factors, and “rms” package was used to construct nomogram. In nomogram's external validation, we calculated the total points of each patient based on the constructed nomogram in the verification group and Cox regression was performed with the total points as a factor. We calculated the C-index and drew calibration curve by Bootstrap method (resampling number B=100) in the training group and the verification group, respectively, for internal and external validation. The higher the C-index is, the more accurate the prognosis is (11). In the calibration curve, if the predicted value is equal to the actual observed value, the curve will be infinitely close to the ideal 45° slant (12). Risk score was constructed, and ROC (receiver operating characteristic) curve was derived to evaluate the predicting value. Both the C- index and area under the ROC curve (AUC) can be used to evaluate the discrimination between the real value and the predicted value of the model (12). Our study procedure is shown in Figure 1.
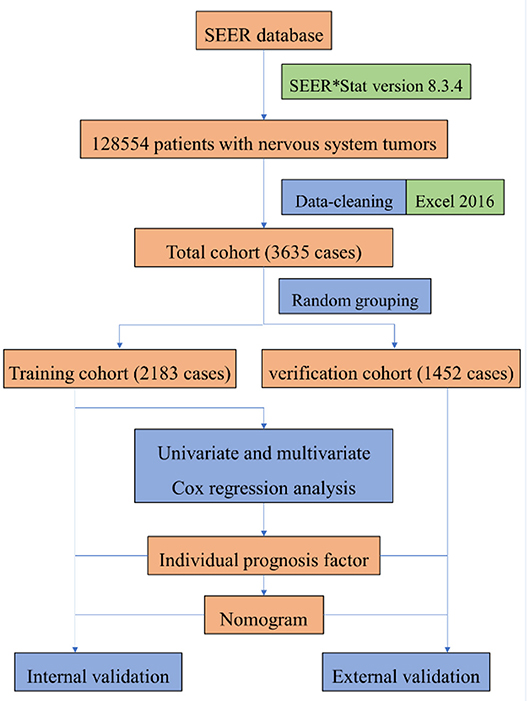
Figure 1. The procedure of building a nomogram for patients with glioblastoma. Firstly, we derived patients with nervous system tumors from the SEER database, then selected patients with glioblastoma, and eliminated the invalid data. Secondly, univariate and multivariate Cox regression analysis were performed to obtain individual variables affecting prognosis. Thirdly, individual variables were used to construct nomogram that predicted the prognosis of patients, then internal and external validation were performed.
Results
Demographics and Clinicopathologic Characteristics of Patients
This study included the following characteristics into the analysis: age, race, sex, year of diagnosis, degree of differentiation, laterality, primary site surgery, radiation sequence with surgery, reason no cancer-directed surgery, radiotherapy, chemotherapy, tumor size, cause-specific death classification, other cause of death classification, sequence number, NHIA (Hispanic, Non-Hisp), age at diagnosis, insurance, marital status at diagnosis (Table 3).
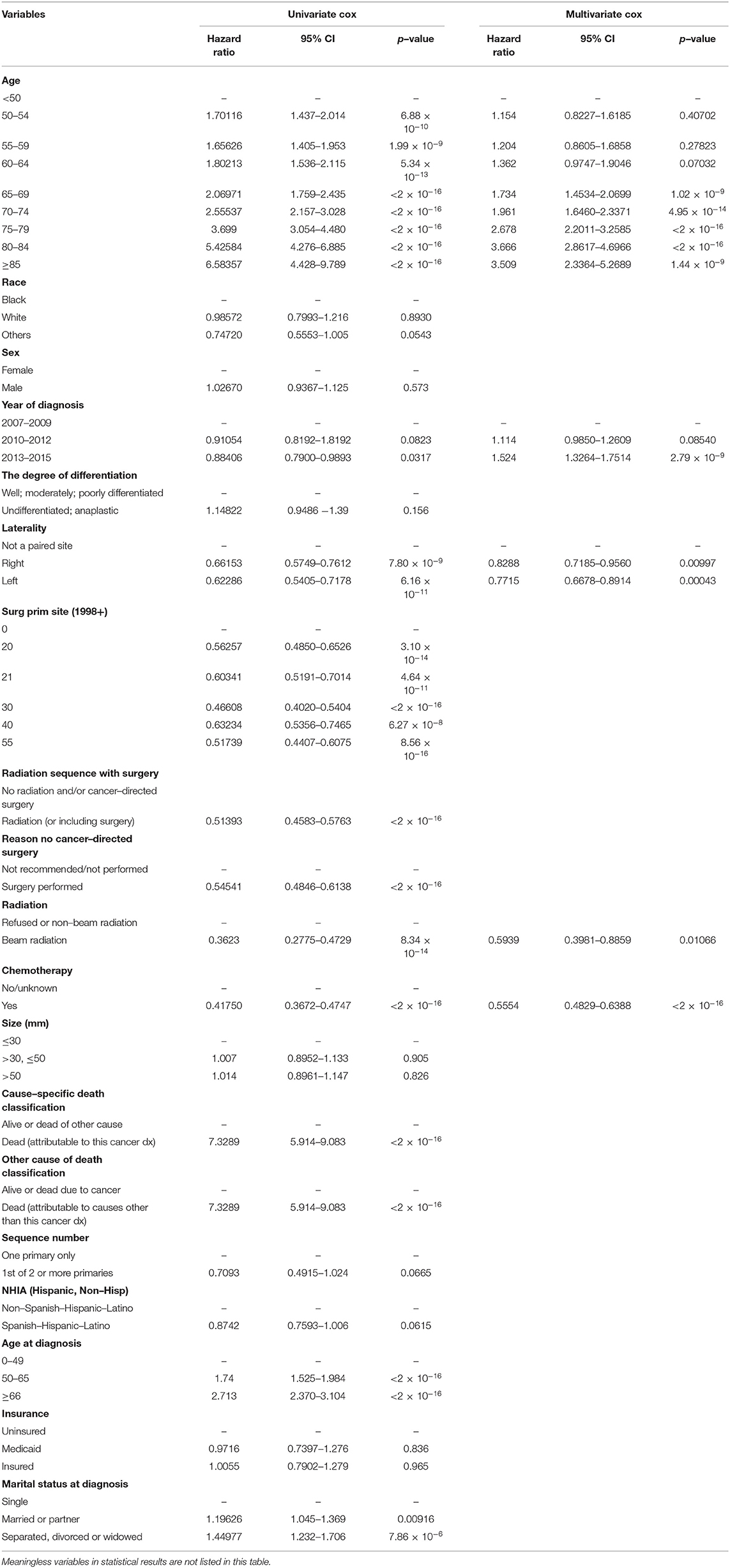
Table 3. Univariate and multivariate analyses of prognostic parameter in glioblastoma using the Cox regression model.
Independent Prognostic Factors in the Training Group
The results of the univariate analysis have been listed in Table 3. Multivariate Cox analyses demonstrated that age, year of diagnosis, laterality, radiotherapy, chemotherapy were independent risk factors for overall survival (OS) (Figure 2A).
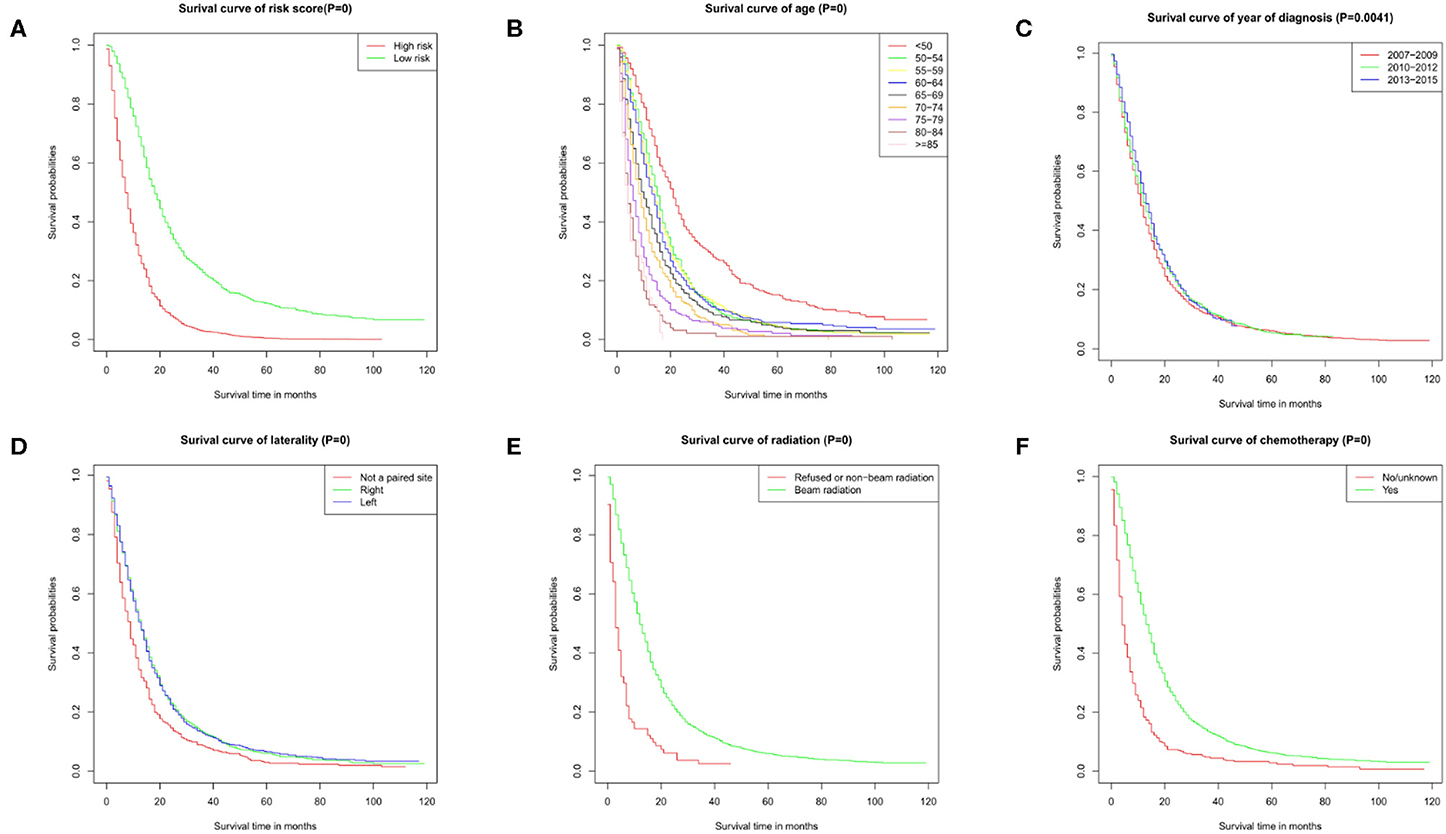
Figure 2. Survival curves. Survival curve of (A) risk score, (B) age, (C) year of diagnosis, (D) laterality, (E) radiation, and (F) chemotherapy. These graphs show the impact of each subtype on survival. P = 0 means P < 0.001.
Prognostic Nomogram for OS
Nomogram was built by the “rms” package in R version 3.5.3 based on the results of multivariate analysis (Figure 3). The rcorrp.cens package in Hmisc was used to calculate the C-index for measuring the performance of the nomogram. The C-index for OS prediction was 0.729 (95% CI, 0.715–0.743). Calibration curves of 1-, 3-, or 5-years survival rates show good agreement between nomogram predictions and actual observations (Figures 4A–C). In training group, 1-, 3-, and 5-years survival AUCs were 0.722, 0.700, and 0.722, respectively (Figures 5A–C). Figures 2B–F showed the OS of the patients with GBM, and the survival curve declined sharply in the first 20 months.
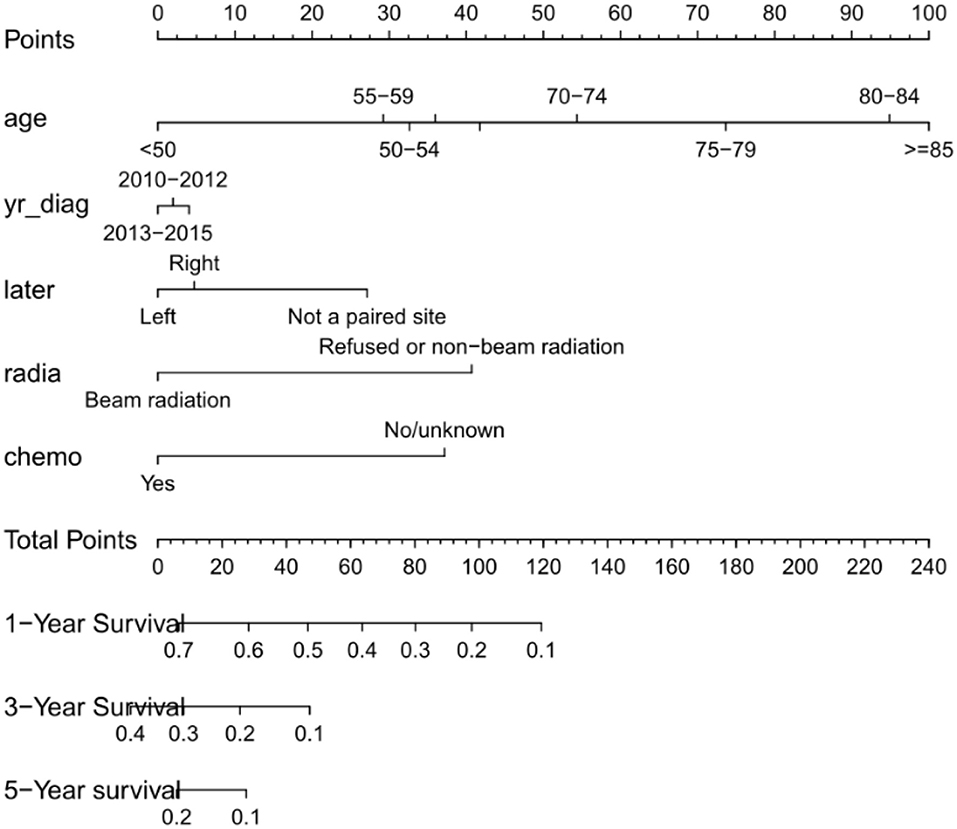
Figure 3. Nomogram predicting patients' 1 -, 3 -, and 5-years over survival with glioblastoma. The specific subtypes of each variables are projected to the point scale to obtain a value. The higher the value, the worse the prognosis of patients. The total value can be obtained by summing up the values of each variables. The 1-year, 3-years, and 5-years over survival of the patient can be obtained by downward projection of the total value in the total point scale. yr_diag, year of diagnosis; later, laterality; radia, radiation; chemo, chemotherapy.
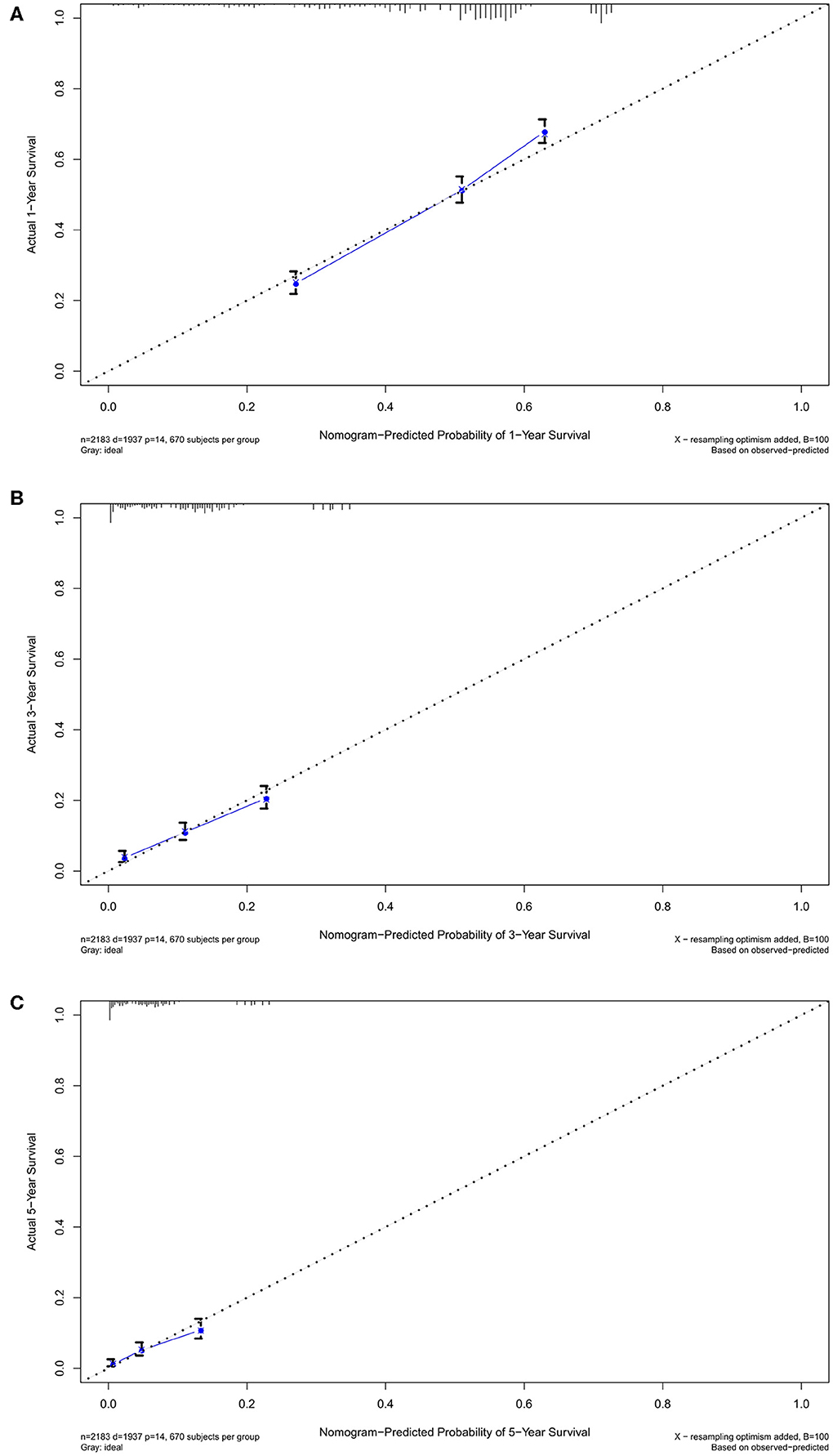
Figure 4. The calibration curves in training cohort. The calibration curves of the nomogram predicting (A) 1-year, (B) 3-years, and (C) 5-years OS.
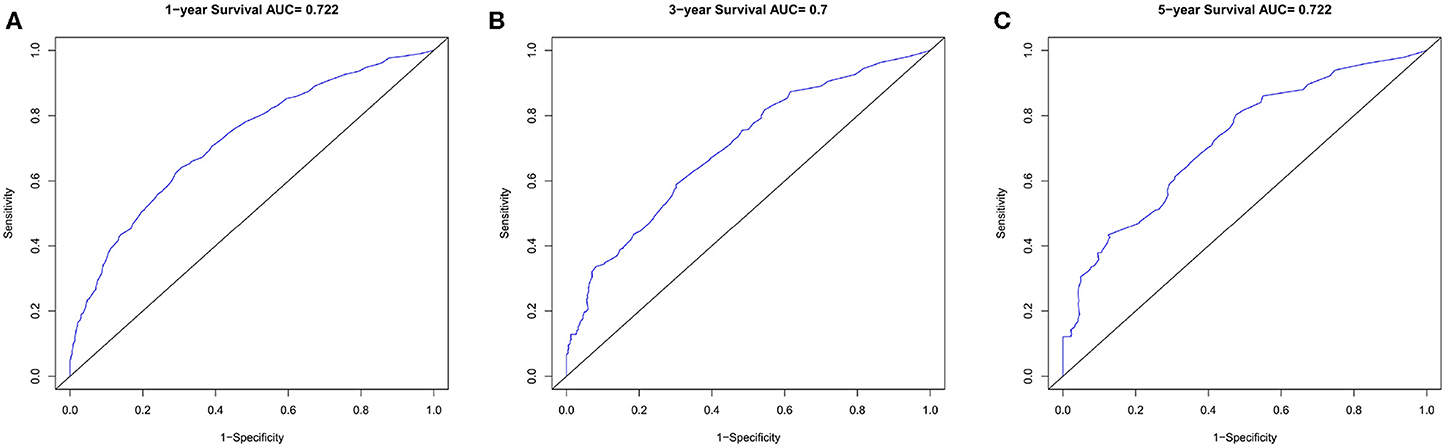
Figure 5. The ROC curves in training cohort. (A) 1-year, (B) 3-years, (C) 5-years survival ROC curves.
Validation of Predictive Accuracy of the Nomogram for OS
In the verification group, the C-index of the nomogram for predicting OS was 0.734 (95% CI, 0.718–0.750), and calibration curves showed good agreement between nomogram-predicted probability and actual observations of 1-, 3-, or 5-years survival (Figures 6A–C). In verification group, 1-, 3-, and 5-years survival AUCs were 0.703, 0.672, and 0.640, respectively (Figures 7A–C).
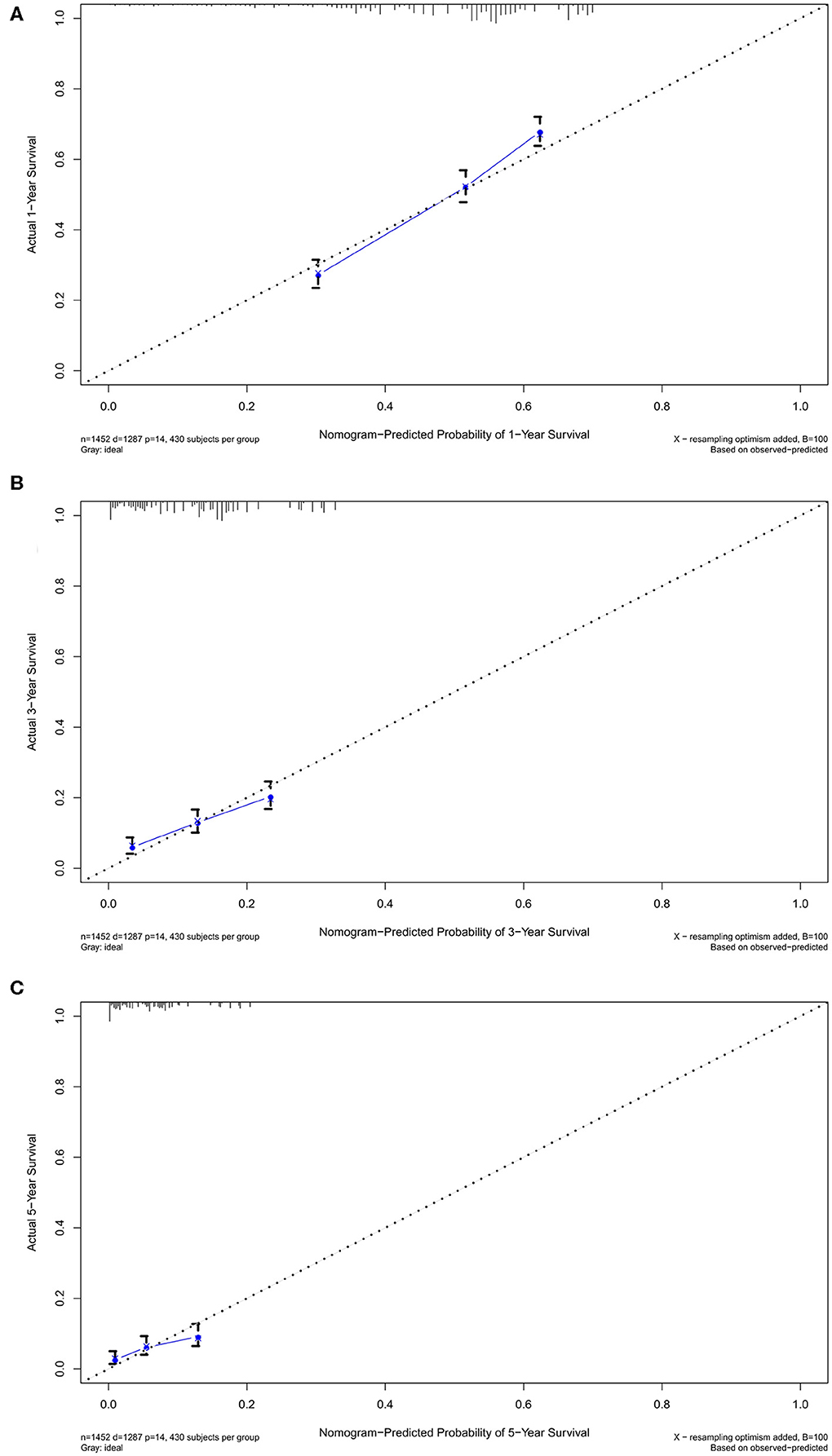
Figure 6. The calibration curves in verification cohort. The calibration curves of the nomogram predicting (A) 1-year, (B) 3-years, and (C) 5-years OS.
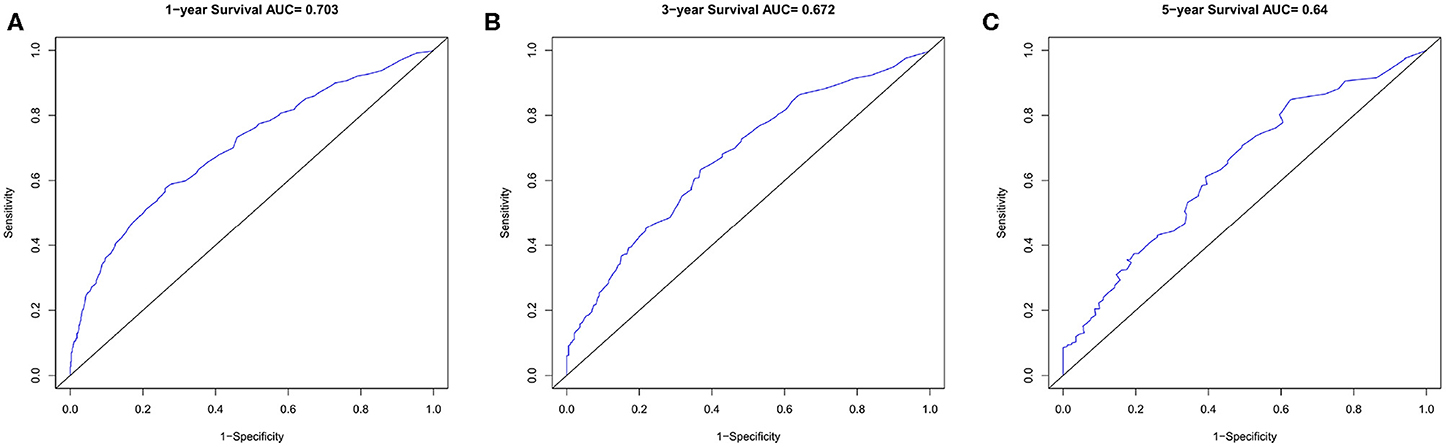
Figure 7. The ROC curves in verification cohort. (A) 1-year, (B) 3-years, (C) 5-years survival ROC curves.
Discussion
Accurate and effective prognosis assessment is of clinical significance for individualized treatment and follow-up treatment of patients with GBM. GBM is usually diagnosed in late stage (13) by MRI with poor prognosis, therefore, a complete prognostic scoring system is essential. Nomogram is a statistical tool that integrates a variety of prognostic risk factors and visualizes the overall impact of these risk factors on survival in each patient (14) to help clinicians develop intervention plan. Compared with other rating systems (such as AJCC 8th edition TNM staging system and the Nathan staging system), nomogram is more convenient and accurate, with a higher C-index, showing better predictive value (15, 16). Many scholars have produced nomograms for some tumors, such as intrahepatic cholangiocarcinoma (17), invasive pulmonary adenocarcinoma (18), colorectal cancer (19), hepatocellular carcinoma with pulmonary metastasis (20), etc. SEER collects 450,000 cancer cases with high-quality information each year, and adjusts the collection of cancer staging information according to changes in cancer staging systems, such as AJCC (7). So that it provides a good data basis for establishing of nomogram.
To construct the nomogram, independent predictors of OS in patients with GBM should be determined first. Univariate and multivariate Cox proportional risk regression models were used to determine independent prognostic factors for OS. Multivariate Cox regression proportional analysis showed that age, year of diagnosis, laterality, radiation, chemotherapy were independent prognostic factors for OS in patients with GBM.
It is an acceptable view that the prognosis of cancer patients is worse with aging. Ladomersky et al. analyzed several databases (SEER, GTEx, and 10 k Immunomes) and found that the death rate of patients with GBM over 65 years old was more than seven times higher than that of patients under 65 years old, thus the prognosis of patients with GBM over 65 years old was much worse than that of patients with GBM under 65 years old (21). In our study, the results of multivariate analysis further showed that, for patients aged at least 65 years old, the older they were, the higher the HR (hazard ratio) was and the worse the prognosis was. That was similar to the results of Bartek's SEER based study, which showed a 0.8% increased risk of death from glioblastoma with each additional year of age at diagnosis (CI 1.008–1.008, p < 0.001) (22). Elderly patients are more likely to develop other high-risk complications (23–26). In addition, aging may promote the initiation or growth of GBM cells by suppressing the immune system, and may reduce the effectiveness of immunotherapy for patients with glioblastoma (21). All those will reduce the prognostic survival rate of patients with GBM.
For newly diagnosed glioblastoma, the most important treatment is the resection of the contrast-enhanced region on imaging, followed by radiotherapy and chemotherapy (27, 28). The results of our multivariate analysis showed that both beam radiotherapy and chemotherapy can immensely reduce the risk of patients with glioblastoma and improve the survival rate. Yaprak et al. (29) and Liao et al. (30) proved the feasibility of radiotherapy for glioblastoma. In our study, the prognosis of patients with beam radiation therapy was better than with non-beam radiation therapy (including radioactive implants, brachytherapy, radioisotopes, etc.) or without radiotherapy. In addition, the results of our study indicate that patients with chemotherapy have a significantly higher survival rate than those without chemotherapy. This may be an important role played by temozolomide, the first-line chemotherapy drug for glioblastoma. Temozolomide's introduction significantly improved the prognosis of patients with glioblastoma. The Stupp protocol, proposed in 2005, is the standard of treatment for glioblastoma. It includes radiotherapy, concomitant and adjuvant chemotherapy with temozolomide (28). This kind of treatment significantly improved survival. Perry et al. (31) randomly divided 562 newly diagnosed glioblastoma patients over the age of 65 into two groups, one receiving only short-term radiation therapy and the other receiving short-term radiation therapy plus adjuvant temozolomide. The results showed that the median overall survival of patients who received radiotherapy with temozolomide was longer than those who received chemotherapy alone (5.3 months vs. 3.9 months; P < 0.001).
The impact of tumor laterality on the prognosis of patients has not been fully investigated so far. Daniel et al. retrospectively analyzed 235 cases of patients with glioblastoma on surgical outcome, which were grouped for left—and right—sided GBM (32). The results showed that KPS (Karnofsky Performance Status) decreased significantly and PFS (progression-free survival) was shorter in patients with left hemisphere tumors, but their OS was not significantly different from those in patients with right hemisphere tumors. In our study, patients whose tumor originated at paired site (e.g., left or right hemisphere) had a better prognosis than those whose tumor originated at non-paired site. And patients whose tumors originated at the left had a lower risk than those whose tumors that originated at the right. Tumor laterality may be a noteworthy prognostic factor because different regions of the brain perform different functions, and neurologists may use conservative therapy to preserve some of the patient's functions for need.
Based on the above results, we propose the following suggestions for the treatment of patients with glioblastoma. Pay attention to the age of the patient, especially those aged 65 or older, because elderly people have a worse prognosis. Radiotherapy (especially beam radiotherapy) and chemotherapy should be used, as they can significantly improve the prognosis of patients with glioblastoma. Increase attention to tumor laterality, it may also be a potential prognostic factor.
However, our study has some limitations. Firstly, the SEER database does not include information about tumor markers, such as MGMT (O (6)-methylguanine-DNA methyltransferase) promoter methylation (33) and IDH (isocitrate dehydrogenase) mutation (34), and the two markers have strong prognostic value in patients with glioblastoma. Secondly, the SEER database lacks records of neurological interventions, so this study was not discussed. Thirdly, the study objects of SEER, the American clinical database of tumors, are predominantly white people and black people but few Asians. That partly limited the application of our nomogram in Asian patients. Fourthly, as a retrospective study, both the training group and the verification group may be affected by selection bias.
Conclusion
In conclusion, nomogram integrates easily available factors and serves as an easy-to-use tool to assist patients with glioblastoma in risk assessment and clinical decision-making. The nomogram proposed in this study may objectively and accurately predict 1-, 3, and 5-year OS of patients with glioblastoma. Additional studies are needed to determine whether it is appropriate for the other patient group.
Data Availability Statement
Publicly available datasets were analyzed in this study. This data can be found here: https://seer.cancer.gov/.
Author Contributions
HLi and XZ performed the statistical analyses. XZ checked the statistical accuracy as an expert in statistics. HLi performed the literature search and wrote the first draft of the manuscript. HLi, LH, YH, HLu, and XZ revised and edited the final version of the manuscript. All authors read and approved the final manuscript.
Funding
This work was supported partly by National Natural Science Foundation of China (81541153), Guangdong Science and Technology Department (2016A050503046 and 2015A050502048) and The Public Service Platform of South China Sea for R&D Marine Biomedicine Resources (GDMUK201808), and Southern Marine Science and Engineering Guangdong Laboratory Zhanjiang (ZJW-2019-07).
Conflict of Interest
The authors declare that the research was conducted in the absence of any commercial or financial relationships that could be construed as a potential conflict of interest.
References
1. Omuro A, DeAngelis LM. Glioblastoma and other malignant gliomas: a clinical review. JAMA. (2013) 310:1842–50. doi: 10.1001/jama.2013.280319
2. Roviello G, Petrioli R, Cerase A, Marsili S, Miracco C, Rubino G, et al. A husband and a wife with simultaneous presentation of glioblastoma multiforme: a case report. Case Rep Oncol. (2013) 6:538–43. doi: 10.1159/000356098
3. Wirsching HG, Galanis E, Weller M. Glioblastoma. Handb Clin Neurol. (2016) 134:381–97. doi: 10.1016/B978-0-12-802997-8.00023-2
4. Liu YC, Lee IC, Chen PY. Biomimetic brain tumor niche regulates glioblastoma cells towards a cancer stem cell phenotype. J Neurooncol. (2018) 137:511–22. doi: 10.1007/s11060-018-2763-2
5. Sherriff J, Tamangani J, Senthil L, Cruickshank G, Spooner D, Jones B, et al. Patterns of relapse in glioblastoma multiforme following concomitant chemoradiotherapy with temozolomide. Br J Radiol. (2013) 86:20120414. doi: 10.1259/bjr.20120414
6. Brandes AA, Tosoni A, Franceschi E, Sotti G, Frezza G, Amista P, et al. Recurrence pattern after temozolomide concomitant with and adjuvant to radiotherapy in newly diagnosed patients with glioblastoma: correlation With MGMT promoter methylation status. J Clin Oncol. (2009) 27:1275–9. doi: 10.1200/JCO.2008.19.4969
7. Cronin KA, Ries LA, Edwards BK. The Surveillance, Epidemiology, and End Results (SEER) program of the National Cancer Institute. Cancer. (2014) 120 (Suppl. 23):3755–7. doi: 10.1002/cncr.29049
8. Liang W, Zhang L, Jiang G, Wang Q, Liu L, Liu D, et al. Development and validation of a nomogram for predicting survival in patients with resected non-small-cell lung cancer. J Clin Oncol. (2015) 33:861–9. doi: 10.1200/JCO.2014.56.6661
9. Balachandran VP, Gonen M, Smith JJ, DeMatteo RP. Nomograms in oncology: more than meets the eye. Lancet Oncol. (2015) 16:e173–80. doi: 10.1016/S1470-2045(14)71116-7
10. Liang B, Yu H, Huang L, Luo H, Zhu X. A prognostic model guides surgical resection in cervical squamous cell carcinoma. Transl Cancer Res. (2020) 9:1711–31. doi: 10.21037/tcr.2020.02.71
11. Huitzil-Melendez FD, Capanu M, O'Reilly EM, Duffy A, Gansukh B, Saltz LL, et al. Advanced hepatocellular carcinoma: which staging systems best predict prognosis? J Clin Oncol. (2019) 28:2889–95. doi: 10.1200/JCO.2009.25.9895
12. Sim J, Teece L, Dennis MS, Roffe C, Team SOSS. Validation and recalibration of two multivariable prognostic models for survival and independence in acute stroke. PLoS One. (2016) 11:e0153527. doi: 10.1371/journal.pone.0153527
13. Alexander BM, Cloughesy TF. Adult glioblastoma. J Clin Oncol. (2017) 35:2402–9. doi: 10.1200/JCO.2017.73.0119
15. Wang Y, Li J, Xia Y, Gong R, Wang K, Yan Z, et al. Prognostic nomogram for intrahepatic cholangiocarcinoma after partial hepatectomy. J Clin Oncol. (2013) 31:1188–95. doi: 10.1200/JCO.2012.41.5984
16. Zhu J, Zhu X, Tu C, Li YY, Qian KQ, Jiang C, et al. Parity and thyroid cancer risk: a meta-analysis of epidemiological studies. Cancer Med. (2016) 5:739–52. doi: 10.1002/cam4.604
17. Ma K, Dong B, Wang L, Zhao C, Fu Z, Che C, et al. Nomograms for predicting overall survival and cancer-specific survival in patients with surgically resected intrahepatic cholangiocarcinoma. Cancer Manag Res. (2019) 11:6907–29. doi: 10.2147/CMAR.S212149
18. Jin C, Cao J, Cai Y, Wang L, Liu K, Shen W, et al. A nomogram for predicting the risk of invasive pulmonary adenocarcinoma for patients with solitary peripheral subsolid nodules. J Thorac Cardiovasc Surg. (2017) 153:462–9.e1. doi: 10.1016/j.jtcvs.2016.10.019
19. Feng Y, Li Y, Cai S, Peng J. Immunological nomograms predicting prognosis and guiding adjuvant chemotherapy in stage II colorectal cancer. Cancer Manag Res. (2019) 11:7279–94. doi: 10.2147/CMAR.S212094
20. Ye G, Wang L, Hu Z, Liang J, Bian Y, Zhan C, et al. Risk and prognostic nomograms for hepatocellular carcinoma with newly-diagnosed pulmonary metastasis using SEER data. PeerJ. (2019) 7:e7496. doi: 10.7717/peerj.7496
21. Ladomersky E, Scholtens DM, Kocherginsky M, Hibler EA, Bartom ET, Otto-Meyer S, et al. The coincidence between increasing age, immunosuppression, and the incidence of patients with glioblastoma. Front Pharmacol. (2019) 10:200. doi: 10.3389/fphar.2019.00200
22. Bartek J Jr, Alattar AA, Dhawan S, Ma J, Koga T, et al. Receipt of brachytherapy is an independent predictor of survival in glioblastoma in the surveillance, epidemiology, and end results database. J Neurooncol. (2019) 145:75–83. doi: 10.1007/s11060-019-03268-y
23. Yancik R, Wesley MN, Ries LA, Havlik RJ, Edwards BK, Yates JW. Effect of age and comorbidity in postmenopausal breast cancer patients aged 55 years and older. JAMA. (2001) 285:885–92. doi: 10.1001/jama.285.7.885
24. Liang X, Li D, Leng S, Zhu X. RNA-based pharmacotherapy for tumors: From bench to clinic and back. Biomed Pharmacother. (2020) 125:109997. doi: 10.1016/j.biopha.2020.109997
25. Zhu X, Luo H, Xu Y. Transcriptome analysis reveals an important candidate gene involved in both nodal metastasis and prognosis in lung adenocarcinoma. Cell Biosci. (2019) 9:92. doi: 10.1186/s13578-019-0356-1
26. Zhu X, Lin MCM, Fan W, Tian L, Wang J, Ng SS, et al. An intronic polymorphism in GRP78 improves chemotherapeutic prediction in non-small cell lung cancer. Chest. (2012) 141:1466–72. doi: 10.1378/chest.11-0469
27. Stummer W, Pichlmeier U, Meinel T, Wiestler OD, Zanella F, Reulen HJ, et al. Fluorescence-guided surgery with 5-aminolevulinic acid for resection of malignant glioma: a randomised controlled multicentre phase III trial. Lancet Oncol. (2006) 7:392–401. doi: 10.1016/S1470-2045(06)70665-9
28. Stupp R, Mason WP, van den Bent MJ, Weller M, Fisher B, Taphoorn MJ, et al. Radiotherapy plus concomitant and adjuvant temozolomide for glioblastoma. N Engl J Med. (2005) 352:987–96. doi: 10.1056/NEJMoa043330
29. Yaprak G, Isik N, Gemici C, Pekyurek M, Ceylaner Bicakci B, Demircioglu F, et al. Stereotactic radiotherapy in recurrent glioblastoma: a valid salvage treatment option. Stereotact Funct Neurosurg. (2020) 98:167–75. doi: 10.1159/000505706
30. Liao G, Zhao Z, Yang H, Li X. Efficacy and safety of hypofractionated radiotherapy for the treatment of newly diagnosed glioblastoma multiforme: a systematic review and meta-analysis. Front Oncol. (2019) 9:1017. doi: 10.3389/fonc.2019.01017
31. Perry JR, Laperriere N, O'Callaghan CJ, Brandes AA, Menten J, Phillips C, et al. Short-course radiation plus temozolomide in elderly patients with glioblastoma. N Engl J Med. (2017) 376:1027–37. doi: 10.1056/NEJMoa1611977
32. Coluccia D, Roth T, Marbacher S, Fandino J. Impact of laterality on surgical outcome of glioblastoma patients: a retrospective single-center study. World Neurosurg. 114:e121–8. (2018) doi: 10.1016/j.wneu.2018.02.084
33. Jovanovic N, Mitrovic T, Cvetkovic VJ, Tosic S, Vitorovic J, Stamenkovic S, et al. The impact of MGMT promoter methylation and temozolomide treatment in serbian patients with primary glioblastoma. Medicina (Kaunas). (2019) 55:34. doi: 10.3390/medicina55020034
Keywords: glioblastoma, nomogram, prognostic factors, predictor, overall survival
Citation: Li H, He Y, Huang L, Luo H and Zhu X (2020) The Nomogram Model Predicting Overall Survival and Guiding Clinical Decision in Patients With Glioblastoma Based on the SEER Database. Front. Oncol. 10:1051. doi: 10.3389/fonc.2020.01051
Received: 10 March 2020; Accepted: 27 May 2020;
Published: 26 June 2020.
Edited by:
André O. von Bueren, Hôpitaux Universitaires de Genève, SwitzerlandReviewed by:
Klaus Pietschmann, Klinikum Chemnitz gGmbH, GermanyCarl Friedrich Classen, University Hospital Rostock, Germany
Copyright © 2020 Li, He, Huang, Luo and Zhu. This is an open-access article distributed under the terms of the Creative Commons Attribution License (CC BY). The use, distribution or reproduction in other forums is permitted, provided the original author(s) and the copyright owner(s) are credited and that the original publication in this journal is cited, in accordance with accepted academic practice. No use, distribution or reproduction is permitted which does not comply with these terms.
*Correspondence: Lianfang Huang, gdyhlf168@163.com; Hui Luo, luohui@gdmu.edu.cn; Xiao Zhu, xzhu@gdmu.edu.cn