- 1School of Graduate Studies, CES University, Medellín, Colombia
- 2Basic Science Research Group, School of Medicine, CES University, Medellín, Colombia
- 3Associated Professor Department of Pathology, University of Antioquia, Medellín, Colombia
- 4Colombian Institute of Tropical Medicine (ICMT), Sabaneta, Colombia
- 5Department of Hepatobiliary and Pancreatic Surgery, CES Clinic, Medellín, Colombia
Purpose: To analyze human and bacteria proteomic profiles in bile, exposed to a tumor vs. non-tumor microenvironment, in order to identify differences between these conditions, which may contribute to a better understanding of pancreatic carcinogenesis.
Patients and Methods: Using liquid chromatography and mass spectrometry, human and bacterial proteomic profiles of a total of 20 bile samples (7 from gallstone (GS) patients, and 13 from pancreatic head ductal adenocarcinoma (PDAC) patients) that were collected during surgery and taken directly from the gallbladder, were compared. g:Profiler and KEGG (Kyoto Encyclopedia of Genes and Genomes) Mapper Reconstruct Pathway were used as the main comparative platform focusing on over-represented biological pathways among human proteins and interaction pathways among bacterial proteins.
Results: Three bacterial infection pathways were over-represented in the human PDAC group of proteins. IL-8 is the only human protein that coincides in the three pathways and this protein is only present in the PDAC group. Quantitative and qualitative differences in bacterial proteins suggest a dysbiotic microenvironment in the PDAC group, supported by significant participation of antibiotic biosynthesis enzymes. Prokaryotes interaction signaling pathways highlight the presence of zeatin in the GS group and surfactin in the PDAC group, the former in the metabolism of terpenoids and polyketides, and the latter in both metabolisms of terpenoids, polyketides and quorum sensing. Based on our findings, we propose a bacterial-induced carcinogenesis model for the biliary tract.
Conclusion: To the best of our knowledge this is the first study with the aim of comparing human and bacterial bile proteins in a tumor vs. non-tumor microenvironment. We proposed a new carcinogenesis model for the biliary tract based on bile metaproteomic findings. Our results suggest that bacteria may be key players in biliary tract carcinogenesis, in a long-lasting dysbiotic and epithelially harmful microenvironment, in which specific bacterial species' biofilm formation is of utmost importance. Our finding should be further explored in future using in vitro and in vivo investigations.
Introduction
Pancreatic ductal adenocarcinoma (PDAC) is a malignant and highly lethal neoplasm of unknown etiology and is usually diagnosed at advanced stages (1). The currently available surgical interventions and chemotherapeutic regimes are unable to provide the desired impact on disease outcomes, and there is a clear, dismal prognosis, as 70–80% of patients will succumb to this disease during the first 2 years post-diagnosis (2). PDAC is the fourth leading cause of cancer-related deaths worldwide (3) and is expected to become the second leading cause of cancer-related deaths by 2025, due both to the improved outcome of other malignancies, and on the stagnation in outcome improvement for PDAC over the past 30 years (4–6). Modifiable and non-modifiable risk factors for PDAC have an unconvincing molecular association with the disease. Modifiable factors seem to distribute haphazardly around the world, and the classic ones, such as tobacco, diabetes, gallstones (GS) and alcohol intake, are absent in a significant proportion of patients (7, 8). The development of interventions that successfully reduce the incidence of this lethal malignancy and improve its outcome is limited by the scarce knowledge of the molecular factors that may play a role in the complex process of PDAC carcinogenesis (9). Hence, any effort to better understand PDAC carcinogenesis, or to unravel novel therapies, may be the starting point in driving future clinical interventions.
Bacteria have been associated with benign and malignant disease, and bacterial carcinogenesis is a process still being characterized in detail. The knowledge from such study may be the starting point to drive clinical interventions focused on cancer prevention. The carcinogenesis associated with viruses is based on the integration of the viral genome into the host DNA (i.e., Human Papilloma Virus, Epstein-Barr) and has been extensively studied and characterized (10). Conversely, bacterial carcinogenesis is a phenomenon thought to be the result of epithelial cells' chronic exposure to a pro-inflammatory milieu exacerbated by bacteria (11, 12). However, this pro-inflammatory, physiopathological mechanism cannot explain convincingly by itself the development of carcinomas in the gastrointestinal and biliary tract, as inflammatory phenomena regularly occur throughout the human lifespan, and just a few human beings develop malignant neoplasms.
The biliary tract including intra-pancreatic bile ducts, is a semi-closed duct system possessing its own microbiota (13–15), lined by cholangiocytes, and in constant contact with bile. Cholangiocytes or cholangiocyte like cells are the proposed cell of origin for a range of biliary tract carcinomas, also named cholangiocarcinoma, in gallbladder, and intra or extrahepatic bile ducts (16). PDAC derives from ductal cholangiocytes or transdifferentiated acinar-to-ductal cholangiocytes (17, 18) covering intra-pancreatic bile ducts (ductal carcinoma), so from the histopathological point of view PDAC and biliary tract carcinomas are not very different (19). In the case of PDAC, local microbiota may have effects on oncogenesis (20) and long term survival (21), but most of the surveys associating PDAC and bacteria demonstrate spurious associations due to inconsistent isolation of specific bacterial species and the lack of a molecular basis for bacteria-induced carcinogenesis (22, 23). Being part of the biliary tract microenvironment, bacteria must contribute to bile protein pool composition in a similar way to cholangiocytes. As cholangiocytes and bacteria are in permanent contact with bile, we hypothesize that bile-associated protein changes could reflect bile duct system alterations in the microenvironment sufficient to transform benign epithelial cells into a malignant phenotype.
Bile is stored and concentrated in the gallbladder, which is a clean reservoir where this biological fluid can be extracted for protein analysis (24). In research, bile samples are typically taken from the distal portion of the biliary tract during endoscopic interventions, such as endoscopic retrograde cholangiopancreatography (ERCP) (25). However, the inflammatory process associated with biliary obstruction in most PDAC patients may alter bile protein composition in the distal portion of the biliary tract and limit the finding of meaningful biological information. The lack of meaningful biological findings hinders the development of a specific model of carcinogenesis for the biliary tract that takes into account its unique physiological conditions, and the interplay of human and bacterial proteins.
We analyzed samples of human bile taken directly from the gallbladder, and not by ERCP, exposed to a pancreatic tumor vs. non-tumor microenvironment. The aim of the study, once samples were analyzed by mass spectrometry, was to find meaningful biological information through pathway inference analysis of the identified human and bacterial proteins. Biological pathway analysis was initially performed using the g:Profiler platform to compare and generate a complete panorama of the gene-protein sets being analyzed, including over-represented KEGG biological pathways (26). Then, we directly analyzed each protein set using the KEGG Mapper Reconstruct Pathway module (27), focusing on over-represented pathways in g:Profiler for human proteins, and prokaryote interaction pathways for bacterial proteins. KEGG has become a world reference database for assisting biological interpretations of molecular data sets. Currently, biological pathway analyses are one of the most reliable strategies for mechanistic insights into omics data, since the kind of evidence that supports the statistical modeling is always experimental and manually curated (28). Thus, in this study, using a paradigm shifting metaproteomic approach, we aimed to unravel novel and meaningful biological information that may contribute to a better understanding of PDAC bacteria-induced carcinogenesis, proposing a new carcinogenesis model for the biliary tract.
Materials and Methods
Ethics and Sample Acquisition
The Institutional Human Ethics Committee at CES University and Clinic approved this study, and patients must give informed consent. Samples were de-identified before performing proteomic analysis. A surgical pathologist collected a total of 20 gallbladder bile samples; seven from patients with gallstones (GS), and 13 from patients with PDAC arising from the head of the pancreas. All patients were Colombians, and residents of Medellín (Colombia). For GS patients, bile was obtained in the operating room immediately after laparoscopic extraction of the gallbladder, puncturing the gallbladder fundus with a syringe, and aspirating at least 5 mL of bile. For PDAC patients, bile was similarly collected, by aspirating bile with a syringe from the gallbladder pancreatoduodenectomy specimens were sent to the pathology lab for a cryosection margin report. Immediately after collection, bile samples were transported on ice, aliquoted, and stored at −80°C until further analysis. Patients with a clinical history of previous malignant neoplasms, chemotherapy, prior biliary tract surgery or biliary stent placement, HIV, pregnancy, chronic pancreatitis, choledocholithiasis, cystic fibrosis, hepatolithiasis, primary biliary cholangitis, liver cirrhosis, primary sclerosing cholangitis, or acute cholecystitis were excluded from this study.
Protein Extraction
Bile samples were thawed at room temperature and processed as previously described with slight modifications (29). Briefly, 1 mL of bile was centrifuged for 10 min at 4°C and 3,000 rpm, and 1 mL of TRI reagent and 1 mL of chloroform were added. The mix was incubated for 5 min at room temperature (20–25°C) and centrifuged for 15 min at 4°C and 12.000 xg to separate proteins. Avoiding the central lipid layer, remaining tube contents (supernatant + pellet) were transferred to a new tube. Then, 1,200 μL of acetone was added, mixed, incubated for 4 h, and centrifuged for 15 min at 4°C at 12,000 xg. Acetone was discarded, and the tubes were dried at room temperature, after which 200 μL of reconstituting buffer was added to the pellet, and the solution dried and lyophilized.
Proteomic Analyses
Proteomic analysis was performed by Creative Proteomics (Ramsey Road, Shirley, NY 11967, USA), briefly, the techniques used are described as follows:
Sample Preparation for Proteomic Analysis
Total proteins were precipitated from the protein solution using methanol and chloroform. Approximately 10 μg of total protein was dissolved in 6 M urea aqueous solution and was denatured with 10 mM DL-dithiothreitol, incubated at 56°C for 1 h, followed by alkylation with 50 mM iodoacetamide, and incubated for 60 min at room temperature, protected from light. Next, 500 mM ammonium bicarbonate (ABC) was added to the solution to make a final concentration of 50 mM ABC with a pH of 7.8. Promega Trypsin was added to the protein solution for digestion at 37°C for 15 h. The generated peptides were further purified with the C18 SPE column (Thermo Scientific) to remove salt. Samples were dried in a vacufuge and stored at −20°C until use.
Nano Liquid Chromatography
An Easy-nLC1000 (ThermoFisher Scientific, USA) coupled to a 100 μm × 10 cm in-house made column packed with a reversed-phase ReproSil-Pur C18-AQ resin (3 μm, 120 Å, Dr. Maisch GmbH, Germany) was used. A sample volume of 5 μL was loaded, with a total flow rate of 600 nL/min, and a mobile phase of A: 0.1% formic acid in water; and B: 0.1% formic acid in acetonitrile. The analytical separation was run using a gradient: from 6 to 9% B for 15 min, from 9 to 14% B for 20 min, from 14 to 30% B for 60 min, from 30 to 40% B for 15 min and from 40 to 95% B for 3 min, eluting with 95% B for 7 min.
Mass Spectrometry and Data Analysis
An Orbitrap Q Exactive™ mass spectrometer (Thermo Fisher Scientific, USA) set on a spray voltage of 2.2 kV and a capillary temperature of 270°C was used. Mass spectrometry resolution was set to 70,000 at 400 m/z and precursor m/z range: between 300.0 and 1800.0. The production scan range starts from m/z 100, activated by collision-induced dissociation (CID), and an isolation width of 3.00. The raw files were analyzed and searched against the human protein database from Uniprot using Maxquant (1.5.6.5). The parameters were set as follows: the protein modifications were carbamidomethylation (C) (fixed), oxidation (M) (variable); the enzyme specificity was set to trypsin; the maximum missed cleavages was set to 2; the precursor ion mass tolerance was set to 10 ppm, and MS/MS tolerance was 0.6 Da.
Human and Bacteria Peptide-Protein List Selection for Analysis
Peptide-protein analysis was performed at ICMT-CES University. Contaminants, albumin, hemoglobin related peptides, and peptides with zero intensity were eliminated from the full human and bacteria list of peptides-proteins. The identifiers of protein were standardized, missing gene names were manually completed, and protein taxonomy was verified.
Then, the full list of shared proteins was adapted to meet the requirements of the Prostar platform online version 1.18.1 (30), seeking for differentially abundant human and bacterial proteins among groups (GS vs. PDAC). The intensity values were normalized with the mean centering method without including variance reduction. Partially observed values were imputed using the SLSA (Structured Least Squares Adaptive) method. The hypothesis test was performed using the Student's t-test, considering a logarithmic change of 2.5 and adjusting the false discovery rate to 0.42% (p-value = 0.00316). The biological validity of imputing non-existent values for non-observed proteins, in order to compare the exclusive groups of proteins, was explored. However we chose to perform the analysis based only on observed values in the two groups, GS and PDAC (shared proteins).
For further qualitative analysis, all the human protein lists of the total, exclusive and differentially abundant proteins from GS and PDAC patients (Figure 1) were included in the retrieve ID/mapping module of the Universal Protein consortium resource (Uniprot http://www.uniprot.org/, UniProt release 2019_10). Then, in order to provide mechanistic insights into the biologically integrated function, Uniprot-standardized human protein lists of entries for each group were analyzed in the g:Profiler web page (https://biit.cs.ut.ee/gprofiler/gost) (26). g:Profiler allows a multi-query approach, which performs an over-representative functional analysis of multiple protein-gene lists, comparing proteins among groups. Default options were maintained in g:Profiler, adding no electronic gene ontology annotations, and Bonferroni correction for multiple test adjustments. Significant, adjusted, over-represented pathways (p-values < 0.01), were used for further analysis in the KEGG Mapper Reconstruct Pathway. KEGG identifiers were obtained from the Uniprot FASTA file of the total, exclusive, and differentially abundant protein list, through BlastKoala (KEGG Orthology and Links Annotation version 2.2 https://www.kegg.jp/blastkoala/) (31). KEGG Mapper Reconstruct Pathway allows visualization and comparison of proteins in signaling pathways to identify qualitative and quantitative differences without coupled statistical analysis. (https://www.genome.jp/kegg/tool/map_pathway.html) (27). On the other hand, useful drugs were explored using the functional database DrugBank through WebGestalt (WEB-based GEne SeT AnaLysis Toolkit updated on 01/14/2019 http://www.webgestalt.org/) (32), by performing an over-representation analysis (ORA), using the database Drugbank and setting the false discovery rate at <0.01 (32) with Bonferroni correction for multiple test adjustments.
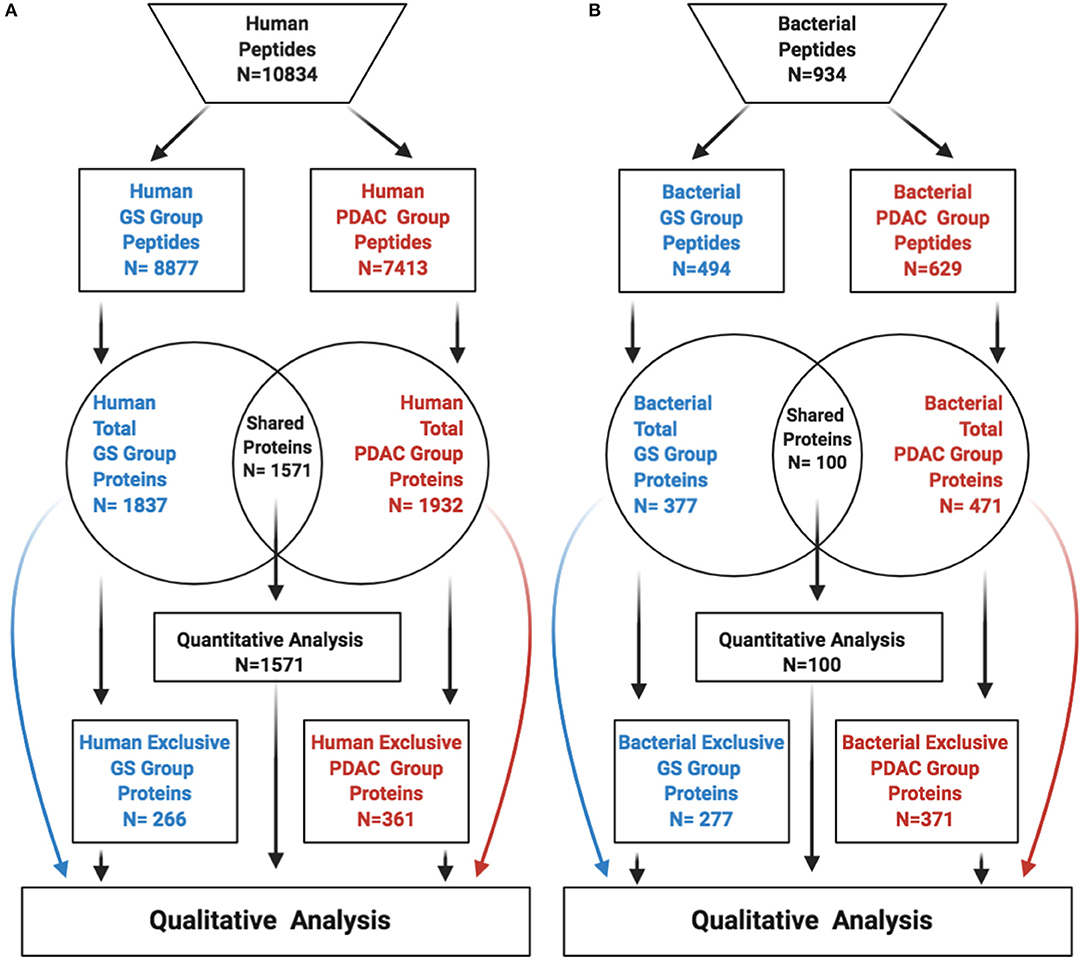
Figure 1. Identified peptides-proteins per group and origin. The figure depicts peptides-proteins identified from human (A), and bacteria (B). GS, gallstones; PDAC, pancreatic ductal adenocarcinoma.
The biological context was analyzed as a whole for the total protein groups by correlating findings with the specific proteins identified for each condition. Many biological pathways were enriched over the Bonferroni p-adjusted value threshold in the total protein groups, but just three of them were related to bacterial infection (Figure 2).
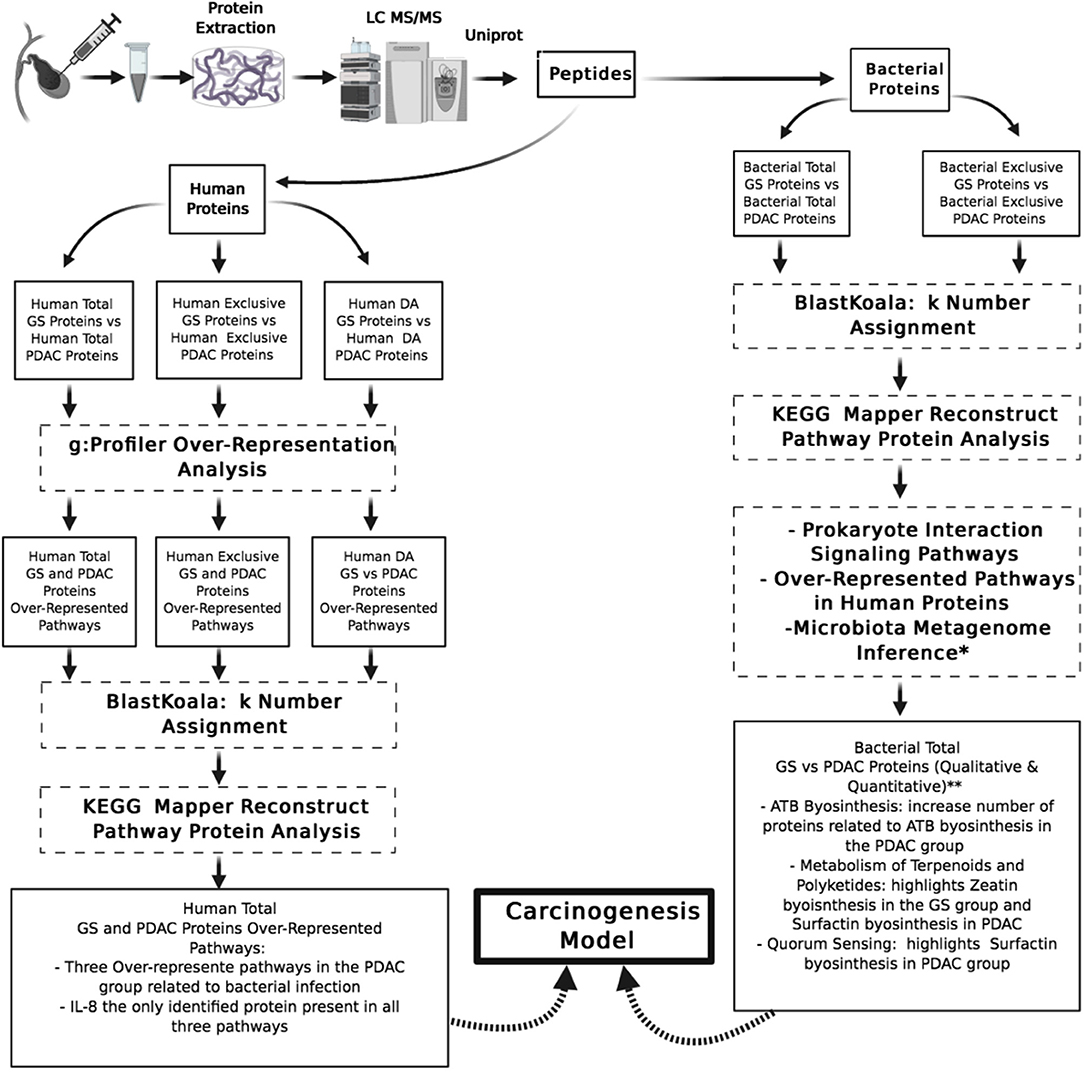
Figure 2. Pipeline of proteins data sets analysis and summary or relevant findings. GS, gallstones; PDAC, pancreatic ductal adenocarcinoma; DA, differentially abundant; KEGG, Kyoto encyclopedia of genes and genomes; IL-8, interleukin 8; ATB, antibiotics. *Small sample microbiota metagenome inference analysis (unpublished results). **Not statistical analysis associated.
For bacterial proteins, the same protocol for contaminant elimination, quality control, and differential abundance analysis was performed as for human proteins. We did not use g:Profiler for bacterial protein analysis because this platform is not conceived for multi-species analysis. The bacterial protein lists of total and exclusive proteins from GS and PDAC patients were also included in the retrieve ID/mapping module of the Universal Protein consortium resource. KEGG identifiers were obtained using BlastKoala from the Uniprot FASTA files, and we focused our attention on prokaryote interaction signaling pathways during the analysis in KEGG Mapper Reconstruct Pathway (33).
Results
A total of 20 bile samples extracted from gallbladders were analyzed, seven of which were taken from patients with GS (mean age of 48 years) (Table 1), and 13 from patients with PDAC (mean age of 56 years). All the patients were residents in Medellín and none of the patients presented clinical or histopathological signs of acute inflammation.
After excluding peptides that were unassociated with any known proteins, a total of 10,834 human peptides were identified with a mean of 542 peptides per sample, 8,877 peptides in the GS group and 7,413 in the PDAC group. Peptides were associated with a total of 2,198 human proteins, 1,837 proteins in the GS group, and 1,932 proteins in the PDAC group. Upon comparison, a total of 1,571 proteins were common to both groups, while 266 proteins were exclusively found in the GS group, and 361 proteins in the PDAC group (Figure 1A). For bacteria, we identified a total of 934 peptides with a mean of 46 peptides per sample, 494 in the GS group and 629 in the PDAC group. Those peptides were associated with a total of 748 bacterial proteins, 377 proteins in the GS group and 471 in the PDAC group. We found 100 proteins shared among the two groups, with 277 exclusive proteins remaining in the GS group and 371 in the PDAC group (Figure 1B). Quantitative differential abundance analysis using Prostar revealed among the shared proteins within the human and bacteria groups, 123 differentially abundant human proteins, 81 in the GS group and 42 in the PDAC group, and no differentially abundant bacterial proteins.
Human Protein Over-Representation Analysis
The g:Profiler platform was used for the over-representation analysis in KEGG signaling pathways. Analyzing the total list of proteins, the platform identified from the 1,837 proteins in the GS group 1,832 (99.7%) and from 1,932 in the PDAC group 1,929 (99.8%). Regarding exclusive and differentially abundant proteins, the platform identified 100% of proteins in the GS and PDAC groups. In the total protein lists, we found five over-represented pathways in the GS group and seven in the PDAC group (Table 2), and in the exclusive protein lists, we identified one over-represented pathway in each group: phagosome in the GS group and metabolic pathways in the PDAC group. The analysis of the differentially abundant list of proteins revealed just one over-represented pathway in the GS group: vasopressin-regulated water reabsorption.
The over-represented pathways were analyzed in KEGG Mapper Reconstruct Pathway focusing our attention in the three g:Profiler over-represented pathways in the PDAC total protein group related to the bacterial infections Shigellosis, Pertussis, and Legionellosis. Analyzing the list of proteins in these three pathways (Table 3), it is notable that IL-8 (interleukin 8) is the only protein coinciding in the three pathways and present only in the PDAC group. This difference is more remarkable when evaluating the pathways of cytokine-cytokine receptor interaction and cytokines and growth factors, the latter in BRITE (Functional hierarchies of biological entities) tables, finding association in the presence of IL-8 with interleukin 11 (IL-11), CCL15 (Chemokine (C-C motif) ligand 15), CSF1 (Macrophage colony-stimulating factor) and CXCL7 (Chemokine (C-X-C motif) ligand 7) in the PDAC group (Table 4). Considering as an interaction point among prokaryotes and eukaryotes, Toll-like and NOD-like receptor pathways, we analyzed those signaling pathways and IL-8 was also present, and only in the PDAC group. In other KEGG signaling pathways with relevance to carcinogenesis processes such as DNA repair, xenobiotic metabolism, and pathways in cancer and pancreatic cancer, we didn't find differences. In the signaling pathways over-represented in exclusive and differentially abundant proteins, we could not find a clear biological meaning. In the WebGestal platform analysis, there were no relevant results for useful, therapeutic drugs using the Drugbank database.
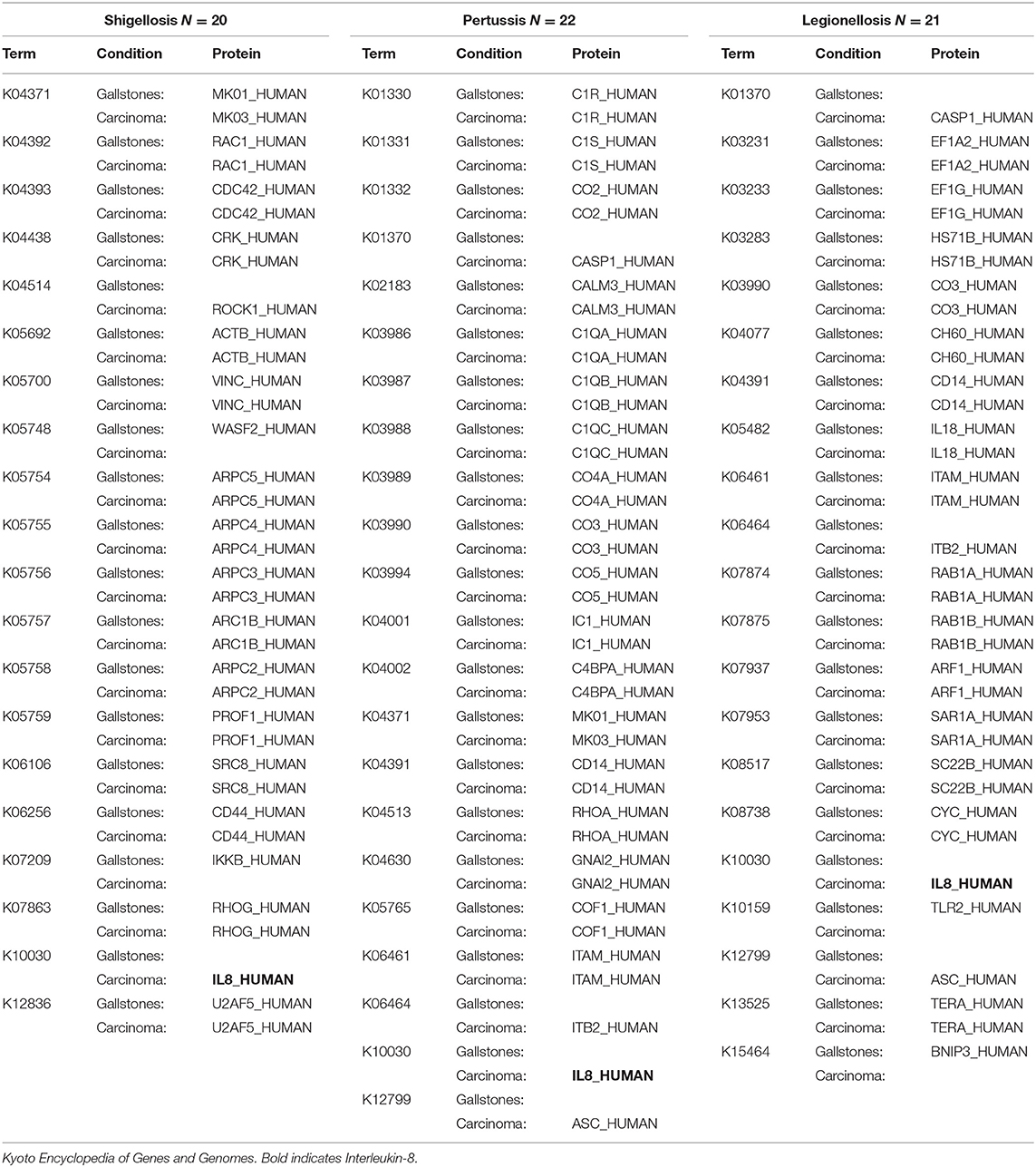
Table 3. Human total proteins per group in KEGG of bacterial infection over-represented signaling pathways.
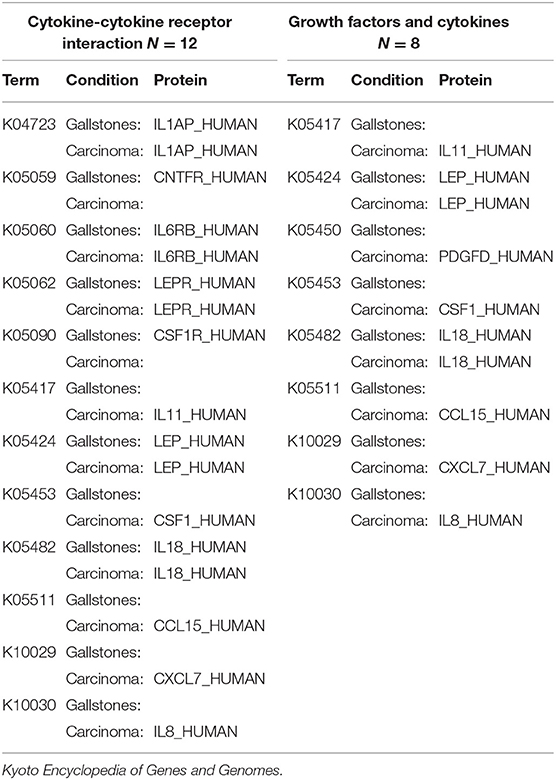
Table 4. Comparison of human total proteins related to cytokine-cytokine receptor interaction and growth factors and cytokines signaling pathways.
Bacterial Protein Analysis
Proportional participation in some taxonomic levels of the imputed protein species is summarized in Table 5. The total protein list was analyzed in KEGG Mapper Reconstruct Pathway focusing on: (1) signaling pathways related to prokaryote interaction, (2) g:Profiler over-represented pathways in human proteins and (3) over-represented pathways in a metagenomic inference analysis (34). The latter analysis was performed from a small microbiota survey within the 20 samples, using bile from the gallbladders of GS patients (N = 3), bile from the gallbladders of PDAC patients (N = 11) and common biliary brush over the tumor from PDAC patients (N = 11) as samples. The results of the analysis show two statistically significant over-represented pathways, pyrimidine deoxyribonucleotide biosynthesis and isoprene biosynthesis (unpublished results).
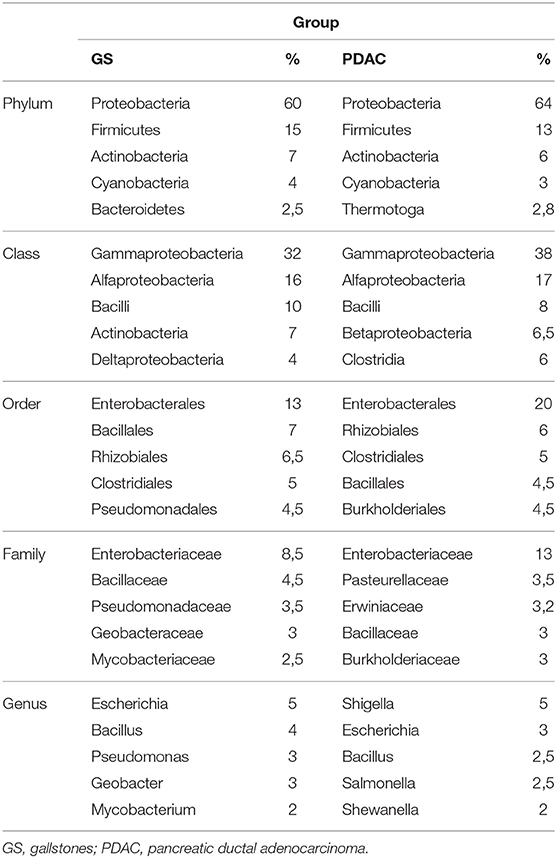
Table 5. Average of the 5 most abundant taxonomic levels per group from identified total bacterial protein.
Upon comparison of the GS and PDAC total protein groups, we found qualitative and quantitative differences regarding quorum sensing, biofilm formation, antibiotic synthesis (biosynthesis of other secondary metabolites) and metabolism of terpenoids and polyketides (Table 6). Regarding metabolism of terpenoids and polyketides, there is a protein involved in zeatin biosynthesis (MIAA_PSECP) that stands out from the other proteins, as it is not present in the PDAC group, is not a protein with an antibiotic function and is specific to that metabolic pathway. Concerning the PDAC group, in the signaling pathway of terpenoid and polyketide metabolism, there is one protein related to surfactin biosynthesis (SRFAB_BACSU), which is also notable, since this protein is also involved in the quorum-sensing signaling pathway. The proteins involved in quorum sensing and biofilm formation show qualitative differences, and the number of proteins present in antibiotic biosynthesis are considerably higher in the PDAC group compared to the GS group. The analysis of bacterial proteins present in the three g:Profiler over-represented human protein signaling pathways related to bacterial infection in the total PDAC group, show no differences in KEGG Mapper Reconstruct Pathway.
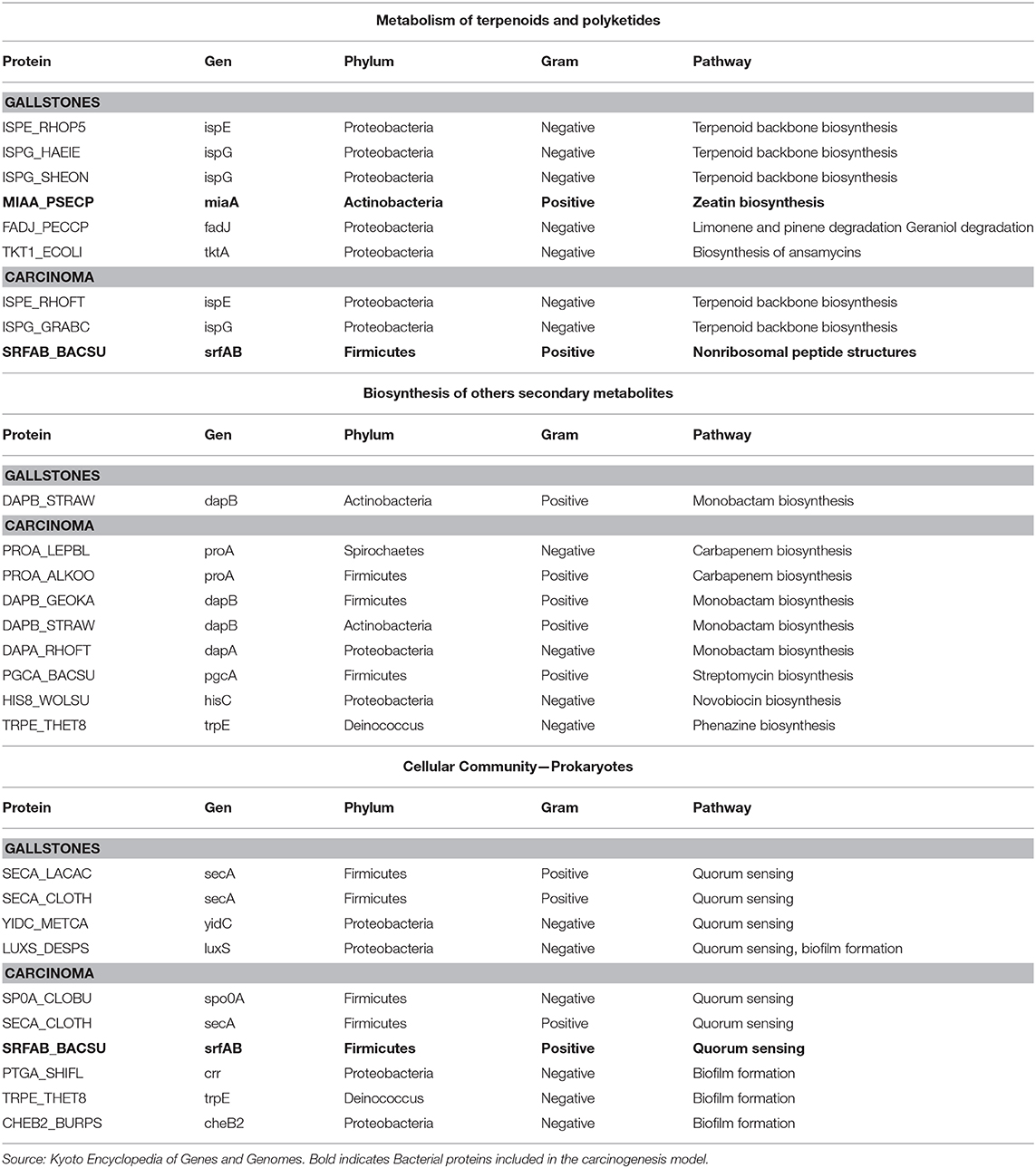
Table 6. Comparison of bacterial list of total proteins in prokaryote interaction signaling pathways and microbiota metagenomic inference over-represented pathways.
Discussion
To the best of our knowledge, this is the first study to compare human and bacterial proteins, using a metaproteomic approach, bile samples exposed to a tumor vs. non-tumor environment in human PDAC and GS patients, respectively. The characterization of a single species protein profile is known as proteomics, while the characterization of a multi-species protein profile is known as metaproteomics (35). The metaproteomic concept has been studied in humans through the characterization of fecal microbiota and the proteins produced by the different local bacterial species, enabling a better comprehension of the local conditions in the gastrointestinal tract (36, 37). In theory, the microenvironment within the biliary tract and the gallbladder will be more resistant to external variation and more accessible for bile retrieval in animal models. For all that, the biliary tract including its reservoir, will be an ideal biological system to evaluate through metaproteomic and microbiota analyses in conjunction, changes related to specific diets, neoplastic conditions, antibiotic use, chemotherapeutic schemas etc.
Finding meaningful biological information from omics' science data sets has been one of the major challenges of science in recent years (38). The relevance of research findings cannot be measured in every biological instance using statistical significance alone, as not all statistically significant results translate into meaningful biological change. Accordingly, in some areas of science, in which we cannot use statistics, or for which we have not developed appropriate tools, we should look for procedural alternatives that at least enable us to explore the real biological value of data sets. In our research, the three g:Profiler over-represented pathways in human proteins show qualitative and quantitative coincidences and differences regarding the presence of certain proteins in the PDAC and GS groups. The detailed analysis of the proteins in each over-represented signaling pathway is the component of the analysis with the greatest importance. Due to the polyfunctionality of bacteria and human proteins, these proteins must be contextualized and analyzed for relevant biological pathways.
IL-8: Carcinogenesis and Prokaryote Interactions
IL-8 was identified as a common protein in the three g:Profiler over-represented signaling pathways in PDAC human total proteins, associated with bacterial infections. IL-8 is a human chemotactic interleukin of the C-X-C family also known as CXCL8, originally discovered in macrophages (39), but also produced by epithelial cells. The effect of IL-8 depends on its interaction with specific membrane receptors coupled to G proteins CXCR1 (C-X-C motif chemokine receptor 1, C-X-C) and CXCR2 (C-X-C motif chemokine receptor 2) (40). Under physiological conditions IL-8 levels are undetectable, increasing in the presence of other pro-inflammatory cytokines like tumor necrosis factor α (TNFα) and interleukin 1β (41, 42). None of the latter two cytokines were identified in the PDAC or GS group, suggesting that alternative pathways can stimulate IL-8 synthesis.
High levels of IL-8 are described as poor outcome predictors in many malignant neoplasms, including PDAC. The cellular endpoint effects induced by the IL-8 CXCR1/CXCR2 axis, in normal epithelial cells, tumor cells or other cells in the tumor microenvironment, promote cellular survival, proliferation, angiogenesis, and a stem cell phenotype (43, 44). Concordantly, high levels of IL-8 in patients with breast, prostate and lung carcinoma, and melanoma are related to aggressive tumor behavior, due to high proliferation rate, local invasion, angiogenesis, and an increase of a stem cell phenotype and metastasis (45). In the special case of PDAC, high levels of IL-8 are also related to aggressive tumor behavior and poor prognosis, with evidence that includes PDAC cell line models (46), high blood levels in PDAC and cholangiocarcinoma patients (47, 48), and over-expression of IL-8 and its receptors in tumor tissue (49) and inflammatory cells infiltrating the tumor (50).
The biological relevance of IL-8 is not limited to neoplasms; there is a special prokaryote behavior linked to the synthesis of this interleukin. Biofilm formation by bacteria such as F. nucleatum and A. naeslundii, and not the planktonic form, stimulates the synthesis of IL-8 by human squamous epithelial cells (51). Supporting the latter concept, several surveys have proved that bacteria biofilm not only stimulates IL-8 synthesis by human squamous epithelial cells, but that stimulation is stronger when the biofilm is formed by multiple bacterial species (52, 53). Furthermore, the similarity of some amino acids in the carboxy-terminal region of IL-8 with cecropins, proteins with antibiotic properties, elicit the analysis of the antibiotic properties of IL-8 through the synthesis of synthetic peptides. These synthetic peptides are thought to be physiologically generated from acidic hydrolysis, and effectively have antibiotic properties which vary according to salt concentration and pH (54).
Some of the PDAC-specific proteins associated with IL-8 are also considered as poor outcome biomarkers in the natural history of malignant neoplasms. High levels of CXCL7 in cholangiocarcinoma tumoral tissue are associated with poor tumor differentiation, local lymph node metastasis, and lymphatic/vascular invasion (55). In renal carcinoma, high levels of CXCL7 are proposed as prognostic factors of chemotherapeutic response (56), and in colon cancer are related to poor survival in patients with liver metastasis (57). Similarly, high levels of CCL17 and IL-11 are associated with poor outcome in malignant neoplasms due to aggressive biological behavior regarding local invasion and metastasis (58–61).
Differences in Prokaryote Interaction Pathways
The metagenomic inference analysis results from the small microbiota group revealed some over-represented pathways. Of special interest is the metabolism of terpenoids and polyketides signaling pathway. This pathway was analyzed using the total list of bacterial proteins, finding qualitative and quantitative differences, among them the presence of zeatin in the GS group and surfactin in the PDAC group. Terpenoids and polyketides are a huge group of substances synthesized by bacteria, fungi, plants, and animals. Zeatin is an isoprenoid derived from adenine with two isoforms, trans and cis, depending on which of the two hydroxyl groups in the lateral chain of isopentenyl is hydroxylated (62). The identified bacterial protein in the GS group participates in the metabolic pathway for cis-zeatin synthesis and is specific to this metabolic pathway. Cis-zeatin is a cytokinin that can be produced by multiple bacterial species (63, 64), with just one published piece of research evaluating its activity in tumor cell lines, proving its anti-tumor potential in leukemia cell lines (65).
The ability to produce surfactin is a property of bacteria from the genus Bacillus, and since the discovery of surfactin in 1968 by Arima, this amphipathic lipopeptide has been found to possess several properties (66). Within these properties are those general to all lipopeptides and antibacterial proteins which act upon Gram-positive and Gram-negative bacteria (67, 68), and other anti-inflammatory (69) and anti-viral effects (70). Of interest in our research, the properties associated with biofilm formation are of utmost importance. Regarding biofilm formation, surfactin has a selective effect, primarily inhibitory, over many bacterial species, though to date there is no clear biological explanation for the selectivity of surfactin for biofilm production/inhibition (71–73).
Besides producing essential compounds for survival, bacteria are able to produce and secrete into the environment low molecular weight compounds called secondary metabolites. Within those secondary metabolites are substances with antibiotic properties that, in a specific microenvironment, confer an advantage upon the bacterium producing the antibiotics, reducing the number of competitors (mainly for nutrient acquisition) (74). Bacterial antibiotic synthesis is a phenomenon influenced by the community and denotes a competitive behavior for survival, and is seemingly species-specific (75). In our analysis, the increased number of identified proteins in the antibiotic synthesis pathways in the PDAC group compared to the GS group is remarkable. Based on that fact, we infer a major competition among species in the PDAC group.
The change in bacteria association, from free-living or planktonic to biofilm formation, relies upon many genetic factors and local conditions (76). Biofilm formation is tightly associated in multiple bacterial species with the increase of c-di-GMP (cyclic diguanylate) intracellular levels, which can also be induced by quorum sensing proteins (77). By means of conventional microbiota analysis, we are unable to determine if the identified bacteria are in a biofilm or not. For this reason, we considered that finding different bacterial proteins among the groups (PDAC and GS) related to quorum sensing and biofilm formation is of biological relevance, as these proteins can be involved in the process of bacteria-induced carcinogenesis. Inflammation is considered a starting point of bacteria associated carcinogenesis. However, this physio-pathological mechanism cannot fully explain the development of carcinomas in the gastrointestinal and biliary tract, as inflammatory states constantly occur throughout the human lifespan, and only a few human beings develop malignant neoplasms in the gastrointestinal system. The bacteria-cancer relationship has been viewed in a reductionist manner as simply a pro-inflammatory milieu initiated by bacteria, in line with the hypothesis of inflammation and cancer proposed by Virchow in 1835 (78). Previous studies have proposed that this pro-inflammatory milieu may be initiated by dysbiosis, which is defined as a change in the normal composition of the microbiota. However, dysbiosis has neither fulfilled the expectations nor provided—to date—a reliable molecular explanation for bacteria-induced carcinogenesis (79).
The Carcinogenesis Model
We hypothesized that there is no such thing as a dysbiotic microbiota, regarding the presence or absence of certain bacterial species. A dysbiotic microbiota is a haphazard composition of bacterial species with products harmful to bacteria and epithelial cells, specific to a particular individual vis-à-vis microbiota-modifying factors. Based on our metaproteomic findings regarding bacterial and human proteins, and its associations, we proposed a biliary tract carcinogenesis model. We are aware of that our proteomic analysis is a snapshot of established PDAC cases, and the propose bacteria-induced carcinogenesis model for the biliary tract is still speculative, not validated, and therefore must be interpreted with caution (Figure 3). The model initiates with unique or multiple dysbiotic factors that promote repeated inflammatory events (80), classically described as stones in the biliary tract, tobacco use, obesity, diabetes mellitus or genetic factors (81). Those promoting factors change the usual biliary tract bacteria composition expected for that individual, shaped according to diet, genetic background, sex, race, age, etc. Promoting factors can create many unusual microbiotas for that individual; though the specific carcinogenic dysbiotic microbiota has a reduced diversity as a sign of competition fostered by highly elevated synthesis of antibiotic products, and qualitative and quantitative differences in bacterial proteins associated with quorum sensing and biofilm formation, such as zeatin and surfactin. High levels of antibiotics maintain the dysbiotic environment, added to the antibiotic effect of surfactin, the latter also selecting, through inhibition, bacteria for biofilm formation. Bacterial species capable of biofilm formation will promote the synthesis of IL-8 by biliary tract epithelial cells. Fragments of IL-8 with antibiotic potential also contribute to maintaining dysbiosis, while the whole protein exerts its pro-neoplastic function of epithelial cellular survival, proliferation, angiogenesis, invasion and stem cell phenotype. The described scenario in conjunction with low levels or absence of zeatin, an anti-neoplastic protein, facilitates the progression of epithelial changes from low-grade dysplasia to adenocarcinoma, through mutation aggregation (82–84). The dysbiotic and harmful epithelial microenvironment needs to continue for a long but unspecified period of time to transform a benign epithelial cell into a malignant one, a period in which the molecular characteristic may be detected.
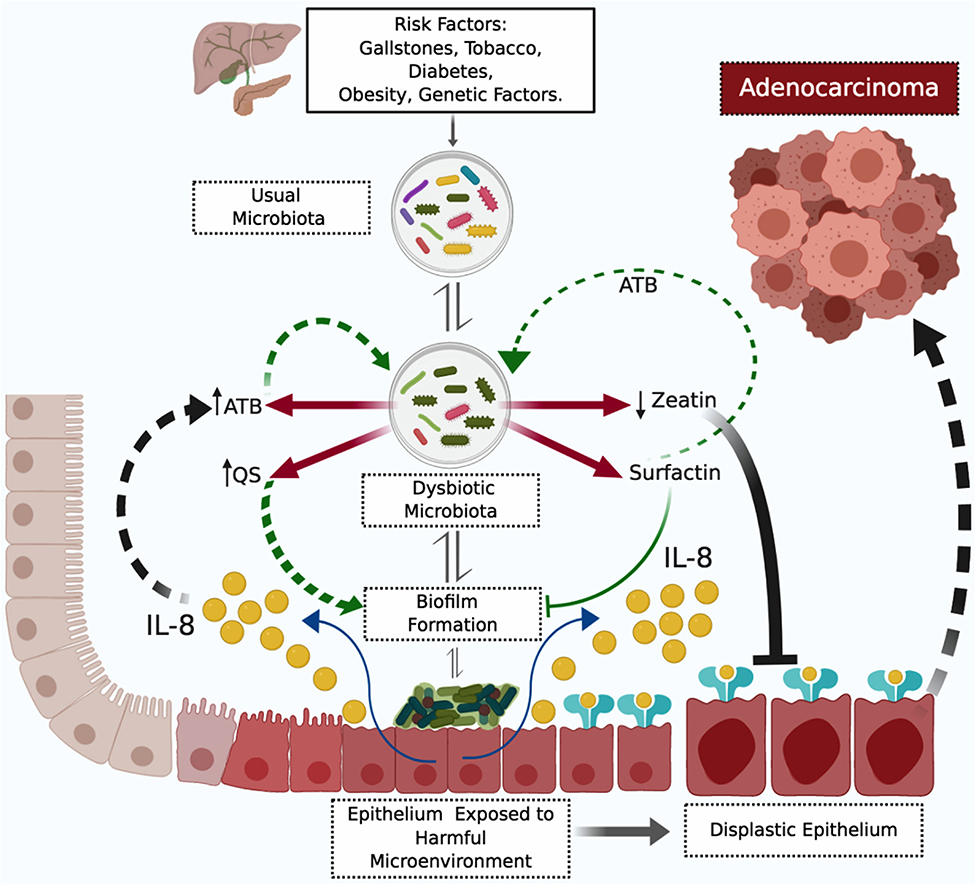
Figure 3. Proposed Model of Biliary Tract Bacterial-Induced Carcinogenesis. The figure depicts a harmful microenvironment originating from prokaryote and eukaryote interaction. The harmful microenvironment initiates with a dysbiotic microbiota product of repetitive inflammatory processes induced by risk factors. The dysbiotic microbiota is specific for low levels of zeatin and high levels of antibiotics (ATB) and surfactin. Surfactin selectively inhibits bacterial biofilm formation—to date—without a molecular explanation for this selectivity. Bacterial biofilm formation stimulates IL-8 (interleukin 8) pro-neoplastic cytokine synthesis by biliary tract epithelial cells. Antibiotics, surfactin, and fragments of IL-8 with antibiotic properties perpetuate the dysbiotic microenvironment. Mutations accumulate in epithelial cells and IL-8 promotes the progression of dysplastic changes to adenocarcinoma, in low zeatin anti-neoplastic protein levels. ATB, antibiotics; IL-8, interleukin 8; QS, quorum sensing proteins.
There are no clear indications for sample size calculations in proteomics research, and the results from a specific protein extraction method, imputation pipeline, and bioinformatic analysis must be validated in further in vitro and in vivo investigations. Microbiota analysis and dysbiosis alone have not answered the question of the bacteria-induced pathology model. Future research may concentrate on improving the throughput of protein identification from complex biological fluids like bile and consider a combined microbiota and metaproteomic approach to analyze bacterial communities and bacterial and human proteins. It is necessary to start thinking of a change in the dysbiosis paradigm, as we hypothesized dysbiosis is not a specific bacterial composition but rather a harmful protein microenvironment that can be created by several “dysbiotic” microbiotas.
Data Availability Statement
The datasets presented in this study can be found in online repositories. The names of the repository/repositories and accession number(s) can be found below: ProteomeXchange Consortium via the PRIDE partner repository with the dataset identifier PXD020151.
Ethics Statement
The studies involving human participants were reviewed and approved by Ethical Committee—Universidad CES. The patients/participants provided their written informed consent to participate in this study.
Author Contributions
AA and NC-C design the study. DD, OP, and AA participated in sample acquisition. MS-J, NC-C, and AA participated in the laboratory procedures, protein analysis, and biological pathways interpretation. AA and NC-C wrote the manuscript. All authors contributed to the article and approved the submitted version.
Funding
This work was supported by Instituto Colombiano de Medicina Tropical and by CES University grant INV.022019.003.
Conflict of Interest
The authors declare that the research was conducted in the absence of any commercial or financial relationships that could be construed as a potential conflict of interest.
References
1. Yadav D, Lowenfels AB. The epidemiology of pancreatitis and pancreatic cancer. Gastroenterology. (2013) 144:1252–61. doi: 10.1053/j.gastro.2013.01.068
2. Ouaïssi M, Giger U, Louis G, Sielezneff I, Farges O, Sastre B. Ductal adenocarcinoma of the pancreatic head: a focus on current diagnostic and surgical concepts. World J Gastroenterol. (2012) 18:3058–69. doi: 10.3748/wjg.v18.i24.3058
3. Zhang Q, Zeng L, Chen Y, Lian G, Qian C, Chen S, et al. Pancreatic cancer epidemiology, detection, and management. Gastroenterol Res Pract. (2016) 2016:8962321. doi: 10.1155/2016/8962321
4. Zhang L, Sanagapalli S, Stoita A. Challenges in diagnosis of pancreatic cancer. World J Gastroenterol. (2018) 24:2047–60. doi: 10.3748/wjg.v24.i19.2047
5. Sener SF, Fremgen A, Menck HR, Winchester DP. Pancreatic cancer: a report of treatment and survival trends for 100,313 patients diagnosed from 1985-1995, using the national cancer database. J Am Coll Surg. (1999) 189:1–7. doi: 10.1016/S1072-7515(99)00075-7
6. Simard EP, Ward EM, Siegel R, Jemal A. Cancers with increasing incidence trends in the united states: 1999 through 2008. CA Cancer J Clin. (2012) 62:118–28. doi: 10.3322/caac.20141
7. Luo G, Zhang Y, Guo P, Ji H, Xiao Y, Li K. Global patterns and trends in pancreatic cancer incidence: age, period, and birth cohort analysis. Pancreas. (2019) 48:199–208. doi: 10.1097/MPA.0000000000001230
8. Vick AD, Hery DN, Markowiak SF, Brunicardi FC. Closing the disparity in pancreatic cancer outcomes: a Closer look at nonmodifiable factors and their potential use in treatment. Pancreas. (2019) 48:242–9. doi: 10.1097/MPA.0000000000001238
9. Camara SN, Yin T, Yang M, Li X, Gong Q, Zhou J, et al. High risk factors of pancreatic carcinoma. Xuebao Yixue Yingdewen Ban. (2016) 36:295–304.
10. Hollingworth R, Grand RJ. Modulation of DNA damage and repair pathways by human tumour viruses. Viruses. (2015) 7:2542–91. doi: 10.3390/v7052542
11. Vergara D, Simeone P, Damato M, Maffia M, Lanuti P, Trerotola M. The cancer microbiota: EMT and inflammation as shared molecular mechanisms associated with plasticity and progression. J Oncol. (2019) 2019:1253727. doi: 10.1155/2019/1253727
12. Elinav E, Nowarski R, Thaiss CA, Hu B, Jin C, Flavell RA. Inflammation-induced cancer: crosstalk between tumours, immune cells and microorganisms. Nat Rev Cancer. (2013)13:759–71. doi: 10.1038/nrc3611
13. Avilés-Jiménez F, Guitron A, Segura-López F, Méndez-Tenorio A, Iwai S, Hernández-Guerrero A, et al. Microbiota studies in the bile duct strongly suggest a role for helicobacter pylori in extrahepatic cholangiocarcinoma. Clin Microbiol Infect. (2016) 22:178.e11-178.e22. doi: 10.1016/j.cmi.2015.10.008
14. Pereira P, Aho V, Arola J, Boyd S, Jokelainen K, Paulin L, et al. Bile microbiota in primary sclerosing cholangitis: impact on disease progression and development of biliary dysplasia. PLoS ONE. (2017) 12:e0182924. doi: 10.1371/journal.pone.0182924
15. Ye F, Shen H, Li Z, Meng F, Li L, Yang J, et al. Influence of the biliary system on biliary bacteria revealed by bacterial communities of the human biliary and upper digestive tracts. PLoS ONE. (2016) 11:e0150519. doi: 10.1371/journal.pone.0150519
16. Bridgewater JA, Goodman KA, Kalyan A, Mulcahy MF. Biliary tract cancer: epidemiology, radiotherapy, and molecular profiling. Am Soc Clin Oncol Educ. (2016) 35:e194-203. doi: 10.1200/EDBK_160831
17. Liou GY, Bastea L, Fleming A, Döppler H, Edenfield BH, Dawson DW, et al. The presence of interleukin-13 at pancreatic ADM/PanIN lesions alters macrophage populations and mediates pancreatic tumorigenesis. Cell Rep. (2017) 19:1322–33. doi: 10.1016/j.celrep.2017.04.052
18. Carrer A, Trefely S, Zhao S, Campbell SL, Norgard RJ, Schultz KC, et al. Acetyl-CoA metabolism supports multistep pancreatic tumorigenesis. Cancer Discov. (2019) 9:416–35. doi: 10.1158/2159-8290.CD-18-0567
19. Khan AS, Dageforde LA. Cholangiocarcinoma. Surg Clin North Am. (2019) 99:315–35. doi: 10.1016/j.suc.2018.12.004
20. Pushalkar S, Hundeyin M, Daley D, Zambirinis CP, Kurz E, Mishra A, et al. The pancreatic cancer microbiome promotes oncogenesis by induction of innate and adaptive immune suppression. Cancer Discov. (2018) 8:403–16. doi: 10.1158/2159-8290.CD-17-1134
21. Riquelme E, Zhang Y, Zhang L, Montiel M, Zoltan M, Dong W, et al. Tumor microbiome diversity and composition influence pancreatic cancer outcomes. Cell. (2019) 178:795–806.e12. doi: 10.1016/j.cell.2019.07.008
22. Fan X, Alekseyenko AV, Wu J, Peters BA, Jacobs EJ, Gapstur SM, et al. Human oral microbiome and prospective risk for pancreatic cancer: a population-based nested case-control study. Gut. (2016) 67:120–7. doi: 10.1158/1538-7445.AM2016-4350
23. Farrell JJ, Zhang L, Zhou H, Chia D, Elashoff D, Akin D, et al. Variations of oral microbiota are associated with pancreatic diseases including pancreatic cancer. Gut. (2012) 61:582–8. doi: 10.1136/gutjnl-2011-300784
24. Pan S, Brentnall TA, Chen R. Proteomics analysis of bodily fluids in pancreatic cancer. Proteomics. (2015) 15:2705–15. doi: 10.1002/pmic.201400476
25. Chen KT, Kim PD, Jones KA, Devarajan K, Patel BB, Hoffman JP, et al. Potential prognostic biomarkers of pancreatic cancer. Pancreas. (2014) 43:22–7. doi: 10.1097/MPA.0b013e3182a6867e
26. Raudvere U, Kolberg L, Kuzmin I, Arak T, Adler P, Peterson H, et al. g:Profiler: a web server for functional enrichment analysis and conversions of gene lists (2019 update). Nucleic Acids Res. (2019) 47:W191–8. doi: 10.1093/nar/gkz369
27. Kanehisa M, Sato Y. KEGG mapper for inferring cellular functions from protein sequences. Protein Sci Publ Protein Soc. (2019) 29:28–35. doi: 10.1002/pro.3711
28. Kanehisa M, Goto S, Sato Y, Kawashima M, Furumichi M, Tanabe M. Data, information, knowledge and principle: back to metabolism in kEGG. Nucleic Acids Res. (2014) 42(Database issue):D199–205. doi: 10.1093/nar/gkt1076
29. Tan AA, Azman SN, Abdul Rani NR, Kua BC, Sasidharan S, Kiew LV, et al. Optimal protein extraction methods from diverse sample types for protein profiling by using two-Dimensional electrophoresis (2DE). Trop Biomed. (2011) 28:620–9.
30. Wieczorek S, Combes F, Lazar C, Giai Gianetto Q, Gatto L, Dorffer A, et al. DAPAR & proStaR: software to perform statistical analyses in quantitative discovery proteomics. Bioinforma Oxf Engl. (2017) 33:135–6. doi: 10.1093/bioinformatics/btw580
31. Kanehisa M, Sato Y, Morishima K. Blastkoala and Ghostkoala: Kegg tools for functional characterization of genome and metagenome sequences. J Mol Biol. (2016) 428:726–31. doi: 10.1016/j.jmb.2015.11.006
32. Liao Y, Wang J, Jaehnig EJ, Shi Z, Zhang B. WebGestalt 2019: gene set analysis toolkit with revamped uIs and aPIs. Nucleic Acids Res. (2019) 47:W199–205. doi: 10.1093/nar/gkz401
33. Oladele Ogunseitan. Cross-species interactions among prokaryotes. In: Microbial Diversity Form and Function in Prokaryotes. Oxford, UK: Blackwell Science Ltd (2005).
34. Douglas GM, Maffei VJ, Zaneveld JR, Yurgel SN, Brown JR, Taylor CM, et al. PICRUSt2 for prediction of metagenome functions. Nat Biotechnol. (2020) 38:685–88. doi: 10.1038/s41587-020-0548-6
35. Wilmes P, Bond PL. Metaproteomics: studying functional gene expression in microbial ecosystems. Trends Microbiol. (2006) 14:92–7. doi: 10.1016/j.tim.2005.12.006
36. Blakeley-Ruiz JA, Erickson AR, Cantarel BL, Xiong W, Adams R, Jansson JK, et al. Metaproteomics reveals persistent and phylum-redundant metabolic functional stability in adult human gut microbiomes of crohn's remission patients despite temporal variations in microbial taxa, genomes, and proteomes. Microbiome. (2019) 7:18. doi: 10.1186/s40168-019-0631-8
37. Peters DL, Wang W, Zhang X, Ning Z, Mayne J, Figeys D. Metaproteomic and metabolomic approaches for characterizing the gut microbiome. Proteomics. (2019) 19:e1800363. doi: 10.1002/pmic.201800363
38. Patel K, Singh M, Gowda H. Bioinformatics methods to deduce biological interpretation from proteomics data. Methods Mol Biol Clifton NJ. (2017) 1549:147–61. doi: 10.1007/978-1-4939-6740-7_12
39. Mukaida N, Harada A, Yasumoto K, Matsushima K. Properties of pro-inflammatory cell type-specific leukocyte chemotactic cytokines, interleukin 8 (IL-8) and monocyte chemotactic and activating factor (MCAF). Microbiol Immunol. (1992) 36:773–89. doi: 10.1111/j.1348-0421.1992.tb02080.x
40. Russo RC, Garcia CC, Teixeira MM, Amaral FA. The cXCL8/IL-8 chemokine family and its receptors in inflammatory diseases. Expert Rev Clin Immunol. (2014) 10:593–619. doi: 10.1586/1744666X.2014.894886
41. Young RS, Wiles BM, McGee DW. IL-22 enhances tNF-α- and iL-1-Induced cXCL8 responses by intestinal epithelial cell lines. Inflammation. (2017) 40:1726–34. doi: 10.1007/s10753-017-0614-5
42. Kasahara T, Mukaida N, Yamashita K, Yagisawa H, Akahoshi T, Matsushima K. IL-1 and tNF-alpha induction of iL-8 and monocyte chemotactic and activating factor (MCAF) mRNA expression in a human astrocytoma cell line. Immunology. (1991) 74:60–7.
43. Waugh DJJ, Wilson C. The interleukin-8 pathway in cancer. Clin Cancer Res Off J Am Assoc Cancer Res. (2008) 14:6735–41. doi: 10.1158/1078-0432.CCR-07-4843
44. Alfaro C, Sanmamed MF, Rodríguez-Ruiz ME, Teijeira Á, Oñate C, González Á, et al. Interleukin-8 in cancer pathogenesis, treatment and follow-up. Cancer Treat Rev. (2017) 60:24–31. doi: 10.1016/j.ctrv.2017.08.004
45. Liu Q, Li A, Tian Y, Wu JD, Liu Y, Li T, et al. The cXCL8-CXCR1/2 pathways in cancer. Cytokine Growth Factor Rev. (2016) 31:61–71. doi: 10.1016/j.cytogfr.2016.08.002
46. Kamohara H, Takahashi M, Ishiko T, Ogawa M, Baba H. Induction of interleukin-8 (CXCL-8) by tumor necrosis factor-alpha and leukemia inhibitory factor in pancreatic carcinoma cells: impact of cXCL-8 as an autocrine growth factor. Int J Oncol. (2007) 31:627–32. doi: 10.3892/ijo.31.3.627
47. Ebrahimi B, Tucker SL, Li D, Abbruzzese JL, Kurzrock R. Cytokines in pancreatic carcinoma: correlation with phenotypic characteristics and prognosis. Cancer. (2004) 101:2727–36. doi: 10.1002/cncr.20672
48. Sun Q, Li F, Sun F, Niu J. Interleukin-8 is a prognostic indicator in human hilar cholangiocarcinoma. Int J Clin Exp Pathol. (2015) 8:8376–84.
49. Chen L, Fan J, Chen H, Meng Z, Chen Z, Wang P, et al. The iL-8/CXCR1 axis is associated with cancer stem cell-like properties and correlates with clinical prognosis in human pancreatic cancer cases. Sci Rep. (2014) 4:5911. doi: 10.1038/srep05911
50. Fang Y, Saiyin H, Zhao X, Wu Y, Han X, Lou W. IL-8-Positive tumor-Infiltrating inflammatory cells are a novel prognostic marker in pancreatic ductal adenocarcinoma patients. Pancreas. (2016) 45:671–8. doi: 10.1097/MPA.0000000000000520
51. Peyyala R, Kirakodu SS, Novak KF, Ebersole JL. Oral microbial biofilm stimulation of epithelial cell responses. Cytokine. (2012) 58:65–72. doi: 10.1016/j.cyto.2011.12.016
52. Ramage G, Lappin DF, Millhouse E, Malcolm J, Jose A, Yang J, et al. The epithelial cell response to health and disease associated oral biofilm models. J Periodontal Res. (2017) 52:325–33. doi: 10.1111/jre.12395
53. Ebersole JL, Peyyala R, Gonzalez OA. Biofilm-induced profiles of immune response gene expression by oral epithelial cells. Mol Oral Microbiol. (2019) 34:1. doi: 10.1111/omi.12251
54. Björstad A, Fu H, Karlsson A, Dahlgren C, Bylund J. Interleukin-8-derived peptide has antibacterial activity. Antimicrob Agents Chemother. (2005) 49:3889–95. doi: 10.1128/AAC.49.9.3889-3895.2005
55. Guo Q, Jian Z, Jia B, Chang L. CXCL7 promotes proliferation and invasion of cholangiocarcinoma cells. Oncol Rep. (2017) 37:1114–22. doi: 10.3892/or.2016.5312
56. Dufies M, Giuliano S, Viotti J, Borchiellini D, Cooley LS, Ambrosetti D, et al. CXCL7 is a predictive marker of sunitinib efficacy in clear cell renal cell carcinomas. Br J Cancer. (2017) 117:947–53. doi: 10.1038/bjc.2017.276
57. Desurmont T, Skrypek N, Duhamel A, Jonckheere N, Millet G, Leteurtre E, et al. Overexpression of chemokine receptor cXCR2 and ligand cXCL7 in liver metastases from colon cancer is correlated to shorter disease-free and overall survival. Cancer Sci. (2015) 106:262–9. doi: 10.1111/cas.12603
58. Li Y, Yu HP, Zhang P. CCL15 overexpression predicts poor prognosis for hepatocellular carcinoma. Hepatol Int. (2016) 10:488–92. doi: 10.1007/s12072-015-9683-4
59. Li Y, Wu J, Zhang P. CCL15/CCR1 axis is involved in hepatocellular carcinoma cells migration and invasion. Tumour Biol J Int Soc Oncodevelopmental Biol Med. (2016) 37:4501–7. doi: 10.1007/s13277-015-4287-0
60. Yang S-M, Li S-Y, Hao-Bin Y, Lin-Yan X, Sheng X. IL-11 activated by lnc-ATB promotes cell proliferation and invasion in esophageal squamous cell cancer. Biomed Pharmacother Biomedecine Pharmacother. (2019) 114:108835. doi: 10.1016/j.biopha.2019.108835
61. Putoczki TL, Thiem S, Loving A, Busuttil RA, Wilson NJ, Ziegler PK, et al. Interleukin-11 is the dominant iL-6 family cytokine during gastrointestinal tumorigenesis and can be targeted therapeutically. Cancer Cell. (2013) 24:257–71. doi: 10.1016/j.ccr.2013.06.017
62. Kamada-Nobusada T, Sakakibara H. Molecular basis for cytokinin biosynthesis. Phytochemistry. (2009) 70:444–9. doi: 10.1016/j.phytochem.2009.02.007
63. Akiyoshi DE, Regier DA, Gordon MP. Cytokinin production by agrobacterium and pseudomonas spp. J Bacteriol. (1987) 169:4242–8. doi: 10.1128/JB.169.9.4242-4248.1987
64. Kakimoto T. Biosynthesis of cytokinins. J Plant Res. (2003) 116:233–9. doi: 10.1007/s10265-003-0095-5
65. Voller J, Zatloukal M, Lenobel R, Dolezal K, Béres T, Krystof V, et al. Anticancer activity of natural cytokinins: a structure-activity relationship study. Phytochemistry. (2010) 71:1350–9. doi: 10.1016/j.phytochem.2010.04.018
66. Siwak E, Jewginski M, Kustrzeba-Wójcicka I. Biological activity of surfactins - a case of a biosurfactant produced by Bacillus subtilis pCM (1949). Acta Biochim Pol. (2015) 62:875–8. doi: 10.18388/abp.2015_1149
67. Das P, Mukherjee S, Sen R. Antimicrobial potential of a lipopeptide biosurfactant derived from a marine bacillus circulans. J Appl Microbiol. (2008) 104:1675–84. doi: 10.1111/j.1365-2672.2007.03701.x
68. Loiseau C, Schlusselhuber M, Bigot R, Bertaux J, Berjeaud J-M, Verdon J. Surfactin from Bacillus subtilis displays an unexpected anti-Legionella activity. Appl Microbiol Biotechnol. (2015) 99:5083–93. doi: 10.1007/s00253-014-6317-z
69. Zhao H, Shao D, Jiang C, Shi J, Li Q, Huang Q, et al. Biological activity of lipopeptides from bacillus. Appl Microbiol Biotechnol. (2017) 101:5951–60. doi: 10.1007/s00253-017-8396-0
70. Yuan L, Zhang S, Wang Y, Li Y, Wang X, Yang Q. Surfactin inhibits membrane fusion during invasion of epithelial cells by enveloped viruses. J Virol. (2018) 92:18. doi: 10.1128/JVI.00809-18
71. Liu J, Li W, Zhu X, Zhao H, Lu Y, Zhang C, et al. Surfactin effectively inhibits staphylococcus aureus adhesion and biofilm formation on surfaces. Appl Microbiol Biotechnol. (2019) 103:4565–74. doi: 10.1007/s00253-019-09808-w
72. Bais HP, Fall R, Vivanco JM. Biocontrol of bacillus subtilis against infection of arabidopsis roots by pseudomonas syringae is facilitated by biofilm formation and surfactin production. Plant Physiol. (2004) 134:307–19. doi: 10.1104/pp.103.028712
73. Moryl M, Spetana M, Dziubek K, Paraszkiewicz K, Rózalska S, Płaza GA, et al. Antimicrobial, antiadhesive and antibiofilm potential of lipopeptides synthesised by bacillus subtilis, on uropathogenic bacteria. Acta Biochim Pol. (2015) 62:725–32. doi: 10.18388/abp.2015_1120
74. Netzker T, Flak M, Krespach MK, Stroe MC, Weber J, Schroeckh V, et al. Microbial interactions trigger the production of antibiotics. Curr Opin Microbiol. (2018) 45:117–23. doi: 10.1016/j.mib.2018.04.002
75. Abrudan MI, Smakman F, Grimbergen AJ, Westhoff S, Miller EL, van Wezel GP, et al. Socially mediated induction and suppression of antibiosis during bacterial coexistence. Proc Natl Acad Sci USA. (2015) 112:11054–9. doi: 10.1073/pnas.1504076112
76. López D, Vlamakis H, Kolter R. Biofilms. Cold Spring Harb Perspect Biol. (2010) 2:a000398. doi: 10.1101/cshperspect.a000398
77. Sakuragi Y, Kolter R. Quorum-sensing regulation of the biofilm matrix genes (pel) of pseudomonas aeruginosa. J Bacteriol. (2007) 189:5383–6. doi: 10.1128/JB.00137-07
78. Balkwill F, Mantovani A. Inflammation and cancer: back to virchow? Lancet Lond Engl. (2001) 357:539–45. doi: 10.1016/S0140-6736(00)04046-0
79. Francescone R, Hou V, Grivennikov SI. Microbiome, inflammation, and cancer. Cancer J Sudbury Mass. (2014) 20:181–9. doi: 10.1097/PPO.0000000000000048
80. Shadhu K, Xi C. Inflammation and pancreatic cancer: an updated review. Saudi J Gastroenterol Off J Saudi Gastroenterol Assoc. (2019) 25:3–13. doi: 10.4103/sjg.SJG_390_18
81. Kleeff J, Korc M, Apte M, La Vecchia C, Johnson CD, Biankin AV, et al. Pancreatic cancer. Nat Rev Dis Primer. (2016) 2:16022. doi: 10.1038/nrdp.2016.22
82. Barreto SG, Dutt A, Chaudhary A. A genetic model for gallbladder carcinogenesis and its dissemination. Ann Oncol Off J Eur Soc Med Oncol ESMO. (2014) 25:1086–97. doi: 10.1093/annonc/mdu006
83. Macgregor-Das AM, Iacobuzio-Donahue CA. Molecular pathways in pancreatic carcinogenesis. J Surg Oncol. (2013) 107:8–14. doi: 10.1002/jso.23213
Keywords: pancreatic cancer, metaproteomic, proteomic, bile, zeatin, surfactin, IL-8, carcinogenesis model
Citation: Arteta AA, Sánchez-Jiménez M, Dávila DF, Palacios OG and Cardona-Castro N (2020) Biliary Tract Carcinogenesis Model Based on Bile Metaproteomics. Front. Oncol. 10:1032. doi: 10.3389/fonc.2020.01032
Received: 05 February 2020; Accepted: 26 May 2020;
Published: 24 July 2020.
Edited by:
Suman S. Thakur, Centre for Cellular and Molecular Biology (CCMB), IndiaReviewed by:
Alessandro Carrer, Veneto Institute of Molecular Medicine (VIMM), ItalyDaniele Vergara, University of Salento, Italy
Copyright © 2020 Arteta, Sánchez-Jiménez, Dávila, Palacios and Cardona-Castro. This is an open-access article distributed under the terms of the Creative Commons Attribution License (CC BY). The use, distribution or reproduction in other forums is permitted, provided the original author(s) and the copyright owner(s) are credited and that the original publication in this journal is cited, in accordance with accepted academic practice. No use, distribution or reproduction is permitted which does not comply with these terms.
*Correspondence: Nora Cardona-Castro, bmNhcmRvbmEmI3gwMDA0MDtjZXMuZWR1LmNv