- 1Translational Sciences Section, Jonsson Comprehensive Cancer Center, School of Nursing, University of California, Los Angeles, Los Angeles, CA, United States
- 2Department of Human Genetics, David Geffen School of Medicine, University of California, Los Angeles, Los Angeles, CA, United States
- 3Department of Epidemiology, Fielding School of Public Health, University of California, Los Angeles, Los Angeles, CA, United States
- 4Center for Human Nutrition, David Geffen School of Medicine, University of California, Los Angeles, Los Angeles, CA, United States
Background: The roles of obesity-related biomarkers and their molecular pathways in the development of postmenopausal colorectal cancer (CRC) have been inconclusive. We examined insulin resistance (IR) as a major hormonal pathway mediating the association between obesity and CRC risk in a Mendelian randomization (MR) framework.
Methods: We performed MR analysis using individual-level data of 11,078 non-Hispanic white postmenopausal women from our earlier genome-wide association study. We identified four independent single-nucleotide polymorphisms associated with fasting glucose (FG), three with fasting insulin (FI), and six with homeostatic model assessment–IR (HOMA-IR), which were not associated with obesity. We estimated hazard ratios (HRs) for CRC by adjusting for potential confounding factors plus genetic principal components.
Results: Overall, we observed no direct association between combined 13 IR genetic instruments and CRC risk (HR = 0.96, 95% confidence interval [CI]: 0.78–1.17). In phenotypic analysis, genetically raised HOMA-IR exhibited its effects on the increased risk and FG and FI on the reduced risk for CRC, but with a lack of statistical power. Subgroup analyses by physical activity level and dietary fat intake with combined phenotypes showed that genetically determined IR was associated with reduced CRC risk in both physical activity-stratified (single contributor: MTRR rs722025; HR = 0.12, 95% CI: 0.02–0.62) and high-fat diet subgroups (main contributor: G6PC2 rs560887; HR = 0.59, 95% CI: 0.37–0.94).
Conclusions: Complex evidence was observed for a potential causal association between IR and CRC risk. Our findings may provide an additional value of intervention trials to lower IR and reduce CRC risk.
Introduction
Postmenopausal women have a high incidence of and mortality from colorectal cancer (CRC). Approximately 90% of newly diagnosed CRC patients and CRC-related deaths occur in women aged 50 years and older (1); thus, their high risk for CRC development and worse prognosis contribute to CRC's third place rank in cancer incidence and mortality among women of the United States and other Westernized counties (2, 3). While obesity (both overall and abdominal) is a well-known risk factor (4, 5), the roles of obesity-related biomarkers and their molecular pathways connected to colorectal carcinogenesis have not been conclusive. In particular, the insulin resistance (IR)/insulin-like growth factor-I (IGF-I) axis has been considered a major hormonal pathway and has played a crucial mediating role in the association between obesity and CRC development (5). For example, in vitro and in vivo studies showed that insulin stimulates the growth of colorectal tumors in cell lines (6) and animal models (7). Additionally, metformin, a medicine used to regulate glucose homeostasis, suppresses the activity of obesity-related CRC cells (8).
Molecular biologic studies suggest potential mechanisms for the association between IR and CRC risk, including overexpression of insulin and IGF-I receptors (9–11) and hyperregulation and dysregulation of downstream cell-signaling pathways (10, 12–14), leading to the reduced apoptotic and enhanced anabolic cellular state necessary for tumor growth and development. However, previous clinical and observational epidemiologic studies for the relationship between IR and CRC risk among women ages 50 years and older are inconsistent. Some studies have found blood glucose levels and homeostatic model assessment–IR (HOMA-IR) associated with CRC (15, 16) while other studies have found no associations of glucose, insulin, and HOMA-IR levels with CRC (17, 18). In particular, one study examining postmenopausal women revealed that fasting glucose levels were associated with increased CRC risk (16), while another study (18) using the same study population showed no clear association between HOMA-IR and CRC risk. Those inconsistent findings may be in part due to selection bias, confounding, and/or modifying effects of obesity and associated lifestyle factors, relatively short-time exposure to biomarkers, and reverse causation; further, the lack of consensus in these findings calls for in-depth research such as Mendelian randomization (MR) studies, which could potentially improve the causal inference.
An MR approach could address those challenges. It examines genetic variants (e.g., single-nucleotide polymorphisms [SNPs]) as an instrumental variable to evaluate the effect of the genetically determined exposure (e.g., IR) on an outcome (e.g., CRC risk) (19). This genetic analysis may be a useful tool to establish a relatively unbiased causal relationship between IR and CRC risk by circumventing potential biases and residual confounding. Since random assortment of alleles occurs when a gamete is formed, this can lead to random assignment of exposure (19, 20). In addition, an MR approach may examine a lifelong exposure to an allele because the random assignment of genetic variations occurs at meiosis (20). Furthermore, MR prevents reverse causation in that the randomly assigned genetic variations precede the phenotypes and clinical outcomes (20, 21).
In the current study, we performed MR analysis by using our earlier genome-wide association study (GWAS) results (22) to test the hypothesis that genetically determined IR has a potential causal association with CRC risk in postmenopausal women.
Materials and Methods
Data Sources and Selection of Candidate Instrumental Variables
We analyzed the data from our earlier meta-analysis of a genome-wide gene–environmental (i.e., behavioral) interaction (G × E) study (22) among 11,078 non-Hispanic white women after menopause. Those women were enrolled in the Women's Health Initiative (WHI) Harmonized and Imputed GWASs under the WHI Database for Genotypes and Phenotypes (dbGaP) Study, accession number phs000200.v11.p3. The detailed study rationale and design have been described elsewhere (23, 24). Briefly, healthy postmenopausal women were enrolled in the WHI study between 1993 and 1998 from more than 40 clinical centers across the United States. They were eligible for the WHI dbGaP study if they had met eligibility requirements for submission to dbGaP and provided DNA samples. The WHI Harmonized and Imputed studies consist of six GWASs. From the six GWASs, we obtained the genotyped data. The genotyped calls were normalized to reference panel GRCh37, and genotype imputation was performed using the 1,000 Genomes Project reference panel (24). In the initial and secondary data-quality cleaning processes, we included SNPs with a missing-call rate of <3%, a Hardy–Weinberg equilibrium of p ≥ 10−4, and an imputation quality of , in our previous G × E GWAS meta-analysis. The study was approved by the institutional review boards of each participating clinical center of the WHI and the University of California, Los Angeles.
We used the results from our meta-analysis of the G × E GWAS for IR and CRC risk. We identified IR-associated SNPs at genome-wide significance (p <5 × 10−8) and used them as genetic instrumental variables in this study. We further pruned SNPs according to linkage disequilibrium (LD) estimates to select SNPs with r2 <0.1. Among the 58 SNPs associated with IR phenotypes overall or in subgroups stratified by obesity, physical activity level, and high-fat diet intake, we ultimately identified the following independent SNPs: (1) four independent SNPs associated with fasting glucose (FG) levels (one in the overall, two in the physically active, and one in the high-fat diet groups); (2) three associated with fasting insulin (FI) levels (one in the obese, one in the physically inactive, and one in the low-fat diet groups); and (3) six associated with HOMA-IR (two in the overall, two in the low-fat diet, and two in the high-fat diet groups).
Statistical Analysis
For each identified SNP, we conducted multiple Cox proportional hazards regression analyses to obtain the hazard ratios (HRs) and 95% confidence intervals (CIs) for CRC risk by checking assumptions with a Schoenfeld residual plot and rho. The analyses were adjusted for potential confounding factors. The confounding factors were selected through literature review (1, 25) for their associations with IR and CRC risk and from the initial analysis process, including univariate and stepwise multiple regression analyses and a multi-collinearity test: 10 genetic principal components (PCs) as well as age, education, family income, depressive symptoms, cardiovascular disease ever, hypertension ever, high cholesterol, family history of CRC, physical activity, smoking, height, body mass index (BMI), waist-to-hip ratio, dietary alcohol, dietary fiber, daily fruits, daily vegetables, percentage of calories from saturated fatty acids (SFA), monounsaturated fatty acids, polyunsaturated fatty acids, and protein, hysterectomy ever, ages at menarche and menopause, breastfeeding, oral contraceptive duration, and exogenous estrogen (E)-only and E plus progestin use.
We first checked basic MR assumptions to see whether our data fulfilled the conditions required for valid causal inference. Traditionally in MR analysis, genetic instruments should not have a weak relationship with their phenotypes. To address that, we estimated a sum of the T-squared statistics across SNPs for the overall and specified groups by phenotype and by subgroup (Table S1). By using the commonly used threshold of 10 units (26), we found that our SNP instruments were well powered for downstream MR analysis.
In addition, an MR approach could be confounded when the analyzed SNPs present biological pleiotropy or are independently associated with CRC risk through intermediate pathways other than IR. To assess whether our data has a potential pleiotropic effect, we utilized the following analytic approaches. Considering that obesity is a well-established risk factor for CRC and could exhibit its pleiotropic effect independently of or interrelatedly with the IR-CRC pathway, we interrogated for the association of obesity (27) with the modeled SNPs to exclude from the MR analysis. No evidence of SNPs having pleiotropic association with obesity was observed. We further conducted an MR-Egger analysis (28) and tested for directional pleiotropy, which indicates that the pleiotropic effect across SNPs is skewed on outcome in one direction rather than being balanced. No significant directional pleiotropic effect of SNPs by phenotype and by subgroup was found (Table S2).
Having found that our SNPs have sufficient strength to predict relevant phenotypes and are less likely to be confounded, we next performed MR analysis by employing the inverse-variance weighted method (29). This quantifies the association between genetically derived IR and CRC risk. During the MR analysis, we took into account a population correlation that could occur when exposure (IR) and outcome (CRC development) were assessed within the same population. We therefore adjusted for Spearman correlation rho between each IR phenotype and CRC in the analysis. For the individual genetic-instrumental effects on the risk for CRC, we estimated the ratio of β coefficients (=ßcolorectalcancer/ßIR) (20). The results were reported as risk ratios (RRs) and 95% CIs and interpreted as the change in CRC risk per unit increase in log-odds of IR or the change in RR (exponentiation of β) for women with IR compared with women without.
To test for additional evidence of pleiotropy, we examined the heterogeneity of MR estimates by using Cochran's Q-test. We considered a two-tailed p <0.05 statistically significant. We used R statistical software (v 3.5.1).
Results
The 13 IR SNPs in the different subgroups from our earlier G × E GWAS and their risk for CRC development are presented in Table 1. In particular, the two SNPs PABPC1P2 rs77772624 and LINC00460 rs17254590, in relation to HOMA-IR, were shown at genome-wide association in the overall and high-fat diet (calories from SFA ≥ 7%) subgroups.
In the MR analysis of individual genetic instruments for the association between their respective phenotype (FG, FI, and HOMA-IR) and CRC risk, we identified three SNPs whose genetically determined effect of IR on CRC risk was statistically significant (Table 2 and Figure 1). The SNPs G6PC rs560887 (FG, HR = 0.59, 95% CI: 0.37–0.94) in the high-fat diet subgroup; MKLN1 rs117911989 (FG, HR = 0.42, 95% CI: 0.21–0.87) in the physically active (metabolic equivalent [MET] ≥ 10) subgroup, and MTRR rs722025 (FI, HR = 0.12, 95% CI: 0.02–0.62) in the physically inactive (MET <10) subgroup were associated with CRC risk. When the genetic instruments were combined by phenotype and evaluated for CRC risk (Table 2), the pooled estimates of genetically derived FG and FI were associated with reduced CRC risk whereas that of genetically derived HOMA-IR was associated with slightly increased CRC risk, although those estimates were not statistically significant.
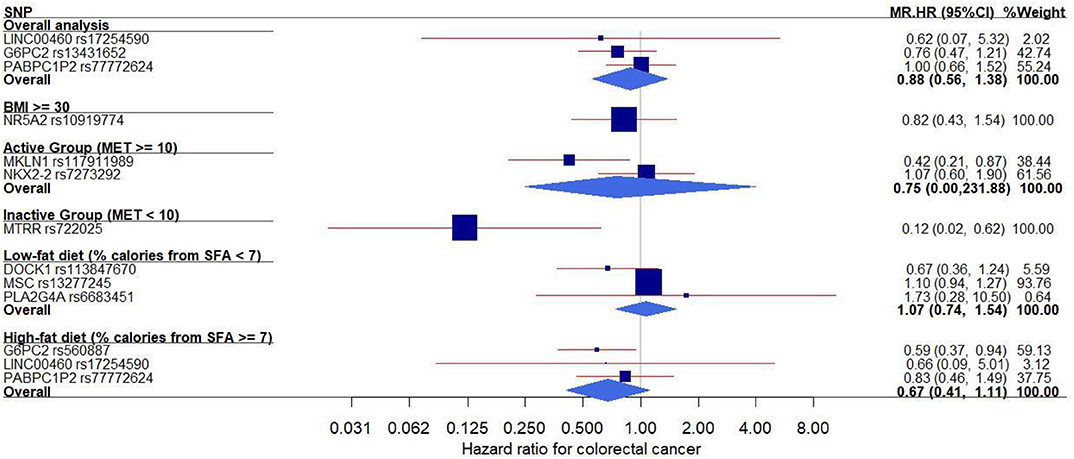
Figure 1. Forest plot of the MR effects of IR on colorectal cancer risk in the overall and subgroups. For each of non-pleiotropic IR SNPs, the plot shows the effects of genetically driven IR (FG, FI, or HOMA-IR) on colorectal cancer risk in the overall and subgroups, presented as the 95% CIs (indicated with red lines) of the estimates and the inverse-variance weights (percentages proportional to the size of the blue squares). BMI, body mass index; CI, confidence interval; FG, fasting glucose; FI, fasting insulin; HOMA-IR, homeostatic model assessment–insulin resistance; HR, hazard ratio; IR, insulin resistance; MET, metabolic equivalent; MR, Mendelian randomization; SFA, saturated fatty acids; SNP, single–nucleotide polymorphism.
In addition, we conducted MR analyses in the subgroups stratified by BMI, physical activity, and dietary fat intake (Figure 1). In both physical activity-stratified subgroups (i.e., MET ≥ 10 and <10), genetically elevated IR was associated with a reduced risk for CRC. Similarly, in the high-fat diet subgroup, genetically raised IR was associated with decreased CRC risk. However, in the low-fat diet (calories from SFA <7%) subgroup, genetically raised IR was associated with increased risk for CRC, although the effect in this low-fat group was not statistically significant. We further performed an overall pooled MR analysis by combining all the IR SNPs (Figure 2) and found no evidence of statistically significant association between genetically predicted IR and CRC risk (HR = 0.96, 95% CI: 0.78–1.17).
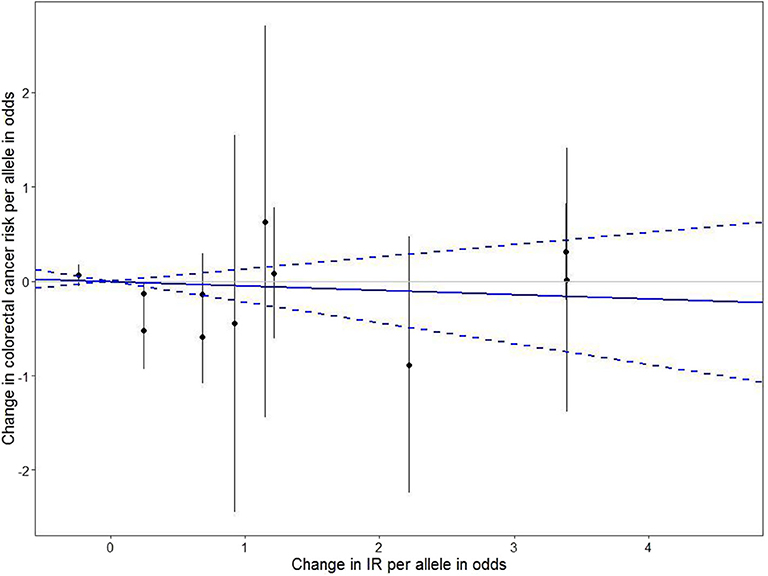
Figure 2. The effect of individual IR-genetic instrumental variables on colorectal cancer risk. Each black dot reflects a genome-wide IR-raising genetic variant. The blue lines indicate regression and 95% CIs of IR on colorectal cancer risk (HR = 0.96, 95% CI: 0.78–1.17). CI, confidence interval; HR, hazard ratio; IR, insulin resistance.
We also conducted a sensitivity test for the effect of IR genetic-instrumental variables on the risk for CRC by replacing current HRs with HRs obtained from the regressions adjusted for age and the 10 genetic PCs only. Similar MR results were observed, and no apparent directional pleiotropy was observed.
Discussion
We evaluated the genetically determined effect of IR phenotypes on the risk for CRC in postmenopausal women by conducting an MR analysis, which could improve causal inference. If the MR study is not affected by pleiotropic effects through any alternative pathway, it could provide as robust a causal inference as randomized clinical trials do (19). Of note, our study included nonoverlapping genetic variants between IR and obesity, indicating the exclusion of potential pleiotropic effect from obesity. In addition, the MR approach could exhibit the lifelong effect of exposure (IR) on CRC risk, and compared with an observational study, its results are less susceptible to reverse causality. Thus, although our MR study was not designed to explore directly biological mechanisms, our findings suggest that the long-standing effect of IR is likely to affect the risk of postmenopausal CRC.
In particular, in our combined MR analysis by phenotype, genetically raised FG and FI exhibited their effects on the reduced risk for CRC, but with a lack of statistical power. Previous observational studies reported no associations of FG and FI levels with CRC risk (17, 18). That may indicate that FG and FI reflect the glycogenolysis activity in relation to insulin sensitivity in the liver (30), thus perhaps representing a relatively short-term phenomenon of IR. In contrast, 2-h glucose levels reflect beta cell function and insulin sensitivity in skeletal muscle (31), which may represent relatively long-term exposure to IR. In addition, glycated hemoglobin, a form of hemoglobin with an attached glucose molecule after exposure to high glucose levels (32), and C-peptide, a molar secretion from beta cells, which is on an equal basis with insulin, may function as integrated indicators of more stable measures of IR (33). Thus, future research into those long-term biomarkers with CRC risk is warranted.
In our individual MR analysis, G6PC2 rs560887 was the main contributor to the MR effect estimate of FG on reduced CRC risk. G6PC2 opposes the action of glucokinase in beta cells and thus regulates glycolytic flux and glucose-stimulated insulin secretion (34). This genetic polymorphism can cause mild hyperglycemia from birth onward and ultimately the development of type 2 diabetes in adulthood. Thus, early detection of the prediabetic condition may lead to the treatment of other cancer risk factors such as hypercholesterolemia, rendering additional protection from CRC later in life (35). Further, this G6PC2 genetic instrument and its association with CRC would have been missed without the incorporation of fatty acids (i.e., observed in the high-fat diet group). This line of inquiry calls for future biological function research.
Additionally, our genetic instrumental analyses for the individual FI-related SNPs indicated that rs722025 in the MTRR gene was a significant contributor to the genetically derived effect of FI on CRC risk. This association was observed only in the physically inactive subgroup. A genetic polymorphism in MTRR in adipose tissue may prompt endoplasmic reticular stress, leading to inhibited insulin signaling, and thus resulting in IR and type 2 diabetes (36). In previous studies, this genetic polymorphism has been associated with several cancer types such as lung (37), stomach (38, 39), liver (40), and colorectal (41–44) cancers. Our findings of its relationship with IR and CRC are consistent with the findings of the aforementioned studies that reported negative (congruent direction with our finding) (41) or positive association with CRC risk (42–44), but our findings draw attention to the interaction with obesity factors because the association between the SNP and CRC risk was detected in physically inactive women only. This suggests that the analysis integrated with obesity-related lifestyle factors is essential. The SNP's protective effect on CRC risk in the inactive subgroup may be due to unmeasured confounders or other epigenetic pathways; thus, further molecular biologic–mechanism studies were needed to confirm our result.
MKLN1 is an intracellular protein that mediates cell responses to the extracellular matrix and that influences cell adhesion and cytoskeleton organization (45, 46). It has been known to be associated with pancreatic (46) and lung cancer (47) and is a novel marker for cardiovascular risk (48). It has also been associated with type 2 diabetes (49). Our findings of its association with IR phenotypes are consistent with previous results, but our study newly reported the association of MKLN1 with CRC risk. This association would have been missed without the incorporation of the physical activity factor, which will further require additional studies on larger populations to clarify the possible role of variation in this gene in colorectal carcinogenesis.
Our MR study results should be interpreted with some caution because of several assumptions required to be met. First, SNP instruments may not be correlated with other SNPs. We properly addressed this issue by pruning correlated SNPs. Second, the genetic variants must explain substantially the respective phenotypes. We included those SNPs having a strong association signal with their related phenotype. For the confounding factors that could introduce bias, we reduced the pleiotropic effect from obesity by using the following methods: (1) in our earlier GWA G × E study for the association of IR, we performed stratification analyses by obesity and associated lifestyle factors, which could have reduced the modifying effects of such factors before this MR analysis, and (2) in the current study, we estimated HRs for CRC by adjusting for potential confounding factors such as lifestyle and reproductive factors as well as 10 genetic PCs and further examined the association between genetically determined IR and CRC risk. Nonetheless, our results could be biased due to residual unmeasured confounding factors.
In addition, our pooled estimates by phenotype were not shown to be statistically significant in the overall analysis, but some significant associations in obesity subgroups were detected. This may reflect the heterogeneity of individual SNPs' estimates in the overall causal pathway connected to CRC risk and suggest the potential existence of genetically determined IR-outcome association that interacts with obesity factors. Obesity may act upstream of IR; that is, the effect of obesity between IR and CRC is substantial, so removing obesity could yield less reliable MR estimates (i.e., a weak direct effect of IR on CRC risk). Further, our results may indicate that biological pathways other than IR exist between obesity and CRC development. Last, we decreased the potential for population structure bias by adjusting for the population correction between IR and CRC in this individual-level analysis of the exposure and outcome data obtained from the same population.
MR results may also be subject to a nonlinear relationship between exposure and outcome. In our study, the genetically determined IR and its association with CRC risk may have been affected by a feedback mechanism (e.g., canalization), resulting in nonlinearity. But such canalization tends to bias MR results toward the null, so altering the statistical directions or significance is less likely (50). Our study may overfit the analysis owing to the nature of the data, where the exposure and outcome were obtained from the same study population. Finally, we examined 13 identified genetic instruments, and the results could have inflated false-positive rates due to multiple comparisons.
In summary, we attempted to improve the causal inference between IR and CRC risk and quantified the association by using genetic instruments in an MR framework. We obtained complex evidence that lifetime exposure to IR is likely to influence the risk for CRC in postmenopausal women. Future metabolic biologic study of this complicated association between IR and CRC by incorporating behavioral factors is warranted to clarify the underlying mechanisms of the associations we observed. Nonetheless, our results may contribute to building additional evidence for promoting intervention trials to lower IR and thus, to reduce CRC risk.
Data Availability Statement
The datasets presented in this article are not readily available because the data that support the findings of this study are available in accordance with policies developed by the NHLBI and WHI in order to protect sensitive participant information and approved by the Fred Hutchinson Cancer Research Center, which currently serves as the IRB of record for the WHI. Requests to access the datasets should be directed to the corresponding author.
Ethics Statement
The studies involving human participants were reviewed and approved by the Institutional Review Boards of each participating clinical center of the WHI and the University of California, Los Angeles. The patients/participants provided their written informed consent to participate in this study.
Author Contributions
SJ, JP, ES, and Z-FZ designed the study. SJ performed the genomic data QC. SJ, JP, and ES performed the statistical analysis and interpreted the data. ES and Z-FZ supervised the genomic data QC and analysis and participated in the study coordination and interpreting the data. SJ secured funding for this project. All participated in the paper writing and editing. All authors have read and approved the submission of the manuscript. All authors contributed to the article and approved the submitted version.
Funding
This study was supported by the National Institute of Nursing Research of the National Institutes of Health under Award Number K01NR017852 and a University of California Cancer Research Coordinating Committee grant (CRN-18-522722).
Conflict of Interest
The authors declare that the research was conducted in the absence of any commercial or financial relationships that could be construed as a potential conflict of interest.
Acknowledgments
Part of the data for this project was provided by the WHI program, which is funded by the National Heart, Lung, and Blood Institute, the National Institutes of Health, and the U.S. Department of Health and Human Services through contracts HHSN268201100046C, HHSN268201100001C, HHSN268201100002C, HHSN268201100003C, HHSN268201100004C, and HHSN271201100004C. The datasets used for the analyses described in this manuscript were obtained from dbGaP at http://www.ncbi.nlm.nih.gov/sites/entrez?db=gap through dbGaP accession (phs000200.v11.p3).
Program Office: National Heart, Lung, and Blood Institute, Bethesda, MD: Jacques Rossouw, Shari Ludlam, Dale Burwen, Joan McGowan, Leslie Ford, and Nancy Geller.
Clinical Coordinating Center: Fred Hutchinson Cancer Research Center, Seattle, WA: Garnet Anderson, Ross Prentice, Andrea LaCroix, and Charles Kooperberg.
Investigators and Academic Centers: Brigham and Women's Hospital, Harvard Medical School, Boston, MA: JoAnn E. Manson; MedStar Health Research Institute/Howard University, Washington, DC: Barbara V. Howard; Stanford Prevention Research Center, Stanford, CA: Marcia L. Stefanick; The Ohio State University, Columbus, OH: Rebecca Jackson; University of Arizona, Tucson/Phoenix, AZ: Cynthia A. Thomson; University at Buffalo, Buffalo, NY: Jean Wactawski-Wende; University of Florida, Gainesville/Jacksonville, FL: Marian Limacher; University of Iowa, Iowa City/Davenport, IA: Robert Wallace; University of Pittsburgh, Pittsburgh, PA: Lewis Kuller; Wake Forest University School of Medicine, Winston-Salem, NC: Sally Shumaker.
Supplementary Material
The Supplementary Material for this article can be found online at: https://www.frontiersin.org/articles/10.3389/fonc.2020.01005/full#supplementary-material
References
1. American Cancer Society. Colorectal Cancer Facts & Figures 2017-2019. Atlanta: American Cancer Society, Inc. (2017). Available online at: https://www.cancer.org/content/dam/cancer-org/research/cancer-facts-and-statistics/colorectal-cancer-facts-and-figures/colorectal-cancer-facts-and-figures-2017-2019.pdf.
2. American Cancer Society. Cancer Fact and Figures, 2019. Atlanta: American Cancer Society, Inc. (2019) Available online at: https://www.cancer.org/content/dam/cancer-org/research/cancer-facts-and-statistics/annual-cancer-facts-and-figures/2019/cancer-facts-and-figures-2019.pdf.
3. Bray F, Ferlay J, Soerjomataram I, Siegel RL, Torre LA, Jemal A. Global cancer statistics 2018: GLOBOCAN estimates of incidence and mortality worldwide for 36 cancers in 185 countries. CA Cancer J Clin. (2018) 68:394–424. doi: 10.3322/caac.21492
4. Jochem C, Leitzmann M. Obesity and colorectal cancer. Recent results in cancer Res. (2016) 208:17–41. doi: 10.1007/978-3-319-42542-9_2
5. Nimptsch K, Pischon T. Obesity biomarkers, metabolism and risk of cancer: an epidemiological perspective. Recent Results Cancer Res. (2016) 208:199–217. doi: 10.1007/978-3-319-42542-9_11
6. Bjork J, Nilsson J, Hultcrantz R, Johansson C. Growth-regulatory effects of sensory neuropeptides, epidermal growth factor, insulin, and somatostatin on the non-transformed intestinal epithelial cell line IEC-6 and the colon cancer cell line HT 29. Scand J Gastroenterol. (1993) 28:879–84. doi: 10.3109/00365529309103129
7. Tran TT, Medline A, Bruce WR. Insulin promotion of colon tumors in rats. Cancer Epidemiol Biomark Prev. (1996) 5:1013–5.
8. Abdelsatir AA, Husain NE, Hassan AT, Elmadhoun WM, Almobarak AO, Ahmed MH. Potential benefit of metformin as treatment for colon cancer: the evidence so far. Asian Pac J Cancer Prev. (2015) 16:8053–8. doi: 10.7314/APJCP.2015.16.18.8053
9. Clayton PE, Banerjee I, Murray PG, Renehan AG. Growth hormone, the insulin-like growth factor axis, insulin and cancer risk. Nat Rev Endocrinol. (2011) 7:11–24. doi: 10.1038/nrendo.2010.171
11. Gallagher EJ, LeRoith D. Minireview: IGF, insulin, and cancer. Endocrinology. (2011) 152:2546–51. doi: 10.1210/en.2011-0231
12. Arcidiacono B, Iiritano S, Nocera A, Possidente K, Nevolo MT, Ventura V, et al. Insulin resistance and cancer risk: an overview of the pathogenetic mechanisms. Exp Diabetes Res. (2012) 2012:789174. doi: 10.1155/2012/789174
13. Zhang H, Wang A, Ma H, Xu Y. Association between insulin receptor substrate 1 Gly972Arg polymorphism and cancer risk. Tumour Biol. (2013) 34:2929–36. doi: 10.1007/s13277-013-0855-3
14. Ruan Y, Ma J, Xie X. Association of IRS-1 and IRS-2 genes polymorphisms with polycystic ovary syndrome: a meta-analysis. Endocr J. (2012) 59:601–9. doi: 10.1507/endocrj.EJ11-0387
15. Rampal S, Yang MH, Sung J, Son HJ, Choi YH, Lee JH, et al. Association between markers of glucose metabolism and risk of colorectal adenoma. Gastroenterology. (2014) 147:78–87.e73. doi: 10.1053/j.gastro.2014.03.006
16. Kabat GC, Kim MY, Strickler HD, Shikany JM, Lane D, Luo J, et al. A longitudinal study of serum insulin and glucose levels in relation to colorectal cancer risk among postmenopausal women. Br J Cancer. (2012) 106:227–32. doi: 10.1038/bjc.2011.512
17. Vulcan A, Manjer J, Ohlsson B. High blood glucose levels are associated with higher risk of colon cancer in men: a cohort study. BMC Cancer. (2017) 17:842. doi: 10.1186/s12885-017-3874-4
18. Kabat GC, Kim MY, Stefanick M, Ho GYF, Lane DS, Odegaard AO, et al. Metabolic obesity phenotypes and risk of colorectal cancer in postmenopausal women. Int J Cancer. (2018) 143:543–51. doi: 10.1002/ijc.31345
19. Shu X, Wu L, Khankari NK, Shu XO, Wang TJ, Michailidou K, et al. Associations of obesity and circulating insulin and glucose with breast cancer risk: a Mendelian randomization analysis. Int J Epidemiol. (2018) 48: 795–806. doi: 10.1093/ije/dyy201
20. Merino J, Leong A, Posner DC, Porneala B, Masana L, Dupuis J, et al. Genetically driven hyperglycemia increases risk of coronary artery disease separately from type 2 diabetes. Diabetes Care. (2017) 40:687–93. doi: 10.2337/dc16-2625
21. Ahmad OS, Leong A, Miller JA, Morris JA, Forgetta V, Mujammami M, et al. A mendelian randomization study of the effect of type-2 diabetes and glycemic traits on bone mineral density. J Bone Miner Res. (2017) 32:1072–81. doi: 10.1002/jbmr.3063
22. Jung SY, Mancuso N, Yu H, Papp J, Sobel E, Zhang ZF. Genome-wide meta-analysis of gene-environmental interaction for insulin resistance phenotypes and breast cancer risk in postmenopausal women. Cancer Prev Res. (2019) 12:31–42. doi: 10.1158/1940-6207.CAPR-18-0180
23. The Women's Health Initiative Study Group. Design of the women's health initiative clinical trial and observational study. The Women's Health Initiative Study Group. Control Clin Trials. (1998) 19:61–109. doi: 10.1016/S0197-2456(97)00078-0
24. NCBI. WHI Harmonized and Imputed GWAS Data. A Sub-Study of Women's Health Initiative. Available online at: http://www.ncbi.nlm.nih.gov/projects/gap/cgi-bin/study.cgi?study_id=phs000746.v1.p3.
25. Nichols HB, Trentham-Dietz A, Hampton JM, Newcomb PA. Oral contraceptive use, reproductive factors, and colorectal cancer risk: findings from Wisconsin. Cancer Epidemiol. (2005) 14:1212–8. doi: 10.1158/1055-9965.EPI-04-0845
26. Pierce BL, Ahsan H, Vanderweele TJ. Power and instrument strength requirements for Mendelian randomization studies using multiple genetic variants. Int J Epidemiol. (2011) 40:740–52. doi: 10.1093/ije/dyq151
27. Locke AE, Kahali B, Berndt SI, Justice AE, Pers TH, Day FR, et al. Genetic studies of body mass index yield new insights for obesity biology. Nature. (2015) 518:197–206. doi: 10.1038/nature14177
28. Bowden J, Davey Smith G, Burgess S. Mendelian randomization with invalid instruments: effect estimation and bias detection through Egger regression. Int J Epidemiol. (2015) 44:512–25. doi: 10.1093/ije/dyv080
29. Burgess S, Butterworth A, Thompson SG. Mendelian randomization analysis with multiple genetic variants using summarized data. Genet Epidemiol. (2013) 37:658–65. doi: 10.1002/gepi.21758
30. Matthews DR, Hosker JP, Rudenski AS, Naylor BA, Treacher DF, Turner RC. Homeostasis model assessment: insulin resistance and beta-cell function from fasting plasma glucose and insulin concentrations in man. Diabetologia. (1985) 28:412–9. doi: 10.1007/BF00280883
31. DeFronzo RA, Tripathy D. Skeletal muscle insulin resistance is the primary defect in type 2 diabetes. Diabetes Care. (2009) 32(Suppl 2):S157–S63. doi: 10.2337/dc09-S302
32. Bunn HF, Gabbay KH, Gallop PM. The glycosylation of hemoglobin: relevance to diabetes mellitus. Science. (1978) 200:21–7. doi: 10.1126/science.635569
33. Van Cauter E, Mestrez F, Sturis J, Polonsky KS. Estimation of insulin secretion rates from C-peptide levels. Comparison of individual and standard kinetic parameters for C-peptide clearance. Diabetes. (1992) 41:368–77. doi: 10.2337/diabetes.41.3.368
34. Bouatia-Naji N, Rocheleau G, Van Lommel L, Lemaire K, Schuit F, Cavalcanti-Proenca C, et al. A polymorphism within the G6PC2 gene is associated with fasting plasma glucose levels. Science. (2008) 320:1085–8. doi: 10.1126/science.1156849
35. Steele AM, Shields BM, Wensley KJ, Colclough K, Ellard S, Hattersley AT. Prevalence of vascular complications among patients with glucokinase mutations and prolonged, mild hyperglycemia. JAMA. (2014) 311:279–86. doi: 10.1001/jama.2013.283980
36. Zhi X, Yang B, Fan S, Li Y, He M, Wang D, et al. Additive Interaction of MTHFR C677T and MTRR A66G polymorphisms with being overweight/obesity on the risk of type 2 diabetes. Int J Environ Res Public Health. (2016) 13:1243. doi: 10.3390/ijerph13121243
37. Aksoy-Sagirli P, Erdenay A, Kaytan-Saglam E, Kizir A. Association of three single nucleotide polymorphisms in MTR and MTRR genes with lung cancer in a turkish population. Genet Test Mol Biomark. (2017) 21:428–32. doi: 10.1089/gtmb.2017.0062
38. Chang SC, Chang PY, Butler B, Goldstein BY, Mu L, Cai L, et al. Single nucleotide polymorphisms of one-carbon metabolism and cancers of the esophagus, stomach, and liver in a Chinese population. PLoS One. (2014) 9:e109235. doi: 10.1371/journal.pone.0109235
39. Zhao T, Xu Z, Gu D, Wu P, Huo X, Wei X, et al. The effects of genomic polymorphisms in one-carbon metabolism pathways on survival of gastric cancer patients received fluorouracil-based adjuvant therapy. Sci Rep. (2016) 6:28019. doi: 10.1038/srep28019
40. Zhang H, Liu C, Han YC, Ma Z, Ma Y, Liu X. Genetic variations in the one-carbon metabolism pathway genes and susceptibility to hepatocellular carcinoma risk: a case-control study. Tumour Biol. (2015) 36:997–02. doi: 10.1007/s13277-014-2725-z
41. Cheng TY, Makar KW, Neuhouser ML, Miller JW, Song X, Brown EC, et al. Folate-mediated one-carbon metabolism genes and interactions with nutritional factors on colorectal cancer risk: Women's Health Initiative Observational Study. Cancer. (2015) 121:3684–91. doi: 10.1002/cncr.29465
42. Zhou D, Mei Q, Luo H, Tang B, Yu P. The polymorphisms in methylenetetrahydrofolate reductase, methionine synthase, methionine synthase reductase, and the risk of colorectal cancer. Int J Biol Sci. (2012) 8:819–30. doi: 10.7150/ijbs.4462
43. Wu PP, Tang RN, An L. A meta-analysis of MTRR A66G polymorphism and colorectal cancer susceptibility. J BUON. (2015) 20:918–22. Available online at: https://www.jbuon.com/archive/20-3-918.pdf
44. Pardini B, Kumar R, Naccarati A, Prasad RB, Forsti A, Polakova V, et al. MTHFR and MTRR genotype and haplotype analysis and colorectal cancer susceptibility in a case-control study from the Czech Republic. Mutat Res. (2011) 721:74–80. doi: 10.1016/j.mrgentox.2010.12.008
45. Adams JC, Seed B, Lawler J. Muskelin, a novel intracellular mediator of cell adhesive and cytoskeletal responses to thrombospondin-1. EMBO J. (1998) 17:4964–74. doi: 10.1093/emboj/17.17.4964
46. Wolpin BM, Rizzato C, Kraft P, Kooperberg C, Petersen GM, Wang Z, et al. Genome-wide association study identifies multiple susceptibility loci for pancreatic cancer. Nat Genet. (2014) 46:994–1000. doi: 10.1038/ng.3052
47. McKay JD, Hung RJ, Han Y, Zong X, Carreras-Torres R, Christiani DC, et al. Large-scale association analysis identifies new lung cancer susceptibility loci and heterogeneity in genetic susceptibility across histological subtypes. Nat Genet. (2017) 49:1126–32. doi: 10.1038/ng.3892
48. Warren HR, Evangelou E, Cabrera CP, Gao H, Ren M, Mifsud B, et al. Genome-wide association analysis identifies novel blood pressure loci and offers biological insights into cardiovascular risk. Nat Genet. (2017) 49:403–15. doi: 10.1038/ng.3768
49. Rouillard AD, Gundersen GW, Fernandez NF, Wang Z, Monteiro CD, McDermott MG, et al. The harmonizome: a collection of processed datasets gathered to serve and mine knowledge about genes and proteins. Database. (2016) 2016:baw100. doi: 10.1093/database/baw100
Keywords: Mendelian randomization, genetically driven insulin resistance, obesity, physical activity, high-fat diet, colorectal cancer, postmenopausal women
Citation: Jung SY, Papp JC, Sobel EM and Zhang Z-F (2020) Mendelian Randomization Study: The Association Between Metabolic Pathways and Colorectal Cancer Risk. Front. Oncol. 10:1005. doi: 10.3389/fonc.2020.01005
Received: 30 March 2020; Accepted: 21 May 2020;
Published: 23 July 2020.
Edited by:
Aga Syed Sameer, King Saud bin Abdulaziz University for Health Sciences, Saudi ArabiaReviewed by:
Mujeeb Zafar Banday, Government Medical College (GMC), IndiaHao Liu, Southern Medical University, China
Copyright © 2020 Jung, Papp, Sobel and Zhang. This is an open-access article distributed under the terms of the Creative Commons Attribution License (CC BY). The use, distribution or reproduction in other forums is permitted, provided the original author(s) and the copyright owner(s) are credited and that the original publication in this journal is cited, in accordance with accepted academic practice. No use, distribution or reproduction is permitted which does not comply with these terms.
*Correspondence: Su Yon Jung, sjung@sonnet.ucla.edu