- 1Imaging Physics Department, University of Texas MD Anderson Cancer Center, Houston, TX, United States
- 2Diagnostic Radiology Department, University of Texas MD Anderson Cancer Center, Houston, TX, United States
Background: Hepatocellular carcinoma (HCC) is the most common liver malignancy and the leading cause of death in patients with cirrhosis. Various treatments for HCC are available, including transarterial chemoembolization (TACE), which is the commonest intervention performed in HCC. Radiologic tumor response following TACE is an important prognostic factor for patients with HCC. We hypothesized that, for large HCC tumors, assessment of treatment response made with automated volumetric response evaluation criteria in solid tumors (RECIST) might correlate with the assessment made with the more time- and labor-intensive unidimensional modified RECIST (mRECIST) and manual volumetric RECIST (M-vRECIST) criteria. Accordingly, we undertook this retrospective study to compare automated volumetric RECIST (A-vRECIST) with M-vRECIST and mRESIST for the assessment of large HCC tumors' responses to TACE.
Methods: We selected 42 pairs of contrast-enhanced computed tomography (CT) images of large HCCs. Images were taken before and after TACE, and in each of the images, the HCC was segmented using both a manual contouring tool and a convolutional neural network. Three experienced radiologists assessed tumor response to TACE using mRECIST criteria. The intra-class correlation coefficient was used to assess inter-reader reliability in the mRECIST measurements, while the Pearson correlation coefficient was used to assess correlation between the volumetric and mRECIST measurements.
Results: Volumetric tumor assessment using automated and manual segmentation tools showed good correlation with mRECIST measurements. For A-vRECIST and M-vRECIST, respectively, r = 0.597 vs. 0.622 in the baseline studies; 0.648 vs. 0.748 in the follow-up studies; and 0.774 vs. 0.766 in the response assessment (P < 0.001 for all). The A-vRECIST evaluation showed high correlation with the M-vRECIST evaluation (r = 0.967, 0.937, and 0.826 in baseline studies, follow-up studies, and response assessment, respectively, P < 0.001 for all).
Conclusion: Volumetric RECIST measurements are likely to provide an early marker for TACE monitoring, and automated measurements made with a convolutional neural network may be good substitutes for manual volumetric measurements.
Introduction
Hepatocellular carcinoma (HCC) is the most common liver malignancy and the leading cause of death in patients with cirrhosis. Despite advances in various treatment modalities over the past several years, the prognosis for HCC remains poor, with 5-year overall survival ranging from 24 to 41% (1, 2). Efforts have been made to improve early detection of HCC by the performance of frequent screening in high-risk populations. However, most cases are still diagnosed at intermediate to advanced stages (3). These patients are not candidates for curative therapies, such as surgical resection or liver transplant. As a result, treatment options for this patient population are limited to loco-regional treatments, including local radiofrequency ablation, radio and chemoembolization, and systemic chemotherapy with Sorafenib (4–6).
In the United States, transarterial chemoembolization (TACE) is the most common intervention for HCC (7). It is the standard of care for patients with intermediate-stage HCC (according to Barcelona clinic liver cancer (BCLC) staging, whether it is large tumor or multi-nodular). In addition, it may be used in advanced HCC prior to systemic therapy or as a bridging therapy prior to surgery (8). There is evidence that repeat TACE may also be beneficial in patients with advanced HCC (9–11). Radiologic tumor response following initial TACE has been shown to be an important prognostic factor for patients with HCC. Baseline imaging is usually obtained 2–3 weeks before therapy and follow-up imaging is performed 4–6 weeks after therapy. The most commonly used criteria for tumor response following HCC is mRECIST (1-dimension) or EASL (2-dimensions).
The recent attempts to improve the accuracy of radiologic response criteria to predict overall survival and Progression-free survival have focused on using quantitative volumetric analysis. This has resulted in the development of the volumetric RECIST (vRECIST) and quantitative EASL (qEASL) methods with better results in predicting patient's outcome than the currently used mRECIST (12–15).
The volumetric assessment of tumor response depends on manual segmentation of tumor and needs contouring of the lesion in every single slice of the study. This process is time consuming and tedious. The manual volumetric assessment show high inter- and intra-observer variability which make it impractical in daily practice (16–18). On the other hand, automated volumetric segmentation has the potential to reduce time for this process and make volumetric assessment of tumors more practical, with lower inter and intra-observer variability than both mRECIST and manual volumetric assessment (12, 18, 19).
Convolutional neural network (CNN) shows promise to achieve automated segmentation of liver and liver masses. These are, however, computationally demanding (12, 13). CNN in tumor segmentation has been found to be more accurate and closer to the manual volumetric segmentation in larger tumors, with far lower accuracy in smaller liver masses (14, 15).
The purpose of this study is to assess the feasibility of volumetric assessment of pre- and post-TACE HCC using fully automated segmentation and to evaluate the correlation of automated volumetric assessment with both manual volumetric assessment and mRECIST measurements.
Materials and Methods
Study Cohort
This is a retrospective, single-institution, IRB approved study. This study included patients with large HCC tumors (≥5 cm) diagnosed and treated at our institution between November 2002 and June 2012. Patients were included in the study if (1) they had undergone TACE as their sole first-line or initial bridging therapy; (2) their medical records included multiphasic, contrast-enhanced CT images that were obtained at baseline and that included no image artifacts (e.g., surgical clips); and (3) their tumor was diagnosed as tumor-node-metastasis (TNM) stage III or IV HCC based on the American Joint Committee on Cancer (AJCC). Although there are numerous HCC scoring systems that incorporate liver functional reserve, patient performance, and gross tumor characteristics (e.g., size, vascular invasion, number of lesions), we chose the TNM staging system because it is the only HCC staging system that considers tumor characteristics (including size) without taking any other factor into consideration (20, 21).
TACE
Briefly, TACE is delivery method of chemotherapy delivery to the tumor through its feeding arteries using trans-arterial approach. The hepatic artery was selected and injected with chemotherapy by super selection of the feeding vasculature using advanced micro-catheters. There are two main chemotherapeutic regimens that may be delivered. In conventional TACE, a mixture of radio-opaque ethiodized emulsion oil (Lipiodol; Guerbet, Villepinte, France) and doxorubicin or cisplatin was injected followed by embolization of the feeding vessels. While in TACE with drug eluting beads (DEB-TACE), a mixture of micro-sphere particles, doxorubicin and soluble non-ionic contrast was injected (22).
Our patients received one of the following chemotherapeutic regimens (Drug regimen details are missed from 2 cases): (1) embolic microsphere beads (Biocompatibles, UK) loaded with doxorubicin (DEBDOX; drug-eluting bead doxorubicin) (15 lesions), (2) cisplatin, doxorubicin, and mitomycin C (22 lesions), or (3) cisplatin and mitomycin C only (3 lesions) (such information is missed from 2 cases).
CT Imaging Technique
All patients underwent dynamic, contrast-enhanced CT scans of the abdomen on 4-, 16-, or 64-slice multidetector CT LightSpeed scanners (GE Healthcare, Chicago, IL) pre-TACE and post-TACE. Liver protocol was used in all studies (the arterial, porto-venous, and delayed phases were captured 17, 60 s, and ~5 min, respectively, after peak enhancement of the descending aorta). Injection was done with an automated contrast injector using a bolus tracking technique and an injection rate of about 3–5 mL/s. The image reconstruction thicknesses were 2.5 mm and 5 mm.
Assessment of Tumor Response
Tumor TACE response was assessed using mRECIST, M-vRECIST, and A-vRECIST (Figure 1). Three different board certified radiologists (KE, JS, and AQ), each with more than 20 years of experience in abdominal imaging, independently measured tumors using mRECIST criteria. The changes in measurements between the follow-up and baseline CT scans were reported, and tumor viability and enhancement in the late arterial phase were taken into consideration. Volume assessment using M-vRECIST and A-vRECIST at baseline and follow-up studies was also done. The Convert3D medical image processing tool was used to extract the segmentation volumes according to the voxel extensions (23).
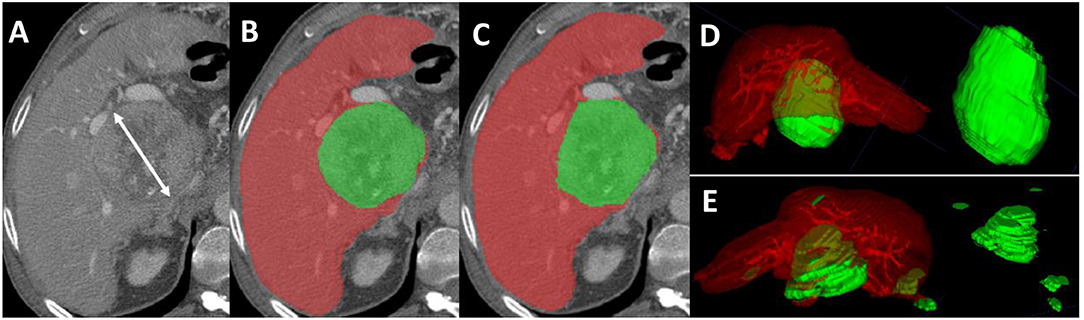
Figure 1. Axial sections from a pre-TACE abdominal CT scan of a 64-year old male patient with advanced HCC. (A) Shows an 8.5 cm lesion measured with mRECIST criteria. (B) Shows the manual segmentation of the hepatic parenchyma (red) and the HCC tissue (green); the tumor volume, measured with M-vRECIST, was 377.25 cm3. (C) Shows the automated segmentation of the parenchyma (red) and HCC tissue (green); the tumor volume measured with A-vRECIST was 187.9 cm3. (D,E) Show the 3-dimensional voxel rendering according to M-vRECIST and A-vRECIST, respectively. A-vRECIST, automated volumetric response evaluation criteria in solid tumors; CT, computed tomography; HCC, hepatocellular carcinoma; mRECIST, modified response evaluation criteria in solid tumors; M-vRECIST, manual volumetric response evaluation criteria in solid tumors; RECIST, response evaluation criteria in solid tumors; TACE, transarterial chemo embolization.
Tumor Segmentation
The porto-venous phase of CT (both baseline and follow-up) were used to simplify lesion assessment, they were exported in DICOM format from our institution's picture archiving and communication system to a separate research server. Subsequently, the images were converted into the format recommended by the Neuroimaging Informatics Technology Initiative (Nifti) to preserve the orientation information for further data processing. Then the files were compressed and the images were reoriented into right-anterior-inferior orientation with Convert3D.
Manual segmentations were performed in the portal-venous phase of contrast administration. This was performed for the baseline and follow-up CT studies. A semi-automated segmentation tool available in Amira Software (Thermo Fisher Scientific, Waltham, MA) was used to delineate the tumors, including the (i) enhancing portions, (ii) non-enhancing portions, and Lipiodol containing portions of the tumors. Enhancing tumor tissue was defined as a “region with uptake of contrast agent in the arterial phase of dynamic contrast CT” while non-enhancing tumor tissue was defined as a “region of no enhancement within HCC on the arterial phase images,” while Lipidol was defined as a “portions of high attenuation in pre-contrast images.” Manual segmentation of the whole tumor in the baseline study was done by one author (AM) to ensure the consistency of the segmentation throughout the dataset. The segmentation was done for all axial CT images. These manual segmentations provided the training data (n = 42 pairs) used to develop a neural network classifier for segmentation of tumors from the background liver tissue. Automated segmentation, performed using a CNN approach (U-Net), was used to segment the liver and tumor in two steps (24). To determine the correlation between mRECIST and MvRECIST and mRECIST and A-vRECIST, we compared the average uni-dimensional mRECIST measurements of the 3 readers to the M-vRECIST and A-vRECIST volumetric assessments.
Statistical Analysis
Statistical analysis was performed using IBM SPSS Statistics V.24.0 software (IBM, Armonk, NY). Inter-reader reliability for mRECIST measurement was assessed using the intraclass correlation coefficient (ICC). The Pearson correlation coefficient (r) was used to measure the correlation between the diameter change (the average reading from mRECIST) and (i) the automated and (ii) manual volume changes after TACE. A P-value of 0.05 was used to determine the statistical significance of the measurements.
Results
Patient Characteristics
There were 320 patients (with complete medical and survival data) found in our institutional database with HCC patients underwent TACE, we excluded 8 patients due to difference in their treatment plan (either TACE was used as second line or combined with other form of therapy). After thorough review of patients imaging studies, another 209 patients were excluded for different reasons (Figure 2). The final 103 patients were categorized according to their TNM stage into stage I, II, III, and IV with 36, 25, 24, and 18 patients. A total of 42 patients met our inclusion criteria for the study (TNM stage III and IV). On average, patients' baseline CT scans were performed 4 weeks (range between 2 and 7 weeks) before the first TACE session, and their follow-up scans were performed 11 weeks (range between 8 and 13 weeks) after the first TACE session. Patients' demographic data and baseline tumor characteristics are provided in Table 1.
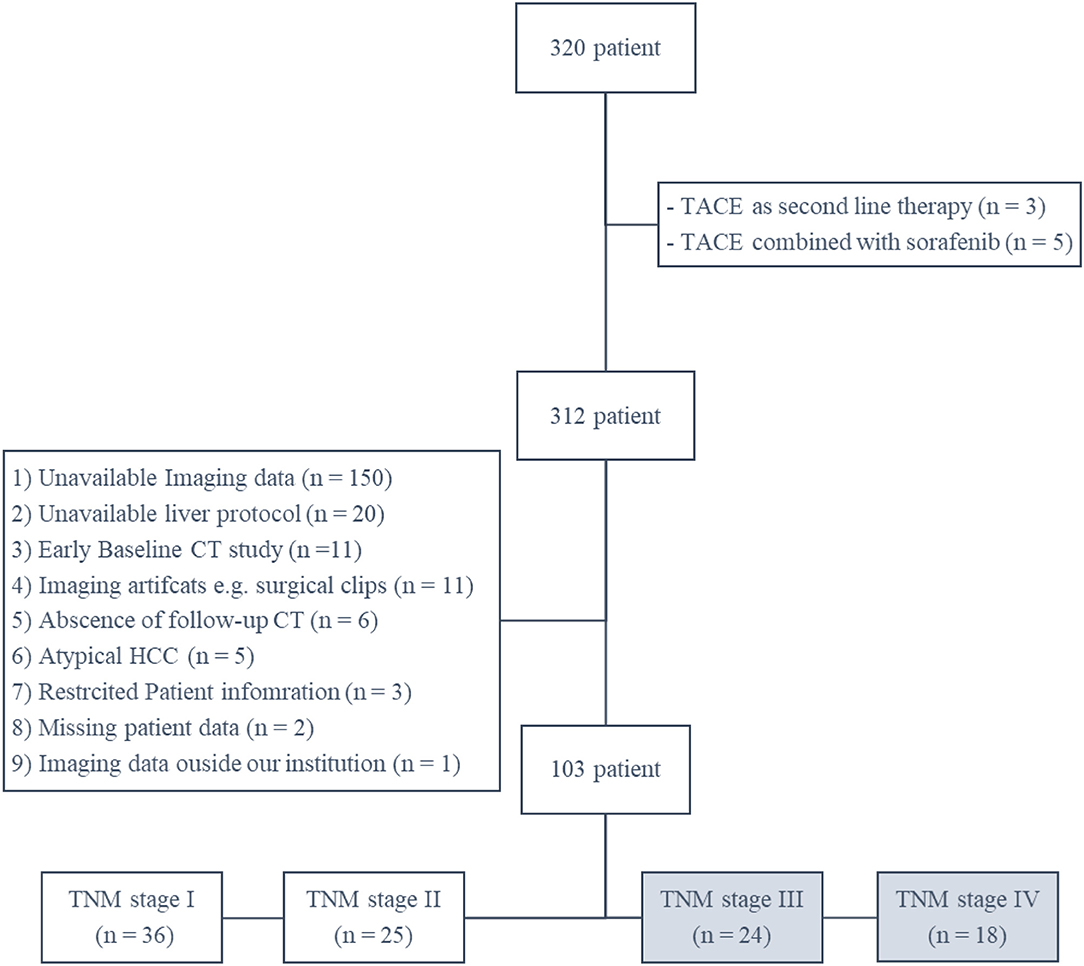
Figure 2. A schematic Flowchart of patient cohort selection process including the exclusion criteria.
Manual and Automated Volumetric Assessment
The mRECIST response comparing the pre- and post-TACE images is listed in Table 2 for the three radiologists'.
The manual vRECIST and automated vRECIST response comparing the pre- and post TACE are presented in Table 3, A-vRECIST was obtained with CNN. Assessment with the ICC showed statistically significant correlation between the three readers (ICC = 0.824; 95% CI = 0.70–0.89; P < 0.001).
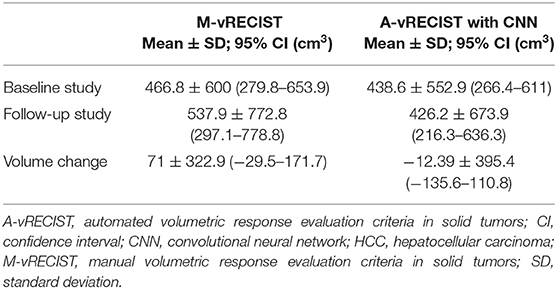
Table 3. Voxel-based volumetric measurements of HCC tumors made using M-vRECIST and A-vRECIST with CNN.
To determine the correlation between mRECIST and vRECIST (both manual and automated), we compared the average unidimensional mRECIST measurements to the M-vRECIST and A-vRECIST volumetric assessments (Figure 1) and compared the diameter changes determined through the manual and automated volumetric assessments. The correlation between mRECIST and M-vRECIST was moderate for both the baseline and the follow-up studies (r = 0.622 and 0.748, respectively). The correlation between tumor diameter measurement changes was higher (r = 0.766). The differences between these measurements were statistically significant (P < 0.001 for all). The correlation between mRECIST and A-vRECIST was similar: r = 0.597 for the baseline and r = 0.648 for the follow-up studies (P < 0.001 for all). For the correlation between the baseline and follow-up tumor measurements, r = 0.774.
We used the Pearson correlation coefficient to compare M-vRECIST and A-vRECIST and found strong linear correlation between the two approaches (r = 0.967 for the baseline studies, r = 0.937 for the follow-up studies, and r = 0.826 for the tumor volume change after TACE [P < 0.001 for all]) (Figures 3, 4).
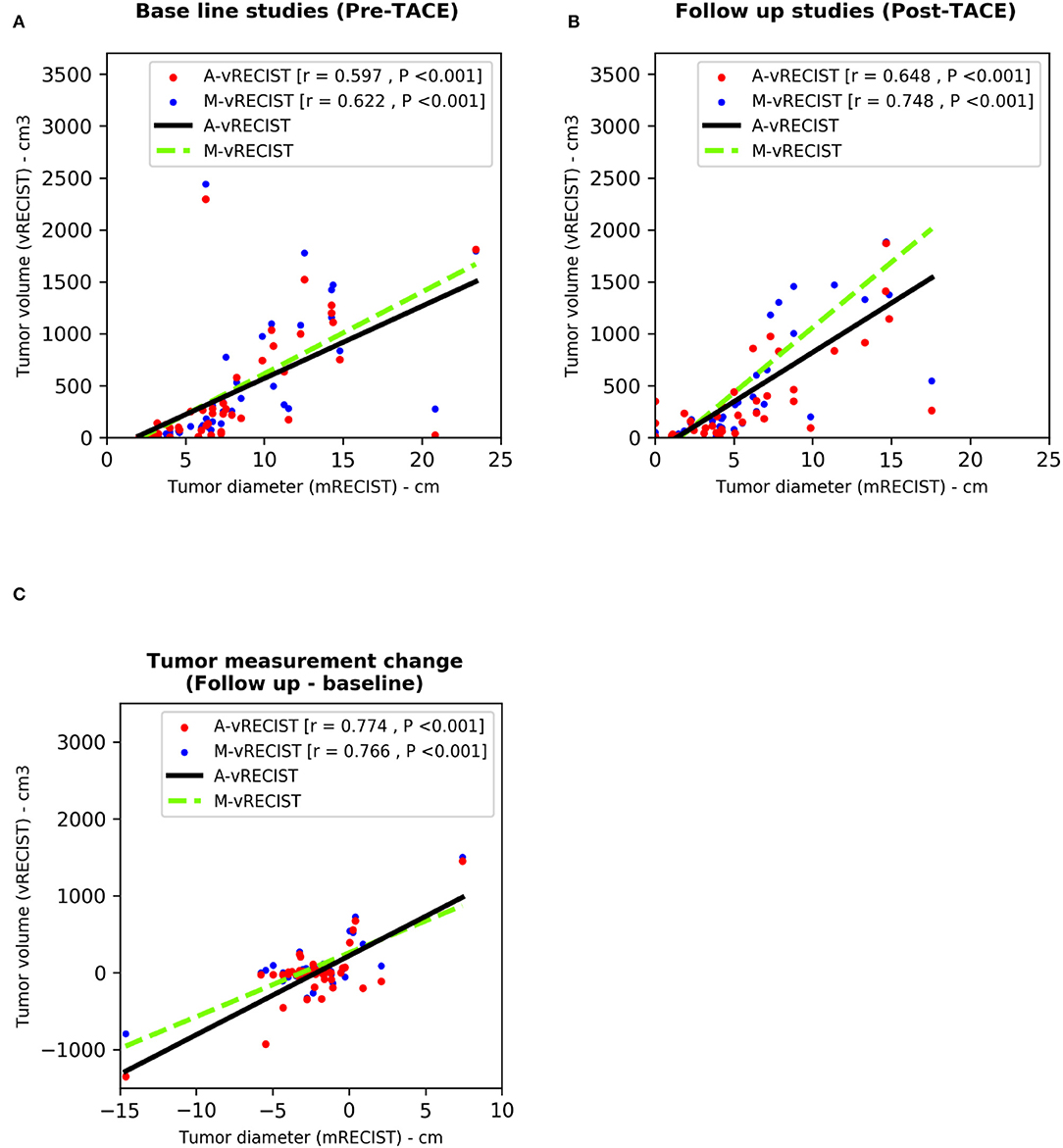
Figure 3. Scatter plots compare tumor assessments from unidimensional mRECIST with those from A-vRECIST and M-vRECIST in pre- and post-TACE studies [graphs (A,B), respectively]. Graph (C) shows the difference between the A-vRECIST and M-vRECIST measurements. The best-fit lines and Pearson coefficients (r) are shown. A-vRECIST, automated volumetric response evaluation criteria in solid tumors; mRECIST, modified response evaluation criteria in solid tumors; M-vRECIST, manual volumetric response evaluation criteria in solid tumors; RECIST, response evaluation criteria in solid tumors; TACE, transarterial chemo embolization.
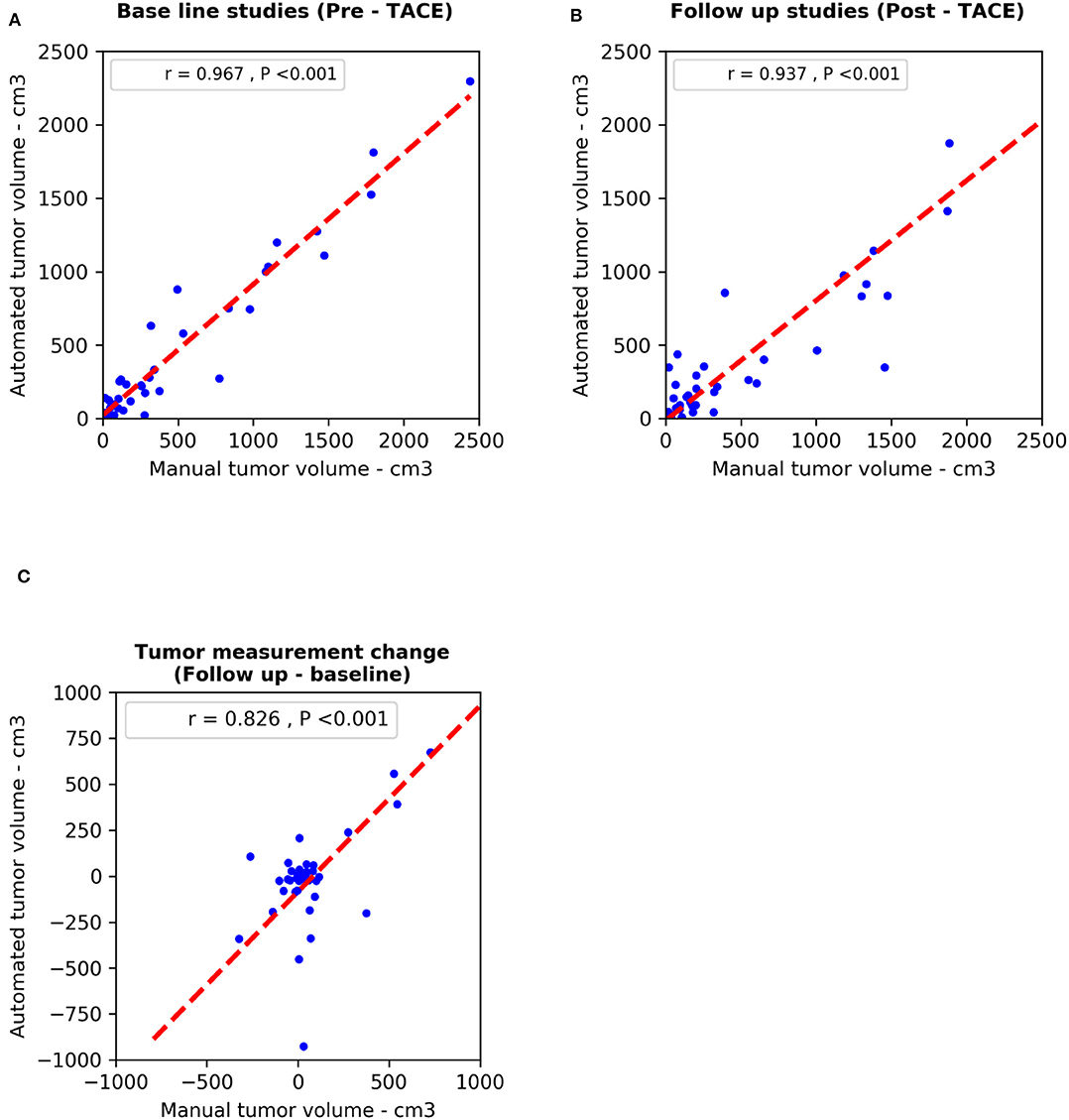
Figure 4. Scatter plots compare diameter changes from M-vRECIST vs. A-vRECIST assessments in the (A) baseline and (B) follow-up studies. Graph (C) shows the tumor measurement changes. The best-fit lines (dashed line) and Pearson correlation coefficients (r) are shown. A-vRECIST, automated volumetric response evaluation criteria in solid tumors; M-vRECIST, manual volumetric response evaluation criteria in solid tumors.
Discussion
In this study, we hypothesized that, for large HCC tumors, assessment of volume changes before and after TACE using A-vRECIST would correlate with the measurement changes using uni-dimentional mRECIST and that, accordingly, A-vRECIST can be used to assess tumor response to TACE therapy.
Volumetric assessment of HCC have been emerged as recent tool for assessing of HCC response to treatment. Although assessment of treatment response of is some patterns of HCC is challenging (especially diffuse/infiltrative type) due to its indistinct borders. Previous studies showed that both uni-dimensional and volumetric measurement highly correlated with the actual pathological tumor volume, with volumetric assessment was similar to pathological volume while uni-dimensional measurement overestimate the volume by 28% (25, 26). Such studies demonstrate the superiority of volumetric assessment to estimate the real tumor volume, which is more important during assessment og treatment response.
Another study showed that HCC response to Sorafenib using volumetric assessment can be used an alternative tool for monitoring therapy better than mRECIST measurement (27). Another study used functional MRI volumetric analysis of HCC tumors to separate patients into responders and non-responders following treatment with combination TACE and Sorafenib (23, 28). Both of these studies made 3D measurements of HCC tumor volumes. However, manual volumetric assessment is time consuming and highly variable leading to motivate scientists to automate this process. Automated tumor volume and enhancement measurements using cross-sectional images are proven to be both reproducible and feasible in clinical application (29). In addition, it have been demonstrated that automated quantitative tumor volume assessment can become part of monitoring response to TACE (29).
There are challenges to the automated segmentation of the liver on CT. Among these is that the attenuation of adjacent organs and tissues that may be very similar to the liver tissue itself. In addition, model-based approaches to segmentation are challenging due to the liver's widely varying shape (30). Also, automated segmentation of small liver tumors showed lower accuracy compared to both manual segmentation and automated segmentation of larger tumors (12, 24). As a result, there have been multiple attempts to develop methods of automated liver segmentation using CT (31, 32).
In our study, we found that A-vRECIST measurements highly correlated with both M-vRECIST and unidimensional mRECIST measurements in large HCCs from patients who had undergone TACE.
Because mRECIST is currently the preferred method of monitoring TACE therapy, we used it as the standard to which vRECIST was compared. Our results showed that, between our experienced radiologists, there was moderate to high inter-reader agreement for monitoring therapy using mRECIST measurements (ICC = 0.824). Our study also showed that correlation between unidimensional mRECIST and the vRECIST measurements was good r = 0.766 for M-vRECIST and r = 0.774 for A-vRECIST.
Results of our vRECIST measurements are likely to provide an early marker for TACE monitoring (23, 28, 32). We found that A-vRECIST measurements made using our neural network model could be a good substitute for M-vRECIST measurements and mRECIST (Figure 5). It also can improve the workflow as an alternate measure of response assessment because the measurements were highly correlated to each other in the baseline study, follow-up study, and volume change results (r = 0.967, 0.937, and 0.826, respectively).
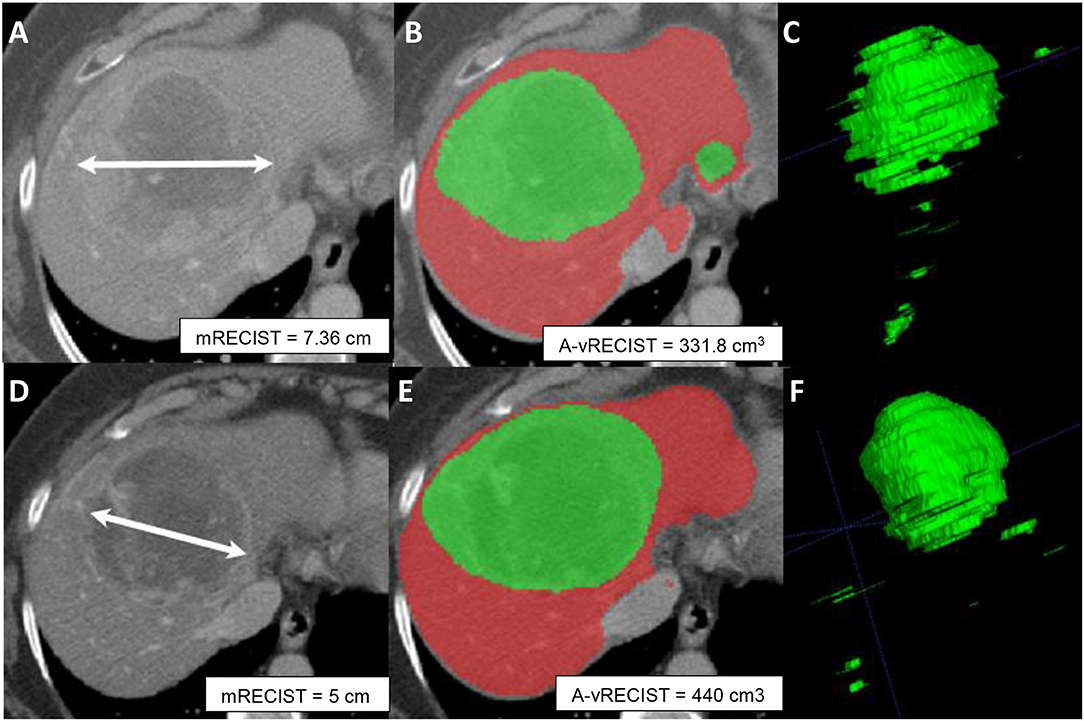
Figure 5. Axial sections from pre-TACE (A–C) and 4-week post-TACE (D–F) scans from a 70-year old male patient with advanced HCC. (A,D) Show changes in tumor size made with mRECIST measurements and indicative of tumor shrinkage. (B,E) Show A-vRECIST segmentation of the hepatic parenchyma (red) and the HCC tissue (green) and indicate tumor size increase. (C,F) Show the 3-dimensional voxel renderings of the A-vRECIST measurements.
Our study had some limitations. First, the small cohort (42 patients) may have masked variability in the automated segmentation results. However, this study was a pilot, and we were aware from the outset that its findings would need to be confirmed prospectively in a larger population with more variable tumor sizes and stages. Second, we did not examine correlation between A-vRECIST and patient's outcome. However, this study serves as a step for further evaluation of clinical importance of A-vRECIST and its relation to patient's survival endpoints. The small differences observed between A-vRECIST and M-vRECIST in the follow-up images (r = 0.648 vs. 0.748, respectively) may have been due to differences in TACE techniques, as the Lipiodol used in conventional TACE can distort CNNs, leading to differences in automated tumor segmentation.
Our next step is to confirm our findings with a larger sample size offering higher variability in tumor sizes and stages. We plan to use A-vRECIST results to classify patients according to their responses to TACE (partial response vs. no response). Also, we will thoroughly study the confounding factors, such as chronic parenchymal liver disease, that may affect the performance of neural networks.
Data Availability Statement
The datasets generated for this study are available on request to the corresponding author.
Ethics Statement
The study was approved by the Institutional Review Board (IRB) at MD Anderson Cancer Center, office of protocol research. The informed consent was waived and approved by our institutional IRB.
Author Contributions
AM contributed to the planning of the project, data collection, curation, data analysis, preparing figures, and write the first draft of the manuscript. KB helped in data curation, planning of the project, and reviewed the final draft of manuscript. AK contributed to the planning of the project, data collection, and shared in writing the first draft of the manuscript. DF and JH technical leaders of the project, construction of the neural network we used, planning of the project, and reviewing the first draft of the project. JS, AQ, and KE three radiologists who read the RECIST of the tumors, helped in planning the project, and also reviewed the final draft of the manuscript. KE provided supervision, support, conceptualization, and guidance throughout the project.
Funding
This article was submitted based on an invitation by the top editor Chunxiao Guo, we are invited to submit this in Frontiers in Oncology-Cancer Imaging and Image-directed Interventions under the special topic Novel Methods for Oncologic Imaging Analysis: Radiomics, Machine Learning, and Artificial Intelligence.
Conflict of Interest
The authors declare that the research was conducted in the absence of any commercial or financial relationships that could be construed as a potential conflict of interest.
Acknowledgments
I would like to acknowledge Scientific Publications Service, Research Medical Library, MD Anderson Cancer Center for their help in editing this article.
Abbreviations
A-vRECIST, automated volumetric response evaluation criteria in solid tumors; CNN, convolutional neural network; CT, computed tomography; HCC, hepatocellular carcinoma; ICC, intra-class correlation coefficient; mRECIST, modified response evaluation criteria in solid tumors; MRI, magnetic resonance imaging; M-vRECIST, manual volumetric response evaluation criteria in solid tumors; RECIST, response evaluation criteria in solid tumors; TACE, transarterial chemo embolization; TNM, tumor-node-metastasis; vRECIST, volumetric response evaluation criteria in solid tumors.
References
1. Pesapane F, Nezami N, Patella F, Geschwind JF. New concepts in embolotherapy of HCC. Med Oncol. (2017) 34:58. doi: 10.1007/s12032-017-0917-2
2. Forner A, Hessheimer AJ, Isabel Real M, Bruix J. Treatment of hepatocellular carcinoma. Crit Rev Oncol Hematol. (2006) 60:89–98. doi: 10.1016/j.critrevonc.2006.06.001
4. Facciorusso A, Serviddio G, Muscatiello N. Local ablative treatments for hepatocellular carcinoma: an updated review. World J Gastrointest Pharmacol Ther. (2016) 7:477–89. doi: 10.4292/wjgpt.v7.i4.477
5. Keating GM. Sorafenib: a review in hepatocellular carcinoma. Target Oncol. (2017) 12:243–53. doi: 10.1007/s11523-017-0484-7
6. Heimbach JK, Kulik LM, Finn RS, Sirlin CB, Abecassis MM, Roberts LR, et al. AASLD guidelines for the treatment of hepatocellular carcinoma. Hepatology. (2018) 67:358–80. doi: 10.1002/hep.29086
7. Shah SR, Riordan SM, Karani J, Williams R. Tumour ablation and hepatic decompensation rates in multi-agent chemoembolization of hepatocellular carcinoma. QJM. (1998) 91:821–8. doi: 10.1093/qjmed/91.12.821
8. Raoul J-L, Forner A, Bolondi L, Cheung TT, Kloeckner R, de Baere T. Updated use of TACE for hepatocellular carcinoma treatment: how and when to use it based on clinical evidence. Cancer Treat Rev. (2019) 72:28–36. doi: 10.1016/j.ctrv.2018.11.002
9. Crocetti L, Bargellini I, Cioni R. Loco-regional treatment of HCC: current status. Clin Radiol. (2017) 72:626–35. doi: 10.1016/j.crad.2017.01.013
10. Prajapati HJ, Kim HS. Treatment algorithm based on the multivariate survival analyses in patients with advanced hepatocellular carcinoma treated with trans-arterial chemoembolization. PLoS ONE. (2017) 12:e0170750. doi: 10.1371/journal.pone.0170750
11. Han K, Kim JH. Transarterial chemoembolization in hepatocellular carcinoma treatment: barcelona clinic liver cancer staging system. World J Gastroenterol. (2015) 21:10327–35. doi: 10.3748/wjg.v21.i36.10327
12. Vorontsov E, Cerny M, Régnier P, Jorio LD, Pal CJ, Lapointe R, et al. Deep learning for automated segmentation of liver lesions at CT in patients with colorectal cancer liver metastases. Radiol Artif Intell. (2019) 1:180014. doi: 10.1148/ryai.2019180014
13. Gruber N, Antholzer S, Jaschke W, Kremser C, Haltmeier M. A joint deep learning approach for automated liver and tumor segmentation. arXiv preprint arXiv:190207971. (2019). doi: 10.1109/SampTA45681.2019.9030909
14. Li X, Chen H, Qi X, Dou Q, Fu C, Heng P. H-DenseUNet: hybrid densely connected UNet for liver and tumor segmentation from CT volumes. IEEE Trans Med Imag. (2018) 37:2663–74. doi: 10.1109/TMI.2018.2845918
15. Yu Q, Shi Y, Sun J, Gao Y, Zhu J, Dai Y. Crossbar-Net: a novel convolutional neural network for kidney tumor segmentation in CT images. IEEE Trans Image Process. (2019) 28:4060–74. doi: 10.1109/TIP.2019.2905537
16. Odland A, Server A, Saxhaug C, Breivik B, Groote R, Vardal J, et al. Volumetric glioma quantification: comparison of manual and semi-automatic tumor segmentation for the quantification of tumor growth. Acta Radiol. (2015) 56:1396–403. doi: 10.1177/0284185114554822
17. van Heeswijk MM, Lambregts DM, van Griethuysen JJ, Oei S, Rao S-X, de Graaff CA, et al. Automated and semiautomated segmentation of rectal tumor volumes on diffusion-weighted MRI: can it replace manual volumetry? Int J Radiat Oncol Biol Phys. (2016) 94:824–31. doi: 10.1016/j.ijrobp.2015.12.017
18. Soltaninejad M, Yang G, Lambrou T, Allinson N, Jones TL, Barrick TR, et al. Automated brain tumour detection and segmentation using superpixel-based extremely randomized trees in FLAIR MRI. Int J Comp Assist Radiol Surg. (2017) 12:183–203. doi: 10.1007/s11548-016-1483-3
19. Kaus MR, Warfield SK, Nabavi A, Black PM, Jolesz FA, Kikinis R. Automated segmentation of MR images of brain tumors. Radiology. (2001) 218:586–91. doi: 10.1148/radiology.218.2.r01fe44586
20. Tu R, Xia L-P, Yu A-L, Wu L. Assessment of hepatic functional reserve by cirrhosis grading and liver volume measurement using CT. World J Gastroenterol. (2007) 13:3956–61. doi: 10.3748/wjg.v13.i29.3956
21. Karademir S. Staging of hepatocellular carcinoma. HPB. (2018) 7:35–41. doi: 10.20517/2394-5079.2018.40
22. Lencioni R, Petruzzi P, Crocetti L. Chemoembolization of hepatocellular carcinoma. Semin Intervent Radiol. (2013) 30:3–11. doi: 10.1055/s-0033-1333648
23. Yushkevich PA, Piven J, Hazlett HC, Smith RG, Ho S, Gee JC, et al. User-guided 3D active contour segmentation of anatomical structures: significantly improved efficiency and reliability. Neuroimage. (2006) 31:1116–28. doi: 10.1016/j.neuroimage.2006.01.015
24. Morshid A, Elsayes KM, Khalaf AM, Elmohr MM, Yu J, Kaseb AO, et al. A machine learning model to predict hepatocellular carcinoma response to transcatheter arterial chemoembolization. Radiol Artif Intell. (2019) 1:e180021. doi: 10.1148/ryai.2019180021
25. Welsh JL, Bodeker K, Fallon E, Bhatia SK, Buatti JM, Cullen JJ. Comparison of response evaluation criteria in solid tumors with volumetric measurements for estimation of tumor burden in pancreatic adenocarcinoma and hepatocellular carcinoma. Am J Surg. (2012) 204:580–5. doi: 10.1016/j.amjsurg.2012.07.007
26. Reynolds AR, Furlan A, Fetzer DT, Sasatomi E, Borhani AA, Heller MT, et al. Infiltrative hepatocellular carcinoma: what radiologists need to know. Radiographics. (2015) 35:371–86. doi: 10.1148/rg.352140114
27. Fuentes D, Ahmed K, Lin JS, Abdel-Wahab R, Kaseb AO, Hassan M, et al. Automated volumetric assessment of hepatocellular carcinoma response to sorafenib: a pilot study. J Comp Assist Tomograp. (2019) 43:499–506. doi: 10.1097/RCT.0000000000000866
28. Chapiro J, Duran R, Lin M, Schernthaner RE, Wang Z, Gorodetski B, et al. Identifying staging markers for hepatocellular carcinoma before transarterial chemoembolization: comparison of three-dimensional quantitative versus non–three-dimensional imaging markers. Radiology. (2014) 275:438–47. doi: 10.1148/radiol.14141180
29. Lin M, Pellerin O, Bhagat N, Rao PP, Loffroy R, Ardon R, et al. Quantitative and volumetric European association for the study of the liver and response evaluation criteria in solid tumors measurements: feasibility of a semiautomated software method to assess tumor response after transcatheter arterial chemoembolization. J Vasc Interv Radiol. (2012) 23:1629–37. doi: 10.1016/j.jvir.2012.08.028
30. Heimann T, Wolf I, Williams T, Meinzer HP. 3D active shape models using gradient descent optimization of description length. In: Christensen GE, Sonka M, editors. Information Processing in Medical Imaging: Proceedings of the Conference. Berlin: Springer (2005) 19:566–77. doi: 10.1007/11505730_47
31. Heimann T, Van Ginneken B, Styner MA, Arzhaeva Y, Aurich V, Bauer C, et al. Comparison and evaluation of methods for liver segmentation from CT datasets. IEEE Trans Med Imag. (2009) 28:1251–65. doi: 10.1109/TMI.2009.2013851
Keywords: volumetric RECIST, hepatocellular carcinoma, TACE, automated segmentation, tumor response
Citation: Moawad AW, Fuentes D, Khalaf AM, Blair KJ, Szklaruk J, Qayyum A, Hazle JD and Elsayes KM (2020) Feasibility of Automated Volumetric Assessment of Large Hepatocellular Carcinomas' Responses to Transarterial Chemoembolization. Front. Oncol. 10:572. doi: 10.3389/fonc.2020.00572
Received: 28 August 2019; Accepted: 30 March 2020;
Published: 07 May 2020.
Edited by:
Lei Deng, Jacobi Medical Center, United StatesReviewed by:
Guolin Ma, China-Japan Friendship Hospital, ChinaYu Jiang, University of Memphis, United States
Su Jong Yu, Seoul National University Hospital, South Korea
Yaojun Zhang, Sun Yat-sen University Cancer Center (SYSUCC), China
Copyright © 2020 Moawad, Fuentes, Khalaf, Blair, Szklaruk, Qayyum, Hazle and Elsayes. This is an open-access article distributed under the terms of the Creative Commons Attribution License (CC BY). The use, distribution or reproduction in other forums is permitted, provided the original author(s) and the copyright owner(s) are credited and that the original publication in this journal is cited, in accordance with accepted academic practice. No use, distribution or reproduction is permitted which does not comply with these terms.
*Correspondence: Khaled M. Elsayes, a21lbHNheWVzJiN4MDAwNDA7bWRhbmRlcnNvbi5vcmc=