- 1Department of Gastric Surgery, Sun Yat-sen University Cancer Center, Guangzhou, China
- 2State Key Laboratory of Oncology in South China and Collaborative Innovation Center for Cancer Medicine, Guangzhou, China
- 3Department of Hematology Oncology, Sun Yat-sen University Cancer Center, Guangzhou, China
- 4Department of Neurosurgery, Sun Yat-sen University Cancer Center, Guangzhou, China
- 5Key Laboratory of Carcinogenesis and Translational Research (Ministry of Education), Department of Gastrointestinal Surgery, Peking University Cancer Hospital and Institute, Beijing, China
Background: Current staging systems are inadequate for evaluating the prognosis of patients with locally advanced gastric cancer (LAGC, stages II–III). Therefore, we developed a serum microRNA (miRNA) signature to facilitate individualized management of these patients.
Methods: Using microarray analysis, we analyzed 12 serum specimens based on different prognoses (good survival group, n = 7; poor survival group, n = 5). We identified and confirmed differential expression of these miRNAs using quantitative reverse transcription PCR (qRT-PCR) of serum from 51 patients with LAGC. A three miRNA-based classifier was established as a training set by Cox proportional hazard regression and risk-score analysis. We validated the prognostic accuracy of this model in an internal validation cohort (Sun Yat-Sen University Cancer Center, SYSUCC validation cohort, n = 50) and an external independent cohort (Beijing Cancer Hospital, BJCH cohort, n = 67).
Results: Three miRNAs were found to be associated with survival of LAGC (P < 0.001 for miR-132, P = 0.011 for miR-548a-3p, and P < 0.001 for miR-1826). A three-miRNA signature was developed for the training set, and a significant difference was found between the survival of low- and high-risk score patients (P < 0.01). The combination of the miRNA signature and tumor–node–metastasis (TNM) stage exhibited superior discrimination. Consistent results were obtained by further validation of the internal set and the BJCH set, which confirmed the predictive value of the model.
Conclusions: We built an easy-to-use prognostic signature using three serum miRNAs as markers. Our miRNA signature may improve postoperative risk stratification and serve as a complement to the TNM staging system.
Introduction
Despite the declining incidence of gastric cancer (GC), it remains the second leading cause of cancer-related death in the world (1, 2). For locally advanced GC (LAGC, stages II–III), gastrectomy with D2 lymphadenectomy combined with adjuvant chemotherapy is the standard treatment, especially in Asia (3). Recently, several studies of neoadjuvant chemotherapy/chemoradiotherapy have reported promising results for LAGC patients, who have a high risk of metastasis (3). However, many patients still exhibit very poor prognosis (3). Given that conventional assessment approaches do not clearly distinguish between patients with a high or low risk of metastasis, it is a major challenge to identify more effective survival prediction methods.
Advances in microRNA (miRNA) expression profiling provide probabilities for tumor prognosis prediction and treatment design. Extensive studies have reported that miRNAs are involved in tumor development, differentiation, and pathogenesis (4–7). It has been suggested that miRNA profiles may be a good alternative to expression profiles of protein-coding genes in tumor classification and prediction (8). Moreover, a model integrating multiple biomarkers could improve predictive efficiency (9, 10). Therefore, miRNA expression profiling could provide more accurate biological information compared with protein-coding gene profiling (11, 12).
In this study, we aimed to develop a reliable, non-invasive serum miRNA signature with prognostic value for LAGC patients.
Materials and Methods
Serum Patients and Tumor RNA Samples
Between January 2002 and October 2008, 113 patients with LAGC at the Cancer Center of Sun Yat-sen University (SYSUCC) (Guangzhou, China) were included in this study. Blood samples from patients who underwent D2-lymphadenectomy were obtained for prognostic miRNA signature establishment and internal validation. Patients with stage II and III GC having complete clinicopathological records were included. Patients were staged according to the 7th American Joint Committee on Cancer (AJCC) tumor–node–metastasis (TNM) staging system (13). None of the patients had received previous treatment with any anticancer therapy prior to surgery. Twelve cases were selected for miRNA microarray analysis. Seven patients who had survival times >60 months (average, 76 months; range, 65–94 months) were identified as the good survival group, whereas five patients who survived <25 months (average, 7 months; range, 3–12 months) were identified as the poor survival group. The remaining 101 cases were randomly classified as either training (n = 51) or testing (n = 50) sets using computer-generated random numbers. For further external validation, another independent cohort (n = 67) from Beijing Cancer Hospital (BJCH) (Beijing, China) was enrolled between November 1, 2008 and May 1, 2010. The process of the study is shown in the flow chart (Figure S5). Pathological diagnosis of GCs was confirmed by at least two professional pathologists. Ethical approval was obtained from both Sun Yat-sen University Cancer Center and Beijing Cancer Hospital research ethics committees.
MiRNA Expression Profiling
Microarray was performed on 12 serum samples miRNA expression profile by CapitalBio (CapitalBioCorp). Procedures are described in detail on the CapitalBio website (http://www.capitalbio.com). Briefly, procedures included total RNA extraction, sample quality control, miRNA isolation using MirVana miRNA isolation Kit (Ambion), FlashTagTM Biotin Labeling of miRNAs (Genisphere), hybridization to Affymetrix GeneChip miRNA microarray (Affymetrix), microarray washing, staining, and scanning. When miRNA expression changed by at least ±2-fold and P < 0.05, miRNAs were considered significantly differentially expressed and selected for quantitative PCR (qPCR) validation. Clustering analysis was performed by Cluster 3.0-based primarily on fold change >1.50 (14).
qRT-PCR Analysis of miRNAs
Quantitative reverse transcription PCR (qRT-PCR) was performed on selected miRNA candidates to validate miRNA array results. Total RNA was extracted from 200 μl of serum using TRIzol LS reagent. Briefly, 600 μl of TRIzol® LS was added into 200 μl of serum. Samples were mixed with a pipette and incubated for 5 min at room temperature. Chloroform (160 μl) was added to samples and shaken vigorously by hand for 15 s, followed by incubation for 10 min at room temperature. Mixtures were centrifuged at 12,000 g for 15 min at 4°C, and 500 μl of the upper aqueous phase was placed into a new tube. An equal volume of 100% isopropanol was added to the aqueous phase and incubated at room temperature for 10 min, followed by centrifugation at 12,000 g for 10 min at 4°C to pellet the RNA. The supernatant was discarded, and the RNA pellets were washed with 1 ml 75% ethanol diluted in RNase-free H2O. The RNA pellet was vortexed and centrifuged at 7,500 g for 5 min at 4°C, dried for 10 min at room temperature, and dissolved in 20 μl of RNase-free H2O.
The expression levels of miRNAs were normalized and quantified to the small nuclear U6 RNA, which was used as an internal control as previously reported (15). After the reaction, cycle threshold (CT) values were determined based on fixed threshold settings. To calculate the expression levels of miRNA, the standard curve of each miRNA was prepared. All reactions, including no-template controls, were run in triplicate. Data were analyzed using the 2−ΔΔCt method. Primer sequences of the miRNAs are shown in Table S2.
Statistical Analysis
The primary set of patients (n = 101) was randomly assigned as either training set (n = 51) or testing set (n = 50). In the training set, we used univariate and multivariate Cox regression analysis to evaluate the association between expression levels of miRNAs and survival (16). MiRNAs with hazard ratio (HR) for death <1 were defined as protective miRNAs, while those HRs >1 were considered risk miRNAs. For miRNAs that significantly correlated with survival, we assigned each patient a risk score calculated by a liner combination of the expression level of the miRNA weighted by the regression coefficient (17). To further test the predictive value of the risk score, the same algorithm was validated in the testing and the BJCH sets.
Comparisons between two groups were completed using t test for continuous variables and chi-square test for categorial variables. The Kaplan–Meier method was used for the analysis between variables and survival, and log-rank test was used for comparing survival curves. Cox regression models were used for univariable and multivariable survival analysis and Cox regression coefficients for generating the risk score classifier. Akaike's information criterion (AIC) method and receiver operating characteristic (ROC) curves were used for evaluating prognostic or predictive accuracy. Higher area under the curve (AUC) values and lower AIC values indicate better discrimination. All statistical analyses were performed using the software statistical package for social sciences version 20.0 (SPSS, Chicago, IL). Results were considered statistically significant when P < 0.05.
MiRNA-Targeted Gene Prediction and Signal Pathway Analysis
To identify possible target genes of these three differently expressed miRNAs, we integrated the predicted genes from TargetScan (http://www.targetscan.org), microRNAdb (http://bioinfo.au.tsinghua.edu.cn/micrornadb/index.php), miTARbase (http://mirtarbase.mbc.nctu.edu.tw/php/index.php), and miRPathDB (https://omictools.com/mirna-pathway-dictionary-database-tool). The online function annotation tool DAVID (https://david.ncifcrf.gov/summary.jsp) was used to annotate the molecular function of target genes and signaling pathways with which they are involved.
Results
Expression Profiles of MicroRNAs
To identify differentially expressed microRNAs between the good and poor survival groups, microarrays of 12 patients' serum specimens were performed to detect relative expression levels of miRNAs (Table S1). We identified two microRNAs that were upregulated more than 2-fold (miR-1826 and miR-132) in the good survival group compared with three miRNAs (miR-548a-3p, miR-638, and miR-1184) that were downregulated more than 2-fold in the good survival group (Table 1). Hierarchical clustering analysis using microRNA fold change >1.50 showed distinct expression patterns between the two groups (Figure 1).
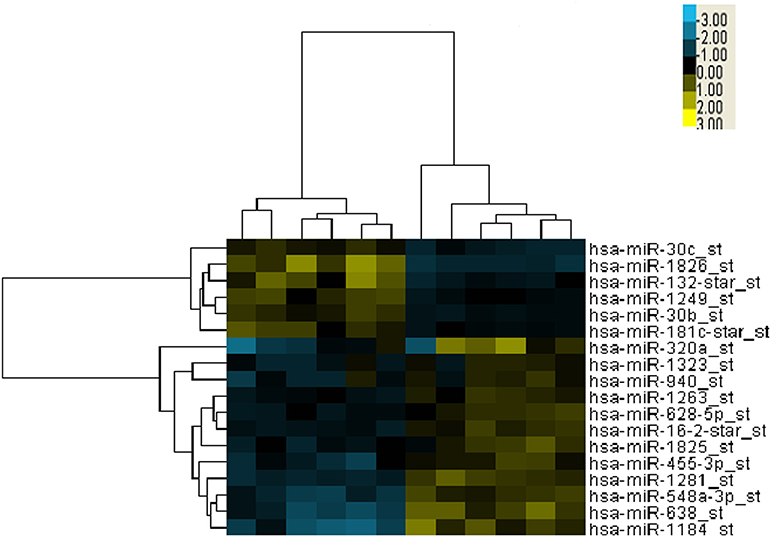
Figure 1. Cluster analysis of expressed microRNAs (miRNAs) in 12 selected locally advanced gastric cancer (LAGC) serum samples. Five miRNAs were identified as dysregulated, including two upregulated in the good survival group and three downregulated in the poor survival group. The criteria of fold change >1.5 was used. Columns represent the samples, and rows represent the miRNAs (blue, black, and yellow represents downregulated, unchanged, and upregulated, respectively).
Three Serum MicroRNA Classifiers and Survival
To further examine if these microRNAs can be used for outcome prediction, the primary set was randomly assigned to training and testing samples to identify prognostic factors. There were no significant differences in clinicopathological features between the two sets (all P > 0.05). The characteristics of the training, testing, and BJCH sets are summarized in Table 2. To explore the relationship between miRNAs and overall survival, we used the Youden index method to generate optimal cutoff points for the five candidate miRNAs in the training data set (18). Three miRNAs (miR-1826, miR-132, and miR-548a-3p) from these five miRNAs were identified by Cox proportional hazard regression. Expression levels of the three miRNAs were significantly associated with patient survival (miR-1826: 95%CI, 0.121–0.529, P < 0.001; miR-132: 95%CI, 0.118–0.517, P < 0.001; and miR-548a-3p: 95%CI, 1.233–5.069, P = 0.011). High expression levels of miR-548a-3p (median, 314) or low expression levels of miR-1826 (median, 1,416) and miR-132 (median, 5.9) predicted unfavorable survival. Differences in median survival with respect to different expression levels of these three miRNAs were statistically significant (P < 0.001 for miR-132, P = 0.008 for miR-548a-3p, and P < 0.001 for miR-1826) (Table 3). Interestingly, similar conclusions were reached in the testing, primary, and BJCH sets when the same threshold values were applied (data not shown). Multivariate Cox regression was then used to build a prognostic model using the three miRNAs selected in the training set. A formula was then derived to calculate the risk score for each patient's overall survival according to the expression levels of these three miRNAs. Based on individual regression coefficients, a risk score model for outcome prediction was built as follows: risk score = (2.057*miR-548a-3p) + (−0.364*expression level of miR-132 expression level) + (−0.350*expression level of miR-1826). In this formula, the plus sign equals a risk factor, and the minus sign equals a protective factor. A higher risk score indicates worse overall survival.
Three Serum MicroRNA Classifiers Predict LAGC Survival
To validate whether the three miRNA classifiers could predict survival of LAGC patients, we classified patients into good or poor survival groups using the median risk score as the cutoff point. Kaplan–Meier analysis and log-rank test indicated that the two groups had significantly different overall survival. The low-risk score group exhibited better survival compared with the high-risk score group (median survival time, 80.00 vs. 7.78 months, respectively) (P < 0.01) (Figure 2A). To further validate whether this classifier was applicable to other cohorts, we analyzed the survival of the testing, primary, and BJCH sets, and the results were consistent with those of the training set. Indeed, high-risk score values increased the possibility of shortened survival more than the low-risk score values (median survival: low- vs. high-risk score groups are 70.00 vs. 12.00 months for the testing set, 70.00 vs. 11.15 months for the primary set, and 50.85 vs. 13.56 months for the BJCH set) (P < 0.05) (Figures 2B–D). Furthermore, elevated HRs indicated worse survival referred to a higher risk score (5.00 for the training set, 10.45 for the testing set, 7.73 for the primary set, and 2.28 for the BJCH set) (Table 4).
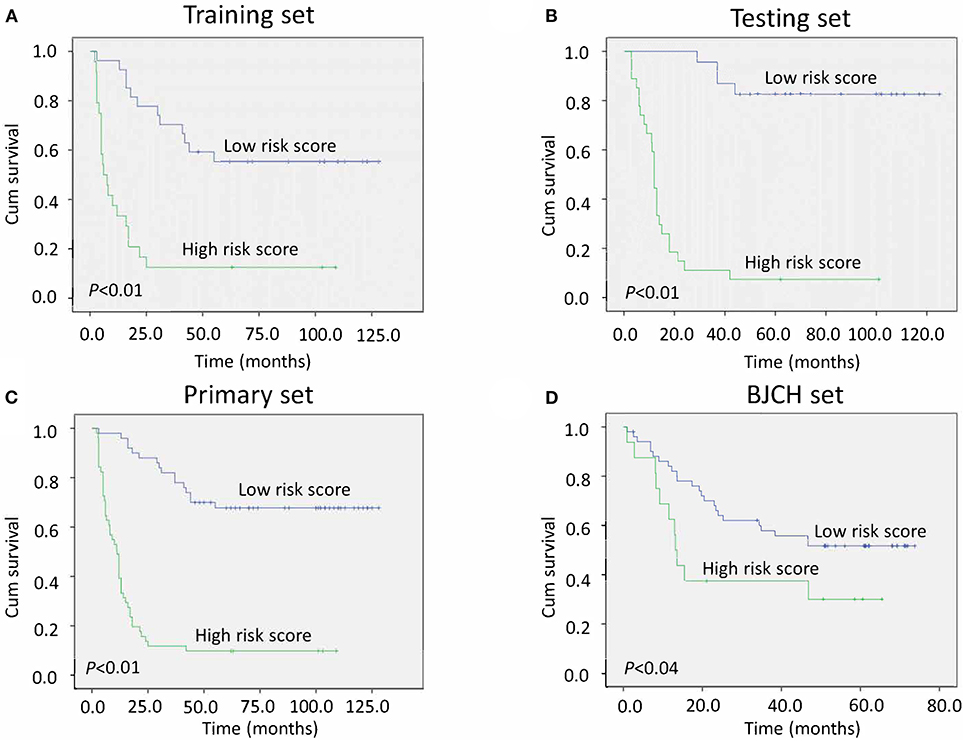
Figure 2. Kaplan–Meier curves of gastric cancer (GC) patients indicate the relationship between risk score and clinical outcome. (A) Survival curves of low-risk scores compared with high-risk scores in the training set. (B) Survival curves of low-risk scores compared with high-risk scores in the testing set. (C) Survival curves of low-risk scores compared with high-risk scores in the primary set. (D) Survival curves of low-risk scores compared with high-risk scores in the Beijing Cancer Hospital (BJCH) set.
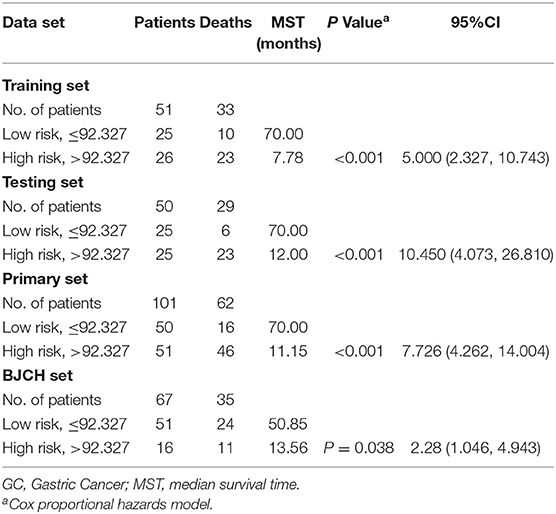
Table 4. Risk score and survival of GC patients in training set, testing set, primary cohort, and BJCH set.
To verify whether this classifier was more specific to discriminate LAGC than other combinations, we combined miRNAs into classifier A (miR-132 and miR-1826), classifier B (miR-132 and miR-548a-3p), classifier C (miR-548a-3p and miR-1826), and classifier D (miR-132, miR-548a-3p and miR-1826) groups. Then, these four classifiers were applied to training, testing, and primary sets. In the training set, classifier D significantly distinguished the good and poor survival groups (P < 0.001) and increased the AUC to 0.830, which was higher than the other three classifiers. The specificity was 0.833, and sensitivity was 0.727. Similar results were found in the testing/primary sets. In total, our classifier increased the specificity and sensitivity more so than either other combined classifiers or individual miRNAs, exhibiting the highest AUC value (Table 5, Figure 3).
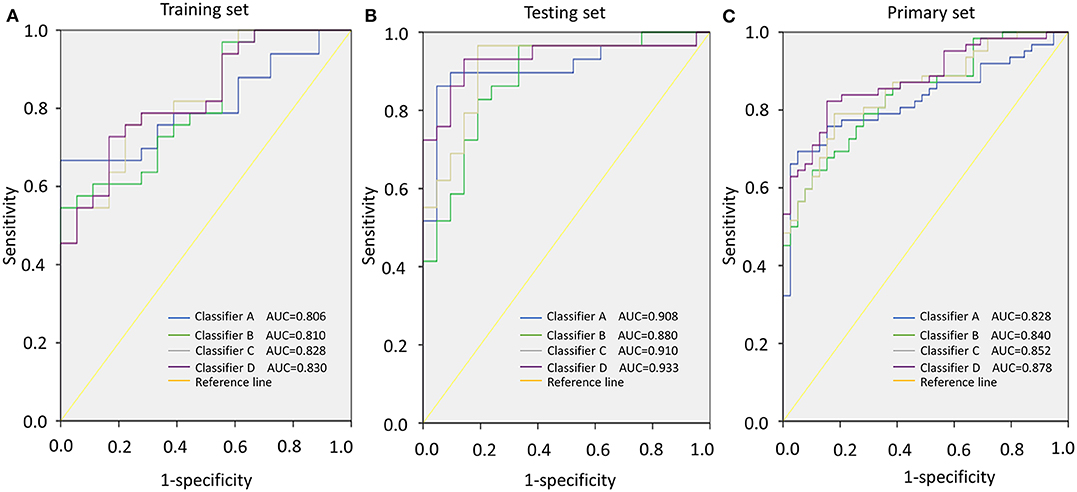
Figure 3. Area under the curve (AUC) according to different classifiers (classifiers A–D). (A) AUCs of classifiers A–D in the training set. (B) AUCs of classifiers A–D in the testing set. (C) AUCs of classifiers A–D in the primary set.
Three Serum MicroRNA Classifiers and TNM Staging
After adjustment for clinicopathological variables, the risk score and TNM stage remained powerful and independent prognostic indicators of overall survival in the primary set (P < 0.01 for risk score and P = 0.021 for TNM stage). We further stratified patients by risk score in stages II and III. Notably, low-risk score groups exhibited better survival than the high-risk score groups in stage III (all P < 0.05, Figure 4). However, different from stage III, no statistical significance was found between low- and high-risk score groups in either primary or BJCH sets in stage II (P > 0.05, Figure S1). The reason for this may be the small sample size of patients with stage II in this study.
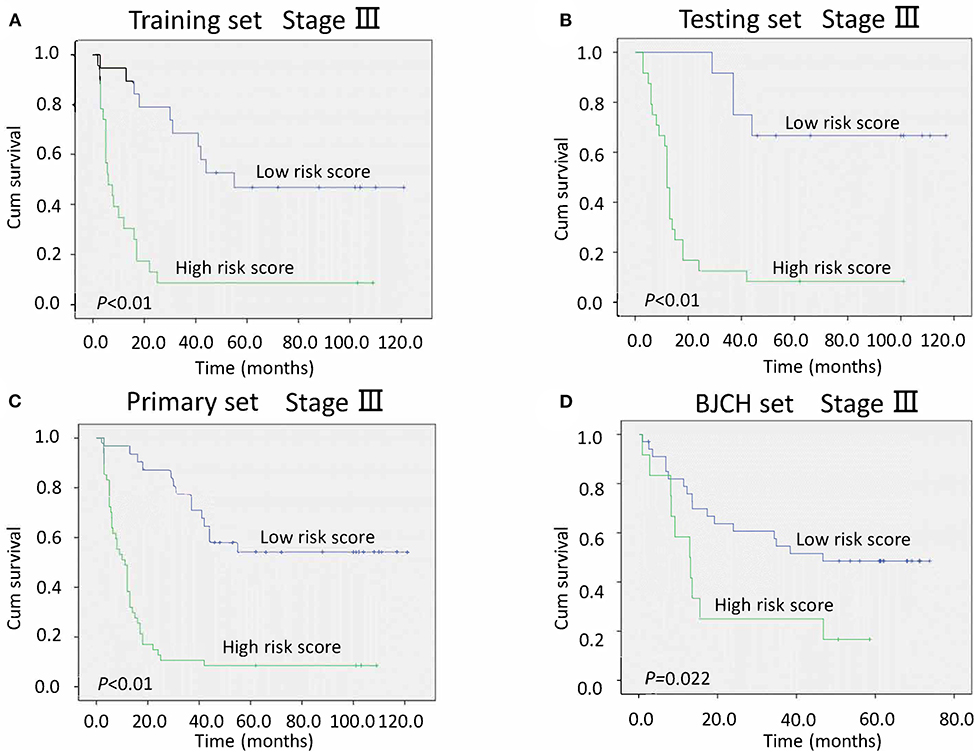
Figure 4. Kaplan–Meier curves of stage III gastric cancer (GC) patients indicate the relationship between risk score and clinical outcome. (A) Survival curves of low-risk scores compared with high-risk scores in the training set. (B) Survival curves of low-risk scores compared with high-risk scores in the testing set. (C) Survival curves of low-risk scores compared with high-risk scores in the primary set. (D) Survival curves of low-risk scores compared with high-risk scores in the Beijing Cancer Hospital (BJCH) set.
Furthermore, we proposed a new stage system combining conventional TNM stage and risk score stage (TNM-RS stage system). Patients with stage II and low-risk scores were defined as TNM-RS 1, those with stage II and high-risk scores were defined as TNM-RS 2, those with stage III and low-risk scores were defined as TNM-RS 3, and those with stage III and high-risk score were defined as TNM-RS 4 (Figures 5A,B). TNM-RS 1 was no different from TNM-RS 2 in overall survival (P > 0.05). Thus, we categorized our patients into three groups: patients in stage II were TNM-RS 1, those in stage III and low-risk scores were defined as TNM-RS 2, and those in stage III and high-risk scores were defined as TNM-RS 3 (Figures 5C,D). TNM stage was also used to classify patients as a reference (Figures 5E,F). As shown in Figures 5C–F, the new proposed TNM-RS stage system demonstrates better discrimination compared to TNM stage alone.
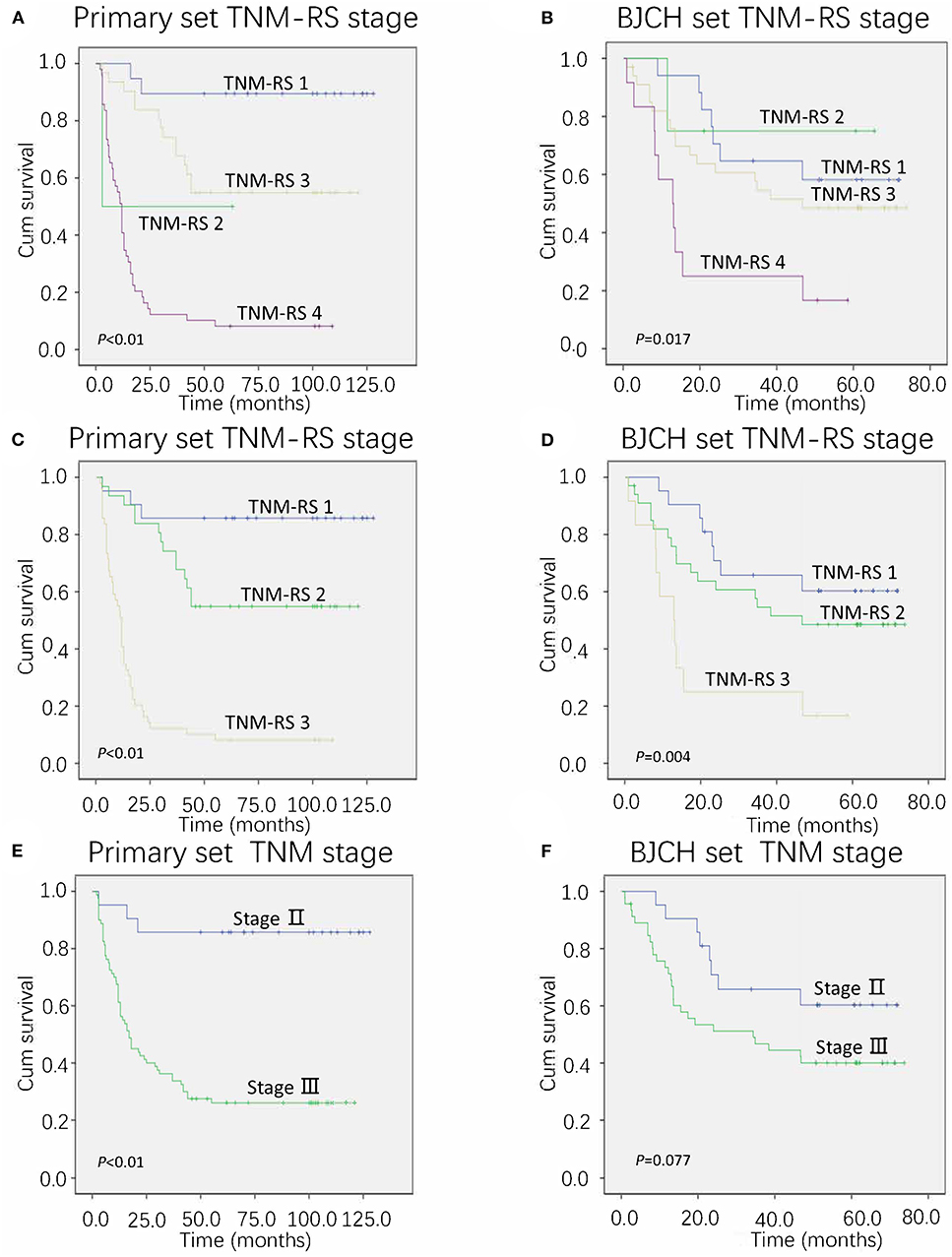
Figure 5. Overall survival of primary and Beijing Cancer Hospital (BJCH) sets according to different staging systems. (A,C) Overall survival of primary set according to tumor–node–metastasis (TNM) and risk score (TNM-RS) staging system. (B,D) Overall survival of BJCH set according to TNM-RS staging system. (E) Overall survival of BJCH set according to the 7th edition of the American Joint Committee on Cancer (AJCC) staging system. (F) Overall survival of BJCH set according to the 7th edition of the AJCC staging system.
To further verify reliability of the TNM-RS stage system, ROCs and AIC methods were performed in both primary and BJCH sets (19). TNM-RS stage system had a higher AUC (primary set, 0.856 vs. 0.707; BJCH, 0.645 vs. 0.589) and smaller AIC index (primary set, 457.2 vs. 504.4; BJCH set, 267.8 vs. 270.8) (Figures 6A,B).
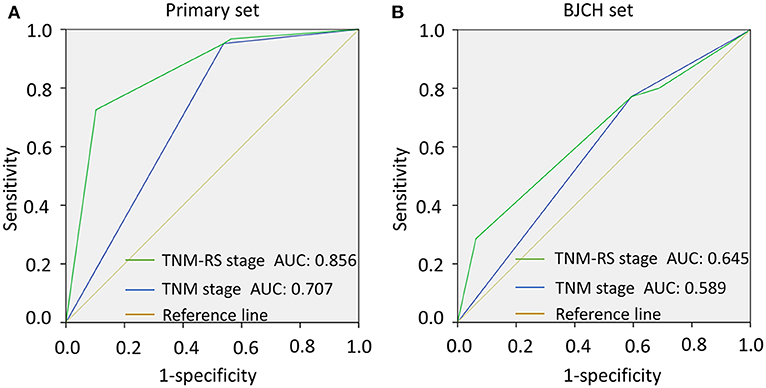
Figure 6. Comparison of area under curve (AUC) according to tumor–node–metastasis (TNM) stage and TNM and risk score (TNM-RS) stage. (A) AUCs of TNM stage and TNM-RS stage in the primary set. (B) AUCs of TNM stage and TNM-RS stage in the Beijing Cancer Hospital (BJCH) set.
Signal Pathway Analysis of Classifier D Targeted Genes
To reveal the possible regulation mechanisms of these three miRNAs in the survival of LAGC patients, we integrated the predicted target genes of the above miRNA-related databases. An overlap of 34 (2%), 881 (9.3%), and 3 (100%) predicted genes were found for miR-132, miR-548a-3p, and miR-1826, respectively (Figure S2). Signal pathway analysis showed that target genes regulated by miR-548a-3p and miR-1826 were both involved in focal adhesion and Wnt pathways. We also found that miR-1826 regulated genes involved in phosphatidylinositol-3-kinase (PI3K)-Akt, Ras, and transforming growth factor beta (TGF-β) pathways. miR-548a-3p regulated genes involved in cell cycle, mitogen-activated protein kinase (MAPK), and Notch pathways. In addition, miR-132 was found to regulate genes involved in positive regulation of protein serine/threonine kinase activity. Gene annotation analysis results showed that genes regulated by these three miRNAs were involved in critical biological process associated with cancers (Table 6 and Figures S3, S4).
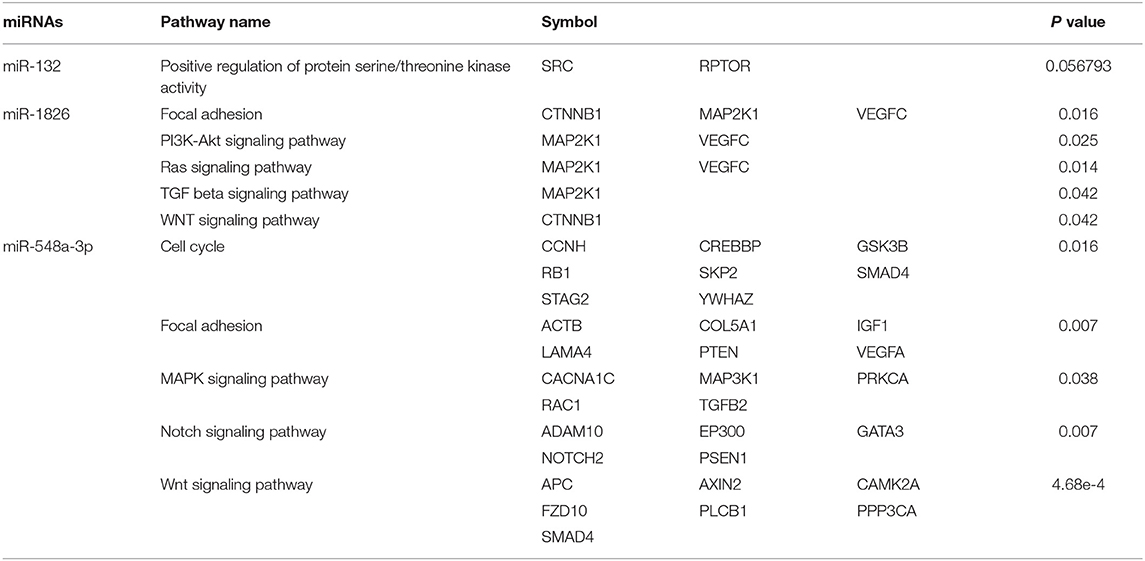
Table 6. Targeted genes regulated by miR-132, miR-1826, and miR-548a-3p that are involved in signal pathways.
Discussion
Compared to a single miRNA, a cluster of biomarkers is a better prognostic tool with much higher sensitivity, specificity, and accuracy. For example, a seven-miRNA signature was identified as a specific biomarker for GC by Li et al. (20). Similarly, Zhang et al. confirmed a predictive value of hsa-miR-375 and hsa-miR-142-5p in recurrence risk (21). However, these miRNAs were from paraffin-embedded tissue blocks and fresh frozen GC tissues, respectively. It is often difficult to acquire desired miRNAs from tissues. Therefore, the nature of invasion, discomfort, and inconvenience limits its application in clinical practice.
Recently, serum miRNAs have been studied extensively compared with conventional tissue miRNAs due to the non-invasiveness of their collection, their stability, and convenience (22, 23). The roles of serum-based miRNA in cancer diagnosis have also been largely demonstrated (22, 23). However, its roles in cancer prognosis have not been adequately evaluated, especially in GC.
In this study, we first established and validated a novel serum signature based on three miRNAs (miR-132, miR-1826, and miR-548a-3p) for LAGC. This model demonstrated better discrimination in survival prediction. Moreover, we revealed that this signature is a powerful and independent prognostic classifier by both internal and external validation.
Previously, expression of miR-132 and miR-1826 in tissue was shown to play a pivotal role in inhibiting cancer progression (24, 25). Conversely, miR-548a-3p was reported to be associated with human lymphoblastoid cell line proliferation and apoptosis as an oncogene (26). These findings are consistent with the currently study. The release mechanism of these miRNAs may be related to microvesicles or exosomes generated by tumors (27, 28).
Furthermore, we established a new staging system (TNM-RS stage) by combining conventional TNM stage and risk score stage. Interestingly, we found that patients with various clinical outcomes were better stratified using these methods. The novel TNM-RS stage exhibited better predictive efficiency than did TNM stage alone. Large variations in clinical outcomes were commonly observed when categorizing patients according to the conventional staging system (29, 30), implying that conventional clinical features are inadequate for prognostic prediction. For the first time, we reveal that a serum miRNA signature has the potential to be a novel complement to the conventional TNM staging system.
Despite their critical role, only a limited number of miRNAs are known to regulate specific target genes (31). The definite gene targets and mechanisms of the classifiers to predict LAGC remain enigmatic. It is reported that downregulation of miR-132 indicates poor survival in colorectal cancer as a result of hypermethylation (32). Similarly, miR-1826 was reported to play an important role as a tumor suppressor through CNTTB1 and MEK1 in von Hippel–Lindau (VHL)-inactivated renal and bladder cancers (33, 34). miR-548a-3p was also found to be associated with protein kinase cascades, lymphocyte proliferation, and apoptosis in human lymphoblastoid cell lines (26). In the current study, we found that these miRNAs might be involved in critical cancer-associated pathways, such as the cell cycle, Wnt, focal adhesion, and Notch. These relative oncogenic mechanisms offer us a new perspective of miRNAs' role in the pathogenesis of LAGC. More integrated investigation of the definitive targets and regulatory mechanisms of miRNAs will help us understand the disease better and guide individualized treatment.
In conclusion, our findings show that a three miRNA-based serum classifier effectively predicts survival in LAGC. This easy-to-use prognostic tool successfully categorizes patients into high- and low-risk groups. Meanwhile, a combination of the miRNA signature and conventional staging system predicts patient prognosis more accurately for LAGC. Given the characteristic of non-invasiveness and easy detection, our serum miRNA signature could further be used in monitoring therapeutic response by tracking dynamic expression profiling and personalized treatment.
Synopsis
Current staging systems based on clinicopathological factors are inadequate in classifying patients with advanced gastric cancer (AGC). In this study, a novel serum-based microRNA signature was established for the survival prediction of the patients with AGC. A combination of the microRNA signature and conventional staging system could predict patients' prognosis more accurately. The more efficient risk stratification for AGC patients underwent surgery was of great importance for more personalized adjuvant treatment subsequently.
Data Availability Statement
The data that support the findings of this study are available on reasonable request from the corresponding authors (Aiwen Wu and Dazhi Xu). The data are not publicly available due to restrictions of informed consent.
Ethics Statement
The studies involving human participants were reviewed and approved by Ethical committees of Sun Yat-sen University Cancer Center. The patients/participants provided their written informed consent to participate in this study.
Author Contributions
DX and AW: substantial contributions to the conception and design. DX, QG, JZ, SC, and JL: development of methodology. SC, JL, QG, and JZ: acquisition of data. SC and JL: analysis and interpretation of data. SC and DX: draft of the article. All authors are critical revision of the article and final approval of the version.
Conflict of Interest
The authors declare that the research was conducted in the absence of any commercial or financial relationships that could be construed as a potential conflict of interest.
Acknowledgments
We thank all the participants who were involved in this study.
Supplementary Material
The Supplementary Material for this article can be found online at: https://www.frontiersin.org/articles/10.3389/fonc.2020.00565/full#supplementary-material
Figure S1. Kaplan-Meier curves of stage II GC patients indicate the relationship between risk score and clinical outcome. (A) Survival curves of low-risk scores compared with high-risk scores in the primary set. (B) Survival curves of low-risk scores compared with high-risk scores in the BJCH set.
Figure S2. Venn diagrams of the number of target genes regulated by miR-132, miR-1826, and miR-548a-3p in databases TargetScan, microRNAdb, miTARbase, and miRPathDB.
Figure S3. Molecular signal pathway networks consisted of target genes that are regulated by miR-132 and miR-1826.
Figure S4. Molecular signal pathway networks consisted of target genes that are regulated by miR-548a-3p.
Figure S5. The flow chart illustrating the process of this study.
Table S1. Clinicalpathological features of the 12 cases for discovery. AJCC, American Joint Committee on Cancer; TNM, Tumor-Node-Metastasis; OS, Overall survival.
Table S2. Sequence of primers.
References
1. De Santis CE, Lin CC, Mariotto AB, Siegel RL, Stein KD, Kramer JL, et al. Cancer treatment and survivorship statistics, 2014. CA Cancer J Clin. (2014) 64:252–71. doi: 10.3322/caac.21235
2. Ferlay J, Soerjomataram I, Dikshit R, Eser S, Mathers C, Rebelo M, et al. Cancer incidence and mortality worldwide: sources, methods and major patterns in GLOBOCAN 2012. Int J Cancer. (2015) 136:E359–86. doi: 10.1002/ijc.29210
3. Hundahl SA, Phillips JL, Menck HR. The national cancer data base report on poor survival of U.S. gastric carcinoma patients treated with gastrectomy: fifth edition american joint committee on cancer staging, proximal disease, and the “different disease” hypothesis. Cancer. (2000) 88:921–32. doi: 10.1002/(SICI)1097-0142(20000215)88:4<921::AID-CNCR24>3.0.CO;2-S
4. He Y, Lin J, Kong D, Huang M, Xu C, Kim TK, et al. Current state of circulating microRNAs as cancer biomarkers. Clin Chem. (2015) 61:1138–55. doi: 10.1373/clinchem.2015.241190
5. Zhao XM, Liu KQ, Zhu G, He F, Duval B, Richer JM, et al. Identifying cancer-related microRNAs based on gene expression data. Bioinformatics. (2015) 31:1226–34. doi: 10.1093/bioinformatics/btu811
6. Shin VY, Chu KM. MiRNA as potential biomarkers and therapeutic targets for gastric cancer. World J Gastroenterol. (2014) 20:10432–9. doi: 10.3748/wjg.v20.i30.10432
7. Esquela-Kerscher A, Slack F. Oncomirs - microRNAs with a role in cancer. Nat Rev Cancer. (2006) 6:259–69. doi: 10.1038/nrc1840
8. Roldo C, Missiaglia E, Hagan JP, Falconi M, Capelli P, Bersani S, et al. MicroRNA expression abnormalities in pancreatic endocrine and acinar tumors are associated with distinctive pathologic features and clinical behavior. J Clin Oncol. (2006) 24:4677–84. doi: 10.1200/JCO.2005.05.5194
9. Halabi S, Lin C, Small E, Armstrong A, Kaplan E, Petrylak D, et al. Prognostic model predicting metastatic castration-resistant prostate cancer survival in men treated with second-line chemotherapy. J Natl Cancer Inst. (2013) 105:1729–37. doi: 10.1093/jnci/djt280
10. Zhang J, Song W, Chen Z, Wei J, Liao Y, Lei J, et al. Prognostic and predictive value of a microRNA signature in stage II colon cancer: a microRNA expression analysis. Lancet Oncol. (2013) 14:1295–306. doi: 10.1016/S1470-2045(13)70491-1
11. Calin GA, Croce CM. MicroRNA signatures in human cancers. Nat Rev Cancer. (2006) 6:857–66. doi: 10.1038/nrc1997
12. Lu J, Getz G, Miska EA, Alvarez-Saavedra E, Lamb J, Peck D, et al. MicroRNA expression profiles classify human cancers. Nature. (2005) 435:834–8. doi: 10.1038/nature03702
13. Washington K. 7th edition of the AJCC cancer staging manual: stomach. Ann Surg Oncol. (2010) 17:3077–9. doi: 10.1245/s10434-010-1362-z
14. Yang Y, Huang N, Hao L, Kong W. A clustering-based approach for efficient identification of microRNA combinatorial biomarkers. BMC Genomics. (2017) 18(Suppl. 2):210. doi: 10.1186/s12864-017-3498-8
15. Jiang J, Lee EJ, Gusev Y, Schmittgen TD. Real-time expression profiling of microRNA precursors in human cancer cell lines. Nucleic Acids Res. (2005) 33:5394–403. doi: 10.1093/nar/gki863
16. Lachin JM. Sample size evaluation for a multiply matched case-control study using the score test from a conditional logistic (discrete Cox PH) regression model. Stat Med. (2008) 27:2509–23. doi: 10.1002/sim.3057
17. Lossos IS, Czerwinski DK, Alizadeh AA, Wechser MA, Tibshirani R, Botstein D, et al. Prediction of survival in diffuse large-B-cell lymphoma based on the expression of six genes. N Engl J Med. (2004) 350:1828–37. doi: 10.1056/NEJMoa032520
18. Youden WJ. Index for rating diagnostic tests. Cancer. (1950) 3:32–5. doi: 10.1002/1097-0142(1950)3:1<32::AID-CNCR2820030106>3.0.CO;2-3
19. Harrell F, Califf R, Pryor D, Lee K, Rosati R. Evaluating the yield of medical tests. JAMA. (1982) 247:2543–6. doi: 10.1001/jama.247.18.2543
20. Li X, Zhang Y, Zhang Y, Ding J, Wu K, Fan D. Survival prediction of gastric cancer by a seven-microRNA signature. Gut. (2010) 59:579–85. doi: 10.1136/gut.2008.175497
21. Zhang X, Yan Z, Zhang J, Gong L, Li W, Cui J, et al. Combination of hsa-miR-375 and hsa-miR-142-5p as a predictor for recurrence risk in gastric cancer patients following surgical resection. Ann Oncol. (2011) 22:2257–66. doi: 10.1093/annonc/mdq758
22. Chen X, Ba Y, Ma L, Cai X, Yin Y, Wang K, et al. Characterization of microRNAs in serum: a novel class of biomarkers for diagnosis of cancer and other diseases. Cell Res. (2008) 18:997–1006. doi: 10.1038/cr.2008.282
23. Mitchell PS, Parkin RK, Kroh EM, Fritz BR, Wyman SK, Pogosova-Agadjanyan EL, et al. Circulating microRNAs as stable blood-based markers for cancer detection. Proc Natl Acad Sci USA. (2008) 105:10513–8. doi: 10.1073/pnas.0804549105
24. Liu F, Cheng Z, Li X, Li Y, Zhang H, Li J, et al. A novel pak1/ATF2/miR-132 signaling axis is involved in the hematogenous metastasis of gastric cancer cells. Mol Ther Nucleic Acids. (2017) 8:370–82. doi: 10.1016/j.omtn.2017.07.005
25. Zhang T, Liu C, Huang S, Ma Y, Fang J, Chen Y. A downmodulated microRNA profiling in patients with gastric cancer. (2017) 2017:1526981. doi: 10.1155/2017/1526981
26. Shim SM, Jung SY, Nam HY, Kim HR, Lee MH, Kim JW, et al. Network signatures of cellular immortalization in human lymphoblastoid cell lines. Biochem Biophys Res Commun. (2013) 441:438–46. doi: 10.1016/j.bbrc.2013.10.081
27. Skog J, Wurdinger T, van Rijn S, Meijer DH, Gainche L, Sena-Esteves M, et al. Glioblastoma microvesicles transport RNA and proteins that promote tumour growth and provide diagnostic biomarkers. Nat Cell Biol. (2008) 10:1470–6. doi: 10.1038/ncb1800
28. Valadi H, Ekstrom K, Bossios A, Sjostrand M, Lee JJ, Lotvall JO. Exosome-mediated transfer of mRNAs and microRNAs is a novel mechanism of genetic exchange between cells. Nat Cell Biol. (2007) 9:654–9. doi: 10.1038/ncb1596
29. Noh S, Park S, Yang H, Chung H, Chung I, Kim S, et al. Adjuvant capecitabine plus oxaliplatin for gastric cancer after D2 gastrectomy (CLASSIC): 5-year follow-up of an open-label, randomised phase 3 trial. Lancet Oncol. (2014) 15:1389–96. doi: 10.1016/S1470-2045(14)70473-5
30. Bang Y, Kim Y, Yang H, Chung H, Park Y, Lee K, et al. Adjuvant capecitabine and oxaliplatin for gastric cancer after D2 gastrectomy (CLASSIC): a phase 3 open-label, randomised controlled trial. Lancet. (2012) 379:315–21. doi: 10.1016/S0140-6736(11)61873-4
31. Rosenfeld N, Aharonov R, Meiri E, Rosenwald S, Spector Y, Zepeniuk M, et al. MicroRNAs accurately identify cancer tissue origin. Nat Biotechnol. (2008) 26:462–9. doi: 10.1038/nbt1392
32. Qin J, Ke J, Xu J, Wang F, Zhou Y, Jiang Y, et al. Downregulation of microRNA-132 by DNA hypermethylation is associated with cell invasion in colorectal cancer. Onco Targets Ther. (2015) 8:3639–48. doi: 10.2147/OTT.S91560
33. Hirata H, Hinoda Y, Ueno K, Nakajima K, Ishii N, Dahiya R. MicroRNA-1826 directly targets beta-catenin (CTNNB1) and MEK1 (MAP2K1) in VHL-inactivated renal cancer. Carcinogenesis. (2012) 33:501–8. doi: 10.1093/carcin/bgr302
Keywords: microRNA, survival, advanced gastric cancer, serum, prognosis
Citation: Chen S, Lao J, Geng Q, Zhang J, Wu A and Xu D (2020) A 3-MicroRNA Signature Identified From Serum Predicts Clinical Outcome of the Locally Advanced Gastric Cancer. Front. Oncol. 10:565. doi: 10.3389/fonc.2020.00565
Received: 14 January 2020; Accepted: 30 March 2020;
Published: 19 June 2020.
Edited by:
Xueqing Yao, South China University of Technology, ChinaReviewed by:
Xuelian Xu, The First Affiliated Hospital of Xinxiang Medical University, ChinaBaitang Ning, United States Food and Drug Administration, United States
Copyright © 2020 Chen, Lao, Geng, Zhang, Wu and Xu. This is an open-access article distributed under the terms of the Creative Commons Attribution License (CC BY). The use, distribution or reproduction in other forums is permitted, provided the original author(s) and the copyright owner(s) are credited and that the original publication in this journal is cited, in accordance with accepted academic practice. No use, distribution or reproduction is permitted which does not comply with these terms.
*Correspondence: Aiwen Wu, draiwenwu@outlook.com; Dazhi Xu, xudzh@sysucc.org.cn
†These authors have contributed equally to this work