- 1Department of Breast and Thyroid Surgery, Renmin Hospital of Wuhan University, Wuhan, China
- 2Department of Neurosurgery, Renmin Hospital of Wuhan University, Wuhan, China
- 3Brain Tumor Clinical Center of Wuhan, Wuhan, China
- 4Faculty of Medicine, University of Paris Sud-Saclay, Le Kremlin-Bicêtre, France
- 5Department of Clinical Laboratory, Renmin Hospital of Wuhan University, Wuhan, China
The tumor microenvironment (TME) is a heterogeneous system that contributes to breast cancer progression. The Cancer Genome Atlas (TCGA) database provides global gene expression profiling data for further analysis of various malignancies, including breast cancer. Based on the ESTIMATE algorithm, immune and stromal scores were calculated according to immune or stromal components in the TME. We divided breast cancer cases into high- and low-score groups and identified differentially expressed genes (DEGs) that were significantly associated with overall survival. We performed enrichment analysis and constructed a protein-protein interaction network and found that the DEGs were mainly involved in primary immunodeficiency, T cell receptor signaling pathway and cytokine-cytokine receptor reaction. Furthermore, we explored the effect of aging on immune and stromal scores, which was validated by lower immune/stromal scores, lower infiltration of T cells and lower expression of immune checkpoints in the elder group. In conclusion, certain differentially expressed immune-related genes contribute to longer overall survival, and aging influences the immune microenvironment and immunotherapy efficacy by changing the tumor-infiltrating lymphocyte (TIL) abundance and checkpoint expression in breast cancer.
Introduction
Breast cancer is the most common malignant tumor in women, with an incidence that increases yearly, and it has the second highest mortality rate among malignant tumors in women (1). Breast cancer is a heterogeneous disease with variable molecular characteristics and discrepant components in the tumor microenvironment (TME). As an extremely heterogeneous system, the TME is composed of infiltrating lymphocytes, macrophages, fibroblasts, extracellular matrix, adipocytes, and other stromal components (2, 3). In addition, the TME has been well recognized as an essential factor in cancer development, growth, and progression (2). In the microenvironment, in addition to tumor cells, immune cells and stromal cells are the major cell types that reprogram the inflammatory and metabolic profiles of cancer cells (3, 4). However, the transcriptome profile of cancer involves parameters derived from both tumor cells and immune cells and stromal cells in the tumor microenvironment. Indeed, previous studies have demonstrated immune or stromal “signatures” in breast cancer, which are possibly relevant to clinical features (5, 6). Thus, it is speculated that the gene expression profile of microenvironment components may impact the specific subtypes of breast cancer. Importantly, whether and to what extent microenvironment effects result in assigning breast cancer to a specific phenotype have not been assessed.
Age is considered a pivotal risk factor for breast cancer, which has become a huge health burden in the senescent population (7). Further, aging can remarkably contribute to breast cancer progression and is associated with a worse disease course (8). Aging and cancer are two highly related biological phenomena, however, the causative relationship between them remains unclear and requires more study. Although many studies have been focused on investigating the mechanism why aging promotes oncogenesis, less attention has been paid to explore the special characteristics of elderly patients with cancer. It is still unknown whether aging can induce unique molecular features and whether aging-associated features should shape treatment strategies in elderly cancer patients.
Based on the global gene expression data in The Cancer Genome Atlas (TCGA) database, algorithms have been developed to calculate tumor purity, such as ESTIMATE (Estimation of Stromal and Immune cells in Malignant Tumor tissues using Expression data) (9). ESTIMATE is an algorithm that calculates immune and stromal scores of cancer to predict the infiltration of immune and stromal cells by analyzing specific gene expression profiles of immune and stromal cells (9). Therefore, we designed an analytical procedure to perform a pan-cancer transcriptomic analysis and investigated senescence-related altered immune cells and immune checkpoints in the tumor microenvironment.
Materials and Methods
Database
The gene expression data of breast cancer patients were downloaded from TCGA data portal (https://tcga-data.nci.nih.gov/tcga/). Clinical information, including age, hormone receptor status and survival, were also obtained from TCGA data portal. We calculated immune scores and stromal scores by applying the ESTIMATE algorithm to gene expression data (9).
Recognition of Differentially Expressed Genes (DEGs)
Differentially expressed genes between the high immune/stromal score group and low immune/stromal score group were detected using the package limma (10). To reduce the false positive rate, we used the Benjamini and Hochberg false discovery rate to adjust p-values. An adjusted p < 0.05 and |logFC| > 1 were defined as the cutoff criteria. Additionally, we used ImageGP (http://www.ehbio.com/ImageGP/index.php/Home/Index/index.html) to construct a visual volcano plot to display the results of the dataset analysis and Morpheus online analysis software (https://software.broadinstitute.org/morpheus/) to construct a heat map and perform hierarchical cluster analysis.
Acquisition of the Common DEGs
The common DEGs, which stemmed from the immune scores and stromal scores, were acquired using a Venn analysis. Subsequently, we constructed a visual Venn diagram to show the results of the intersection using Bioinformatics & Evolutionary Genomics online tools (http://bioinformatics.psb.ugent.be/webtools/Venn/).
Enrichment Analysis of DEGs
Gene ontology (GO) analysis is an approach to annotate genes and gene products through analyzing high-throughput genome or transcriptome data (11). Kyoto Encyclopedia of Genes and Genomes (KEGG) is a database collection that can help in analysis of genomes, biological pathways, diseases, and drugs (12). The Database for Annotation, Visualization and Integrated Discovery (DAVID, https://david.ncifcrf.gov/) is an online bioinformatics tool providing a means for functional analysis of a number of genes and proteins (13). Significance levels were set at p < 0.05. We used DAVID to visualize the core biological process (BP), molecular function (MF), cellular component (CC), and pathways among the DEGs. Furthermore, we utilized ImageGP (http://www.ehbio.com/ImageGP/index.php/Home/Index/index.html) to construct a visual enrichment plot to display the outcomes.
Construction of a PPI Network
Search Tool for the Retrieval of Interacting Genes (STRING) is an online tool that helps evaluate protein-protein interaction (PPI) networks (14). We used the STRING app in Cytoscape to analyze DEGs to identify potential relationships among the DEGs. We set the cutoff criteria as a confidence score ≥ 0.4 and maximum number of interactors = 0. Moreover, we utilized the Molecular Complex Detection (MCODE) app to screen modules in the PPI network in Cytoscape with the following criteria: degree cut-off = 2, node score cut-off = 0.2, k-core = 2, and max. depth = 100 (15). DAVID was used to perform pathway analysis of genes in these modules. To explore the potential information, we carried out GO and KEGG pathway analyses.
Statistical Analysis
Overall survival (OS) curves of individual DEGs that were significantly associated with OS were downloaded from the website http://gepia.cancer-pku.cn/. Kaplan-Meier survival analyses of immune/stromal scores and age were performed using GraphPad Prism 8.0.1 software, p-values were calculated with a log-rank test, and p < 0.05 was set as the cut-off. All relevant statistical analyses were performed with SPSS 22.0 software (IBM Corporation, Armonk, NY, USA), and significance levels were set at p < 0.05.
Results
Immune Scores and Stromal Scores Are Significantly Associated With Breast Cancer Subtypes
We downloaded gene expression data and clinical information of breast cancer patients from TCGA database. After exclusion of patients with incomplete clinical information and the normal control group, 1,089 breast cancer patients were included in our study. Based on the pathological diagnosis given in TCGA database, breast cancer was classified into five clear subtypes: infiltrating ductal carcinoma, medullary carcinoma, metaplastic carcinoma, infiltrating lobular carcinoma and mucinous carcinoma. The 1,089 cases included 779 (71.5%) infiltrating ductal carcinoma, 203 (18.6%) infiltrating lobular carcinoma, 5 (0.5%) medullary carcinoma, 9 (0.8%) metaplastic carcinoma, 17 (1.6%) mucinous carcinoma, 29 (2.7%) mixed histology, and 47 (4.3%) unknown subtypes. As analyzed using the ESTIMATE algorithm, immune scores ranged from −1342.120 to 3728.873, and stromal scores ranged from −2141.790 to 2112.231 (Figures 1A,B). As the most common types of breast cancer, the immune and stromal scores of infiltrating ductal carcinoma were lower than those of infiltrating lobular carcinoma (Figures 1A,B; p < 0.001, and p < 0.05, respectively). The mucinous carcinoma subtype had the lowest scores, whereas metaplastic carcinoma had the highest stromal score, and medullary carcinoma had the highest immune score, indicating that there was not much correlation between immune and stromal scores and the subtypes of breast cancer.
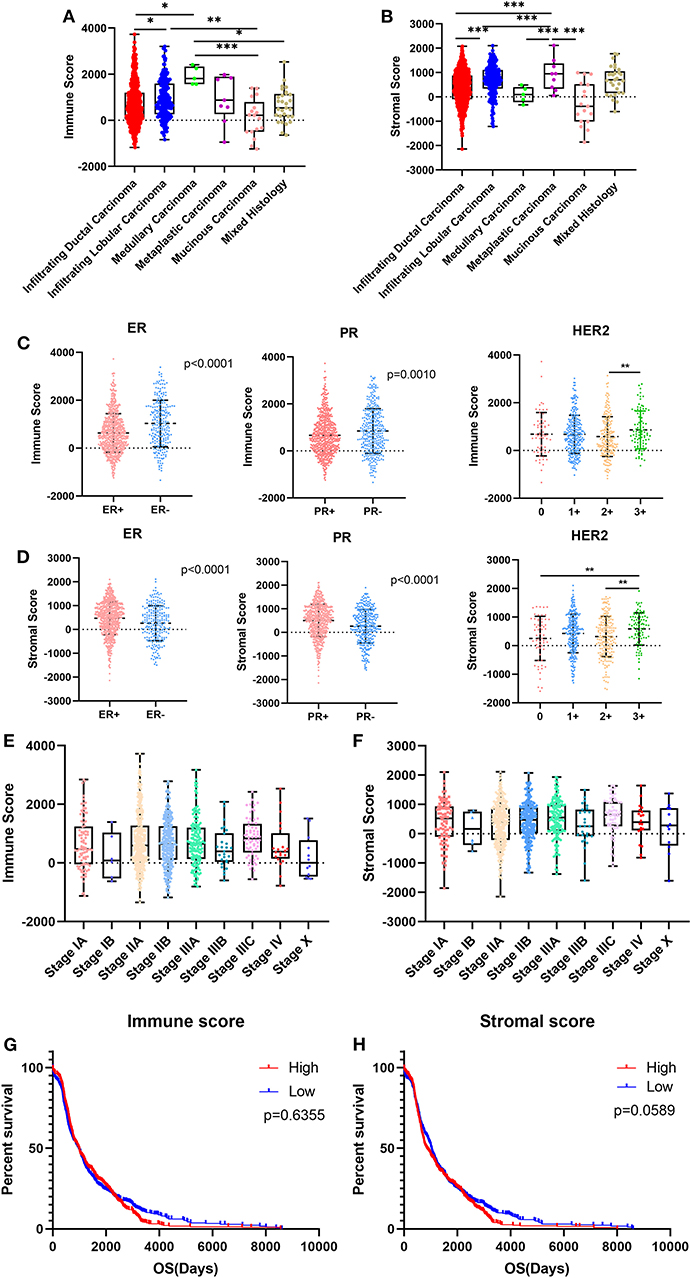
Figure 1. Immune scores and stromal scores are associated with breast cancer subtypes and patient overall survival. (A,B) Distribution of immune scores or stromal scores among breast cancer pathological subtypes. (C,D) Distribution of immune scores or stromal scores among breast cancer molecular subtypes. (E,F) Distribution of immune scores or stromal scores among breast cancer stages. (G,H) Patients were divided into high- and low-score groups according to their immune scores or stromal scores. Kaplan-Meier plots were generated to compare overall survival (days) in the high- and low-score groups. Differences were assessed with a log-rank test. *p < 0.05, **p < 0.01, and ***p < 0.001 for analysis of variance (ANOVA).
Given that this pathological classification of breast cancer is of limited clinical application, we focused on the molecular subtypes of breast cancer classified by hormone receptor status and human epidermal growth factor receptor-2 (HER2) status. For the immune score, cases with negative hormone receptor status and positive HER2 status had higher scores, whereas cases with positive hormone receptor status and positive HER2 status had higher stromal scores (Figures 1C,D).
We compared immune and stromal scores at different stages of breast cancer and found that there were no significant differences in either the immune or the stromal scores (Figures 1E,F).
We analyzed the potential association between overall survival (OS) and immune/stromal scores. According to the immune and stromal scores, we divided all 1,089 cases into two groups, a high-score group and low-score group. Kaplan-Meier survival curves showed that although the median OS of cases with low immune scores was shorter than that of cases with high immune scores the difference was not significant (Figure 1G; 965d vs. 975d, p = 0.6355). In the stromal score group, the median OS of cases with low stromal scores was longer than that of cases with high stromal scores, but again, the difference was not significant (Figure 1H; 1,025 d vs. 856 d, p = 0.0589). It seems that immune/stromal scores cannot significantly predict the OS of breast cancer patients.
Comparison of Gene Expression Profiles With Immune Scores and Stromal Scores in Breast Cancer
To investigate the association between gene expression profiles and immune/stromal scores, we analyzed gene expression data of all 1,089 breast cancer cases divided into high/low immune/stromal score groups. Based on immune scores, a volcano plot showed that 851 genes were upregulated and 67 genes were downregulated in the high-score group compared with the low-score group (Figure 2A; fold change > 2.0, p < 0.05). In the high stromal score group, 1,342 genes were upregulated, and 38 genes were downregulated (Figure 2B; fold change > 2.0, p < 0.05). In addition, a Venn diagram demonstrated that 397 genes were commonly upregulated while only 6 genes were commonly downregulated in the high-score groups (Figure 2C). Heatmaps also validated these differentially expressed genes (DEGs) (Figures 2D,E). To explore the function and pathway of these DEGs, we performed gene ontology (GO) function analysis using DAVID and KEGG pathway enrichment analysis. The GO results showed that DEGs were particularly enriched in the following biological processes (BPs): immune response, regulation of immune system process and cell surface receptor signaling pathway (Figure 2F). GO cell component (CC) analysis demonstrated that DEGs were enriched in integral component of plasma membrane, intrinsic component of plasma membrane and cell surface (Figure 2G). GO molecular function results showed that DEGs were enriched in molecular transducer activity, receptor activity and signal transducer activity (Figure 2H). The significantly enriched pathways shown by KEGG pathway analysis included cytokine-cytokine receptor reaction, hematopoietic cell lineage cell, adhesion molecules (CAMs) and chemokine signaling pathway (Figure 2I).
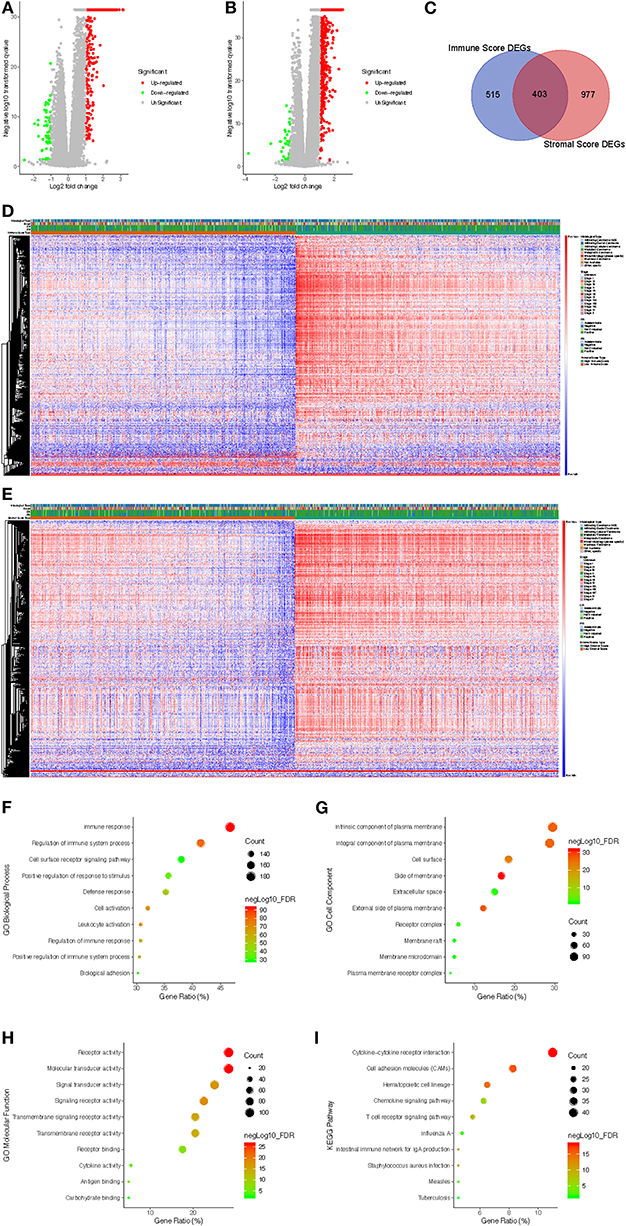
Figure 2. Comparison of gene expression profiles with immune scores and stromal scores in breast cancer. (A) The significantly upregulated and downregulated genes in the high immune score group compared with the low score group are shown in the volcano plot (fold change > 2.0, p < 0.05). (B) The significantly upregulated and downregulated genes in the high stromal score group compared with the low score group are shown in the volcano plots (fold change > 2.0, p < 0.05). (C) Venn diagrams showing the commonly upregulated genes in both the high immune score group and high stromal score group. (D) Immune score DEGs in breast cancer displayed in a heat map. (E) Stromal score DEGs in breast cancer displayed in a heat map. (F–H) GO analysis results showing that commonly upregulated DEGs were particularly enriched in BP (F), CC (G), and MF (H). (I) The significantly enriched pathways of the commonly upregulated DEGs determined by KEGG analysis. GO, gene ontology; BP, biological process; CC, cell component; MF, molecular function; KEGG, Kyoto Encyclopedia of Genes and Genomes.
Association Between DEGs and Overall Survival
To investigate the potential effects of individual DEGs on OS, we downloaded overall survival curves from the website http://gepia.cancer-pku.cn/. Among all the 403 DEGs, only 129 upregulated genes were significantly associated with OS and predicted better prognosis of patients (Figure 3; p < 0.05). What's more, we validated those selected DEGs in the Breast Cancer Metabric data downloaded from cBioPortal (http://download.cbioportal.org/brca_metabric.tar.gz). We drew survival curves using the Graphpad software and found that higher expression of 102 genes was significantly associated with longer overall survival (Figure 6). To explore the crosstalk between these DEGs, we performed protein-protein interactions network analysis using the online tool STRING. By focusing on the genes that had the most connections with other genes, we obtained 3 typical networks, and interestingly, all were associated with immune response. In the first module, CD69, CD27, CD2, CD3E, and LCK were prominent nodes (Figure 4A). For the second module, PDCD1 (PD-1), CD5, SELL, and CD19 had the most connections (Figure 4B). In the last selected module, GZMA, GZMK, CD3D, and KLRB1 were the most important nodes (Figure 4C). Further, we analyzed the related pathways using KEGG and found that the top associated pathways were primary immunodeficiency, T cell receptor signaling pathway and cytokine-cytokine receptor reaction (Figure 4D).
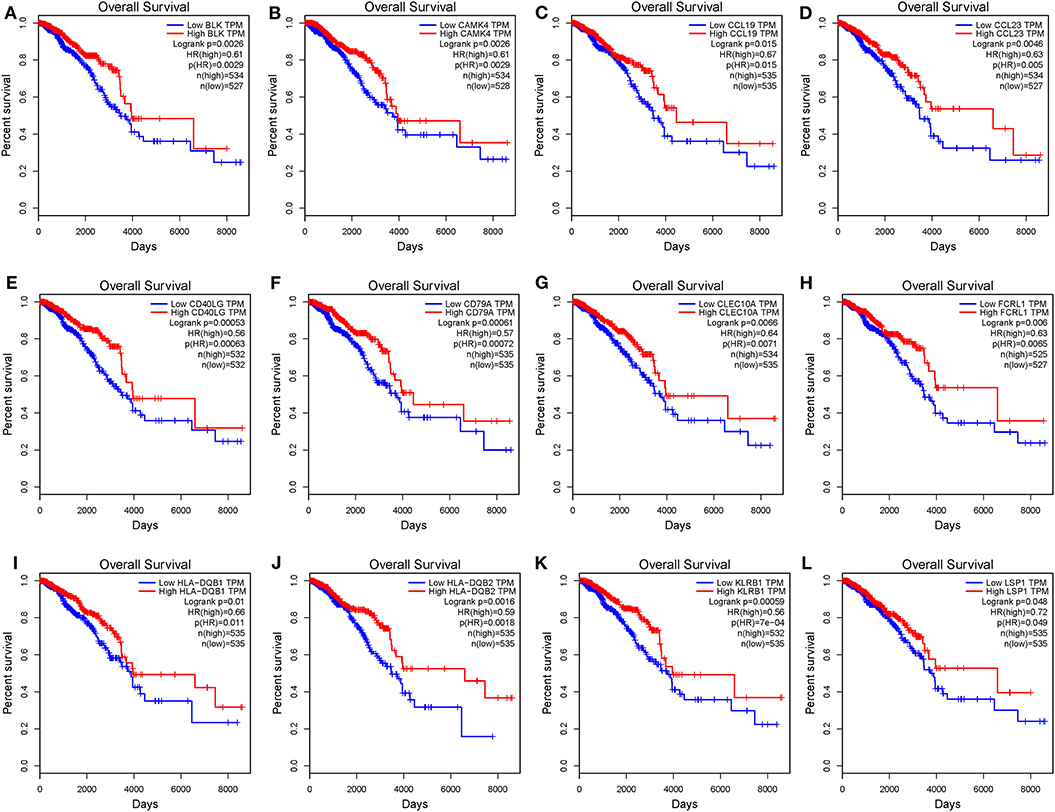
Figure 3. Common DEGs that significantly impact overall survival. (A–L) Twelve commonly upregulated DEGs that were significantly associated with better prognosis. OS, overall survival (in days).
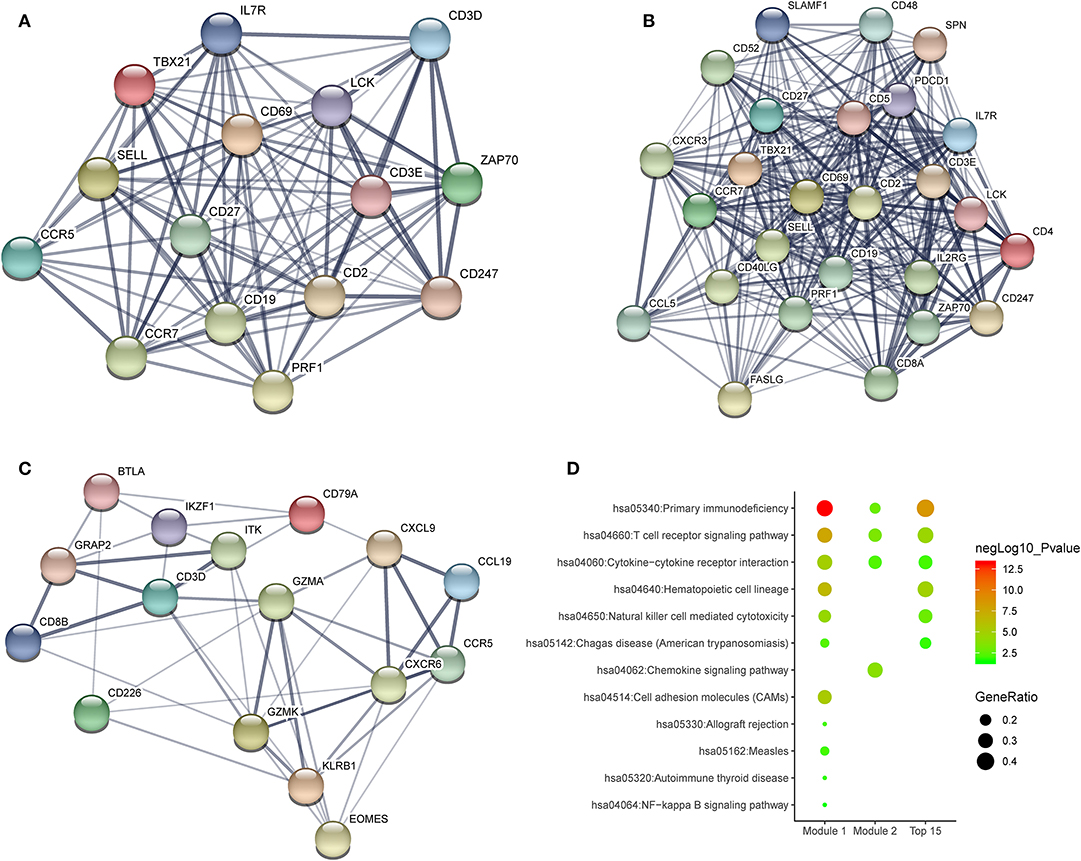
Figure 4. Potential crosstalk between significantly DEGs. (A–C) Top 3 modules from a protein-protein interaction network of 129 significantly DEGs. (D) The most enriched KEGG pathways in the top 3 modules.
Aging Modified the Immune Microenvironment as Well as Checkpoints
As a strong aging-associated carcinoma (16), we were curious whether aging was associated with the immune/stromal scores of breast cancer patients. We grouped patients older than 64 years old in an eld group and patients younger than 51 years old in a young group. Patients with ages ranging from 51 to 64 years old were excluded from this analysis. We compared the immune/stromal scores in the eld/young groups and found that the median immune score of the young group was higher than that of the eld group (Figure 5A; 768.7 vs. 639.1, p = 0.046). In addition, the median stromal score of the young group was also higher than that of the eld group (Figure 5B; 492.3 vs. 350.8, p = 0.0084). We also compared immune scores and stromal scores in young and elderly groups in different breast cancer subtypes (Figures 8A,B). Unfortunately, there were no significant differences in two groups among different subtypes, except for the stromal score of the eld group was lower than that of the young group in Luminal A subtype (318.7 vs 592.9, p = 0.000947). Kaplan-Meier survival analysis demonstrated that the median overall survival of patients in the young group was longer than that of patients in the eld group (Figure 5C; 1206d vs. 747d, p < 0.0001). Our results showed that aging was significantly associated with lower immune/stromal scores and with poor overall survival. To explore the relationship between aging and immune/stromal scores, we compared the infiltration level of immune cells in the tumor microenvironment in the eld/young groups. The results demonstrated that infiltration of cytotoxic T cells, memory T cells, B cells and lymph vessels in the young group was higher than that in the eld group (Figure 5D). However, the infiltration of Treg cells was higher in the young group (Figure 5D). What's more, we added analysis about the infiltration levels of TILs in different breast cancer subtypes. As shown in Figure 7, the infiltration levels of cytotoxic T cells, Treg cells, B cells, memory T cell, iDcs and mast cells in the TME of TNBC patients were higher than that of Luminal A and Luminal B patients, which were consistent with previous study. In addition, we analyzed immune checkpoints, which are highly associated with cancer immunotherapy. The expression levels of PD-1 and CTLA-4 were significantly higher in the young group than in the eld group (Figure 5E; p = 0.013, and p = 0.015, respectively). We also compared the expression of checkpoints in young and elderly groups in different breast cancer subtypes (Figure 8C). It was disappointing that there were no significant association between age and the expression of checkpoints in different subtypes. We also analyzed the relationship between aging and the expression of PD-1, CTLA-4, PD-L1, and PD-L2 and found that almost all the checkpoints were negatively linearly associated with aging, except PD-L1 (Figure 5F), although only CTLA-4 expression was significantly associated with aging (Figure 5F; p = 0.032). Furthermore, TP53 is one of the most common mutations in breast cancer (17), and the number of cases with TP53 mutation in the eld group was lower than that in the young group (Figure 5G; p = 0.005). TP53 mutation was also associated with higher immune scores in the eld group (Figure 5H; p < 0.001), which may indicate that more mutations were accompanied by a greater immune response due to the production of tumor neoantigens. Moreover, changes in gene mutation frequency might be involved in aging-related changes in the microenvironment.
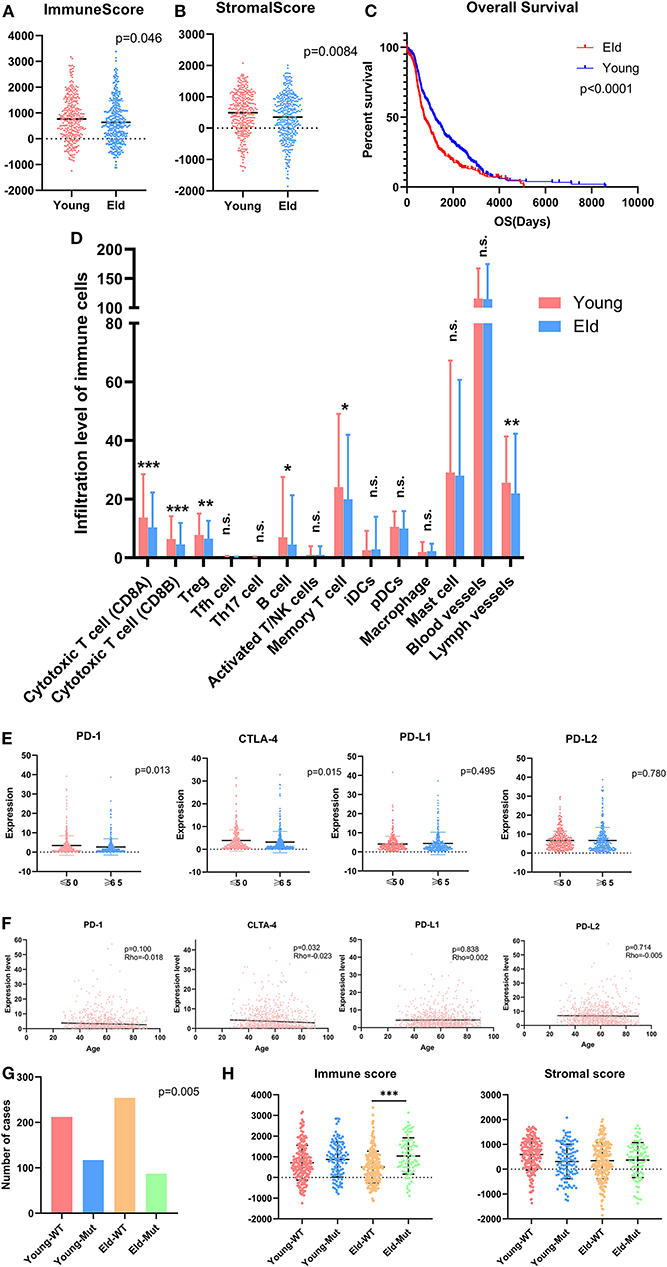
Figure 5. Aging alters the immune microenvironment as well as checkpoint expression levels. (A,B) Distribution of immune scores or stromal scores according to the age of breast cancer patients: young group (≤50 years old) vs. eld group (≥65 years old). (C) Aging was significantly associated with worse prognosis (p < 0.0001). (D) Age altered the infiltration of immune cells in the microenvironment, especially T cells, B cells and Treg cells. (E) Common checkpoints in breast cancer. (F) Association between age and checkpoints in breast cancer. (G) Distribution of immune scores in the young or eld group with or without TP53 mutation. p-value for chi-square test. (H) Distribution of stromal scores in the young or eld group with or without TP53 mutation. WT, wild-type TP53; Mut, TP53 mutation. *p < 0.05, **p < 0.01, and ***p < 0.001 for analysis of variance (ANOVA).
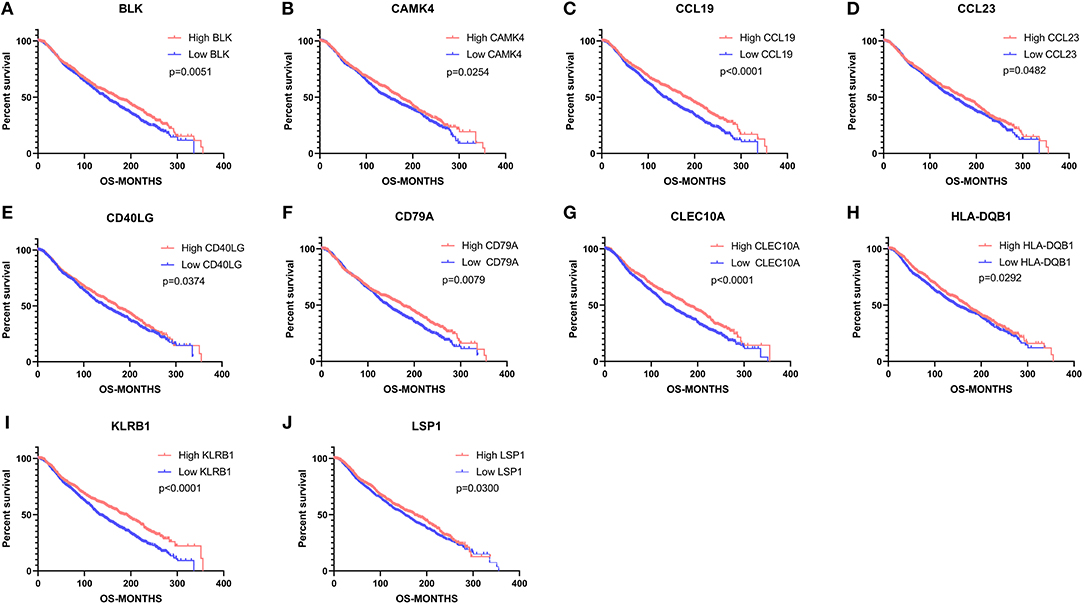
Figure 6. Validation of common DEGs that significantly impact overall survival in the Breast Cancer Metabric data downloaded from cBioPortal. (A–J) 10 commonly upregulated DEGs that were significantly associated with better prognosis. OS, overall survival (in months).
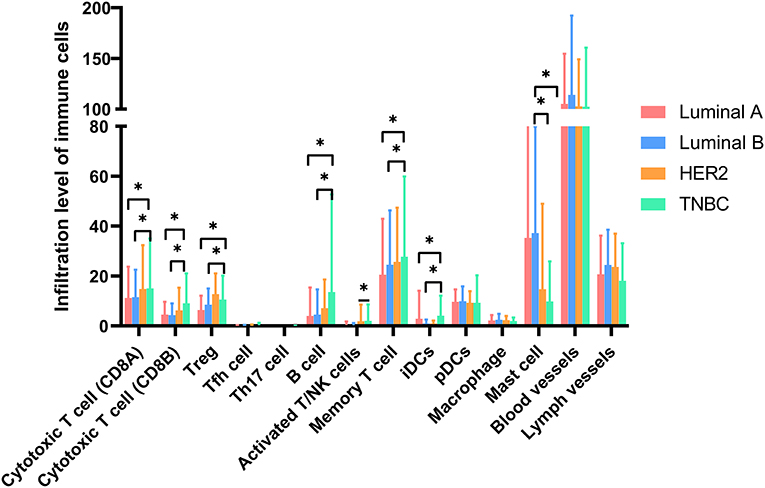
Figure 7. Molecular subtypes altered the infiltration of immune cells in the microenvironment, especially T cells, B cells and Treg cells. *p < 0.05 for multiple t-test.
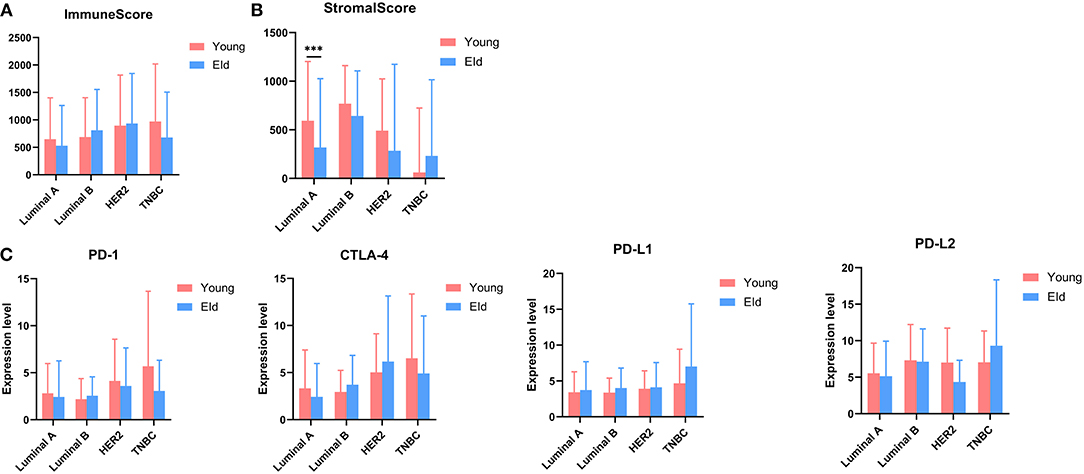
Figure 8. (A,B) Distribution of immune scores or stromal scores in different subtypes according to the age of breast cancer patients: young group (≤50 years old) vs. eld group (≥65 years old). (C) The expression of common checkpoints in breast cancer subtypes. ***p < 0.001 for multiple t-test.
Discussion
There is growing evidence that abnormal immune/inflammatory responses in the tumor microenvironment are essential mechanisms that promote cancer progression (2). We attempted to excavate correlated genes that were significantly associated with the overall survival of breast cancer patients based on TCGA data. Using the ESTIMATE algorithm, we calculated the immune/stromal scores of each selected patient according to the expression of involved genes in the two groups (9). By comparing gene expression profiles of patients with high or low scores, we identified 403 commonly DEGs (397 genes upregulated, and 6 genes downregulated). We also identified 129 upregulated DEGs that predicted better prognosis of patients, and high immune/stromal scores were found be a protective factor in breast cancer patients. In addition, we verified that aging is related to less infiltration of immune cells and lower expression of immune checkpoints, which contribute to poor prognosis.
In terms of pathological differences, we found that the immune and stromal scores of infiltrating ductal carcinoma were lower than those of infiltrating lobular carcinoma, and metaplastic carcinoma had the highest stromal score, while medullary carcinoma had the highest immune score. Recent studies have demonstrated that different pathological breast cancer subtypes have different genetic characteristics. TP53 and PIK3CA mutations are common in ductal breast carcinoma (18, 19), while mucinous carcinoma lacks PIK3CA mutation (20). ERBB2 mutation is commonly present in both infiltrating lobular carcinoma and mucinous carcinoma (21, 22). TP53 is the most commonly mutated gene in metaplastic breast carcinoma (23), and hypermethylation of the BRCA1 promoter has been detected in medullary carcinoma (24). These differences may result from the heterogeneity of the tumor microenvironment, which has been confirmed by observation of differences in infiltrating lymphocytes in different subtypes of breast cancer (25–27). Tumor-infiltrating lymphocyte (TIL) numbers in infiltrating lobular carcinoma are significantly lower than in infiltrating ductal carcinoma (27), and TILs in medullary carcinoma are primarily CD8+ T lymphocytes (26). Given that immune/stromal scores were positively associated with overall survival of patients, patients with high scores are more likely to have longer disease-free survival and to benefit from immunotherapy. We came to the hypothesis that patients with infiltrating ductal carcinoma or medullary carcinoma might be more sensitive to immunotherapy. However, metaplastic cancer, which is a rare subtype of breast cancer, is poorly responsive to neoadjuvant chemotherapy (28). Patients with metaplastic cancer may benefit from targeted therapy or radiation therapy.
Our results demonstrated that patients with a negative HR status and a positive HER-2 status had the highest immune scores, while the highest stromal scores were observed in patients with both a positive HR status and positive HER2 status. Moreover, TILs were discovered to be a good prognostic value in TNBC but fail in the luminal subtypes (29). This correlation was subsequently independently confirmed in 481 TNBC sample prospectively collected during two phase III adjuvant randomized BC trials (30). Growing evidence focused on immunotherapy for breast cancer has demonstrated that higher numbers of TILs and Treg cells are significantly associated with the TNBC and HER2 overexpression subtypes (31, 32), and more PD-1+ TILs have been found in TNBC and were correlated with higher immune scores (33). In recent clinical trials, immunotherapy, such as blockade of PD-1 (Pembrolizumab), PD-L1 (Durvalumab) and CTLA-4 (Tremelimumab), reached a higher objective response (OR) in TNBC than in HR+ patients (32, 34). The presence of TILs, especially CD8+ T cells, and the expression of checkpoints contributed to the better response to immunotherapy. The infiltration level of TILs could almost be a predictive factor for therapy response (32). Mechanistically, activated HER2 induces the production of CCL2 (C-C Motif Chemokine Ligand 2) through the PI3K-NF-κB axis, promoting recruitment and activation of infiltrated immune cells (35), and TNBC patients were found to have a higher tumor mutation burden (TMB) and to present neoantigens that are correlated with a more effective immunotherapy response (36), while estrogen and estrogen receptor (ER) signaling appears to have little impact on the immune environment (37). With regard to stromal signatures, cancer-associated fibroblasts (CAFs) are one of the most important stromal cell types in the breast cancer microenvironment. CAF-related proteins, such as fibroblast activation protein alpha (FAPα), podoplanin, S100A4, platelet-derived growth factor receptor alpha (PDGFRα), and PDGFRβ, were found to be upregulated in HER2-overexpressing patients (38). The expression of matrix metalloproteinase 2 (MMP2), which facilitates tumor invasion, has been shown to be significantly increased in HER2 subtype patients but not in TNBC patients (39). In ER+ cases, hormone therapy remains the most important therapy; however, acquired resistance to endocrine therapy is a clinical obstacle. Compared with single hormone therapy, a combination of hormone therapy and interleukin-2, interleukin-12 and interferons presented a better anti-tumor effect via synergism with antiestrogens (40). In addition, combining immune checkpoint inhibitors and targeted therapies, such as targeting of PD-1 and PD-L1 combined with HER2-targeted therapy (Trastuzumab), can improve the prognosis of PD-L1-positive patients (41). Above all, immunotherapy is a promising approach for TNBC and HER2-overexpressing subtypes, and patients with the HER2-overexpressing subtype as well as the HR+ and TNBC subtypes can benefit from targeted therapies. Combination therapies with immunotherapy in breast cancer molecular subtypes are worth further investigation.
Among the DEGs identified, we performed overall survival analysis and found that 129 upregulated DEGs were positively associated with better prognosis. However, a previous study on glioblastoma (GBM) obtained the opposite results, reporting that the selected upregulated DEGs were correlated with poor prognosis of patients (42). Similar studies of non–small cell lung cancer (NSCLC) and pancreatic cancer showed that a high immune score predicted a good prognosis (43, 44), which was consistent with our results. The brain is a natural immune exemption zone because of the blood-brain barrier, and thus, its microenvironment is very different from that in breast cancer. Compared with primary breast cancer, paired brain metastases presented significantly lower TIL abundance (45). The expression of the CAF-related proteins stromal podoplanin, stromal PDGFRα and stromal PDGFRβ is reduced in brain metastases (46). However, in GBM, one of the most aggressive brain tumors, the dominant infiltrating immune cells are tumor-associated macrophages (TAMs), which facilitate tumor growth via their proangiogenic and immunosuppressive effects (47). Furthermore, CD8+ TILs are the most common infiltrating immune cells in NSCLC (48). This could partially explain the confusing results in GBM that were not consistent with those in breast cancer. A combination of immunotherapy and chemotherapy (such as atezolizumab plus nab-paclitaxel) was shown to improve the progression-free survival (PFS) and overall survival rate in metastatic TNBC (11, 41), and application of a vaccine against the GBM-specific WT1 peptide significantly increased PFS and overall survival in patients by inducing production of anti-WT1 antibodies and T cell responses (49). In the present study, protein-protein interaction network results showed that upregulated DEGs were primarily associated with immune response, including primary immunodeficiency, T cell receptor signaling pathway and cytokine-cytokine receptor reaction, which predicted a better prognosis via an increased immune response. Together, combination immune and stromal scores or further refinement scores can predict the prognosis of breast cancer or screen out people eligible for immunotherapy.
Aging was significantly associated with lower immune/stromal scores and poor prognosis in breast cancer patients due to reduce infiltration of immune cells and lower expression of checkpoints. The incidence of breast cancer and associated mortality rate increased with age, and almost one half of newly diagnostic breast cancer cases occurred at age 65 and older (50, 51). Barajas-Gómez and colleagues compared the concentration of cytokines between patients and healthy young donors and found that pro-inflammatory cytokine levels, including IL-2, IL-6, and IL-8, were increased in the elderly, whereas anti-inflammatory cytokines, such as IL-4 and IL-10, exhibited decreased expression (52). This low intensity and chronic inflammatory microenvironment promoted tumorigenesis and progression. In elderly patients, proliferation and activity of CD4+ and CD8+ T cells and B cells decreased, while those of immunosuppressive cells increased (53), which formed an immunosuppressive microenvironment that facilitated tumor progression (16). However, few prospective clinical trials have focused on the elderly, and limited studies have demonstrated that older patients benefit from chemotherapy as well as targeted therapies but exhibit more severe cardiotoxicity and bone marrow disorders (54). In addition, anti-PD1 and anti-CTLA-4 immunotherapy was shown to improve OS in both older and younger patients (55). The CheckMate-067 study focused on the combination of nivolumab and ipilimumab for advanced melanoma and showed that patients older than 65 years had a shorter OS than younger patients (56). Above all, the microenvironment changes with age, which may reduce the effects of immunotherapy.
This study still has some shortcomings. The data were only derived from TCGA database and lacked verification with real-world data. We only analyzed transcriptome data, without assessing data associated with non-coding transcriptomes, epigenetic differences or proteomics. We only used bioinformatics analysis, and the study lacked evidence obtained through other methods, such as immunohistochemistry and proteomics, and lacked further validation with a patient-derived xenograft (PDX) mouse model.
In conclusion, based on ESTIMATE algorithm-derived immune/stromal scores, we identified immune microenvironment-related genes that are associated with better patient prognosis. Aging was found to be a risk factor for tumorigenesis and progression and influenced the immune microenvironment and immunotherapy efficacy by altering the number of TILs and checkpoint expression levels. This work may provide new targets for breast cancer treatment that need further investigation in the future.
Data Availability Statement
Publicly available datasets were analyzed in this study. The data can be found in the TCGA database.
Author Contributions
BL, RG, and QW designed the study. BL, RG, QW, QY, SZ, and ZX conducted the study, collected and analyzed the data. BL, RG, QW, QY, SiS, SZ, and ShS interpreted the data. BL wrote this article. BL, RG, and QW took responsibility for the integrity of the data analysis. ShS was responsible for editing and submitting this manuscript. All authors approved the submission.
Funding
This work was supported by a National Natural Science Foundation of China (NSFC) grant (Grant No: 81471781) and a National major scientific instruments and equipment development projects grant (Grant No: 2012YQ160203) to ShS. This work was also supported by a Hubei Province health and family planning scientific research project to SiS (Grant No. WJ2019Q044), and a project (Grant No. WJ2019M188) to ZX. This work was also supported by a Guide Foundation of Remin Hospital of Wuhan University to SZ (Grant No. RMYD2018M78).
Conflict of Interest
The authors declare that the research was conducted in the absence of any commercial or financial relationships that could be construed as a potential conflict of interest.
Acknowledgments
We thank American Journal Experts for professional language editing.
Abbreviations
BP, biological process; CAFs, cancer-associated fibroblasts; CC, cellular component; CCL2, C-C Motif Chemokine Ligand 2; DAVID, The Database for Annotation, Visualization and Integrated Discovery; DEGs, differentially expressed genes; ER, estrogen receptor; ESTIMATE, Estimation of Stromal and Immune cells in Malignant Tumor tissues using Expression data; FAPα, fibroblast activation protein alpha; GBM, glioblastoma; GO, Gene ontology; HER2, human epidermal growth factor receptor-2; KEGG, Kyoto Encyclopedia of Genes and Genomes; MCODE, the Molecular Complex Detection; MF, molecular function; NSCLC, non–small cell lung cancer; OS, Overall survival; PDGFRα, platelet-derived growth factor receptor alpha; PDX, patient-derived xenograft; PFS, progression-free survival; PPI, protein-protein interaction; STRING, Search Tool for the Retrieval of Interacting Genes; TAMs, tumor-associated macrophages; TCGA, The Cancer Genome Atlas; TIL, tumor-infiltrating lymphocyte; TMB, tumor mutation burden; TME, tumor microenvironment.
References
1. Siegel RL, Miller KD, Jemal A. Cancer statistics. (2018). CA Cancer J Clin. (2018) 68:7–30. doi: 10.3322/caac.21442
2. Iyengar NM, Gucalp A, Dannenberg AJ, Hudis CA. Obesity and cancer mechanisms: tumor microenvironment and inflammation. J Clin Oncol. (2016) 34:4270–6. doi: 10.1200/JCO.2016.67.4283
3. Wu Q, Li J, Li Z, Sun S, Zhu S, Wang L, et al. Exosomes from the tumour-adipocyte interplay stimulate beige/brown differentiation and reprogram metabolism in stromal adipocytes to promote tumour progression. J Exp Clin Cancer Res. (2019) 38:223. doi: 10.1186/s13046-019-1210-3
4. Hoy AJ, Balaban S, Saunders DN. Adipocyte-Tumor cell metabolic crosstalk in breast cancer. Trends Mol Med. (2017) 23:381–92. doi: 10.1016/j.molmed.2017.02.009
5. Winslow S, Leandersson K, Edsjo A, Larsson C. Prognostic stromal gene signatures in breast cancer. Breast Cancer Res. (2015) 17:23. doi: 10.1186/s13058-015-0530-2
6. Dennison JB, Shahmoradgoli M, Liu W, Ju Z, Meric-Bernstam F, Perou CM, et al. High intratumoral stromal content defines reactive breast cancer as a low-risk breast cancer subtype. Clin Cancer Res. (2016) 22:5068–78. doi: 10.1158/1078-0432.CCR-16-0171
7. Siegel RL, Miller KD, Jemal A. Cancer statistics. (2015). CA Cancer J Clin. (2015) 65:5–29. doi: 10.3322/caac.21254
8. Abu Rabi Z, Zoranovic T, Milovanovic J, Todorovic-Rakovic N, Nikolic-Vukosavljevic D. Breast cancer in postmenopausal patients: impact of age. J BUON. (2015) 20:723–9.
9. Yoshihara K, Shahmoradgoli M, Martinez E, Vegesna R, Kim H, Torres-Garcia W, et al. Inferring tumour purity and stromal and immune cell admixture from expression data. Nat Commun. (2013) 4:2612. doi: 10.1038/ncomms3612
10. Ritchie ME, Phipson B, Wu D, Hu Y, Law CW, Shi W, et al. Limma powers differential expression analyses for RNA-sequencing and microarray studies. Nucleic Acids Res. (2015) 43:e47. doi: 10.1093/nar/gkv007
11. Schmid P, Adams S, Rugo HS, Schneeweiss A, Barrios CH, Iwata H, et al. Atezolizumab and Nab-paclitaxel in advanced triple-negative breast cancer. N Engl J Med. (2018) 379:2108–121. doi: 10.1056/NEJMoa1809615
12. Ogata H, Goto S, Sato K, Fujibuchi W, Bono H, Kanehisa M. KEGG: Kyoto encyclopedia of genes and genomes. Nucleic Acids Res. (1999) 27:29–34. doi: 10.1093/nar/27.1.29
13. Huang da W, Sherman BT, Lempicki RA. Systematic and integrative analysis of large gene lists using DAVID bioinformatics resources. Nat Protoc. (2009) 4:44–57. doi: 10.1038/nprot.2008.211
14. Szklarczyk D, Franceschini A, Wyder S, Forslund K, Heller D, Huerta-Cepas J, et al. STRING v10: protein-protein interaction networks, integrated over the tree of life. Nucleic Acids Res. (2015) 43:D447–52. doi: 10.1093/nar/gku1003
15. Geng RX, Li N, Xu Y, Liu JH, Yuan FE, Sun Q, et al. Identification of core biomarkers associated with outcome in glioma: evidence from bioinformatics analysis. Dis Markers. (2018) 2018:3215958. doi: 10.1155/2018/3215958
16. Wu Y, Wei J, Chen X, Qin Y, Mao R, Song J, et al. Comprehensive transcriptome profiling in elderly cancer patients reveals aging-altered immune cells and immune checkpoints. Int J Cancer. (2019) 144:1657–63. doi: 10.1002/ijc.31875
17. Bertheau P, Lehmann-Che J, Varna M, Dumay A, Poirot B, Porcher R, et al. p53 in breast cancer subtypes and new insights into response to chemotherapy. Breast. (2013) 22(Suppl. 2):S27–9. doi: 10.1016/j.breast.2013.07.005
18. Hendry S, Pang JB, Byrne DJ, Lakhani SR, Cummings MC, Campbell IG, et al. Relationship of the breast ductal carcinoma in situ immune microenvironment with clinicopathological and genetic features. Clin Cancer Res. (2017) 23:5210–7. doi: 10.1158/1078-0432.CCR-17-0743
19. Myers MB, Banda M, McKim KL, Wang Y, Powell MJ, Parsons BL. Breast cancer heterogeneity examined by high-sensitivity quantification of PIK3CA, KRAS, HRAS, and BRAF mutations in normal breast and ductal carcinomas. Neoplasia. (2016) 18:253–63. doi: 10.1016/j.neo.2016.03.002
20. Kehr EL, Jorns JM, Ang D, Warrick A, Neff T, Degnin M, et al. Mucinous breast carcinomas lack PIK3CA and AKT1 mutations. Hum Pathol. (2012) 43:2207–12. doi: 10.1016/j.humpath.2012.03.012
21. Christgen M, Bartels S, Radner M, Raap M, Rieger L, Christgen H, et al. ERBB2 mutation frequency in lobular breast cancer with pleomorphic histology or high-risk characteristics by molecular expression profiling. Genes Chromosom Cancer. (2019) 58:175–85. doi: 10.1002/gcc.22716
22. Ross JS, Gay LM, Nozad S, Wang K, Ali SM, Boguniewicz A, et al. Clinically advanced and metastatic pure mucinous carcinoma of the breast: a comprehensive genomic profiling study. Breast Cancer Res Treat. (2016) 155:405–13. doi: 10.1007/s10549-016-3682-6
23. Joneja U, Vranic S, Swensen J, Feldman R, Chen W, Kimbrough J, et al. Comprehensive profiling of metaplastic breast carcinomas reveals frequent overexpression of programmed death-ligand 1. J Clin Pathol. (2017) 70:255–9. doi: 10.1136/jclinpath-2016-203874
24. Osin P, Lu YJ, Stone J, Crook T, Houlston RS, Gasco M, et al. Distinct genetic and epigenetic changes in medullary breast cancer. Int J Surg Pathol. (2003) 11:153–8. doi: 10.1177/106689690301100301
25. Guo X, Fan Y, Lang R, Gu F, Chen L, Cui L, et al. Tumor infiltrating lymphocytes differ in invasive micropapillary carcinoma and medullary carcinoma of breast. Mod Pathol. (2008) 21:1101–7. doi: 10.1038/modpathol.2008.72
26. Igari F, Sato E, Horimoto Y, Takahashi Y, Isomura T, Arakawa A, et al. Diagnostic significance of intratumoral CD8+ tumor-infiltrating lymphocytes in medullary carcinoma. Hum Pathol. (2017) 70:129–38. doi: 10.1016/j.humpath.2017.10.020
27. Desmedt C, Salgado R, Fornili M, Pruneri G, van den Eynden G, Zoppoli G, et al. Immune infiltration in invasive lobular breast cancer. J Natl Cancer Inst. (2018) 110:768–76. doi: 10.1093/jnci/djx268
28. Han M, Salamat A, Zhu L, Zhang H, Clark BZ, Dabbs DJ, et al. Metaplastic breast carcinoma: a clinical-pathologic study of 97 cases with subset analysis of response to neoadjuvant chemotherapy. Mod Pathol. (2019) 32:807–16. doi: 10.1038/s41379-019-0208-x
29. Loi S, Sirtaine N, Piette F, Salgado R, Viale G, van Eenoo F, et al. Prognostic and predictive value of tumor-infiltrating lymphocytes in a phase III randomized adjuvant breast cancer trial in node-positive breast cancer comparing the addition of docetaxel to doxorubicin with doxorubicin-based chemotherapy: BIG 02-98. J Clin Oncol. (2013) 31:860–7. doi: 10.1200/JCO.2011.41.0902
30. Adams S, Gray RJ, Demaria S, Goldstein L, Perez EA, Shulman LN, et al. Prognostic value of tumor-infiltrating lymphocytes in triple-negative breast cancers from two phase III randomized adjuvant breast cancer trials: ECOG 2197 and ECOG 1199. J Clin Oncol. (2014) 32:2959–66. doi: 10.1200/JCO.2013.55.0491
31. Glajcar A, Szpor J, Hodorowicz-Zaniewska D, Tyrak KE, Okon K. The composition of T cell infiltrates varies in primary invasive breast cancer of different molecular subtypes as well as according to tumor size and nodal status. Virchows Arch. (2019) 475:13–23. doi: 10.1007/s00428-019-02568-y
32. Hammerl D, Smid M, Timmermans AM, Sleijfer S, Martens JWM, Debets R. Breast cancer genomics and immuno-oncological markers to guide immune therapies. Semin Cancer Biol. (2018) 52(Pt 2):178–88. doi: 10.1016/j.semcancer.2017.11.003
33. Shi F, Chang H, Zhou Q, Zhao YJ, Wu GJ, Song QK. Distribution of CD4(+) and CD8(+) exhausted tumor-infiltrating lymphocytes in molecular subtypes of Chinese breast cancer patients. Onco Targets Ther. (2018) 11:6139–45. doi: 10.2147/OTT.S168057
34. Nanda R, Chow LQ, Dees EC, Berger R, Gupta S, Geva R, et al. Pembrolizumab in patients With advanced triple-negative breast cancer: phase Ib KEYNOTE-012 study. J Clin Oncol. (2016) 34:2460–7. doi: 10.1200/JCO.2015.64.8931
35. Triulzi T, Forte L, Regondi V, Di Modica M, Ghirelli C, Carcangiu ML, et al. HER2 signaling regulates the tumor immune microenvironment and trastuzumab efficacy. Oncoimmunology. (2019) 8:e1512942. doi: 10.1080/2162402X.2018.1512942
36. Jiang T, Shi W, Wali VB, Pongor LS, Li C, Lau R, et al. Predictors of chemosensitivity in triple negative breast cancer: an integrated genomic analysis. PLoS Med. (2016) 13:e1002193. doi: 10.1371/journal.pmed.1002193
37. Burks H, Pashos N, Martin E, McLachlan J, Bunnell B, Burow M. Endocrine disruptors and the tumor microenvironment: a new paradigm in breast cancer biology. Mol Cell Endocrinol. (2017) 457:13–9. doi: 10.1016/j.mce.2016.12.010
38. Park SY, Kim HM, Koo JS. Differential expression of cancer-associated fibroblast-related proteins according to molecular subtype and stromal histology in breast cancer. Breast Cancer Res Treat. (2015) 149:727–41. doi: 10.1007/s10549-015-3291-9
39. Catteau X, Simon P, Noel JC. Stromal expression of matrix metalloproteinase 2 in cancer-associated fibroblasts is strongly related to human epidermal growth factor receptor 2 status in invasive breast carcinoma. Mol Clin Oncol. (2016) 4:375–8. doi: 10.3892/mco.2015.721
40. Nicolini A, Carpi A, Ferrari P, Biava PM, Rossi G. Immunotherapy and hormone-therapy in metastatic breast cancer: a review and an update. Curr Drug Targets. (2016) 17:1127–39. doi: 10.2174/1389450117666160201114752
41. Esteva FJ, Hubbard-Lucey VM, Tang J, Pusztai L. Immunotherapy and targeted therapy combinations in metastatic breast cancer. Lancet Oncol. (2019) 20:e175–86. doi: 10.1016/S1470-2045(19)30026-9
42. Jia D, Li S, Li D, Xue H, Yang D, Liu Y. Mining TCGA database for genes of prognostic value in glioblastoma microenvironment. Aging. (2018) 10:592–605. doi: 10.18632/aging.101415
43. Gentles AJ, Bratman SV, Lee LJ, Harris JP, Feng W, Nair RV, et al. Integrating tumor and stromal gene expression signatures with clinical indices for survival stratification of early-stage non-small cell lung cancer. J Natl Cancer Inst. (2015) 107:djv211. doi: 10.1093/jnci/djv211
44. Tahkola K, Mecklin JP, Wirta EV, Ahtiainen M, Helminen O, Bohm J, et al. High immune cell score predicts improved survival in pancreatic cancer. Virchows Arch. (2018) 472:653–65. doi: 10.1007/s00428-018-2297-1
45. Ogiya R, Niikura N, Kumaki N, Yasojima H, Iwasa T, Kanbayashi C, et al. Comparison of immune microenvironments between primary tumors and brain metastases in patients with breast cancer. Oncotarget. (2017) 8:103671–81. doi: 10.18632/oncotarget.22110
46. Kim HM, Jung WH, Koo JS. Expression of cancer-associated fibroblast related proteins in metastatic breast cancer: an immunohistochemical analysis. J Transl Med. (2015) 13:222. doi: 10.1186/s12967-015-0587-9
47. Chen Z, Hambardzumyan D. Immune microenvironment in glioblastoma subtypes. Front Immunol. (2018) 9:1004. doi: 10.3389/fimmu.2018.01004
48. Takashima Y, Sakakibara-Konishi J, Hatanaka Y, Hatanaka KC, Ohhara Y, Oizumi S, et al. Clinicopathologic features and immune microenvironment of non-small-cell lung cancer with primary resistance to epidermal growth factor receptor tyrosine kinase inhibitors. Clin Lung Cancer. (2018) 19:352–9.e1. doi: 10.1016/j.cllc.2018.02.004
49. Lieberman NAP, Vitanza NA, Crane CA. Immunotherapy for brain tumors: understanding early successes and limitations. Expert Rev Neurother. (2018) 18:251–9. doi: 10.1080/14737175.2018.1425617
50. Shoemaker ML, White MC, Wu M, Weir HK, Romieu I. Differences in breast cancer incidence among young women aged 20-49 years by stage and tumor characteristics, age, race, and ethnicity, 2004-2013. Breast Cancer Res Treat. (2018) 169:595–606. doi: 10.1007/s10549-018-4699-9
51. Barginear MF, Muss H, Kimmick G, Owusu C, Mrozek E, Shahrokni A, et al. Breast cancer and aging: results of the U13 conference breast cancer panel. Breast Cancer Res Treat. (2014) 146:1–6. doi: 10.1007/s10549-014-2994-7
52. Barajas-Gomez BA, Rosas-Carrasco O, Morales-Rosales SL, Pedraza Vazquez G, Gonzalez-Puertos VY, Juarez-Cedillo T, et al. Relationship of inflammatory profile of elderly patients serum and senescence-associated secretory phenotype with human breast cancer cells proliferation: role of IL6/IL8 ratio. Cytokine. (2017) 91:13–29. doi: 10.1016/j.cyto.2016.12.001
53. Zhang Y, Ertl HC. Aging: T cell metabolism within tumors. Aging. (2016) 8:1163–4. doi: 10.18632/aging.100979
54. Freedman RA. Treatment of breast cancer in the elderly. Curr Oncol Rep. (2015) 17:51. doi: 10.1007/s11912-015-0475-8
55. Terret C, Russo C. Pharmacotherapeutic management of breast cancer in elderly patients: the promise of novel agents. Drugs Aging. (2018) 35:93–115. doi: 10.1007/s40266-018-0519-5
Keywords: breast cancer, immune score, stromal score, overall survival, aging
Citation: Li B, Geng R, Wu Q, Yang Q, Sun S, Zhu S, Xu Z and Sun S (2020) Alterations in Immune-Related Genes as Potential Marker of Prognosis in Breast Cancer. Front. Oncol. 10:333. doi: 10.3389/fonc.2020.00333
Received: 24 November 2019; Accepted: 25 February 2020;
Published: 12 March 2020.
Edited by:
Benjamin Frey, University of Erlangen Nuremberg, GermanyReviewed by:
Luis De La Cruz-Merino, Hospital Universitario Virgen Macarena, SpainPaul Andrew Beavis, Peter MacCallum Cancer Centre, Australia
Copyright © 2020 Li, Geng, Wu, Yang, Sun, Zhu, Xu and Sun. This is an open-access article distributed under the terms of the Creative Commons Attribution License (CC BY). The use, distribution or reproduction in other forums is permitted, provided the original author(s) and the copyright owner(s) are credited and that the original publication in this journal is cited, in accordance with accepted academic practice. No use, distribution or reproduction is permitted which does not comply with these terms.
*Correspondence: Zhiliang Xu, strongcici850720@sina.com; Shengrong Sun, sun137@sina.com
†These authors have contributed equally to this work