- 1Cell Signal Transduction Laboratory, Bioinformatics Center, School of Basic Medical Sciences, School of Software, Institute of Biomedical Informatics, Henan University, Kaifeng, China
- 2Department of Anesthesia, Stanford University, Stanford, CA, United States
Prognostic biomarkers are of great significance to predict the outcome of patients with cancer, to guide the clinical treatments, to elucidate tumorigenesis mechanisms, and offer the opportunity of identifying therapeutic targets. To screen and develop prognostic biomarkers, high throughput profiling methods including gene microarray and next-generation sequencing have been widely applied and shown great success. However, due to the lack of independent validation, only very few prognostic biomarkers have been applied for clinical practice. In order to cross-validate the reliability of potential prognostic biomarkers, some groups have collected the omics datasets (i.e., epigenetics/transcriptome/proteome) with relative follow-up data (such as OS/DSS/PFS) of clinical samples from different cohorts, and developed the easy-to-use online bioinformatics tools and web servers to assist the biomarker screening and validation. These tools and web servers provide great convenience for the development of prognostic biomarkers, for the study of molecular mechanisms of tumorigenesis and progression, and even for the discovery of important therapeutic targets. Aim to help researchers to get a quick learning and understand the function of these tools, the current review delves into the introduction of the usage, characteristics and algorithms of tools, and web servers, such as LOGpc, KM plotter, GEPIA, TCPA, OncoLnc, PrognoScan, MethSurv, SurvExpress, UALCAN, etc., and further help researchers to select more suitable tools for their own research. In addition, all the tools introduced in this review can be reached at http://bioinfo.henu.edu.cn/WebServiceList.html.
Introduction
The prognosis estimation of tumor patient is of great significance to guide clinical treatments and facilitate the elucidation of tumorigenesis mechanism. In current clinical practice, prognosis is determined by many factors, such as disease stage, clinical performance, treatment experience and understanding of the cancer development. However, these properties are relative subjective and may lead to inaccurate prognostic estimates, and may even lead to inappropriate anticancer management strategy. Genotype-Tissue Expression (GTEx) and the Cancer Genome Atlas (TCGA) projects offer a large number of RNA sequence data of normal and cancer samples, providing unprecedented opportunities for many fields such as cancer bioinformatics and precision medicine to improve our understanding in cancer development and treatment (1, 2). Molecular prognostic biomarkers are the basic components of precision medicine. Data mining and other biological analysis make it possible to predict the prognosis of tumors at the molecular level (3–5). Accurate clinical estimation using prognostic biomarkers helps determining optimal anti-cancer treatment. At the same time, it provides assistance in developing more detailed hospice care plans. So in recent years, the discovery of prognostic biomarkers has become a hot topic in precision medicine.
Numerous studies have evidenced that molecular markers in DNA, RNA and protein level can be as prognostic biomarkers in cancer, and guide the effect of treatment either independently or in addition with present prognosis systems (6–8). In these study, Kaplan-Meier method and multivariate Cox proportional hazards regression models were commonly used to evaluate the associations between molecular markers and survival of patients with cancer (9, 10). However, these biomarkers are not suitable for clinical application due to the lack of independent validation and poor repeatability between different studies.
Mining data from public datasets and making assessments and predictions can be challenging and time-consuming. To extract useful information from these datasets, it requires researchers with strong bioinformatics expertise. To allow more researchers be able to quickly extract information they need, online tools that can easily perform survival analysis from these data are needed. The rapid growth of public datasets has enabled some research groups to focus on collecting omics datasets and developing online bioinformatics prognostic tools and web servers. These various prognostic analysis tools provide valuable evidence and ideas for cancer researchers. However, for many researchers and clinicians, it may be difficult to find the most suitable tool for their own research quickly. This review attempts to provide a comprehensive overview of the commonly used online prognostic tools for cancer prognostic analysis. In addition, the main challenges and future directions in this field are also discussed in this paper.
Materials and Methods
Literature research and data collection: the survival analysis tools reviewed in this paper include online prognostic bioinformatics tools and web servers developed by applying different types of profiling data (genomics, epigenomics, proteomics etc.) from clinical samples of different cohorts. Search Strategy for prognostic tools was executed in PubMed and Google Scholar from Jan 1, 2000 to August 31, 2019. Search terms include: “survival analysis,” “web server,” “prognostic biomarker” and “cancer,” keywords combination was used for search. The search was limited to English language. There are 886 articles that matched to above criteria. In the review, 22 representative databases that can be used for the prognosis analysis of multiple cancer types were selected for detailed description; because most of the prognostic tools for single type of cancer were included in the above databases, so we just gave a brief introduction. Ten of these databases are based on mRNA profiling data for prognostic analysis, three databases based on ncRNA profiling data, two databases based on protein data, two databases based on DNA data, and five databases based on multi-omics data. The literature retrieval process is shown in Figure 1. The release time of prognostic databases is presented in Figure 2. The date of the last search and collating data for these databases was December 10, 2019.
Results
Web Servers for Survival Analysis Based on mRNA Data
In the past two decades, high-throughput gene chips and next-generation sequencing technologies have provided opportunities to explore important cancer-related molecules, therapeutic targets, diagnostic, and prognostic biomarkers. With the implementation of the Cancer Genome Atlas (TCGA) project, a large number of epigenome, transcriptome, and proteome data of tumor samples became publicly accessible. Researchers can analyze the correlation between these data and survival, and look for prognostic biomarkers. Many studies have shown that mRNA expression is closely related to cancer prognosis (11–13). In order to promote the development and evaluation of prognostic biomarkers, some research groups have developed prognosis tools and web servers based on mRNA data by mining TCGA and GEO (Gene Expression Omnibus) data and adding complex statistical calculation. This review introduces 14 bioinformatics tools for evaluating cancer prognosis based on mRNA data (Table 1).
LOGpc1
LOGpc is a web server that contains a large number of datasets for survival analysis, which provides 13 types of survival terms for 28,098 cancer patients from 26 types of malignant tumors, including OSlms, OSblca, OSkirc and other 23 online prognostic tools (14–21). These patient samples were collected mainly from TCGA and GEO cohorts. LOGpc is free and easy to operate. Twenty six types of tumors are classified into 11 system categories according to TCGA. Currently, only official gene symbol input is acceptable in LOGpc. When user input the gene symbol and set the relative parameters, then click on the “Kaplan-Meier plot” button and the results will be displayed on the output webpage. In order to meet the specific needs from different researchers, clinical confounding factors can also be defined for advanced subgroup analysis.
GENT22
GENT2 provides the differential expression analysis and prognosis analysis based on tumor subtypes (22). The users can search the gene expression profiles of different tissues, and compare the expression levels between tissue subtypes. For survival analysis, this tool provides Kaplan Meier plot with log rank test and establishing Cox proportional risk model for meta-analysis. At present, it provides survival analysis for 27 cancer types, including 46 subtypes of 19 cancer types.
PROGgeneV23
PROGgeneV2 is a web-based tool for studying the prognosis of genes in a variety of cancers (23, 24). In current it comprises 193 datasets for 27 cancer types. The users can perform survival analysis of single gene, multi genes and two genes expression ratio, and also use the function of adjusting covariate survival model. Users can upload customized gene datasets for survival analysis of interested genes and compare the results with previously published studies.
SurvExpress4
SurvExpress is for studying risk assessment and survival analysis. It contains more than 29,000 samples of 26 cancer types with clinical information from 144 datasets (25). The outputs generated by SurvExpress include the Kaplan-Meier plots by risk group, a heat map of gene expression values and a visual association of available clinical information to risk groups. Survival ROC estimates the specificity and time-dependent sensitivity for survival risk groups.
PRECOG5
PRECOG is a system for integrating genomic profiles and cancer clinical data, it covers 39 different cancer types, including about 19,000 samples with overall survival data from 165 cancer expression datasets (26). It allows researchers to query whether gene expression correlates with patient survival. For simple display, 39 different histologic types of tumors were divided into 18 groups. The correlation between gene expression and overall survival was assessed by univariate Cox regression. PRECOG also provides gene prognosis analysis for pan-cancer. However, new users need to register and log in.
Oncomine6
Oncomine is a cancer gene chip database and integrated data mining platform, aiming at mining cancer gene information (27, 28). Oncomine has more complete cancer mutation spectrum, gene expression data and related clinical information, which provides insights to identify new biomarkers or new therapeutic targets. With Oncomine, users can get the results of differential expression, co-expression analysis, molecular concepts analysis, interaction network, correlation analysis between gene expression and survival status, but Kaplan-Meier plot isn't displayed directly. Meta-analysis can also be used to compare various studies to determine more reliable and consistent results. Oncomine Research Edition is free, but needs a valid academic email address to register and log in.
PrognoScan7
Prognoscan is a platform for predicting the relationship between gene expression and patient survival based on a large number of public cancer microarray datasets with clinical information. It provides a variety of survival terms for 14 cancer types (29). One of its advantages is that survival analysis in this tool performs the minimum P-value method and optimal cut-off is provided.
KMplotter8
The Kaplan Meier plotter (KMplotter) can be used for single gene or multiple gene prognosis analysis for many kinds of malignant tumors (30–32). Researchers can assess the effect of mRNA and miRNA expression on the survival rate of 21 cancer types by pan-cancer analysis. When the users input the relevant gene name and select the appropriate gene expression cut-off point, the comparison results between the two groups will be displayed with 95% confidence interval, risk ratio and log rank P-value. An Auto best cut-off is provided to compute all possible cut-off values to get the best performing threshold in survival analysis.
GSCALite9
GSCALite is a tool for analyzing expression/variation/ clinical correlation of gene sets in cancers with dynamic and visualization manner (33). It provides three survival analysis modules for a gene set based on cancer multi-omics data of TCGA. (1) Differential mRNA expression of gene set between tumor and matched normal samples, gene expression between subtypes of each selected cancer, and its effect on overall survival rate. (2) The influence of SNV (single nucleotide variants) frequency and mutation type of gene set on the overall survival rate in a cancer type. (3) Differential expression of methylation between tumor and matched normal samples, and the effect on the survival rate of selected cancer types. It allows users to search for prognostic markers at transcriptome level, epigenetic modification, and DNA mutation. Users can query the cancer pathway activity related to gene expression and the correlation between genes and drug sensitivity, it is convenient for researchers to study drug resistance of tumor.
UALCAN10
UALCAN is a web-based tool for analyzing TCGA RNA-seq and clinical data to evaluate the association of gene expression and patient survival, allows users to conduct differential expression analysis and survival analysis for interested genes and access the expression and survival information of a given gene in 31 types of cancers by performing pan-cancer analysis (34). Currently, UALCAN provides protein differential expression analysis for breast cancer, colon cancer, and other three cancer types, but does not provide survival analysis based on protein data. UALCAN also provides additional information about the selected genes or targets by linking to Pubmed, TargetScan, DRUGBANK, and so on, this helps researchers collect more valuable information and data.
GEPIA11
GEPIA is an interactive web-based tool for survival analysis based on gene expression, it offer the choice of selecting overall survival (OS) or disease-free survival (DFS) for the analysis (35, 36). According to the characteristics of gene normalization, GEPIA allows two different genes to be input at the same time for survival analysis. GEPIA also presents the top genes most related to the survival of cancer patients. This function is very helpful for the users. In addition to providing patient survival analysis, GEPIA has other functions such as differential expression analysis based between different cancer types, multiple gene comparison, similar genes detection.
CAS-Viewer12
CAS-viewer is a web-based tool for multiple level comprehensive analysis by integrating multi-omics data such as mRNA, miRNA, methylation, SNP, and clinical information across different cancer types (37). It links the differential transcriptional expression rate with methylation, miRNA, and splicing regulatory elements of 33 cancer types. “Clinical correlation” module presents Kaplan Meier plot showing the correlation between PSI (percent spliced in) value and survival rate, and in this way users can identify potential transcripts related to different survival outcomes of each cancer type.
MEXPRESS13
MEXPRESS is an intuitive web tool for analysis of gene expression, DNA methylation, and association with clinical information including patient survival (38). It provides a very different visual interface, allows users to compare specific genomic features (such as DNA methylation) with gene expression and clinical information. Researchers can study the relationship between DNA methylation and gene expression and multiple clinical variables by using MEXPRESS platform.
CaPSSA14
CaPSSA supports users to detect the prognostic value of patient subgroups based on gene expression, mutation or genomic alterations of query genes (39). Importantly, it also supports custom histochemical data analysis with clinical information. For candidate gene sets that user-supplied, interactive patient stratification is supported based on gene expression profiles and genomic alterations, the results of log-rank test and Kaplan Meier plots will be displayed for evaluating the prognostic value.
Web Servers for Studying Prognostic Implications of ncRNA
In the past decade, a large number of studies have shown that non-coding RNA (ncRNA) plays an increasingly important role in epigenetic regulation. ncRNAs involved in the network can affect many molecular targets which are related to the development of cancer, and many ncRNAs are considered as driving factors or suppressors of carcinogenesis (40). MicroRNA (miRNA) as one type of ncRNAs regulates mRNA at the transcriptional or post-transcriptional level (41). Studies have shown that lncRNA (long non-coding RNA) plays an important role in many life activities such as dose compensation effect, epigenetic regulation, cell cycle and cell differentiation, and has become a hot spot in tumor genetics research (42). Their expression in cancer has been studied by high-throughput methods, generating valuable sources of public available datasets. An important step in developing ncRNA biomarkers is to evaluate them in independent cohorts. To help and simplify the assessment of ncRNA signatures in cancer prognosis, several ncRNA prognostic databases have been developed by some research teams using public profiling data (Table 2).
PROGmiRV215
PROGmiRV2 is a pan-cancer miRNA prognostics database, whose miRNA data comes from GEO and TCGA (43). Compared with version 1, the datasets and samples of the new version have increased greatly, prognosis analysis has been improved from single cancer type analysis to pan-cancer analysis, and the survival indicators provided have increased from one to three (overall survival, recurrence free survival, and metastasis free survival). Users are also allowed to upload their own customized dataset for prognosis analysis, but registration and login are required.
SurvMicro16
SurvMicro is a bioinformatics tool for analyzing cancer prognosis based on miRNA. Its data comes from GEO, TCGA, and ArrayExpress (44). SurvMicro comprises 43 datasets and more than 6,000 samples in 15 different cancer types. Cox multiple fitting was used to evaluate the risk of prognosis, the prognosis index was obtained by calculating the sum of miRNA expression value and Cox coefficients. According to the ranking of prognosis index, users would know the risk group of poor prognosis.
OncoLnc17
OncoLnc is an interactive tool for studying survival correlations for lncRNA, miRNA, and mRNA (45, 46). OncoLnc contains patient survival data of 21 cancer types from TCGA mRNAs, miRNAs, and MiTranscriptome data. The users can divide patients into subgroups according to gene expression levels, measure the result between subgroups. OncoLnc allows users to view the results of Kaplan Meier plots of one or multiple types of cancers at one time, provide Cox regression results, and download the full data used in the analysis. It also allows users to explore the survival relevance of inquired genes in 21 types of cancers at one time, this function is helpful to study whether specific genes play important roles in cancer prognosis.
TANRIC18
TANRIC is an interactive platform for multiple analysis of lncRNA in cancer (47). It includes the expression profile of lncRNA in more than 6,000 patient samples of 20 cancer types from TCGA and other three independent datasets. TANRIC consists of six modules, users can get the annotation data of lncRNA through module “My lncRNA,” and analyze whether lncRNA is related to the survival time of patients (including subtypes prognosis analysis). Users can also use other functions TANRIC to recognize the differential expression of lncRNA in tumor and normal tissue, as well as in tumor subtype or tumor stage, evaluate the differential expression of lncRNA in wild type and gene mutation cancer, evaluate the influence of lncRNA expression on drug sensitivity, and find some signal pathways related to cancer subtype defined by lncRNA.
Web Servers for Survival Analysis Based on Protein Data
Functional proteomics is a powerful way to understand the pathophysiological mechanism and find the therapeutic target of cancer. In order to find biomarkers for prognosis and targets for treatment improvement, it is necessary to study the correlation between protein and survival. As a part of the Cancer Genome Atlas (TCGA) Project and other works, reverse-phase protein array (RPPA) was used to measure the protein expression in a large number of clinical cancer samples and cell lines (48, 49). This technology provides a necessary condition for the establishment of repeatable prediction model and protein prediction database. Here, we introduce two protein survival analysis databases based on RPPA data (Table 3).
TCPAv3.019
TCPAv3.0 is an updated version of TCPA to explore and analyze protein expression based on TCGA RPPA data (50, 51). It integrates protein data and other TCGA data (somatic mutations, SCNAs, DNA methylation, mRNA and miRNA expression, and patient clinical information) and gives comprehensive protein-centric analyses. The users can find protein markers or pathway events that are significantly related to patient survival by using Cox proportional risk model and log rank test. The users can identify which proteins associated with the prognosis of different cancers and subtypes by pan-cancer analysis. The pan-cancer analysis module using multi-omic TCGA data provides researchers a unique way to validate specific protein-driven multi-omic hypotheses in multiple cancer types.
TRGAted20
TRGAted is an intuitive tool for analyzing the correlation between more than 200 proteins and survivals in 31 types of cancers (52). RPPA data (Level 4) contained in TRGAted come from the TCPA Portal. The cancer clinical information provided are comprehensive, including: gender, age, tumor stage, histological type, response to treatment. Users can use Cox proportional hazard model to analyze the prognosis of all proteins in each cancer type, or for a single protein across all cancer types. Comparison with TCPAv3.0, TRGAted provides more survival indicators, and its function of visualizing all proteins in a cancer type can help researchers find survival related proteins in the specific cancer more easily. The users are allowed to download and modify TRGAted for better usability under GPLv3 (GNU General Public License v3.0).
Web Servers for Prognosis Analysis Based on DNA Data
Patients with genetic mutations in tumor cells are more likely to display poor pathological features, resulting in significantly altered overall survival (53). The new generation of sequencing technology has accelerated the study of somatic genetics, identifying patient subgroups with different genomic alteration patterns could facilitate to stratify patients with different clinical outcomes and to propose putative biomarkers. In addition to DNA mutation, DNA methylation is the most studied epigenetic modification which is crucial for facilitating vital biological processes such as embryonic development, genomic imprinting, and X-chromosome inactivation. Aberrant DNA methylation may lead to changes in cellular micro-environment, affect the gene expression pattern, and ultimately result in various pathological conditions including carcinogenesis (54, 55). Several recently developed high-throughput techniques facilitate genome-wide DNA methylation profiling. Some prognostic tools were also developed to facilitate the evaluation of the prognostic properties of CpG methylation data (Table 4).
MethSurv21
MethSurv is a web tool dedicating for survival analysis based on DNA methylation data including 7,358 samples in 25 different cancer types from TCGA (56). Methsurv provides multiple survival terms analysis, and the home page contains the following modules: single CpG, region based analysis, all cancers, top biomarkers, and gene visualization. Users can retrieve CpG survival analysis results of selected areas of a chromosome, and also search for a gene of interest to explore the survival statistics of all CpGs available. Users can see top biomarkers arranged according to p-value of all CpG labeled cancer types in the whole genome. In brief, MethSurv is a valuable platform for preliminary screening of methylation cancer biomarkers.
cBioPortal22
cBioPortal provides a visual tool for interactive exploration of multiple cancer genomic datasets (57, 58). It integrates and simplifies the data including somatic mutation, mRNA and microRNA expression, DNA copy-number alterations(CNAs) and methylation, protein, and phosphoprotein RPPA data, so that the users can obtain graphical summaries of large-scale cancer genomic data intuitively. It enables users to inquiry survival analysis based on DNA mutation data and CNA data, the results of OS, and DFS of patients are presented intuitively in the form of Kaplan-Meier plots. Pan-cancer analysis is also allowed.
Prognostic Tools for Single Type of Cancer
Through literature search, 11 prognostic tools for single type of cancer were found (Table 5). MiRpower is a part of KMplotter database to analyze the prognostic relevance of miRNAs in breast cancer (31). OSlms, OSescc, OSkirc, OSblca, OScc, OSbrca, OSacc, and OSuvm are bioinformatics tools included in the LOGpc platform for survival analysis of leiomyosarcoma, esophageal squamous cell carcinoma, kidney renal clear cell carcinoma, bladder cancer, cervical cancer, breast cancer, adrenocortical carcinoma, and uveal melanoma (14–21). OvMark and BreastMark are online web servers for prognosis analysis of ovarian cancer and breast cancer, users can detect the prognostic potential of about 17,000 genes and 341 miRNAs in ovarian cancer and breast cancer (59, 60).
Discussion
The development of public databases (such as TCGA and GEO) provides a large number of genomic, epigenomic, transcriptional and proteomic data, and provides the possibility for gene function analysis and biological mechanism discussion (1, 2). The rapid growth of multi-omics data provides more opportunities for the research of cancer molecular mechanism and biological target, but for the researchers without strong computing power and bioinformatics background, they might face many difficulties and challenges in data mining and analysis. Since the EAPC (European Association for Palliative Care) made recommendations for the development of cancer prognostic tools in 2005, a number of prognostic tools have been developed, evolved, and validated (61). In this review, we summarized 22 prognostic bioinformatics tools, which provide survival analysis or with other functions. We analyzed and compared their key information and characteristics, follow-up information for each tool is presented in Table 6, strength and limitation are displayed in additional files (Table S1). With these tools, researchers can easily explore a large number of datasets from complex data platform, find genes, ncRNAs, proteins, gene modifications, or mutations associated with patient survival, ask specific questions and test their hypotheses (48, 62, 63). Comprehensive expression analysis can be carried out by simple clicks, which greatly promotes data mining in research fields, scientific discussions and treatment discovery processes. These tools have the potentials to integrate and personalize the prognostic information for individual patients and provide refined risk estimates for uncertain clinical management scenarios. Meanwhile each database has its own strengths. Some databases focus on survival analysis by collecting datasets of various cancer types, such as LOGpc, PROGgeneV2, KM Plotter, PrognoScan, TRGAted. Some databases provide other functions, UALCAN, and GEPIA have the function of top differential gene display, which provide a way for clinicians and researchers to select possible target genes for diagnosis or treatment, Oncomine, and TCPA provide multidimensional analysis and comparison of datas. GSCALite, TANRIC can be used for drug screening and treatment options by analyzing the correlation between therapeutic targets and lncRNAs. Advances in genome technology and computational biology provide us with an unprecedented opportunity to understand molecular events associated with cancer, and to apply precise cancer treatment. We hope this review will be helpful to clinicians and oncologists who are interested in finding prognostic or predictive features of cancer.
Limitation and Prospective
Although these tools provide great convenience for prognostic biomarker development, several key aspects of these prognostic tools remain elusive. Differences in datasets collected and split points may result in significantly different results, so we collected datasets and their source of these web servers (Figure 3 and Tables S2–S5) and found excluding TCGA data, there are significant differences in other data sources. This may be one of the reasons why the analysis results of different tools are not completely consistent. In the future, efforts should be made in data optimization, prognostic tools should be improved to be able to predict multi-gene markers, select optimal cut-off computation, use hierarchical clustering and consider complex multi-omics networks of interactions. In addition more molecular subtypes and clinical information including tumor tissue image and treatment data should be collected and mined to identify more meaningful prognostic markers through more detailed subtype analysis.
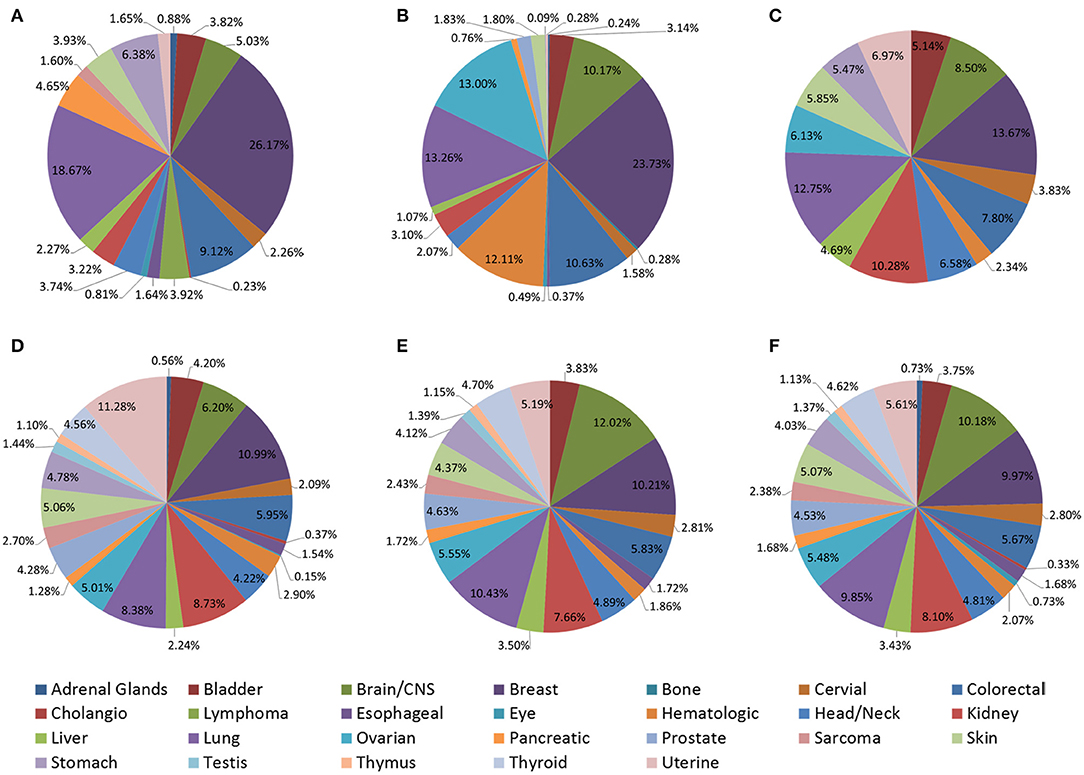
Figure 3. Distribution of cancer types in web servers. (A) LOGpc (mRNA level); (B) PROGmiRV2 (miRNA level); (C) OncoLnc (lncRNA level); (D) CaPSSA (mutation level); (E) GSCALite (methylation level); (F) TCPAv3.0 (protein level).
Author Contributions
HZ, GZ, LZ, QW, and XG collected data, set up web pages, and drafted the paper. HL, YH, LX, ZY, YL, YA, HD, and WZ contributed to critical revision of the manuscript for intellectual content. All authors edited and approved the final manuscript.
Funding
This study was supported by National Natural Science Foundation of China (No. 81602362), Supporting grants of Henan University (No. 2015YBZR048; No. B2015151), Yellow River Scholar Program (No. H2016012), and Program for Innovative Talents of Science and Technology in Henan Province (No. 18HASTIT048), Program for Science and Technology Development in Henan Province (No. 162102310391, No. 172102210187), Program for Scientific and Technological Research of Henan Education Department (No. 14B520022), Program for Young Key Teacher of Henan Province (2016GGJS-214), Kaifeng Science and Technology Major Project (18ZD008), Supporting grant of Bioinformatics Center of Henan University (No. 2018YLJC01).
Conflict of Interest
The authors declare that the research was conducted in the absence of any commercial or financial relationships that could be construed as a potential conflict of interest.
Supplementary Material
The Supplementary Material for this article can be found online at: https://www.frontiersin.org/articles/10.3389/fonc.2020.00068/full#supplementary-material
Table S1. Feature analysis of included prognostic web servers.
Table S2. Datasets and samples of prognostic web servers based on mRNA.
Table S3. Datasets and samples of prognostic web servers based on ncRNA.
Table S4. Datasets and samples of prognostic web servers based on protein.
Table S5. Datasets and samples of prognostic web servers based on DNA methylation and mutation.
Footnotes
1. ^http://bioinfo.henu.edu.cn/DatabaseList.jsp
3. ^http://genomics.jefferson.edu/proggene/
4. ^http://bioinformatica.mty.itesm.mx:8080/Biomatec/SurvivaX.jsp
5. ^https://precog.stanford.edu/
7. ^http://www.prognoscan.org/
8. ^http://kmplot.com/analysis/
9. ^http://bioinfo.life.hust.edu.cn/web/GSCALite/
10. ^http://ualcan.path.uab.edu/index.html
11. ^http://gepia.cancer-pku.cn/
12. ^http://genomics.chpc.utah.edu/cas/
15. ^http://xvm145.jefferson.edu/progmir/
16. ^http://bioinformatica.mty.itesm.mx/SurvMicro
20. ^https://nborcherding.shinyapps.io/TRGAted
References
1. Tomczak K, Czerwinska P, Wiznerowicz M. The cancer genome atlas (TCGA): an immeasurable source of knowledge. Contemp Oncol. (2015) 19:A68–77. doi: 10.5114/wo.2014.47136
2. Barrett T, Wilhite SE, Ledoux P, Evangelista C, Kim IF, Tomashevsky M, et al. NCBI GEO: archive for functional genomics data sets–update. Nucleic Acids Res. (2013) 41:D991–5. doi: 10.1093/nar/gks1193
3. Xu XL, Gong Y, Zhao DP. Elevated PHD2 expression might serve as a valuable biomarker of poor prognosis in lung adenocarcinoma, but no lung squamous cell carcinoma. Eur Rev Med Pharmacol Sci. (2018) 22:8731–9. doi: 10.26355/eurrev_201812_16638
4. Sun D, Wang X, Sui G, Chen S, Yu M, Zhang P. Downregulation of miR-374b-5ppromotes chemotherapeutic resistance in pancreatic cancer by upregulating multiple anti-apoptotic proteins. Int J Oncol. (2018) 52:1491–503. doi: 10.3892/ijo.2018.4315
5. Yang J, Li A, Li Y, Guo X, Wang M. A novel approach for drug response prediction in cancer cell lines via network representation learning. Bioinformatics. (2019) 35:1527–−35. doi: 10.1093/bioinformatics/bty848
6. Hong L, Han Y, Zhang H, Fan D. Prognostic markers in esophageal cancer: from basic research to clinical use. Expert Rev Gastroenterol Hepatol. (2015) 9:887–9. doi: 10.1586/17474124.2015.1041507
7. Kang H, Kiess A, Chung CH. Emerging biomarkers in head and neck cancer in the era of genomics. Nat Rev Clin Oncol. (2014) 12:11–26. doi: 10.1038/nrclinonc.2014.192
8. Burkhart RA, Ronnekleiv-Kelly SM, Pawlik TM. Personalized therapy in hepatocellular carcinoma: molecular markers of prognosis and therapeutic response. Surg Oncol. (2017) 26:138–45. doi: 10.1016/j.suronc.2017.01.009
9. Chou CK, Liu RT, Kang HY. MicroRNA-146b: a novel biomarker and therapeutic target for human papillary thyroid cancer. Int J Mol Sci. (2017) 18:636. doi: 10.3390/ijms18030636
10. Gu X, Xue J, Ai L, Sun L, Zhu X, Wang Y, et al. SND1 expression in breast cancer tumors is associated with poor prognosis. Ann N Y Acad Sci. (2018) 1433:53–60. doi: 10.1111/nyas.13970
11. Xie L, Dang Y, Guo J, Sun X, Xie T, Zhang L, et al. High KRT8 expression independently predicts poor prognosis for lung adenocarcinoma patients. Genes. (2019) 10:E36. doi: 10.3390/genes10010036
12. Szász AM, Lánczky A, Nagy Á, Förster S, Hark K, Green JE, et al. Cross-validation of survival associated biomarkers in gastric cancer using transcriptomic data of 1,065 patients. Oncotarget. (2016) 7:49322–33. doi: 10.18632/oncotarget.10337
13. Guerrero-Martínez JA, Reyes JC. High expression of SMARCA4 or SMARCA2 is frequently associated with an opposite prognosis in cancer. Sci Rep. (2018) 8:2043. doi: 10.1038/s41598-018-20217-3
14. Wang Q, Xie L, Dang Y, Sun X, Xie T, Guo J, et al. OSlms: a web server to evaluate the prognostic value of genes in leiomyosarcoma. Front Oncol. (2019) 9:190. doi: 10.3389/fonc.2019.00190
15. Wang Q, Wang F, Lv J, Xin J, Xie L, Zhu W, et al. Interactive online consensus survival tool for esophageal squamous cell carcinoma prognosis analysis. Oncol Lett. (2019) 18:1199–206. doi: 10.3892/ol.2019.10440
16. Xie L, Wang Q, Dang Y, Ge L, Sun X, Li N, et al. OSkirc: a web tool for identifying prognostic biomarkers in kidney renal clear cell carcinoma. Future Oncol. (2019) 15:3103–10. doi: 10.2217/fon-2019-0296
17. Zhang G, Wang Q, Yang M, Yuan Q, Dang Y, Sun X, et al. OSblca: a web server for investigating prognostic biomarkers of bladder cancer patients. Front Oncol. (2019) 9:466. doi: 10.3389/fonc.2019.00466
18. Wang Q, Zhang L, Yan Z, Xie L, An Y, Li H, et al. OScc: an online survival analysis web server to evaluate the prognostic value of biomarkers in cervical cancer. Future Oncol. (2019) 15:3693–9. doi: 10.2217/fon-2019-0412
19. Yan Z, Wang Q, Sun X, Ban B, Lu Z, Dang Y, et al. OSbrca: a web server for breast cancer prognostic biomarker investigation with massive data from tens of cohorts. Front Oncol. (2019) 9:1349. doi: 10.3389/fonc.2019.01349
20. Xie L, Wang Q, Nan F, Ge L, Dang Y, Sun X, et al. OSacc: gene expression-based survival analysis web tool for adrenocortical carcinoma. Cancer Manag Res. (2019) 11:9145–52. doi: 10.2147/CMAR.S215586
21. Wang F, Wang Q, Li N1, Ge L, Yang M, An Y, et al. OSuvm: an interactive online consensus survival tool for uveal melanoma prognosis analysis. Mol Carcinog. (2020) 59:56–61. doi: 10.1002/mc.23128
22. Park SJ, Yoon BH, Kim SK, Kim SY. GENT2: an updated gene expression database for normal and tumor tissues. BMC Med Genomics. (2019) 12:101. doi: 10.1186/s12920-019-0514-7
23. Goswami CP, Nakshatri H. PROGgene: gene expression based survival analysis web application for multiple cancers. J Clin Bioinform. (2013) 3:22. doi: 10.1186/2043-9113-3-22
24. Goswami CP, Nakshatri H. PROGgeneV2: enhancements on the existing database. BMC Cancer. (2014) 14:970. doi: 10.1186/1471-2407-14-970
25. Aguirre-Gamboa R, Gomez-Rueda H, Martínez-Ledesma E, Martínez-Torteya A, Chacolla-Huaringa R, Rodriguez-Barrientos A, et al. SurvExpress: an online biomarker validation tool and database for cancer gene expression data using survival analysis. PLoS ONE. (2013) 8:e74250. doi: 10.1371/journal.pone.0074250
26. Gentles AJ, Newman AM, Liu CL, Bratman SV, Feng W, Kim D, et al. The prognostic landscape of genes and infiltrating immune cells across human cancers. Nat Med. (2015) 21:938–45. doi: 10.1038/nm.3909
27. Rhodes DR, Yu J, Shanker K, Deshpande N, Varambally R, Ghosh D, et al. Oncomine: a cancer microarray database and integrated data-mining platform. Neoplasia. (2004) 6:1–6. doi: 10.1016/S1476-5586(04)80047-2
28. Rhodes DR, Kalyana-Sundaram S, Mahavisno V, Varambally R, Yu J, Briggs BB, et al. Oncomine 3.0: genes, pathways, and networks in a collection of 18,000 cancer gene expression profiles. Neoplasia. (2007) 9:166–80. doi: 10.1593/neo.07112
29. Mizuno H, Kitada K, Nakai K, Sarai A. PrognoScan: a new database for meta-analysis of the prognostic value of genes. BMC Med Genomics. (2009) 2:18. doi: 10.1186/1755-8794-2-18
30. Györffy B, Lanczky A, Eklund AC, Denkert C, Budczies J, Li Q, et al. An online survival analysis tool to rapidly assess the effect of 22,277 genes on breast cancer prognosis using microarray data of 1809 patients. Breast Cancer Res Treat. (2010) 123:725–31. doi: 10.1007/s10549-009-0674-9
31. Nagy Á, Lánczky A, Menyhárt O, Gyorffy B. Validation of miRNA prognostic power in hepatocellular carcinoma using expression data of independent datasets. Sci Rep. (2018) 8:9227. doi: 10.1038/s41598-018-27521-y
32. Lánczky A, Nagy Á, Bottai G, Munkácsy G, Szabó A, Santarpia L, et al. miRpower: a web-tool to validate survival-associated miRNAs utilizing expression data from 2178 breast cancer patients. Breast Cancer Res Treat. (2016) 160:439–46. doi: 10.1007/s10549-016-4013-7
33. Liu CJ, Hu FF, Xia MX, Han L, Zhang Q, Guo AY. GSCALite: a web server for gene set cancer analysis. Bioinformatics. (2018) 34:3771–2. doi: 10.1093/bioinformatics/bty411
34. Chandrashekar DS, Bashel B, Balasubramanya SAH, Creighton CJ, Ponce-Rodriguez I, Chakravarthi BVSK, et al. UALCAN: a portal for facilitating tumor subgroup gene expression and survival analyses. Neoplasia. (2017) 19:649–58. doi: 10.1016/j.neo.2017.05.002
35. Tang Z, Li C1, Kang B, Gao G, Li C, Zhang Z. GEPIA: a web server for cancer and normal gene expression profiling and interactive analyses. Nucleic Acids Res. (2017) 45:W98–102. doi: 10.1093/nar/gkx247
36. Tang Z, Kang B, Li C, Chen T, Zhang Z. GEPIA2: an enhanced web server for large-scale expression profiling and interactive analysis. Nucleic Acids Res. (2019) 47:W556–60. doi: 10.1093/nar/gkz430
37. Han S, Kim D, Kim Y, Choi K, Miller JE, Kim D, et al. CAS-viewer: web-based tool for splicing-guided integrative analysis of multi-omics cancer data. BMC Med Genomics. (2018) 11:25. doi: 10.1186/s12920-018-0348-8
38. Koch A, Jeschke J, Van Criekinge W, van Engeland M, De Meyer T. MEXPRESS update 2019. Nucleic Acids Res. (2019) 47:W561–5. doi: 10.1093/nar/gkz445
39. Jang Y, Seo J, Kim S, Lee S. CaPSSA: visual evaluation of cancer biomarker genes for patient stratification and survival analysis using mutation and expression data. Bioinformatics. (2019) 35:btz516. doi: 10.1093/bioinformatics/btz516
40. Anastasiadou E, Jacob LS, Slack FJ. Non-coding RNA networks in cancer. Nat Rev Cancer. (2018) 18:5–18. doi: 10.1038/nrc.2017.99
41. Zhu Y, Wang J, Wang F, Yan Z, Liu G, Ma Y, et al. Differential microRNA expression profiles as potential biomarkers for pancreatic ductal adenocarcinoma. Biochemistry. (2019) 84:575–82. doi: 10.1134/S0006297919050122
42. Li J, Li Z, Zheng W, Li X, Wang Z, Cui Y, et al. LncRNA-ATB: an indispensable cancer-related long noncoding RNA. Cell Prolif. (2017) 50:12381. doi: 10.1111/cpr.12381
43. Goswami CP, Nakshatri H. PROGmiR: a tool for identifying prognostic miRNA biomarkers in multiple cancers using publicly available data. J Clin Bioinform. (2012) 2:23. doi: 10.1186/2043-9113-2-23
44. Aguirre-Gamboa R, Trevino V. SurvMicro: assessment of miRNA-based prognostic signatures for cancer clinical outcomes by multivariate survival analysis. Bioinformatics. (2014) 30:1630–2. doi: 10.1093/bioinformatics/btu087
45. Anaya J. OncoRank: a pan-cancer method of combining survival correlations and its application to mRNAs, miRNAs, and lncRNAs. Peer J Preprints. (2016) 4:e2574. doi: 10.7287/peerj.preprints.2574v1
46. Anaya J. OncoLnc: linking TCGA survival data to mRNAs, miRNAs, and lncRNAs. Peer J Comput Sci. (2016) 2:e67. doi: 10.7717/peerj-cs.67
47. Li J, Han L, Roebuck P, Diao L, Liu L, Yuan Y, et al. TANRIC: an interactive open platform to explore the function of lncRNAs in cancer. Cancer Res. (2015) 75:3728–37. doi: 10.1158/0008-5472.CAN-15-0273
48. Li J, Akbani R, Zhao W, Lu Y, Weinstein JN, Mills GB, et al. Explore, visualize, and analyze functional cancer proteomic data using the cancer proteome atlas. Cancer Res. (2017) 77:e51–4. doi: 10.1158/0008-5472.CAN-17-0369
49. Hennessy BT, Lu Y, Gonzalez-Angulo AM, Carey MS, Myhre S, Ju Z, et al. A technical assessment of the utility of reverse phase protein arrays for the study of the functional proteome in non-microdissected human breast cancers. Clin Proteomics. (2010) 6:129–51. doi: 10.1007/s12014-010-9055-y
50. Li J, Lu Y, Akbani R, Ju Z, Roebuck PL, Liu W, et al. TCPA: a resource for cancer functional proteomics data. Nat Methods. (2013) 10:1046–7. doi: 10.1038/nmeth.2650
51. Chen MM, Li J, Wang Y, Akbani R, Lu Y, Mills GB, et al. TCPA v3.0: an integrative platform to explore the pan-cancer analysis of functional proteomic data. Mol Cell Proteomics. (2019) 18:S15–25. doi: 10.1074/mcp.RA118.001260
52. Borcherding N, Bormann NL, Voigt AP, Zhang W. TRGAted: a web tool for survival analysis using protein data in the Cancer Genome Atlas. F1000Res. (2018) 7:1235. doi: 10.12688/f1000research.15789.1
53. Swift SL, Lang SH, White H, Misso K, Kleijnen J, Quek RG. Effect of DNA damage response mutations on prostate cancer prognosis: a systematic review. Future Oncol. (2019) 15:3283–303. doi: 10.2217/fon-2019-0298
54. Gyorffy B, Bottai G, Fleischer T, Munkácsy G, Budczies J, Paladini L, et al. Aberrant DNA methylation impacts gene expression and prognosis in breast cancer subtypes. Int J Cancer. (2016) 138:87–97. doi: 10.1002/ijc.29684
55. Chen X, Zhao C, Zhao Z, Wang H, Fang Z. Specific glioma prognostic subtype distinctions based on DNA methylation patterns. Front Genet. (2019) 10:786. doi: 10.3389/fgene.2019.00786
56. Modhukur V, Iljasenko T, Metsalu T, Lokk K, Laisk-Podar T, Vilo J. MethSurv: a web tool to perform multivariable survival analysis using DNA methylation data. Epigenomics. (2018) 10:277–88. doi: 10.2217/epi-2017-0118
57. Gao J, Aksoy BA, Dogrusoz U, Dresdner G, Gross B, Sumer SO, et al. Integrative analysis of complex cancer genomics and clinical profiles using the cBioPortal. Sci Signal. (2013) 6:pl1. doi: 10.1126/scisignal.2004088
58. Cerami E, Gao J, Dogrusoz U, Gross BE, Sumer SO, Aksoy BA, et al. The cBio cancer genomics portal: an open platform for exploring multidimensional cancer genomics data. Cancer Discov. (2012) 2:401–4. doi: 10.1158/2159-8290.CD-12-0095
59. Madden SF, Clarke C, Stordal B, Carey MS, Broaddus R, Gallagher WM, et al. OvMark: a user-friendly system for the identification of prognostic biomarkers in publically available ovarian cancer gene expression datasets. Mol Cancer. (2014) 13:241. doi: 10.1186/1476-4598-13-241
60. Madden SF, Clarke C, Gaule P, Aherne ST, O'Donovan N, Clynes M, et al. BreastMark: an integrated approach to mining publicly available transcriptomic datasets relating to breast cancer outcome. Breast Cancer Res. (2013) 15:R52. doi: 10.1186/bcr3444
61. Simmons CPL, McMillan DC, McWilliams K, Sande TA, Fearon KC, Tuck S, et al. Prognostic tools in patients with advanced cancer: a systematic review. J Pain Symptom Manage. (2017) 53:962–70. doi: 10.1016/j.jpainsymman.2016.12.330
62. Deng JL, Xu YH, Wang G. Identification of potential crucial genes and key pathways in breast cancer using bioinformatic analysis. Front Genet. (2019) 10:695. doi: 10.3389/fgene.2019.00695
63. Coebergh van den Braak RRJ, Sieuwerts AM, Kandimalla R, Lalmahomed ZS, Bril SI, van Galen A, et al. High mRNA expression of splice variant SYK short correlates with hepaticdisease progression in chemonaive lymph node negative colon cancer patients. PLoS ONE. (2017) 12:e0185607. doi: 10.1371/journal.pone.0185607
Keywords: web server, tool, prognosis, survival, cancer
Citation: Zheng H, Zhang G, Zhang L, Wang Q, Li H, Han Y, Xie L, Yan Z, Li Y, An Y, Dong H, Zhu W and Guo X (2020) Comprehensive Review of Web Servers and Bioinformatics Tools for Cancer Prognosis Analysis. Front. Oncol. 10:68. doi: 10.3389/fonc.2020.00068
Received: 31 October 2019; Accepted: 15 January 2020;
Published: 05 February 2020.
Edited by:
Pasquale Simeone, Università degli Studi G. d'Annunzio Chieti e Pescara, ItalyReviewed by:
Daniele Vergara, University of Salento, ItalyChenkai Ma, Commonwealth Scientific and Industrial Research Organisation (CSIRO), Australia
Copyright © 2020 Zheng, Zhang, Zhang, Wang, Li, Han, Xie, Yan, Li, An, Dong, Zhu and Guo. This is an open-access article distributed under the terms of the Creative Commons Attribution License (CC BY). The use, distribution or reproduction in other forums is permitted, provided the original author(s) and the copyright owner(s) are credited and that the original publication in this journal is cited, in accordance with accepted academic practice. No use, distribution or reproduction is permitted which does not comply with these terms.
*Correspondence: Xiangqian Guo, eHFndW9AaGVudS5lZHUuY24=
†These authors have contributed equally to this work