- 1Cell Signal Transduction Laboratory, Department of Preventive Medicine, Bioinformatics Center, School of Basic Medical Sciences, School of Software, Institute of Biomedical Informatics, Henan University, Kaifeng, China
- 2Department of Anesthesia, Stanford University, Stanford, CA, United States
Potential prognostic mRNA biomarkers are exploited to assist in the clinical management and treatment of breast cancer, which is the first life-threatening tumor in women worldwide. However, it is technically challenging for untrained researchers to process high dimensional profiling data to screen and validate the potential prognostic values of genes of interests in multiple cohorts. Our aim is to develop an easy-to-use web server to facilitate the screening, developing, and evaluating of prognostic biomarkers in breast cancers. Herein, we collected more than 7,400 cases of breast cancer with gene expression profiles and clinical follow-up information from The Cancer Genome Atlas and Gene Expression Omnibus data, and built an Online consensus Survival analysis web server for Breast Cancers, abbreviated OSbrca, to generate the Kaplan–Meier survival plot with a hazard ratio and log rank P-value for given genes in an interactive way. To examine the performance of OSbrca, the prognostic potency of 128 previously published biomarkers of breast cancer was reassessed in OSbrca. In conclusion, it is highly valuable for biologists and clinicians to perform the preliminary assessment and validation of novel or putative prognostic biomarkers for breast cancers. OSbrca could be accessed at http://bioinfo.henu.edu.cn/BRCA/BRCAList.jsp.
Introduction
Breast cancer is one of the leading cancers and the primary cause of mortality in women. The global burden of breast cancer is still increasing (1). It is predicted that by 2021, the incidence of breast cancer will increase to 85 per 100,000 women in China (2). Currently, clinicopathological risk factors are primarily used to estimate prognosis. These clinicopathological risks include stage, histological grade, tumor size, lymph node infiltrate, and so on (3). Molecular subtypes influence the survival of breast cancer. According to three protein expression statuses [estrogen receptor, progesterone receptor, and human epidermal growth factor receptor 2 (HER2)], breast cancer can be categorized into four classes: luminal A, luminal B, basal-like, and HER2+ (4). Because of the heterogeneity and survival difference of breast cancer, the utmost interests for researchers are how to validate the prognostic and predictive candidate genes in appropriately powered breast cancer cohorts using the massive published expression levels of various genes profiles with clinical outcome.
So far, a number of poor clinical outcome associated genes have been identified. The most famous prognostic significance of breast cancer is the estrogen receptor gene, which is expressed in 50–70% of clinical tumor cases (5). Progesterone receptor and HER2 are two other important prognostic-related and predictive genes for breast cancer. In addition, a lot of new prognostic genes are exploited for diagnosing and curing breast cancer, such as breast cancer 1/2, TP53, cyclin D1, cyclin E, cathepsin D, cystatin E/M, and plexin B1 (6–8). Many studies showed that using multigenes as a panel of biomarkers may work more accurately to predict clinical outcome (9). Therefore, multivariate cohorts are needed to identify novel genes, and these genes need to be exploited to cure and evaluate prognosis of breast cancer.
By combining clinical follow-up data and high-throughput profiling data, we have reached a better understanding in the study of breast carcinoma. In this study, we collected the gene expression profiling data with follow-up information of breast cancers, which were mainly from The Cancer Genome Atlas (TCGA) and Gene Expression Omnibus (GEO) database. Our aim is to provide a high powerful web server with massive data to generate survival plots to assess the relevance of the expression levels of interested genes on the clinical outcome for breast cancer patients. The Online consensus Survival analysis web server for Breast Cancers offers a web server to clinicians or non-bioinformatics researchers to appraise or exploit potential prognostic genes. Users can predict the prognostic potency of gene of interests using OSbrca.
Methods and Experiment
Data Collection
The gene expression profiling datasets for breast cancer were mainly composed of TCGA and GEO cohorts (Table 1) according to the following four criteria: (1) the cohort must have at least 50 breast cancer cases, (2) the cohort must contain individual clinical follow-up information, (3) the probe annotation should be completed or probe could be translated to gene symbol by ID conversion, such as DIVID, and (4) only platforms with more than 50 individual samples were selected if GEO cohorts having more than one platform.
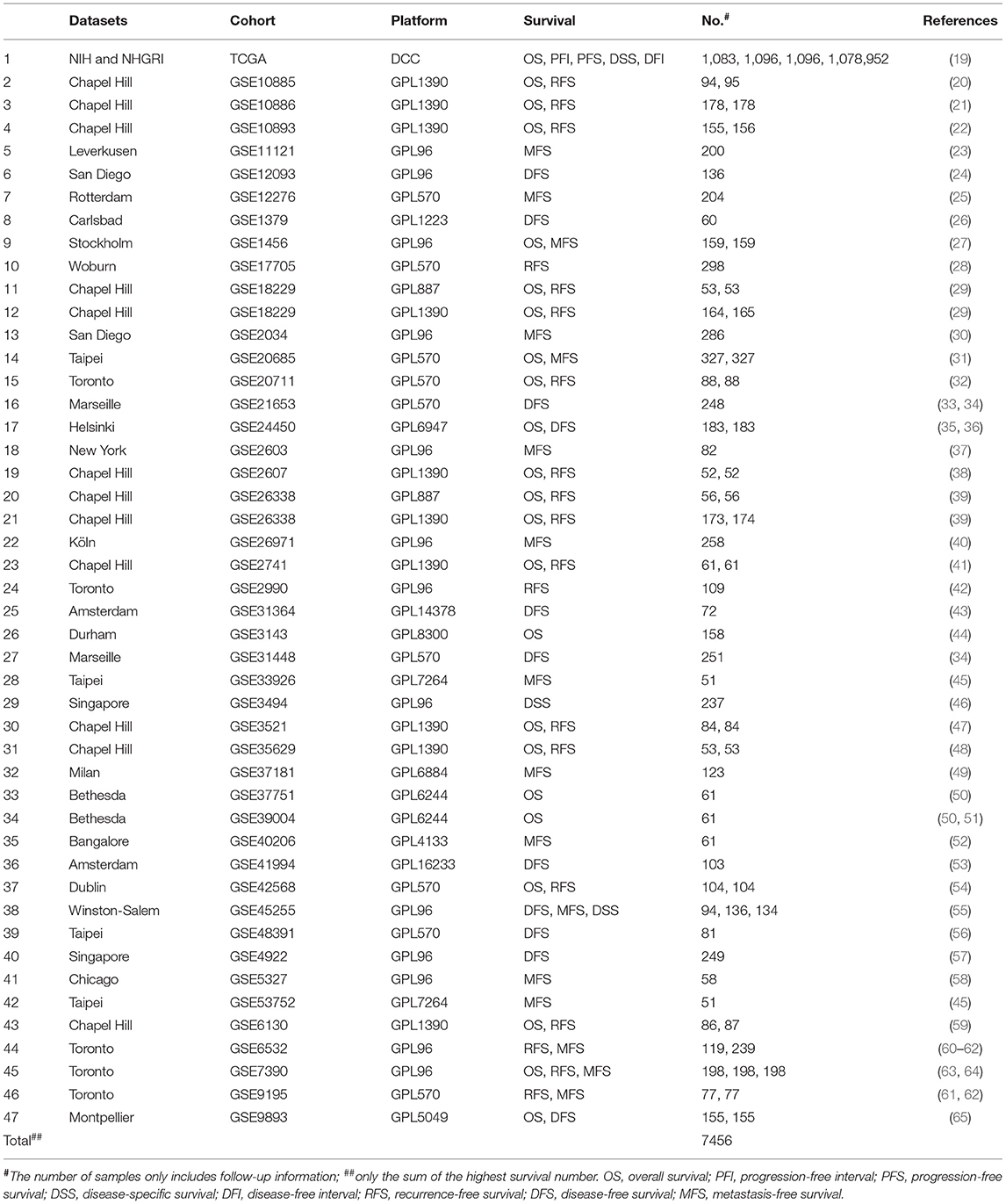
Table 1. The basic information of The Cancer Genome Atlas (TCGA) and Gene Expression Omnibus (GEO) of breast cancer cohorts in Online consensus Survival analysis web server for Breast Cancers (OSbrca).
Development of OSbrca
The OSbrca server is deployed in a tomcat server as previously described with minor modification (10). In brief, front-end application was exploited in HTML and JSP to retrieve user inputs and display the output on the web page. Java and R were also used in the server application to control the analysis request and return the results. The gene expression profiles and clinical data were stored and managed by the SQL Server database. The R and SQL Server were linked by third middleware (The R packages, “RODBC” and “JDBC”). The R package “survminer” and “survival” generate Kaplan–Meier (KM) survival curves with log-rank P-value and calculate the hazard ratio (HR) with 95% confidence intervals (95%CI). The KM survival curves measure the effect of genes on survival using breast cancer data (11). Log-rank test is the standard method of survival data comparison, which is widely used in survival analysis (12). HR and 95% confidence interval (95% CI) were calculated by univariate Cox regression analysis. OSbrca can be accessed in http://bioinfo.henu.edu.cn/BRCA/BRCAList.jsp.
Collection and Authenticating Previously Reported Prognostic Biomarkers of Breast Cancer
To collect previously published biomarkers of breast cancer in the PubMed, three key words were used: breast cancer, prognostic, and biomarker. One hundred and twenty-eight previously identified prognostic biomarkers are listed in Table S1. To examine the performance of OSbrca, each reported prognostic biomarker was analyzed in OSbrca, by categorizing patients with “upper 25%” (the upper 25% expression vs. the bottom 75% expression). In addition, OSbrca is a web server for cross-validation of the potential prognostic biomarkers among tens of breast cancer cohorts. As a result, the methodology of validation in OSbrca includes two parts. First, we performed the validation of prognostic biomarkers between different breast cancer cohorts, and this independent validation between cohorts is of great importance for biomarker development; second, validation of previously reported prognostic biomarkers in OSbrca presented the reliability of OSbrca.
Results
Collection of Gene Expression Profiles With Clinical Follow-Up Information of Breast Cancer
Breast cancer is the leading mortality in women and is one of the most widely studied cancers. Thus, the urge for breast cancer patient is to exploit novel therapy target and prognostic biomarkers, which would offer the opportunities to assist the clinical management and treatment. However, it is technically challenging for untrained researchers to process the high dimensional profiling data to screen and validate the potential prognostic values of genes of interests in multiple cohorts. To build OSbrca, we have collected more than 7,400 samples of breast cancer expression profiles with clinical follow-up information, mainly obtained from TCGA (1,092 samples) and GEO cohorts (6,364 samples) (Table 1). OSbrca includes overall survival (OS, 3,786 patients from 23 cohorts), progression-free interval (1,096 patients only from TCGA cohort), progression-free survival (1,096 patients only from TCGA cohort), disease-specific survival (1,499 patients from three cohorts), disease-free interval (952 patients only from TCGA cohort), recurrence-free survival (RFS, 2,207 patients from 19 cohorts), disease-free survival (DFS, 1,632 patients from 11 cohorts), and metastasis-free survival (MFS, 2,508 patients from 16 cohorts). In other words, the OSbrca can predict those eight survival endpoints basing on breast cancer clinical information, such as RFS.
The Architecture of the OSbrca Web Server for Breast Cancer
Based on the expression profiles and clinical outcome of breast cancers, OSbrca can determine the prognostic values of interested genes using KMPlot, HR, and log-rank P-value. OSbrca has implemented several optional clinical confounding factors, such as data source, age, stage, histological type, molecular subtype, survival, and ER/PgR/HER2 status. Users can select different cutoff, such as the upper 25%, for gene expression levels when categorizing the breast cancer population. The interface of the OSbrca is simple and friendly. Users could input the particular official gene symbol with all the default parameters and then click “Kaplan–Meier plot” button. The KMPlot with HR and log-rank P-value will be displayed on the output web page.
Evaluation of the Previously Reported Prognostic Biomarkers of Breast Cancer in OSbrca
We have designed OSbrca to be a user-friendly and easy-to-use online web server to analyze and evaluate the prognostic values of particular genes in 48 breast cancer cohorts using existing high-throughput profiling breast cancer data. To measure the performance and determine the reliability of OSbrca, we have collected previously published prognostic biomarkers of breast cancer (Table S1) and tested their prognostic potency in OSbrca. Fu et al. have demonstrated that PGK1 was overexpressed in tumor tissue and was an indication of worse survival biomarker in breast cancer (13). Using OSbrca, we showed that PGK1 gene was indeed a poor survival biomarker in breast cancer cohorts (top 6 samples): TCGA [OS, HR (95% CI) = 2.42 (1.74–3.36), P < 0.0001], GSE20685 [OS, HR (95% CI) = 2.11 (1.35–3.39), P = 0.001], GSE17705 [RFS, HR (95% CI) = 2.44 (1.51–3.95), P < 0.001], GSE2034 [MFS, HR (95% CI) = 1.60 (1.06–2.41), P = 0.0257], GSE269721 [MFS, HR (95% CI) = 1.83 (1.06–3.15), P = 0.0291], and GSE31448 [DFS, HR (95% CI) = 1.67 (1.03–2.69), P = 0.0364] (Figure 1). We also test another reported poor DFS biomarker RRM2. Figure 2 shows that RRM2 gene was an indication of worse survival indicator in five out of six breast cancer cohorts (top 6 samples), except in the cohort of GSE17705 (Figure 2). One hundred and twenty-eight previous reported prognostic biomarkers were validated in OSbrca shown in Table S1. Based on our studies using OSbrca, 62% analyzed biomarkers (79/128) showed consistent performance as reported in the literature, but some biomarkers showed contradictory outcomes to previous results. Taking the AOCA1 gene as an another example, a previous study showed that the AOCA1 gene could potentially predict a worse clinical prognosis in breast cancer (14). However, the analysis from OSbrca suggested that breast cancer patients with the overexpression of the AOCA1 gene would potentially have a better clinical outcome (Table S1). In summary, all the results showed that the OSbrca web server is very reliable through validating previously reported biomarkers of breast cancer.
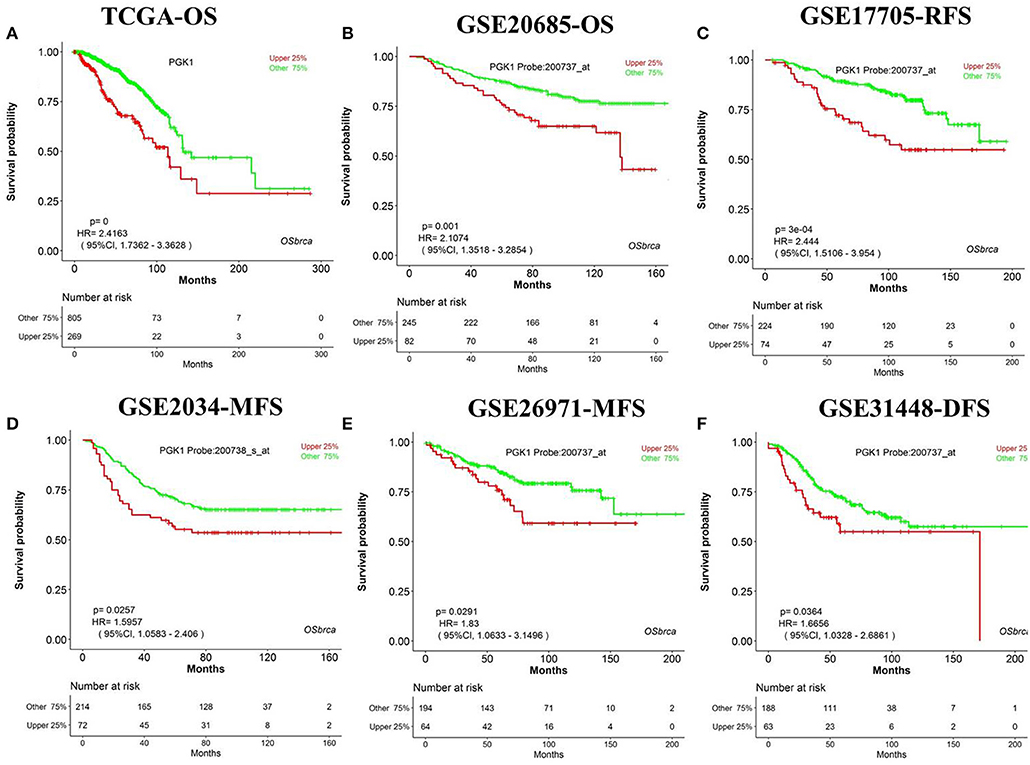
Figure 1. Validation previously reported gene PGK1 in Online consensus Survival analysis web server for Breast Cancers (OSbrca). PGK1 gene is high expressed in tumor tissue as a worse prognostic survival biomarker in breast cancer. (A) OS of TCGA, (B) OS of GSE20685, (C) RFS of GSE17705, (D) MFS of GSE2034, (E) MFS of GSE269721, (F) DFS of GSE31448. PGK1, phosphoglycerate kinase 1; OS, overall survival; DFS, disease-free survival; RFS, recurrence-free survival; MFS, metastasis-free survival.
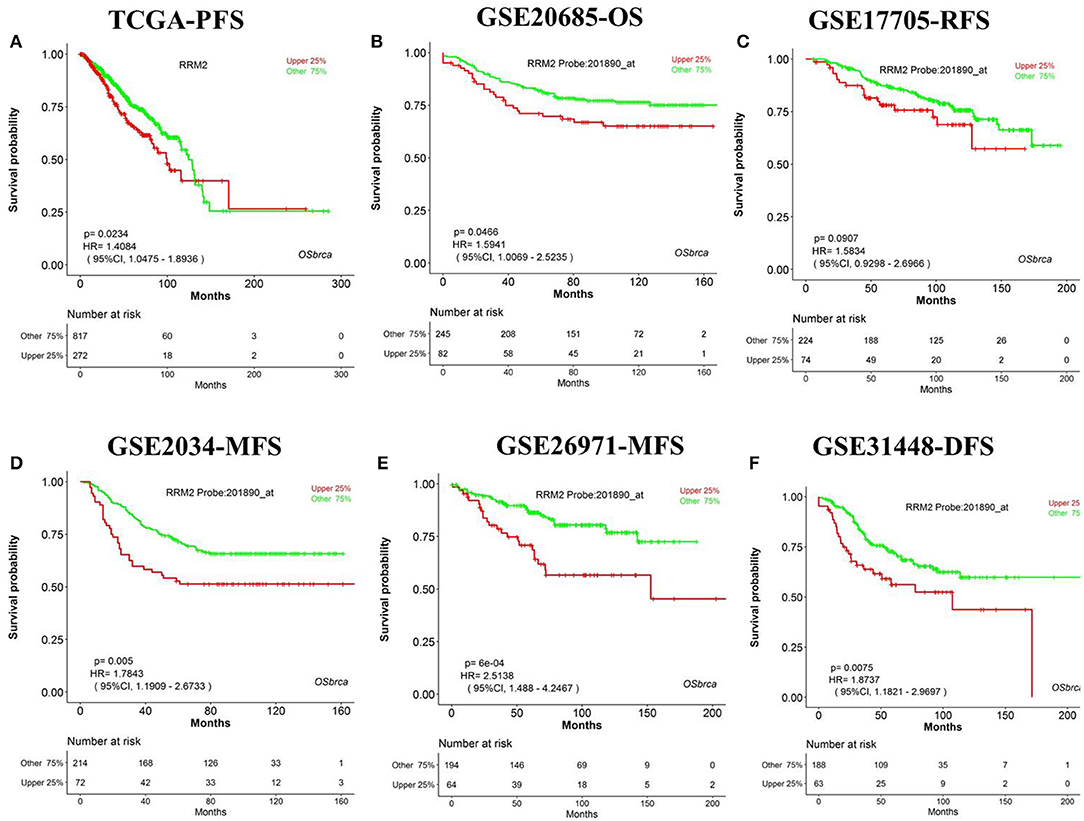
Figure 2. Validation previously reported gene RRM2 in Online consensus Survival analysis web server for Breast Cancers (OSbrca). RRM2 gene is a poor prognostic biomarker in breast cancer. (A) Progression-free survival (PFS) of The Cancer Genome Atlas (TCGA); (B) OS of GSE20685; (C) RFS of GSE17705; (D) MFS of GSE2034; (E) MFS of GSE269721; (F) DFS of GSE31448. RRM2, ribonucleotide reductase regulatory subunit M2; PFS, progression-free survival; OS, overall survival; RFS, recurrence-free survival; MFS, metastasis-free survival; DFS, disease-free survival.
Discussion
Breast cancer is widely profiled by RNA-sequences and gene microarrays, such as TCGA. Thus, the core and focus issue is how to excavate potential therapy targets and to develop prognostic biomarkers by possessing massive high-throughput profiles. Based on massive data of different cohorts, we integrated 48 cohorts of breast cancer datasets and established an online web server, named OSbrca. OSbrca implanted a selective set of clinical parameters, including tumor grade, age, status of ER/PgR/HER2, menopause status, and so on. The OSbrca could output the KMPlot with HR and log rank P-value for given genes in an interactive way. In addition, users can study genes in a particular country or race using OSbrca, such as Chinese breast cancer patients. Herein, we retrospectively validated the previously reported prognostic biomarkers of breast cancer. The results showed that most previous reported biomarkers could be identified by some different cohorts of OSbrca (Figures 1, 2, and Table S1). In addition, OSbrca is an across-validation web server used to exploit breast cancer biomarkers based on different independent cohorts of breast cancer. Cross-validation in OSbrca means that it is important to exploit prognostic biomarkers among tens of breast cancer cohorts and also presents the reliability of OSbrca.
So far, there are some online prognostic websites for breast cancer, such as KM plotter (11), PROGgene (15), ITTACA (16), PrognoScan (17), OncoLnc, and GEPIA (18), but the size of datasets used in these tools is relatively small and limited compared to OSbrca. Specifically, OSbrca integrates 48 cohorts that contain more than 7,400 patients with RNA-sequencing and gene microarray data. It allows researchers to revisit previous protein biomarkers and exploit novel prognostic biomarkers. There are some limitations of this study, such as the loss of different platform integration, lacking noncoding gene information, which will be solved in the new-version of this tool. In addition, when new cohorts become available, we will update OSbrca in a timely manner.
In conclusion, the OSbrca web server integrates more than 7,400 follow-up breast samples and is highly valuable for researchers with a limited bioinformatics background to access and uncover prognostic-related biomarkers for breast cancer.
Data Availability Statement
The data for this manuscript can be accessed at OSbrca http://bioinfo.henu.edu.cn/BRCA/BRCAList.jsp. The raw data supporting the conclusions of this manuscript will be made available by the authors, without undue reservation, to any qualified researcher.
Author Contributions
XG: research design. ZY, XS, LX, BB, ZL, YD, WZ, and LZ: collect and deal with data. ZY, LX, XS, YD, LZ, YL, and XG: draft of the manuscript. QW and XG: establish OSbrca Web Server. BB, XS, ZL, and YL: collect and validate previous reported biomarkers of breast cancer. ZY, QW, XS, LX, BB, YD, LZ, WZ, and XG: critical revision of the manuscript.
Funding
This study was supported by the following funding: Kaifeng Science and Technology Major Project (18ZD008), National Natural Science Foundation of China (Nos. 81602362 and 81801569), Program for Science and Technology Development in Henan Province (Nos. 162102310391, 172102210187, and 192102310302), Program for Innovative Talents of Science and Technology in Henan Province (No. 18HASTIT048), Supporting grants of Henan University (Nos. 2015YBZR048 and B2015151), and Yellow River Scholar Program (No. H2016012).
Conflict of Interest
The authors declare that the research was conducted in the absence of any commercial or financial relationships that could be construed as a potential conflict of interest.
Supplementary Material
The Supplementary Material for this article can be found online at: https://www.frontiersin.org/articles/10.3389/fonc.2019.01349/full#supplementary-material
References
1. Akram M, Iqbal M, Daniyal M, Khan AU. Awareness and current knowledge of breast cancer. Biol Res. (2017) 50:33. doi: 10.1186/s40659-017-0140-9
2. Ziegler RG, Anderson WF, Gail MH. Increasing breast cancer incidence in China: the numbers add up. J Natl Cancer Inst. (2008) 100:1339–41. doi: 10.1093/jnci/djn330
3. Tofigh A, Suderman M, Paquet ER, Livingstone J, Bertos N, Saleh SM, et al. The prognostic ease and difficulty of invasive breast carcinoma. Cell Rep. (2014) 9:129–42. doi: 10.1016/j.celrep.2014.08.073
4. Sana M, Malik HJ. Current and emerging breast cancer biomarkers. J Cancer Res Therapeutics. (2015) 11:508–13. doi: 10.4103/0973-1482.163698
5. Han SJ, Guo QQ, Wang T, Wang YX, Zhang YX, Liu F, et al. Prognostic significance of interactions between ER alpha and ER beta and lymph node status in breast cancer cases. Asian Pacific J Cancer Prev. (2013) 14:6081–4. doi: 10.7314/APJCP.2013.14.10.6081
6. Donepudi M, Kondapalli K, Amos S, Venkanteshan P. Breast cancer statistics and markers. J Cancer Res Therapeutics. (2014) 10:506–11. doi: 10.4103/0973-1482.137927
7. Liu J, Lichtenberg T, Hoadley KA, Poisson LM, Lazar AJ, Cherniack AD, et al. An integrated TCGA pan-cancer clinical data resource to drive high-quality survival outcome analytics. Cell. (2018) 173:400–16.e11. doi: 10.1158/1538-7445.AM2018-3287
8. Rody A, Holtrich U, Gaetje R, Gehrmann M, Engels K, von Minckwitz G, et al. Poor outcome in estrogen receptor–positive breast cancers predicted by loss of < em>Plexin B1 < /em>. Clin Cancer Res. (2007) 13:1115–22. doi: 10.1158/1078-0432.CCR-06-2433
9. Alexe G, Dalgin GS, Scanfeld D, Tamayo P, Mesirov JP, DeLisi C, et al. High expression of lymphocyte-associated genes in node-negative HER2+ breast cancers correlates with lower recurrence rates. Cancer Res. (2007) 67:10669–76. doi: 10.1158/0008-5472.CAN-07-0539
10. Wang Q, Xie L, Dang Y, Sun X, Xie T, Guo J, et al. OSlms: a web server to evaluate the prognostic value of genes in leiomyosarcoma. Front Oncol. (2019) 9:190. doi: 10.3389/fonc.2019.00190
11. Gyorffy B, Lanczky A, Eklund AC, Denkert C, Budczies J, Li Q, et al. An online survival analysis tool to rapidly assess the effect of 22,277 genes on breast cancer prognosis using microarray data of 1,809 patients. Breast Cancer Res Treat. (2010) 123:725–31. doi: 10.1007/s10549-009-0674-9
12. Peto R, Pike MC, Armitage P, Breslow NE, Cox DR, Howard SV, et al. Design and analysis of randomized clinical trials requiring prolonged observation of each patient. II. analysis and examples. Br J Cancer. (1977) 35:1–39. doi: 10.1038/bjc.1977.1
13. Fu D, He C, Wei J, Zhang Z, Luo Y, Tan H, et al. PGK1 is a potential survival biomarker and invasion promoter by regulating the HIF-1alpha-mediated epithelial-mesenchymal transition process in breast cancer. Cell Physiol Biochem. (2018) 51:2434–44. doi: 10.1159/000495900
14. Lai Y-CC, Chang S-J, Kostoro J, Kwan A-L, Chai C-Y. Vascular adhesion protein-1 as indicator of breast cancer tumor aggressiveness and invasiveness. APMIS. (2018) 126:755–61. doi: 10.1111/apm.12885
15. Goswami CP, Nakshatri H. PROGgene: gene expression based survival analysis web application for multiple cancers. J Clin Bioinformatics. (2013) 3:22. doi: 10.1186/2043-9113-3-22
16. Elfilali A, Lair S, Verbeke C, La RP, Radvanyi F, Barillot E. ITTACA: a new database for integrated tumor transcriptome array and clinical data analysis. Nucleic Acids Res. (2006) 34:D613–6. doi: 10.1093/nar/gkj022
17. Mizuno H, Kitada K, Nakai K, Sarai A. PrognoScan: a new database for meta-analysis of the prognostic value of genes. BMC Med Genomics. (2009) 2:18. doi: 10.1186/1755-8794-2-18
18. Tang Z, Li C, Kang B, Gao G, Li C, Zhang Z. GEPIA: a web server for cancer and normal gene expression profiling and interactive analyses. Nucleic Acids Res. (2017) 45:W98–102. doi: 10.1093/nar/gkx247
19. Weinstein JN, Collisson EA, Mills GB, Shaw KR, Ozenberger BA, Ellrott K, et al. The cancer genome atlas pan-cancer analysis project. Nat Genet. (2013) 45:1113–20. doi: 10.1038/ng.2764
20. Hennessy BT, Gonzalez-Angulo AM, Stemke-Hale K, Gilcrease MZ, Krishnamurthy S, Lee JS, et al. Characterization of a naturally occurring breast cancer subset enriched in epithelial-to-mesenchymal transition and stem cell characteristics. Cancer Res. (2009) 69:4116–24. doi: 10.1158/0008-5472.CAN-08-3441
21. Parker JS, Mullins M, Cheang MC, Leung S, Voduc D, Vickery T, et al. Supervised risk predictor of breast cancer based on intrinsic subtypes. J Clin Oncol. (2009) 27:1160–7. doi: 10.1200/JCO.2008.18.1370
22. Weigman VJ, Chao HH, Shabalin AA, He X, Parker JS, Nordgard SH, et al. Basal-like Breast cancer DNA copy number losses identify genes involved in genomic instability, response to therapy, and patient survival. Breast Cancer Res Treat. (2012) 133:865–80. doi: 10.1007/s10549-011-1846-y
23. Schmidt M, Bohm D, von Torne C, Steiner E, Puhl A, Pilch H, et al. The humoral immune system has a key prognostic impact in node-negative breast cancer. Cancer Res. (2008) 68:5405–13. doi: 10.1158/0008-5472.CAN-07-5206
24. Zhang Y, Sieuwerts AM, McGreevy M, Casey G, Cufer T, Paradiso A, et al. The 76-gene signature defines high-risk patients that benefit from adjuvant tamoxifen therapy. Breast Cancer Res Treat. (2009) 116:303–9. doi: 10.1007/s10549-008-0183-2
25. Bos PD, Zhang XH, Nadal C, Shu W, Gomis RR, Nguyen DX, et al. Genes that mediate breast cancer metastasis to the brain. Nature. (2009) 459:1005–9. doi: 10.1038/nature08021
26. Ma XJ, Wang Z, Ryan PD, Isakoff SJ, Barmettler A, Fuller A, et al. A two-gene expression ratio predicts clinical outcome in breast cancer patients treated with tamoxifen. Cancer Cell. (2004) 5:607–16. doi: 10.1016/j.ccr.2004.05.015
27. Pawitan Y, Bjohle J, Amler L, Borg AL, Egyhazi S, Hall P, et al. Gene expression profiling spares early breast cancer patients from adjuvant therapy: derived and validated in two population-based cohorts. Breast Cancer Res. (2005) 7:R953–64. doi: 10.1186/bcr1325
28. Symmans WF, Hatzis C, Sotiriou C, Andre F, Peintinger F, Regitnig P, et al. Genomic index of sensitivity to endocrine therapy for breast cancer. J Clin Oncol. (2010) 28:4111–9. doi: 10.1200/JCO.2010.28.4273
29. Prat A, Parker JS, Karginova O, Fan C, Livasy C, Herschkowitz JI, et al. Phenotypic and molecular characterization of the claudin-low intrinsic subtype of breast cancer. Breast Cancer Res. (2010) 12:R68. doi: 10.1186/bcr2635
30. Wang Y, Klijn JG, Zhang Y, Sieuwerts AM, Look MP, Yang F, et al. Gene-expression profiles to predict distant metastasis of lymph-node-negative primary Breast Cancer. Lancet. (2005) 365:671–9. doi: 10.1016/S0140-6736(05)17947-1
31. Kao KJ, Chang KM, Hsu HC, Huang AT. Correlation of microarray-based breast cancer molecular subtypes and clinical outcomes: implications for treatment optimization. BMC Cancer. (2011) 11:143. doi: 10.1186/1471-2407-11-143
32. Dedeurwaerder S, Desmedt C, Calonne E, Singhal SK, Haibe-Kains B, Defrance M, et al. DNA methylation profiling reveals a predominant immune component in breast cancers. EMBO Mol Med. (2011) 3:726–41. doi: 10.1002/emmm.201100801
33. Sabatier R, Finetti P, Cervera N, Lambaudie E, Esterni B, Mamessier E, et al. A gene expression signature identifies two prognostic subgroups of basal breast cancer. Breast Cancer Res Treat. (2011) 126:407–20. doi: 10.1007/s10549-010-0897-9
34. Sabatier R, Finetti P, Adelaide J, Guille A, Borg JP, Chaffanet M, et al. Down-regulation of ECRG4, a candidate tumor suppressor gene, in human breast cancer. PLoS ONE. (2011) 6:e27656. doi: 10.1371/journal.pone.0027656
35. Muranen TA, Greco D, Fagerholm R, Kilpivaara O, Kampjarvi K, Aittomaki K, et al. Breast tumors from CHEK2 1100delC-mutation carriers: genomic landscape and clinical implications. Breast Cancer Res. (2011) 13:R90. doi: 10.1186/bcr3015
36. Heikkinen T, Greco D, Pelttari LM, Tommiska J, Vahteristo P, Heikkila P, et al. Variants on the promoter region of PTEN affect breast cancer progression and patient survival. Breast Cancer Res. (2011) 13:R130. doi: 10.1186/bcr3076
37. Minn AJ, Gupta GP, Siegel PM, Bos PD, Shu W, Giri DD, et al. Genes that mediate breast cancer metastasis to lung. Nature. (2005) 436:518–24. doi: 10.1038/nature03799
38. Perreard L, Fan C, Quackenbush JF, Mullins M, Gauthier NP, Nelson E, et al. Classification and risk stratification of invasive breast carcinomas using a real-time quantitative RT-PCR assay. Breast Cancer Res. (2006) 8:R23. doi: 10.1186/bcr1399
39. Harrell JC, Prat A, Parker JS, Fan C, He X, Carey L, et al. Genomic analysis identifies unique signatures predictive of brain, lung, and liver relapse. Breast Cancer Res Treat. (2012) 132:523–35. doi: 10.1007/s10549-011-1619-7
40. Filipits M, Rudas M, Jakesz R, Dubsky P, Fitzal F, Singer CF, et al. A new molecular predictor of distant recurrence in ER-positive, HER2-negative breast cancer adds independent information to conventional clinical risk factors. Clin Cancer Res. (2011) 17:6012–20. doi: 10.1158/1078-0432.CCR-11-0926
41. Weigelt B, Hu Z, He X, Livasy C, Carey LA, Ewend MG, et al. Molecular portraits and 70-gene prognosis signature are preserved throughout the metastatic process of breast cancer. Cancer Res. (2005) 65:9155–8. doi: 10.1158/0008-5472.CAN-05-2553
42. Sotiriou C, Wirapati P, Loi S, Harris A, Fox S, Smeds J, et al. Gene expression profiling in breast cancer: understanding the molecular basis of histologic grade to improve prognosis. J Natl Cancer Inst. (2006) 98:262–72. doi: 10.1093/jnci/djj052
43. Molloy TJ, Roepman P, Naume B, van't Veer LJ. A prognostic gene expression profile that predicts circulating tumor cell presence in breast cancer patients. PLoS ONE. (2012) 7:e32426. doi: 10.1371/journal.pone.0032426
44. Bild AH, Yao G, Chang JT, Wang Q, Potti A, Chasse D, et al. Oncogenic pathway signatures in human cancers as a guide to targeted therapies. Nature. (2006) 439:353–7. doi: 10.1038/nature04296
45. Kuo WH, Chang YY, Lai LC, Tsai MH, Hsiao CK, Chang KJ, et al. Molecular characteristics and metastasis predictor genes of triple-negative breast cancer: a clinical study of triple-negative breast carcinomas. PLoS ONE. (2012) 7:e45831. doi: 10.1371/journal.pone.0045831
46. Miller LD, Smeds J, George J, Vega VB, Vergara L, Ploner A, et al. An expression signature for p53 status in human breast cancer predicts mutation status, transcriptional effects, and patient survival. Proc Natl Acad Sci USA. (2005) 102:13550–5. doi: 10.1073/pnas.0506230102
47. Hu Z, Fan C, Livasy C, He X, Oh DS, Ewend MG, et al. A compact VEGF signature associated with distant metastases and poor outcomes. BMC Med. (2009) 7:9. doi: 10.1186/1741-7015-7-9
48. Ulirsch J, Fan C, Knafl G, Wu MJ, Coleman B, Perou CM, et al. Vimentin DNA methylation predicts survival in breast cancer. Breast Cancer Res Treat. (2013) 137:383–96. doi: 10.1007/s10549-012-2353-5
49. Callari M, Musella V, Di Buduo E, Sensi M, Miodini P, Dugo M, et al. Subtype-dependent prognostic relevance of an interferon-induced pathway metagene in node-negative. Breast Cancer Mol Oncol. (2014) 8:1278–89. doi: 10.1016/j.molonc.2014.04.010
50. Terunuma A, Putluri N, Mishra P, Mathe EA, Dorsey TH, Yi M, et al. MYC-driven accumulation of 2-hydroxyglutarate is associated with breast cancer prognosis. J Clin Investig. (2014) 124:398–412. doi: 10.1172/JCI71180
51. Tang W, Zhou M, Dorsey TH, Prieto DA, Wang XW, Ruppin E, et al. Integrated proteotranscriptomics of breast cancer reveals globally increased protein-mRNA concordance associated with subtypes and survival. Genome Med. (2018) 10:94. doi: 10.1186/s13073-018-0602-x
52. Bashir M, Damineni S, Mukherjee G, Kondaiah P. Activin-A signaling promotes epithelial–mesenchymal transition, invasion, and metastatic growth of breast cancer. NPJ Breast Cancer. (2015) 1:15007. doi: 10.1038/npjbcancer.2015.7
53. Jansen MP, Knijnenburg T, Reijm EA, Simon I, Kerkhoven R, Droog M, et al. Hallmarks of aromatase inhibitor drug resistance revealed by epigenetic profiling in breast cancer. Cancer Res. (2013) 73:6632–41. doi: 10.1158/0008-5472.CAN-13-0704
54. Clarke C, Madden SF, Doolan P, Aherne ST, Joyce H, O'Driscoll L, et al. Correlating transcriptional networks to breast cancer survival: a large-scale coexpression analysis. Carcinogenesis. (2013) 34:2300–8. doi: 10.1093/carcin/bgt208
55. Nagalla S, Chou JW, Willingham MC, Ruiz J, Vaughn JP, Dubey P, et al. Interactions between immunity, proliferation and molecular subtype in breast cancer prognosis. Genome Biol. (2013) 14:R34. doi: 10.1186/gb-2013-14-4-r34
56. Huang CC, Tu SH, Lien HH, Jeng JY, Huang CS, Huang CJ, et al. Concurrent gene signatures for han chinese breast cancers. PLoS ONE. (2013) 8:e76421. doi: 10.1371/journal.pone.0076421
57. Ivshina AV, George J, Senko O, Mow B, Putti TC, Smeds J, et al. Genetic reclassification of histologic grade delineates new clinical subtypes of breast cancer. Cancer Res. (2006) 66:10292–301. doi: 10.1158/0008-5472.CAN-05-4414
58. Minn AJ, Gupta GP, Padua D, Bos P, Nguyen DX, Nuyten D, et al. Lung metastasis genes couple breast tumor size and metastatic spread. Proc Natl Acad Sci USA. (2007) 104:6740–5. doi: 10.1073/pnas.0701138104
59. Mullins M, Perreard L, Quackenbush JF, Gauthier N, Bayer S, Ellis M, et al. Agreement in breast cancer classification between microarray and quantitative reverse transcription PCR from fresh-frozen and formalin-fixed, paraffin-embedded tissues. Clin Chem. (2007) 53:1273–9. doi: 10.1373/clinchem.2006.083725
60. Loi S, Haibe-Kains B, Desmedt C, Lallemand F, Tutt AM, Gillet C, et al. Definition of clinically distinct molecular subtypes in estrogen receptor-positive breast carcinomas through genomic grade. J Clin Oncol. (2007) 25:1239–46. doi: 10.1200/JCO.2006.07.1522
61. Loi S, Haibe-Kains B, Desmedt C, Wirapati P, Lallemand F, Tutt AM, et al. Predicting prognosis using molecular profiling in estrogen receptor-positive breast cancer treated with tamoxifen. BMC Genomics. (2008) 9:239. doi: 10.1186/1471-2164-9-239
62. Loi S, Haibe-Kains B, Majjaj S, Lallemand F, Durbecq V, Larsimont D, et al. PIK3CA mutations associated with gene signature of low mTORC1 signaling and better outcomes in estrogen receptor-positive breast cancer. Proc Natl Acad Sci USA. (2010) 107:10208–13. doi: 10.1073/pnas.0907011107
63. Desmedt C, Piette F, Loi S, Wang Y, Lallemand F, Haibe-Kains B, et al. Strong time dependence of the 76-gene prognostic signature for node-negative breast cancer patients in the TRANSBIG multicenter independent validation series. Clin Cancer Res. (2007) 13:3207–14. doi: 10.1158/1078-0432.CCR-06-2765
64. Patil P, Bachant-Winner PO, Haibe-Kains B, Leek JT. Test set bias affects reproducibility of gene signatures. Bioinformatics. (2015) 31:2318–23. doi: 10.1093/bioinformatics/btv157
Keywords: survival, breast cancer, prognosis, biomarker, OSbrca
Citation: Yan Z, Wang Q, Sun X, Ban B, Lu Z, Dang Y, Xie L, Zhang L, Li Y, Zhu W and Guo X (2019) OSbrca: A Web Server for Breast Cancer Prognostic Biomarker Investigation With Massive Data From Tens of Cohorts. Front. Oncol. 9:1349. doi: 10.3389/fonc.2019.01349
Received: 27 April 2019; Accepted: 15 November 2019;
Published: 20 December 2019.
Edited by:
Aleix Prat, Hospital Clínic de Barcelona, SpainReviewed by:
Yoichi Naito, National Cancer Center Hospital East, JapanSteven Narod, University of Toronto, Canada
Copyright © 2019 Yan, Wang, Sun, Ban, Lu, Dang, Xie, Zhang, Li, Zhu and Guo. This is an open-access article distributed under the terms of the Creative Commons Attribution License (CC BY). The use, distribution or reproduction in other forums is permitted, provided the original author(s) and the copyright owner(s) are credited and that the original publication in this journal is cited, in accordance with accepted academic practice. No use, distribution or reproduction is permitted which does not comply with these terms.
*Correspondence: Xiangqian Guo, xqguo@henu.edu.cn
†These authors have contributed equally to this work