- 1MedFuture - Research Center for Advanced Medicine, Iuliu Hatieganu University of Medicine and Pharmacy, Cluj-Napoca, Romania
- 2Department of Hematology, Research Center for Functional Genomics and Translational Medicine, Iuliu Hatieganu University of Medicine and Pharmacy, Cluj-Napoca, Romania
- 3Department of Hematology, Ion Chiricuta Clinical Cancer Center, Cluj-Napoca, Romania
- 4Department of Experimental Therapeutics, The University of Texas MD Anderson Cancer Center, Houston, TX, United States
- 5Center for RNA Interference and Non-Coding RNAs, The University of Texas MD Anderson Cancer Center, Houston, TX, United States
- 6MedFuture - Research Center for Advanced Medicine, Research Center for Functional Genomics and Translational Medicine, Iuliu Hatieganu University of Medicine and Pharmacy, Cluj-Napoca, Romania
- 7Department of Functional Genomics and Experimental Pathology, Ion Chiricuta Clinical Cancer Center, Cluj-Napoca, Romania
Acute myeloid leukemia (AML) represents 80% of adult leukemias and 15–20% of childhood leukemias. AML are characterized by the presence of 20% blasts or more in the bone marrow, or defining cytogenetic abnormalities. Laboratory diagnoses of myelodysplastic syndromes (MDS) depend on morphological changes based on dysplasia in peripheral blood and bone marrow, including peripheral blood smears, bone marrow aspirate smears, and bone marrow biopsies. As leukemic cells are not functional, the patient develops anemia, neutropenia, and thrombocytopenia, leading to fatigue, recurrent infections, and hemorrhage. The genetic background and associated mutations in AML blasts determine the clinical course of the disease. Over the last decade, non-coding RNAs transcripts that do not codify for proteins but play a role in regulation of functions have been shown to have multiple applications in the diagnosis, prognosis and therapeutic approach of various types of cancers, including myeloid malignancies. After a comprehensive review of current literature, we found reports of multiple long non-coding RNAs (lncRNAs) that can differentiate between AML types and how their exogenous modulation can dramatically change the behavior of AML cells. These lncRNAs include: H19, LINC00877, RP11-84C10, CRINDE, RP11848P1.3, ZNF667-AS1, AC111000.4-202, SFMBT2, LINC02082-201, MEG3, AC009495.2, PVT1, HOTTIP, SNHG5, and CCAT1. In addition, by performing an analysis on available AML data in The Cancer Genome Atlas (TCGA), we found 10 lncRNAs with significantly differential expression between patients in favorable, intermediate/normal, or poor cytogenetic risk categories. These are: DANCR, PRDM16-DT, SNHG6, OIP5-AS1, SNHG16, JPX, FTX, KCNQ1OT1, TP73-AS1, and GAS5. The identification of a molecular signature based on lncRNAs has the potential for have deep clinical significance, as it could potentially help better define the evolution from low-grade MDS to high-grade MDS to AML, changing the course of therapy. This would allow clinicians to provide a more personalized, patient-tailored therapeutic approach, moving from transfusion-based therapy, as is the case for low-grade MDS, to the introduction of azacytidine-based chemotherapy or allogeneic stem cell transplantation, which is the current treatment for high-grade MDS.
Background on Myeloid Malignancies
Hematopoiesis is the complex process of unidirectional and continuous formation and release of blood cells into circulation. Normal hematopoiesis is polyclonal and takes place in the hematogenous bone marrow (BM) (1–3). Pluripotent stem cells have the capacity to differentiate into multiple cell lineages (4–7), and this differentiation is irreversible during normal human physiology. The production of hematogenous BM is impressive, forming about 1010 erythrocytes and 108-109 leukocytes every hour, regulated by numerous cytokines for the maintenance of lineages under normal parameters (8–10). When in need, production can increase up to seven times normal levels. Pluripotent stem cells differentiate into myeloid and lymphoid multipotent stem cells that further differentiate into cells oriented on a lineage, called colony forming units (CFU). This name depicts their capacity to produce colonies in vitro in the presence of growth factors, also called progenitors (11, 12).
All cells of the BM, including progenitors, precursors, mature cells and stromal cells, interact in a complex manner through cytokines, messenger RNAs (mRNAs), microRNAs, and other non-coding RNAs (ncRNAs) (13–16), by targeting genes responsible for cell proliferation, differentiation, methylation, and acquisition of resistance to therapy. Granulopoiesis is the process that gives rise to elements of the granulocyte series: segmented neutrophils, eosinophils, and basophils. Acute myeloid leukemia (AML) develops in different stages of granulopoiesis, each with a specific set of long non-coding RNAs (lncRNAs) (Figure 1). The progenitors of this series are the myeloid stem cell and the granulo-monocytic colony forming unit, which are not morphologically identifiable (17, 18).
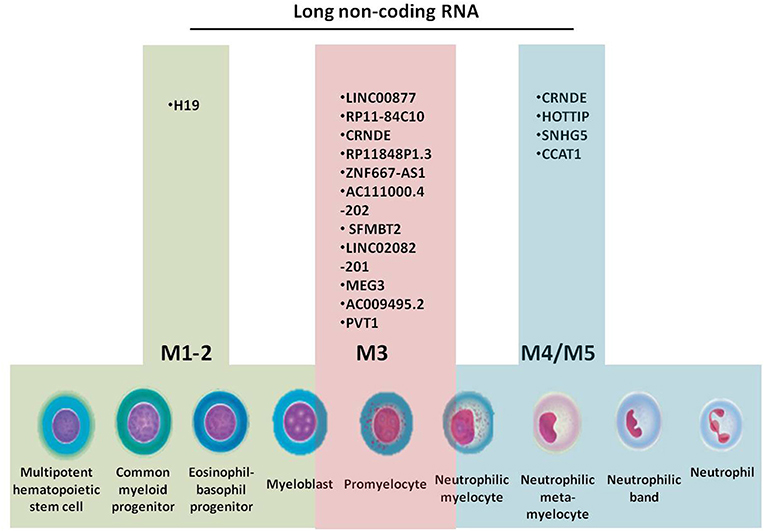
Figure 1. Granulopoiesis, the generation of myeloid cells, French-American-British (FAB) classification of AML and the specific long non-coding RNAs for each AML subtype.
Non-coding RNAs are small RNA molecules that are not translated into a protein (19), and have the potential of bringing new insights in the clinic for diagnosis, prognosis and therapy. DNA is a molecule composed of around 3 billion base pairs, out of which only 5–10% are transcribed, with protein-coding genes accounting for <2% of the human genome (20–22). Between 60% and 90% of the human genome is transcribed in non-coding transcripts, these transcripts being further divided into two categories depending on their primary role: either housekeeping or regulatory genes (23). Regulatory genes include microRNAs (miRNAs), circular RNAs and lncRNAs (24, 25) involved in regulation of gene transcription and translation, along with key cellular mechanisms such as differentiation, proliferation, or inflammation. In this manuscript, we aim to provide novel comprehensive insights on the role of lncRNAs in the pathogenesis of myeloid malignancies, illustrating their diagnostic and prognostic potential.
Myeloid precursors are formed by at least four generations of cells between granulo-monocytic CFUs and the mature elements of the series. Young precursors have azurophil granulations, also called primary granulations. Lineage-specific granulations (neutrophil, eosinophil, basophile) appear at the myelocyte stage. All lineages have the same maturation steps, with the only difference occurring in lineage specific granulations (26–29).
In normal conditions, the BM contains <5% blasts. When a mutation appears in a myeloid progenitor of the BM, the result is the development of a myelodysplastic syndrome (<20% blasts) or an acute leukemia (more than 20% BM blasts). Myelodysplastic syndromes (MDS) are clonal disorders of hematopoietic stem cells, characterized by cytopenia in the context of hyper or normocellular bone marrow, multilineage dysplasia, and frequent chromosomal abnormalities (30–33). The World Health Organization (WHO) classification based on morphology, cytochemistry, immunophenotype, cytogenetics, and clinical examination is described in Table 1 and Figure 2 (34–36).
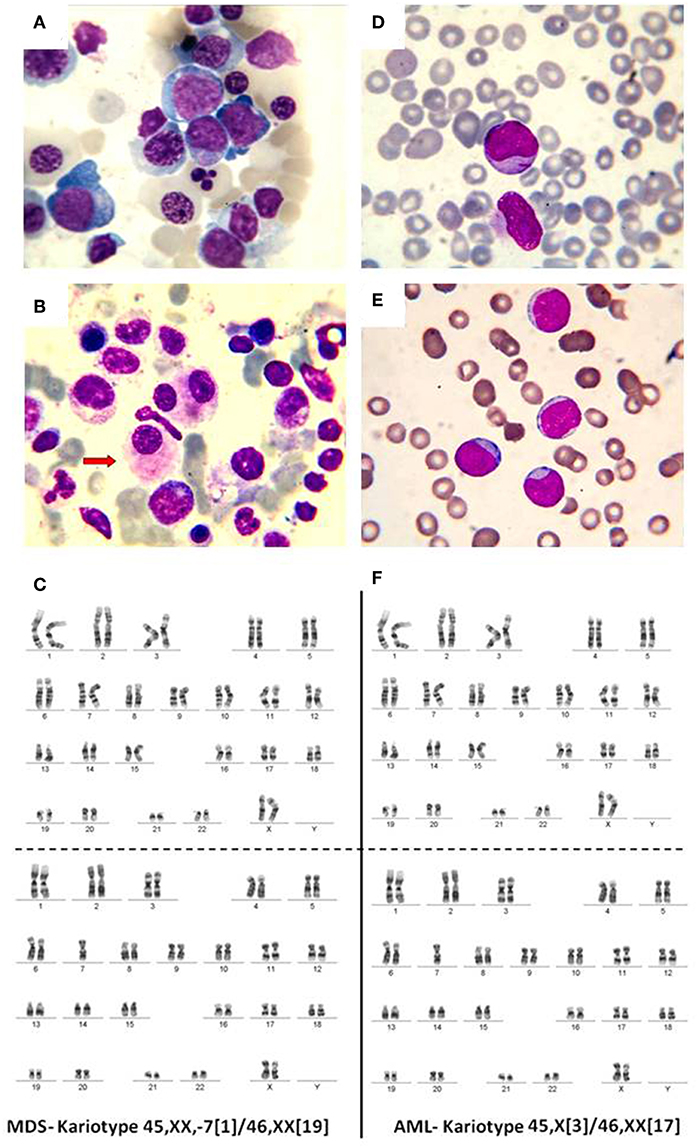
Figure 2. (A) Bone marrow, May-Grunwald-Giemsa stain, 1,000x. Myelodysplastic syndrome. Hyperplasic erythroid lineage, megaloblasts, oxyphilic megaloblast with sprouted nuclei, (B) myelodysplastic syndrome, dysmegakaryocytopoiesis. (C) MDS karyotype classified as intermediate risk. (D) Myeloblasts. Acute myeloid leukemia, (E) weakly differentiated myeloblasts. Acute myeloid leukemia, (F) AML karyotype classified as intermediate risk.
Cytogenetics in the Clinic for Myeloid Malignancies
Recurrent cytogenetic abnormalities are considered diagnostic for myelodysplastic syndrome, in the presence of cytopenia of unknown etiology and in the absence of myelodysplastic changes. Morphologic changes that are characteristic for myelodysplastic syndrome are described in Table 2 (37–39).
Depending on genetic background, MDS can either respond to standard therapies, or can evolve into an acute leukemia (40–43). Acute leukemias are caused by mutations in the unipotent or pluripotent stem cells from the bone marrow that yield an overproduction of immature cells and inhibition of normal hematopoiesis, resulting in anemia, thrombocytopenia, and neutropenia (42, 44–47). The type of malignant cell is defined according to the lineage, whether it is myeloid, lymphoid or mixed. Acute leukemias also occur when a hematopoietic stem cell acquires a sequence of mutations that confer an advantage of clonal proliferation (43, 48–52). The incidence of AML increases with age and represents 80% of adult leukemia (53) and 15–20% of childhood acute leukemiac (54). Congenital leukemia is a rare disease that is acquired in the neonatal period (45). For AML, the symptomatology results from bone marrow infiltration by the malignant cell, which inhibits normal hematopoiesis. Leukemic cells are not functional; thus, the patient develops anemia, neutropenia, and thrombocytopenia, leading to fatigue, recurrent infections, and hemorrhage.
A common characteristic of acute leukemia is the hypercellularity of the bone marrow, with more than 20% blasts at the time of diagnosis. Blast subtype represents the principal diagnostic criteria between acute lymphoblastic leukemia and AML. WHO classification of AML divides them into four principal subtypes: AML with recurrent cytogenetic abnormalities, AML with dysplasia, AML associated with therapy and myelodysplastic syndrome, and the “not otherwise specified” AML (34).
Cytogenetics and molecular biology are of key importance in myeloid malignancies, as previously shown by several groups including ours (55–58). In AML with recurrent cytogenetic abnormalities, these very recurrent cytogenetics represent the most important trait, as their names clearly describes them (55–57). The best prognostics are associated with t(8;21) (q22;q22) and inv(16)(p13;q22), where a high percentage of patients achieve complete remission (58, 59). Patients with AML are routinely profiled for the presence of mutations in FLT3, NPM1, CEBPA, and, more recently, TP53 (59–61). According to the German-Austrian Acute Myeloid Leukemia Study Group, coordinated by Dohner et al., the genotype of mutant NPM1 without FLT3-ITD, the mutant CEBPA genotype, and younger age were each significantly associated with complete remission. The benefit of the transplant was limited to the subgroup of patients with the genotype FLT3-ITD or the genotype consisting of wild-type NPM1 and CEBPA without FLT3-ITD.
AML with dysplasia is associated with at least two dysplastic lineages and the leukemic clone develops following a previous MDS clone. Dyserythropoiesis is described as multinucleated erythroblasts, magaloblastosis, cytoplasmic vacuoles or karyorrhexis (62). The leukemic clone develops as an evolutionary hallmark of MDS and patients generally present with a reduction in the number of erythrocytes, neutrophils and thrombocytes (63, 64). Because of frequent pancytopenia, the prognosis is generally bad. Several predictors for transformation have been identified and include mutations of genes in growth signaling pathways (NRAS, KRAS, PTPN11, FLT3), mutations in genes more commonly observed in AML (NPM1, WT1, IDH2), and certain cytogenetic abnormalities (monosomy 7, complex karyotype, loss of 17p). Gene expression profiles that divide MDS into two major categories identify a progenitor gene signature subtype associated with a high risk of AML transformation (65).
Therapy-related MDS (t-MDS) and therapy-related AML (t-AML) are considered one entity in therapy-related myeloid malignancies because of their similar pathogenesis, rapid progression from t-MDS to t-AML, and their equally poor prognosis. A small percentage of patients present with favorable risk fusion genes, whereas 50% have adverse cytogenetics. The most frequent molecular aberration in t-AML and t-MDS affects TP53 (33%). The selection of pre-existing treatment-resistant hematopoietic stem cell clones with the TP53 mutation has been shown as an important mechanism in the development of t-MNs and explains the high frequency of TP53 mutations in these patients (66, 67).
Current Research on Long Non-Coding RNAs in Myeloid Malignancies
As previously mentioned, ncRNAs are transcripts that do not possess protein-coding activity, but regulate cell behavior through interactions established at the DNA, RNA, or protein levels. Due to their versatility, the ncRNAs are often proposed as diagnostic and prognostic tools (68, 69) or as part of combined therapy in cancer (70–72). The role of ncRNAs has been extensively analyzed during the past decade, with the focus being on miRNAs (73–77). LncRNAs are a class of transcripts with sequence lengths of more than 200 nucleotides (78, 79). They are involved in various molecular processes, such as chromatin interaction (80), modulating gene transcription through binding the promoter region (81), acting as a competing endogenous RNAs (ceRNAs) for microRNAs (82), interacting with the ribosome and thus interfering in translation as well as interacting with various proteins (83, 84) and determining cellular localization (84). Non-coding transcription across antisense strands of genes is a universal mechanism for both yeast and vertebrates. The antisense transcription stimulates nucleosome occupancy and acetylation of histone proteins, but does not always alter the transcription of protein-coding genes. Antisense transcription has a high level of histone turnover and makes genes more susceptible to changing signals due to a wide range of chromatic configuration. This is specific to eukaryotic cells and allows these cells to adapt to external environmental stress (85–87). Due to this supportive evidence, the role of lncRNAs in hematological malignancies is being investigated and numerous studies offer compelling evidence for the correlation between altered lncRNA expression and various clinico-pathological characteristics of leukemia patients.
SBF2-AS1, DANCR, LINC00239, LINC00319, LINC00265, LEF1-AS1, and ZFAS1 are lncRNAs found to be overexpressed in AML (88–94). In vitro, the inhibition of SBF2-AS1 lncRNA causes leukemia cells to undergo apoptosis and cell cycle arrest. This lncRNA sponges the tumor suppressor miR-188-5p. The lncRNA DANCR is downregulated in undifferentiated hematopoietic progenitors, both malignant and BM-derived normal stem cells (89, 95, 96), the CD34-positive leukemic stem cells, as well as in normal BM-derived. However, for normal stem cells, siRNA-mediated knock out of DANCR does not affect normal hematopoiesis. In AML cells, DANCR silencing leads to impaired AML progression through inhibited self-renewal capacity and dormancy of leukemia cells. In vivo experiments also showed that the siRNA treatment leads to increased overall survival. This lncRNA acts by activating the c-Myc transcription factor through the WNT signaling pathway (89).
Linc00239 regulates chemoresistance to doxorubicin and exerts a protective effect against apoptotic cell death, promotes cell viability, cell cycle distribution, colony formation and migration. This lncRNA leads to mTOR/AKT activation (90). The overactivation of SOX61 in AML leads to increased cell proliferation and inhibited cell apoptosis in KG-1 cells and THP-1 cell (97). LINC00265 causes G0/G1 cell cycle arrest, decreased proliferative rates, apoptosis, and reduction of migratory capabilities and is involved in the phosphorylation of PI3K/AKT (92).
Some lncRNAs act as tumor suppressors in AML. H22954 is a novel lncRNA, downregulated in the bone marrow of AML patients. Its decreased expression is linked to a higher risk of relapse. H22954 expression inhibits AML cell proliferation in vitro and its overexpression leads to cell apoptosis. In vivo, it inhibits tumor growth in a mouse xenograft model as it interacts with the 3′UTR BCL2, a well-known antiapoptotic oncoprotein (98).
In AML with a monocytic/monoblastic phenotype, HOTTIP and LINC00152 are overexpressed specifically in the bone marrow microenvironment of patients with AML FAB classification M5. In vitro experiments have proven that the inhibition of LINC00152 leads to impaired proliferation of leukemia cells and cell cycle arrest (99, 100). LINC00152 decreases the self-renewal capacity of leukemia cells (99), whereas HOTTIP acts by sponging the tumor suppressor miR-608 and the consequent overstimulation of the oncogene DDA1 (DET1- and DDB1-Associated Protein 1) (100). LINC00152 has a similar molecular activity, by sponging miR-193a and indirectly increasing the expression of Cyclin Dependent Kinase 9 (CDK9). A subtype of AML is acute promyelocytic leukemia (APL), the former FAB M3 AML, with a particular genetic background and therapy protocol, based on the use of retinoic acid (101–103). In APL, the overexpression of ENST00000484765, ENST00000509010, and ENST00000416842 translates into a negative overall survival with a higher percentage of cancer stem cells present in the bone marrow, whereas the overexpression of ENST00000505646, lnc-SFMBT2-4 is translated into a better survival rate for the patients (104). Therefore, the expression analysis of these lncRNAs could be incorporated into current clinical practice (104).
TUG1 (105, 106), SNHG5 (107), LINC00926, LRRC75A-AS1, FAM30A (108), IRAIN (109), ENSG00000260257, and ENSG00000236537 (110) can stratify AML patients into those with higher survival rates or lower survival rates, according to their expression level.
UCA1 is upregulated in the AML cell line HL-60, and its inhibition decreases cell viability, migration and invasion capacity (111), while surpassing chemoresistance (112). This lncRNA targets two tumor suppressor microRNAs, namely miR-126 in AML (111) and miR-125A in de novo AML (112).
H19 is a potential biomarker of AML response to therapy, being downregulated in CR. It has the ID2 gene as a potential downstream target. This lncRNA impairs apoptosis and promotes cell proliferation in AML (113). MALAT1 is a well-known lncRNA with oncogenic potential in solid cancers. In AML, a number of studies have reaffirmed its oncogenic role. MALAT1 correlated with the expression of NTRK3 gene can differentiate between patients carrying various mutations. For instance, patients with t(15;17) and the PML-RARA fusion gene have increased co-expression of MALAT1 and NTRK3; patients with t(8;21) exhibit an overexpression of MALAT1 and underexpression of NTRK3. As follows, in the clinical setting AML patients carrying the ETV6-NTRK3 gene fusion and associated MALAT1 overexpression can exhibit a better therapeutic response to NTRK3 inhibitor, AZ-23 (114).
HOTAIR is another oncogenic lncRNA with well-established roles in various tumors. The expression of HOTAIR is upregulated in AML cell lines (HL-60, K562) (115, 116) and in peripheral or bone marrow mononuclear cells from AML patients. It stimulates AML progression, by promoting a higher number of blast and stimulated cell proliferation (115–117).
ZEAS1 is upregulated in AML cell lines, and its knockdown lowers cell proliferation, induces apoptosis and cell cycle arrest in the G0/S phase (118). CCDC26 is upregulated in AML cell line K562, where it stimulates cell division by impairing the translation of KIT proteins (119). Further details on the role of lncRNAs in leukemia are found in Table 3.
The search for lncRNAs biomarkers for AML can yield negative results. For instance, Sayad et al. have tried to analyze the expression level of FAS antisense RNA 1 (FAS-AS1) in the peripheral blood of AML patients vs. healthy controls. The study found no significant change in expression between the two groups, even though FAS has been found to play key roles in cellular apoptosis (120). In a study involving an Iranian population, it was proven that HOTAIR is not differentially expressed in the peripheral blood from healthy vs. AML patients (121). Several lncRNAs were reported to have both oncogenic and tumor suppressor roles in AML. On the one hand, MEG3 is upregulated in APL (122), whereas in AML cell lines, it was found to function as a tumor suppressor (123). On the other hand, TCGA analysis revealed that CRINDE is downregulated in APL (122) and upregulated in bone marrow stem cells (124), bone marrow samples and AML cell lines (125). This could be attributed to the fact that the AML cell lines were harvested from patients with other AML subtypes or due to differences in gene expression analysis.
Long Non-Coding RNAs in Myeloid Malignancies—Analysis of TCGA Data
In the present manuscript we also present a TCGA data analysis on myeloid malignancies. The TCGA data on the clinical characteristics of the patients and RNASeq counts was downloaded from the GDC TCGA Acute Myeloid Leukemia (LAML) cohort, which included 151 patients (https://xenabrowser.net/datapages/).
From the RNAcentral database (https://rnacentral.org/search?q=RNA), we downloaded the full set of human lncRNAs found in the Ensembl database. A total of 27.969 Ensemble ID codes for lncRNAs were retrieved and using a Venn diagram (http://bioinfogp.cnb.csic.es/tools/venny/), we identified the list of lncRNAs from the TCGA dataset, reaching a total of 275 lncRNAs. We ran a wide-range gene expression analysis with an online gene expression analysis tool based on the Jupyter Notebook in Python (126).
The RNASeq data showed an evenly distributed million reads per sample across the three analyzed cytogenetic risk groups: Figure 3 compares the expression level of lncRNAs in poor vs. normal risk category patients, Figure 4 depicts favorable vs. normal risk category, whereas Figure 5 depicts favorable vs. poor risk patients.
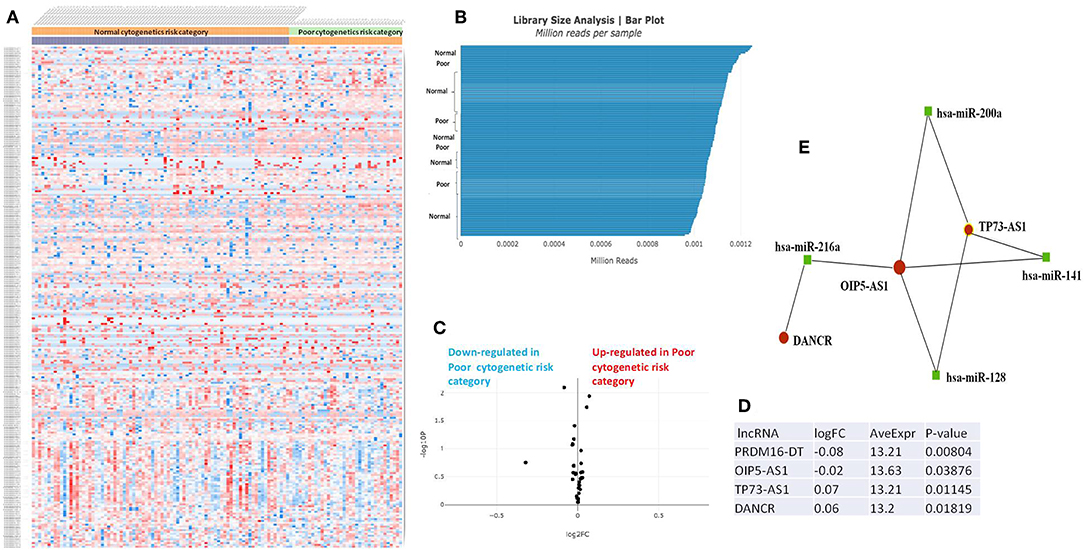
Figure 3. (A) Heat map comparing the expression level of lncRNAs in Poor vs. Normal risk category. (B) Library Size Analysis between TCGA samples belonging to Poor or Normal risk category. (C) Volcano plot analysis of lncRNA expression differentiating Poor vs. Normal risk category. The set FC was at 1 and p value at <0.05. (D) Table of lncRNAs with significant different expression between the Poor and Normal risk category, their fold change, average expression and p-value. (E) LncRNA-microRNA interaction network analyzing the common ceRNA activities of the lncRNAs with different expression between Poor or Normal risk category.
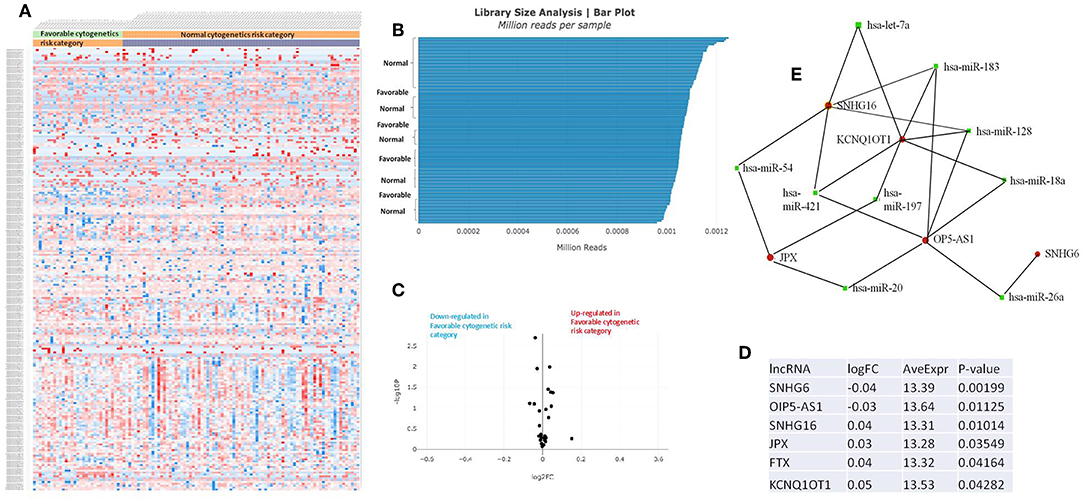
Figure 4. (A) Heat map comparing the expression level of lncRNAs in Favorable vs. Normal risk category. (B) Library Size Analysis between TCGA samples belonging to Favorable or Normal risk category. (C) Volcano plot analysis of lncRNA expression differentiating Favorable vs. Normal risk category. The set FC was at 1 and p value at <0.05. (D) Table of lncRNAs with significant different expression between the Favorable and Normal risk category, their fold change, average expression, and p-value. (E) LncRNA-microRNA interaction network analyzing the common ceRNA activities of the lncRNAs with different expression between Favorable or Normal risk category.
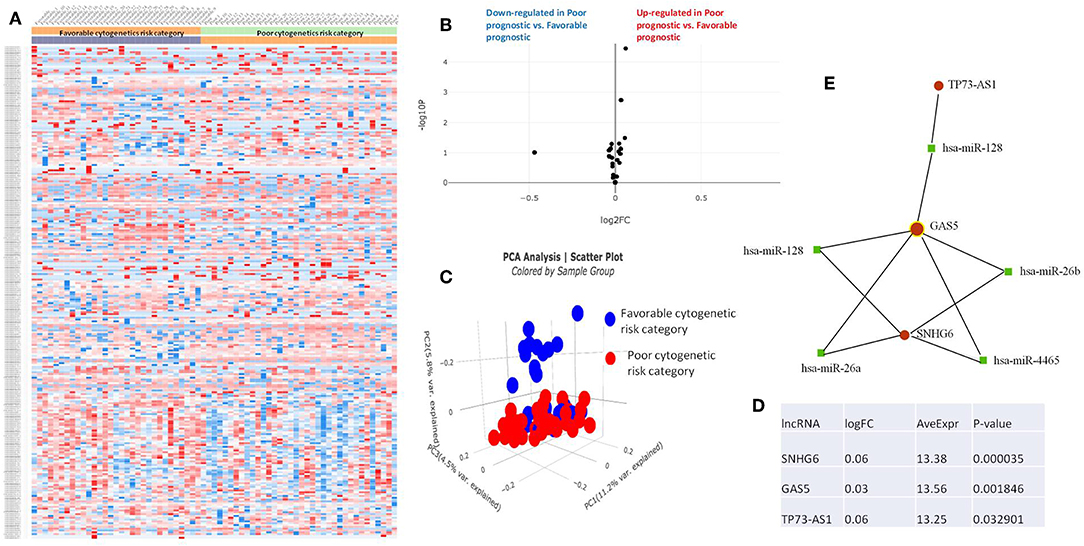
Figure 5. (A) Heat map comparing the expression level of lncRNAs in Favorable vs. Poor risk category. (B) Volcano plot analysis of lncRNA expression differentiating Poor vs. Normal risk category. The set FC was at 1 and p value at <0.05. (C) Principal component analysis (PCA) comparing the Favorable risk category vs. Poor risk category. (D) Table of lncRNAs with significant different expression between the Poor and Normal risk category, their fold change, average expression and p-value. (E) LncRNA-microRNA interaction network analyzing the common ceRNA activities of the lncRNAs with different expression between Poor or Normal risk category.
The heatmaps comparing the expression of lncRNAs between the poor vs. normal risk category, favorable vs. normal risk category, poor vs. favorable risk category, showed a heterogeneous distribution of lncRNA expression. By calculating the fold change between the peripheral blood gene expressions between each compared category, we were able to identify a total number of 10 lncRNAs that were significantly differentially expressed between each of the two analyzed groups.
Principal Component Analysis (PCA) revealed a significant clustering of lncRNA expression in the case of favorable vs. poor prognostic cytogenetic risk category (Figure 5). With the help of the online tool miRNet (https://www.mirnet.ca/miRNet/faces/home.xhtml), we were able to construct three lncRNA-microRNA interaction networks that have shown common ceRNA activity between the lncRNAs with differential expression between the analyzed groups. To better illustrate the difference in expression between the three groups, we developed violin plots in the Jupyter Notebook. PRDM16-DT has a highly statistically significant (p <0.001) downregulated expression in the favorable risk category in comparison with normal or poor cytogenetic risk categories (Figure 6).
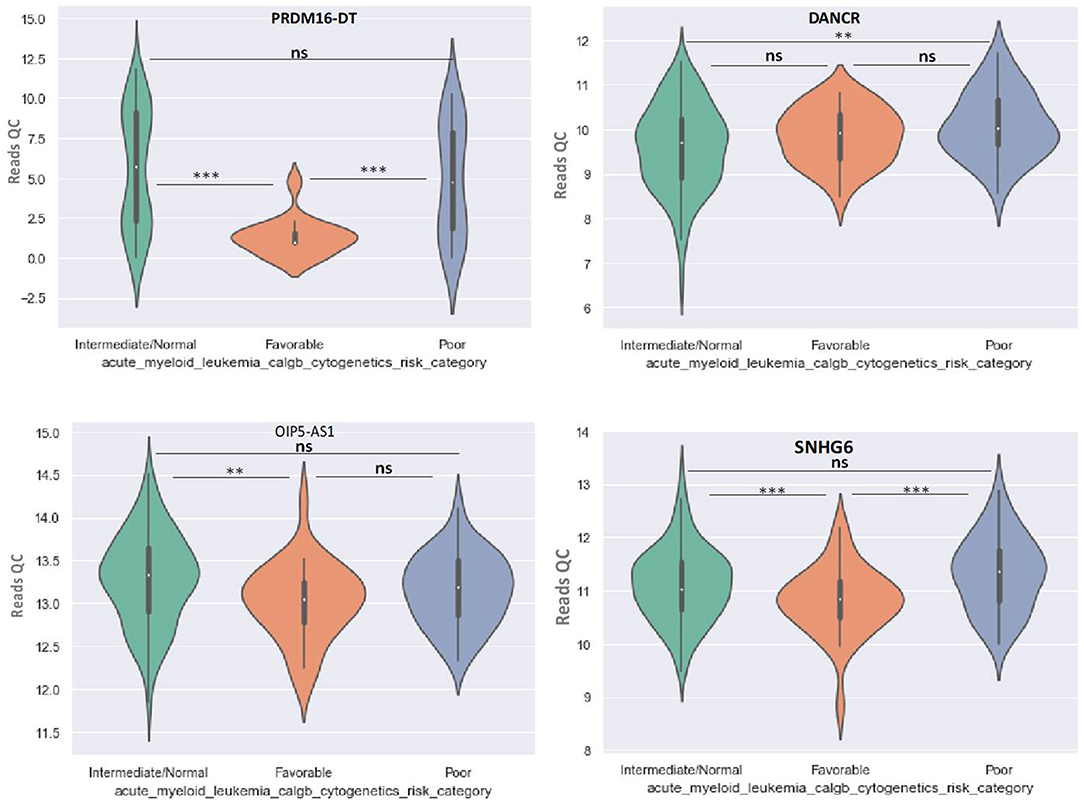
Figure 6. Violin plot of lncRNA with different expression between Normal, Favorable, and Poor risk category for PRDM16-DT, DANCR, OIP5-AS1, SNHG16 lncRNAs. ** represents statistically significant data. *** represents very highly statistically significant data.
DANCR is upregulated in poor prognostic cytogenetic patients in comparison to the intermediate/normal risk category, while the difference between favorable and poor or normal risk is not significant (Figure 6).
OIP5-AS1 is down-regulated in favorable risk categories in comparison with normal risk category (p < 0.01). The difference in expression between the other two categories is not significant (Figure 6). SNHG16, JPX FTX, and KCNQ1OT1 are significantly upregulated in the cytogenetically favorable risk category. The difference in expression between the other two categories is not significant (Figure 7).
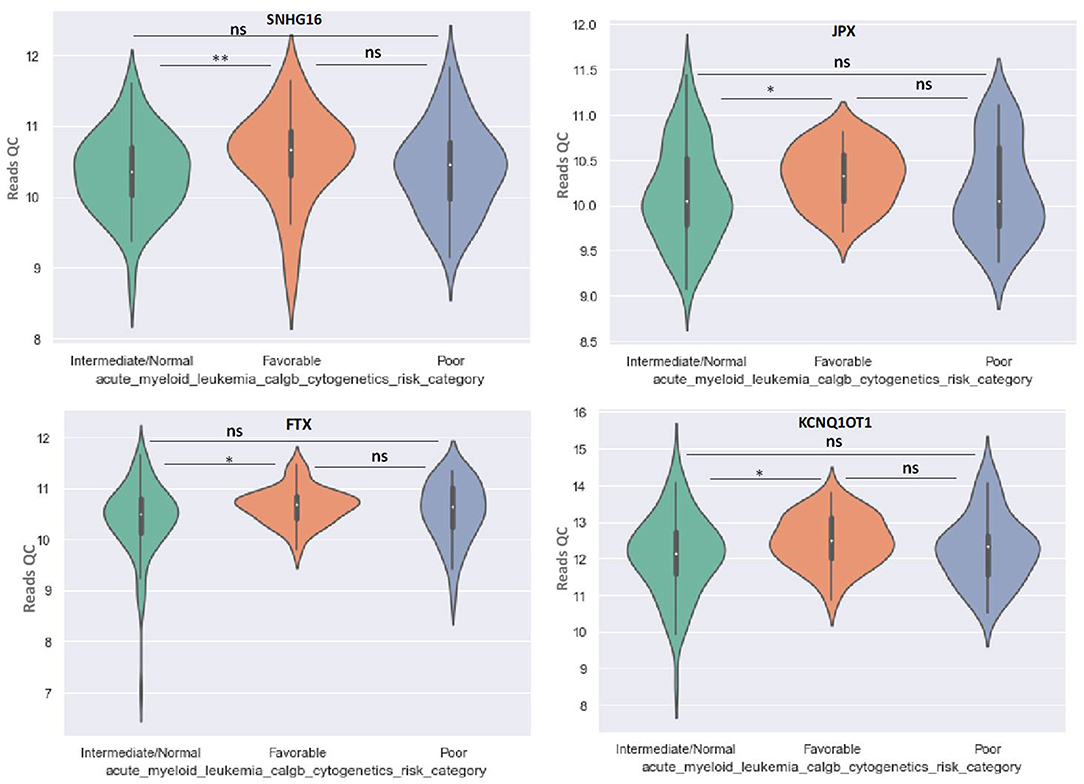
Figure 7. Violin plot of lncRNA with different expression between Normal, Favorable, and Poor risk category for SNHG16, JPX, FTX, and KCNQ1OT1 lncRNAs. * represents statistically significant data. ** represents very highly statistically significant data.
GAS5 can differentiate between favorable and poor risk categories, being overexpressed in patients with poor cytogenetic prognostic (Figure 8). TP73-AS1 is upregulated in poor risk category in comparison with favorable and normal risk categories (p < 0.01) (Figure 8).
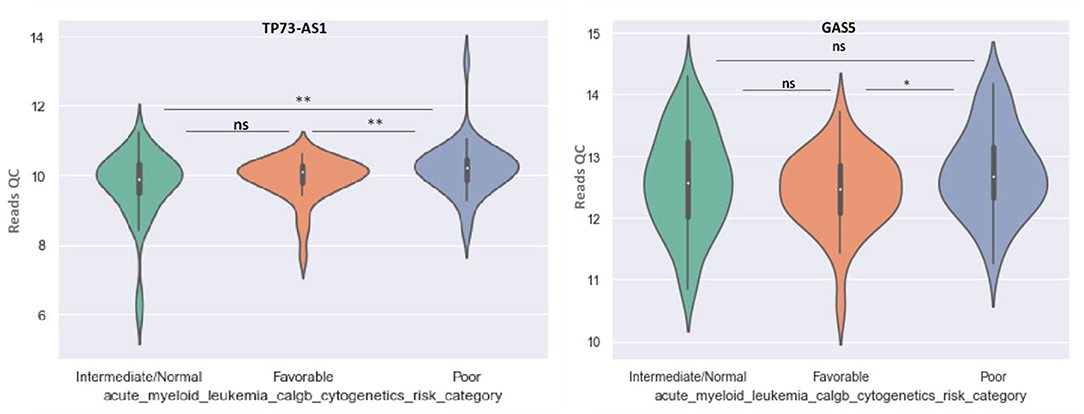
Figure 8. Violin plot of lncRNA with different expression between Normal, Favorable, and Poor risk category for TP73-AS1 and GAS5 lncRNAs.
There is published data on analysis made in AML cell lines after long-term exposure to chemotherapeutics or bone marrow analysis post-treatment. The lncRNA named urothelial carcinoma-associated 1 (UCA1) is generally overexpressed in AML and it was proven that acquired chemoresistance to adriamycin in HL60 cells is correlated with increased expression of UCA1. In adriamycin–resistant AML cells UCA1 sponges miR-125a thus releasing hexokinase 2 (HK2) and hypoxia-inducible factor 1α (HIF-1α) from its control. The overexpression of UCA1 in post-treatment AML cells was confirmed by clinical analysis done on pediatric patients (112). Linc00239 is another lncRNA whose increased expression is related to a higher resistance to doxorubicin, as shown by the KG-1 and HL-60 AML cell lines. However, it is involved in the intrinsic resistance to chemotherapy and its expression in acquired chemoresistance was not evaluated (90). HOXA-AS2 is overexpressed in the bone marrow of patients who acquired adriamycin resistance. There are two downstream targets, miR-520c-3p and S100A4, that may explain the involvement of this lncRNA in chemoresistance. Still, more experimental data is needed (127). Correlations between lncRNAs and the clinical outcome of AML treatment are still not validated in an experimental setting. This is the case of IRAIN, a lncRNA whose low expression is correlated with a worse prognostic after chemotherapy by a higher relapse rate (109). The increased mannosylation (addition of a mannose glycoside) on the membrane proteins is a process through which the AML cells U937 acquire adriamycin resistance. ALG3 alpha-1,3- mannosyltransferase is an enzyme responsible for this process. In ADR/U937 cells, the lncRNA FTX is overexpressed. This lncRNA targets miR-342, thus decreasing its expression and it releases ALG3 mRNA from the RNA interference inhibition (128).
The most commonly course of action in the case of a combinatorial treatment that would involve a lncRNA and a chemotherapeutic would be first sensitizing the cells, with the help of the lncRNA therapeutic inhibition or overexpression, depending on its initial pathological expression level, and then cells are completely eliminated with the help of the chemotherapeutic. In adriamycin-resistant AML cells, if the lncRNA UCA1 is exogenously inhibited, the chemoresistance is impaired, thus the cells are killed by their exposure to adriamycin (112). The knockdown of HOXA-AS2 in adriamycin-resistant AML cells, and further exposure of cells to the same chemotherapeutic resulted in decreased cell viability in vitro and tumor growth in vivo, thus proving the resensitizing process of AML cells (127).
In order to get a better view of the malignant function of the lncRNAs found to have different expression values between the included cytogenetic risk categories, we researched the literature to find the role of these lncRNAs in other malignancies, as well as their previously reported functions in AML (Table 4).
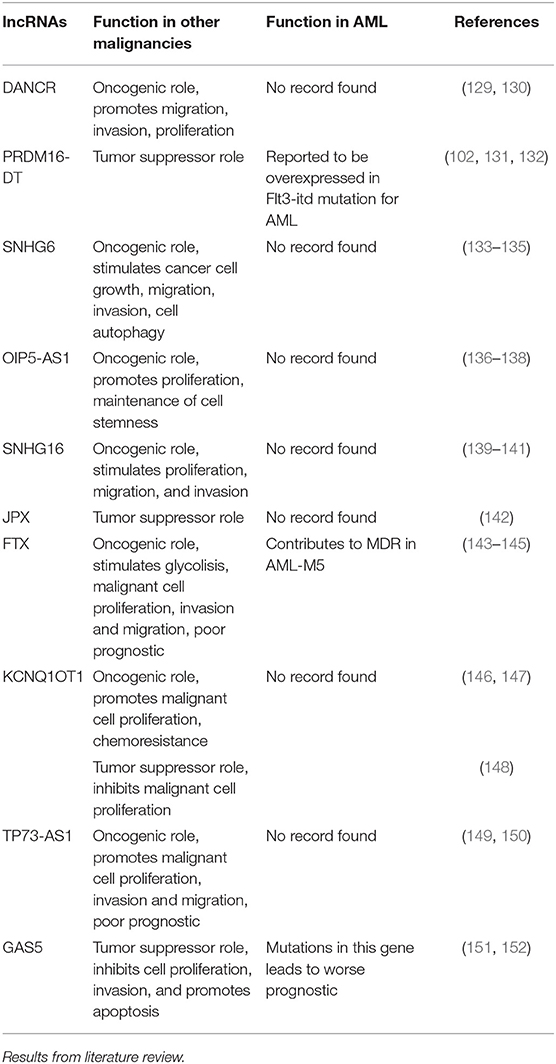
Table 4. General and specific role of lncRNAs with differential expression between different cytogenetic risk categories in AML.
Long Non-Coding RNAs in Myeloid Malignancies—Analysis of Preclinical in vivo Data
The use of mouse models has the advantage of testing in vivo the findings initially reported in vitro, and potentially using these findings for therapeutic purposes, specifically new therapies for hematological malignancies with no cure other than allogeneic transplantation, as is the case with MDS currently.
ANRIL is a lncRNA which has been reported to reprogram glucose metabolism in AML cells in vitro through the induced expression of the Adiponectin receptor (AdipoR1). The in vivo experimental model, in which NOD-SCID mice were injected with either shRNA against ANRIL or scrambled shRNA, followed by flow cytometry analysis of their BM sample, validated the in vitro data and offered more reliability to the study (153). The same experimental design was used in order to prove the role of HOXA-AS2 overexpression and inhibition of the miR-520c-3p/S100A4 axis in the case of chemoresistance development in AML (127). HOTAIR is a lncRNA which was shown to decrease the in vivo tumor formation capacity through the demethylation of the promoter region in the case of the tumor suppressor gene HOXA5 (154). The in vitro silencing of the zinc finger antisense 1 (ZFAS1) lncRNA led to a higher percentage of AML apoptotic cells and a slower rate of proliferation of AML cells, while in vivo it resulted in the formation of smaller tumors, as compared to untreated mice (94).
We have previously reported the potential role of the lncRNA CCAT2 in the highly amplified 8q24.21 region, with implications for both myeloid malignancies and digestive cancers (55, 129, 155). Fosselteder et al. describe CCAT2 as a lncRNA with genomic localization at the chromosomal region 8q24 amplified in many malignancies (130). This was first described in colon cancer by Ling et al. (131) and later in breast adenocarcinoma by Redis et al. (129, 132). The 8q24 region is highly conserved and encompasses the MYC gene. In myeloid malignancies, a joint study between our institutions was initiated from the observation that CCAT2 is overexpressed in CD34+ cells in the bone marrow and in mononuclear cells from peripheral blood isolated from MDS patients, as compared with age-matched healthy individuals. We later discovered that the SNP rs6983267 overlapping CCAT2 is found in two allelic forms that contain either T or G and by using allele specific CCAT2 transgenic mice, confirming this data on patient samples from the US and Romania. In vivo, using transgenic mice after 7–9 months, both CCAT2-G mice and CCAT2-T mice had clinical symptoms of an underlying malignancy when compared to the wild-type siblings, having severe leukopenia and lymphocytopenia, moderate anemia and thrombocytosis (55). The CCAT2 role in cancer progression is valid for other types of malignancies and the amplified cancer-associated chromosome 8q24 modulates the Wnt target gene Myc (133), thus affecting the proliferative and self-renewal potential of cancer stem cells (134, 135). Even if this lncRNA was validated in several solid malignancies, including hepatocellular carcinoma, renal cancer and pancreatic cancer (136–138), it has yet to be properly validated in myeloid malignancies. Should it be, it holds great promise to explain the progression of a myelodysplastic syndrome into an acute leukemia.
One of the limitations of the manuscript is the insufficient information presented regarding the potential use of lncRNAs as therapeutics. Very little information has been published thus far regarding the topic, as the mechanisms that underlie their function have yet to be fully elucidated. One direction would be to use these molecules as potential targets. If a long non-coding RNA functions via cis regulatory mechanisms, it is extremely challenging to restore its function without expressing at the specific genomic locus (139). However, blocking of the function of long non-coding RNAs could be achieved by several strategies, with the most straightforward approach of downregulation with RNA interference (140). To regain the function of a lncRNA that is lost or downregulated in a myeloid malignancy, the simplest method is to supply with synthetic lncRNA molecules with same function (141). This could be achieved with a non-coding RNA mimic or with its expression vectors (142, 143). When transfected into cells, lncRNA mimics could be processed into a single-strand RNA molecule to target coding genes similar to the endogenous lncRNA, but viable experimental data has yet to be published.
Still, lncRNAs may represent interesting potential targets in AML therapy and monitoring, as they constitute most cellular transcripts and play pivotal roles in hematopoiesis (144, 145). For example, the lncRNA linc00239 (NR_026774.1), 662 nucleotides in length, is upregulated in AML patients, on malignant behaviors and chemosensitivity in AML cells. The presence of linc00239 increases chemoresistance to doxorubicin in AML cells partially by preventing doxorubicin-induced apoptotic cell death and is linked to the activation of the phosphatidylinositol 3-kinase (PI3K)/Akt/mammalian target of rapamycin (mTOR) pathway. Thus, the inhibition of PI3K/Akt/mTOR using 1 μM NVP-BEZ235 (BEZ) abolished the inhibitory effect of linc00239 on chemosensitivity and the preventative effect on doxorubicin-induced cell death (90). Their use could be implemented in risk stratification and prognosis in the clinic. Thus, in a clinical trial setting that enrolled 275 non-M3 AML patients in Taipei, Tsai et al. have shown that higher lncRNA scores were significantly associated with older age and adverse gene mutations. Further, the higher-score patients had shorter overall and disease-free survival than lower-score patients, which were also confirmed in both internal and external validation cohorts (TCGA database) (104). Multivariate analyses linked a lncRNA score to an independent prognosticator in AML, irrespective of the risk based on the ELN classification. In the ELN intermediate-risk subgroup, lncRNA scoring system could well dichotomize the patients into two groups with distinct prognosis. Within the ELN intermediate-risk subgroup, they showed that allogeneic hematopoietic stem cell transplantation could provide better outcomes on patients with higher lncRNA scores. Through a bioinformatics approach, they identified high lncRNA scores correlated with leukemia/hematopoietic stem cell signatures. Thus, incorporation of lncRNA scoring system in the 2017 ELN classification can improve risk-stratification of AML patients and help in clinical decision-making.
Conclusion
There are several lncRNAs that have been implicated in several roles in AML. ANRIL, RP11-732M18.3, LNC_104449, CASC15, CCDC26, DANCR, LINC00467, MIR9-3HG, AL035071.1, AP001042.1, RP11-395P13.6-001, FAM30A, H22954, LEF1-AS1, LINC00152, LINC00239, LINC00265, LINC00319, LINC00504, LINC00899, LINC00926, LNC177417, LRRC75A-AS1, MALAT1, NEAT1, PANDAR, PILNA, RP11-222k16.2, RP11-305O.6, SBF2-AS1, TUG1, UCA1, ZFAS1 have potential oncogenic roles in AML. RP11-704M14.1, SFMBT2-4:1, IRAIN, TET2 function as tumor suppressors in AML.
There are two lncRNAs with both oncogenic and tumor suppressor roles in AML. MEG3 is upregulated in AML-M3, but in AML cell lines is downregulated. CRINDE is downregulated in AML-M3 and upregulated in AML bone marrow samples and cell lines.
There are lncRNAs with specific expressions for each FAB AML subtype. H19 is upregulated in M1-2 AML. LINC00877, RP11-84C10.2, RP11-848P1.3, ZNF667-AS1 are downregulated in M3 AML, and LINC02082-201, AC009495.2 are upregulated in M3 AML. HOTTIP, SNHG5, CCAT1 are upregulated specifically in M4-5 AML. CCAT1, PVT1, and XLOC_109948 are overexpressed in AML patients bearing increased risk mutations. HOTAIR is upregulated only in stem cells from bone marrow samples of AML patients.
Our original analysis of TCGA data on AML revealed that PRDM16-DT is downregulated, and DANCR is upregulated in poor cytogenetic prognostic AML samples compared with normal cytogenetic risk. OIP5-AS1 is downregulated in both poor and favorable prognostic compared with normal cytogenetic risk. SNHG6 is underexpressed in poor prognostic compared with intermediate cytogenetic risk and overexpressed in poor prognostic compared with favorable prognostic. SNHG16, JPX, FTX, KCNQ1OT1 are upregulated in AML samples with favorable risk as compared with normal risk. GAS5 is overexpressed in poor prognostic compared with favorable prognostic, whereas TP73-AS1 is upregulated specifically in poor prognostic patients, being overexpressed in comparison with favorable and normal cytogenetic risk. These lncRNAs have the potential of becoming future biomarkers of cytogenetic risk, however they still need further validation on bone marrow samples, peripheral blood from another cohort, along with additional gene expression analysis methods.
The potential of lncRNAs in their use as biomarkers or therapeutic targets is still in its infancy. More knowledge has to be gained on their established molecular interactions, biological roles, standardization of isolation and gene expression analysis. As follows, more data is needed to fully introduce the idea of clinical uses of lncRNAs in the clinical evaluation or treatment of AML, especially in a large cohort non-interventional clinical trial that would be able to compare the sensitivity and specificity of currently applied diagnostic/prognostic methods.
Author Contributions
A-AZ did the bioinformatics analysis. CT and A-AZ wrote the manuscript. IS critically revised the manuscript. GC and IB-N contributed to the design of the manuscript and supervised the project.
Funding
A-AZ was financed by an internal grant of the Iuliu Hatieganu University of Medicine and Pharmacy—School for Doctoral Studies (PCD 2018-2019). GC is the Felix L. Haas Endowed Professor in Basic Science. Work in Dr. Calin's laboratory was supported by National Institutes of Health (NIH/NCATS) grant UH3TR00943-01 through the NIH Common Fund, Office of Strategic Coordination (OSC), the NCI grants 1R01 CA182905-01 and 1R01CA222007-01A1, an NIGMS 1R01GM122775-01 grant, a U54 grant #CA096297/CA096300—UPR/MDACC Partnership for Excellence in Cancer Research 2016 Pilot Project, a Team DOD (CA160445P1) grant, a Chronic Lymphocytic Leukemia Moonshot Flagship project, a Sister Institution Network Fund (SINF) 2017 grant, and the Estate of C. G. Johnson, Jr. IB-N was supported by a POC Grant, Competitively Operational Program, 2014–2020, no. 35/01.09.2016, MySMIS Code 103375 (CANTEMIR) and by Funding Agency-Ministry of Research and Innovation PNCDI III 2015-2020—ECHITAS, no. 29PFE/18.10.2018. CT was supported from three ongoing Grants of the Romanian Government: Institutional Development Fund (CNFIS-FDI-2019-0636), a joint Romania–China Bilateral Collaboration Grant 2018–2019 (contract 14/2018) and by a National Research Grant awarded for Frontiers Research Projects (PN-III-P4-ID-PCCF-2016-112) awarded to the Babes Bolyai University in collaboration with the Ion Chiricuta Oncology Institute Cluj Napoca.
Conflict of Interest
The authors declare that the research was conducted in the absence of any commercial or financial relationships that could be construed as a potential conflict of interest.
Abbreviations
GAS5, Growth Arrest Specific 5; AML, acute myeloid leukemia; ANRIL, antisense RNA in the INK4 locus; BM, bone marrow CASC15, cancer susceptibility 15; CCAT1, colon cancer associated transcript 1; CRNDE, colorectal neoplasia differentially expressed; DANCR, differentiation antagonizing non-protein coding RNA; FTX, FTX transcript, XIST regulator; H19-H19, imprinted maternally expressed transcript; HOTAIR, HOX transcript antisense RNA; HOTTIP, HOXA distal transcript antisense RNA; IRAIN, IGF1R antisense imprinted non-protein coding RNA; JPX-PX transcript, XIST activator; KCNQ1OT1, KCNQ1 opposite strand/antisense transcript 1; LEF1-AS1, lymphoid enhancer binding factor 1 antisense RNA 1; MALAT1, metastasis associated lung adenocarcinoma transcript 1; MAPRE1, microtubule associated protein RP/EB family member 1; MEG3, maternally expressed 3; NEAT1, nuclear paraspeckle assembly transcript 1; NK cells, natural killer cells; NTRK3, neurotrophic receptor tyrosine kinase 3; OIP5-AS1, Opa interacting protein 5 antisense RNA 1; PANDAR, promoter of CDKN1A antisense DNA damage activated RNA; PRDM16-DT, PR/SET domain 16 divergent transcript; SBF2-AS1, SET binding factor 2-antisense RNA 1; SNHG, small nucleolar RNA host gene; SOX4, SRY-Box 4; TET2, tet methylcytosine dioxygenase 2; TP73-AS1, tumor protein P73 antisense RNA 1; TUG1, Taurine up-regulated 1; UCA1, urothelial cancer associated 1; ZFAS1, ZNFX1 antisense RNA 1.
References
1. Garcia-Garcia A, de Castillejo CL, Mendez-Ferrer S. BMSCs and hematopoiesis. Immunol Lett. (2015) 168:129–35. doi: 10.1016/j.imlet.2015.06.020
2. Ghiaur G, Ferkowicz MJ, Milsom MD, Bailey J, Witte D, Cancelas JA, et al. Rac1 is essential for intraembryonic hematopoiesis and for the initial seeding of fetal liver with definitive hematopoietic progenitor cells. Blood. (2008) 111:3313–21. doi: 10.1182/blood-2007-08-110114
3. Ma O, Hong S, Guo H, Ghiaur G, Friedman AD. Granulopoiesis requires increased C/EBPα compared to monopoiesis, correlated with elevated Cebpa in immature G-CSF receptor versus M-CSF receptor expressing cells. PLoS ONE. (2014) 9:e95784. doi: 10.1371/journal.pone.0095784
4. Quesenberry PJ, Colvin GA, Abedi M, Lambert JF, Moore B, Demers D, et al. The marrow stem cell: the continuum. Bone Marrow Transplant. (2003) 32(Suppl. 1):S19–22. doi: 10.1038/sj.bmt.1703938
5. Quesenberry PJ, Colvin GA, Lambert JF. The chiaroscuro stem cell: a unified stem cell theory. Blood. (2002) 100:4266–71. doi: 10.1182/blood-2002-04-1246
6. Quesenberry PJ, Dooner G, Dooner M, Colvin G. The stem cell continuum: considerations on the heterogeneity and plasticity of marrow stem cells. Stem Cell Rev. (2005) 1:29–36. doi: 10.1385/SCR:1:1:029
7. Quesenberry PJ, Colvin GA, Abedi M, Dooner G, Dooner M, Aliotta J, et al. The stem cell continuum. Ann N Y Acad Sci. (2005) 1044:228–35. doi: 10.1196/annals.1349.028
8. Laurenti E, Gottgens B. From haematopoietic stem cells to complex differentiation landscapes. Nature. (2018) 553:418–26. doi: 10.1038/nature25022
9. Gottgens B. Regulatory network control of blood stem cells. Blood. (2015) 125:2614–20. doi: 10.1182/blood-2014-08-570226
10. Morrison SJ, Scadden DT. The bone marrow niche for haematopoietic stem cells. Nature. (2014) 505:327–34. doi: 10.1038/nature12984
11. Park D, Sykes DB, Scadden DT. The hematopoietic stem cell niche. Front Biosci. (2012) 17:30–9. doi: 10.2741/3913
12. Papayannopoulou T, Scadden DT. Stem-cell ecology and stem cells in motion. Blood. (2008) 111:3923–30. doi: 10.1182/blood-2007-08-078147
13. Kim M, Civin CI, Kingsbury TJ. MicroRNAs as regulators and effectors of hematopoietic transcription factors. Wiley Interdiscip Rev RNA. (2019) 10:e1537. doi: 10.1002/wrna.1537
14. Sweeney CL, Teng R, Wang H, Merling RK, Lee J, Choi U, et al. Molecular analysis of neutrophil differentiation from human induced pluripotent stem cells delineates the kinetics of key regulators of hematopoiesis. Stem Cells. (2016) 34:1513–26. doi: 10.1002/stem.2332
15. Fabbri M, Croce CM, Calin GA. MicroRNAs in the ontogeny of leukemias and lymphomas. Leuke Lymphoma. (2009) 50:160–70. doi: 10.1080/10428190802535114
16. Georgantas RW III, Hildreth R, Morisot S, Alder J, Liu CG, Heimfeld S, et al. CD34+ hematopoietic stem-progenitor cell microRNA expression and function: a circuit diagram of differentiation control. Proc Natl Acad Sci USA. (2007) 104:2750–5. doi: 10.1073/pnas.0610983104
17. Calin GA, Croce CM. Genomics of chronic lymphocytic leukemia microRNAs as new players with clinical significance. Semin Oncol. (2006) 33:167–73. doi: 10.1053/j.seminoncol.2006.01.010
18. Fabbri M, Garzon R, Andreeff M, Kantarjian HM, Garcia-Manero G, Calin GA. MicroRNAs and noncoding RNAs in hematological malignancies: molecular, clinical and therapeutic implications. Leukemia. (2008) 22:1095–105. doi: 10.1038/leu.2008.30
19. Llave C, Xie Z, Kasschau KD, Carrington JC. Cleavage of Scarecrow-like mRNA targets directed by a class of Arabidopsis miRNA. Science. (2002) 297:2053–6. doi: 10.1126/science.1076311
20. International Human Genome Sequencing Consortium. Finishing the euchromatic sequence of the human genome. Nature. (2004) 431:931–45. doi: 10.1038/nature03001
21. Cheng J, Kapranov P, Drenkow J, Dike S, Brubaker S, Patel S, et al. Transcriptional maps of 10 human chromosomes at 5-nucleotide resolution. Science. (2005) 308:1149–54. doi: 10.1126/science.1108625
22. Kapranov P, Cheng J, Dike S, Nix DA, Duttagupta R, Willingham AT, et al. RNA maps reveal new RNA classes and a possible function for pervasive transcription. Science. (2007) 316:1484–8. doi: 10.1126/science.1138341
23. Mattick JS, Makunin IV. Non-coding RNA. Hum Mol Genet. (2006) 15:R17–29. doi: 10.1093/hmg/ddl046
24. Esteller M. Non-coding RNAs in human disease. Nature Rev Genet. (2011) 12:861–74. doi: 10.1038/nrg3074
25. Vasudevan S, Tong Y, Steitz JA. Switching from repression to activation: microRNAs can up-regulate translation. Science. (2007) 318:1931–4. doi: 10.1126/science.1149460
26. Abdulsalam AH, Sabeeh N, Bain BJ. Myeloblasts with unusual morphology. Am J Hematol. (2011) 86:499. doi: 10.1002/ajh.21976
27. Delas MJ, Jackson BT, Kovacevic T, Vangelisti S, Munera Maravilla E, Wild SA, et al. lncRNA spehd regulates hematopoietic stem and progenitor cells and is required for multilineage differentiation. Cell Rep. (2019) 27:719–29.e6. doi: 10.1016/j.celrep.2019.03.080
28. D'Souza SS, Maufort J, Kumar A, Zhang J, Smuga-Otto K, Thomson JA, et al. GSK3β inhibition promotes efficient myeloid and lymphoid hematopoiesis from non-human primate-induced pluripotent stem cells. Stem Cell Rep. (2016) 6:243–56. doi: 10.1016/j.stemcr.2015.12.010
30. Zeidan AM, Shallis RM, Wang R, Davidoff A, Ma X. Epidemiology of myelodysplastic syndromes: why characterizing the beast is a prerequisite to taming it. Blood Rev. (2019) 34:1–15. doi: 10.1016/j.blre.2018.09.001
32. Kennedy JA, Ebert BL. Clinical implications of genetic mutations in myelodysplastic syndrome. J Clin Oncol. (2017) 35:968–74. doi: 10.1200/JCO.2016.71.0806
33. Steensma DP. Good riddance to the term “refractory anemia” in myelodysplastic syndromes. Leuk Res. (2016) 51:22–6. doi: 10.1016/j.leukres.2016.10.007
34. Arber DA, Orazi A, Hasserjian R, Thiele J, Borowitz MJ, Le Beau MM, et al. The 2016 revision to the World Health Organization classification of myeloid neoplasms and acute leukemia. Blood. (2016) 127:2391–405. doi: 10.1182/blood-2016-03-643544
35. van Spronsen MF, Westers TM, Rozema H, Ossenkoppele GJ, Kibbelaar RE, Hoogendoorn M, et al. Validation of and proposals for refinements of the WHO 2016 classification for myelodysplastic syndromes. Am J Hematol. (2017) 92:E631–4. doi: 10.1002/ajh.24867
36. Bennett JM. Changes in the Updated 2016: WHO classification of the myelodysplastic syndromes and related myeloid neoplasms. Clin Lymphoma Myeloma Leuk. (2016) 16:607–9. doi: 10.1016/j.clml.2016.08.005
37. Montalban-Bravo G, Garcia-Manero G. Myelodysplastic syndromes: 2018 update on diagnosis, risk-stratification and management. Am J Hematol. (2018) 93:129–47. doi: 10.1002/ajh.24930
38. Wall M. Recurrent cytogenetic abnormalities in myelodysplastic syndromes. Methods Mol Biol. (2017) 1541:209–22. doi: 10.1007/978-1-4939-6703-2_18
39. Ades L, Itzykson R, Fenaux P. Myelodysplastic syndromes. Lancet. (2014) 383:2239–52. doi: 10.1016/S0140-6736(13)61901-7
40. Desai P, Hassane D, Roboz GJ. Clonal hematopoiesis and risk of acute myeloid leukemia. Best Pract Res Clin Haematol. (2019) 32:177–85. doi: 10.1016/j.beha.2019.05.007
41. Minzel W, Venkatachalam A, Fink A, Hung E, Brachya G, Burstain I, et al. Small molecules co-targeting CKIα and the transcriptional kinases CDK7/9 control AML in preclinical models. Cell. (2018) 175:171–85.e25. doi: 10.1016/j.cell.2018.07.045
42. Abelson S, Collord G, Ng SWK, Weissbrod O, Mendelson Cohen N, Niemeyer E, et al. Prediction of acute myeloid leukaemia risk in healthy individuals. Nature. (2018) 559:400–4. doi: 10.1038/s41586-018-0317-6
43. Shlush LI, Mitchell A, Heisler L, Abelson S, Ng SWK, Trotman-Grant A, et al. Tracing the origins of relapse in acute myeloid leukaemia to stem cells. Nature. (2017) 547:104–8. doi: 10.1038/nature22993
44. Alexander TB, Gu Z, Iacobucci I, Dickerson K, Choi JK, Xu B, et al. The genetic basis and cell of origin of mixed phenotype acute leukaemia. Nature. (2018) 562:373–9. doi: 10.1038/s41586-018-0436-0
45. Sas V, Blag C, Zaharie G, Puscas E, Lisencu C, Andronic-Gorcea N, et al. Transient leukemia of down syndrome. Crit Rev Clin Lab Sci. (2019) 56:247–59. doi: 10.1080/10408363.2019.1613629
46. Barbieri I, Tzelepis K, Pandolfini L, Shi J, Millan-Zambrano G, Robson SC, et al. Promoter-bound METTL3 maintains myeloid leukaemia by m(6)A-dependent translation control. Nature. (2017) 552:126–31. doi: 10.1038/nature24678
47. Corces MR, Chang HY, Majeti R. Preleukemic hematopoietic stem cells in human acute myeloid leukemia. Front Oncol. (2017) 7:263. doi: 10.3389/fonc.2017.00263
48. Hirsch P, Zhang Y, Tang R, Joulin V, Boutroux H, Pronier E, et al. Genetic hierarchy and temporal variegation in the clonal history of acute myeloid leukaemia. Nat Commun. (2016) 7:12475. doi: 10.1038/ncomms12475
49. Chen Z, Shojaee S, Buchner M, Geng H, Lee JW, Klemm L, et al. Signalling thresholds and negative B-cell selection in acute lymphoblastic leukaemia. Nature. (2015) 521:357–61. doi: 10.1038/nature14231
50. Mullighan CG. The genomic landscape of acute lymphoblastic leukemia in children and young adults. Hematology Am Soc Hematol Educ Program. (2014) 2014:174–80. doi: 10.1182/asheducation-2014.1.174
51. Wong TN, Ramsingh G, Young AL, Miller CA, Touma W, Welch JS, et al. Role of TP53 mutations in the origin and evolution of therapy-related acute myeloid leukaemia. Nature. (2015) 518:552–5. doi: 10.1038/nature13968
52. Dima D, Oprita L, Rosu AM, Trifa A, Selicean C, Moisoiu V, et al. Adult acute megakaryoblastic leukemia: rare association with cytopenias of undetermined significance and p210 and p190 BCR-ABL transcripts. OncoTargets Ther. (2017) 10:5047–51. doi: 10.2147/OTT.S146973
53. Thein MS, Ershler WB, Jemal A, Yates JW, Baer MR. Outcome of older patients with acute myeloid leukemia: an analysis of SEER data over 3 decades. Cancer. (2013) 119:2720–7. doi: 10.1002/cncr.28129
54. Derwich K, Mitkowski D, Skalska-Sadowska J. Acute myeloid leukemia in pediatric patients: a review about current diagnostic and treatment approaches. In: Lasfar A, editor. Myeloid Leukemia. London: IntechOpen (2018). doi: 10.5772/intechopen.70937
55. Shah MY, Ferracin M, Pileczki V, Chen B, Redis R, Fabris L, et al. Cancer-associated rs6983267 SNP and its accompanying long noncoding RNA CCAT2 induce myeloid malignancies via unique SNP-specific RNA mutations. Genome Res. (2018) 28:432–47. doi: 10.1101/gr.225128.117
56. Creutzig U, Zimmermann M, Reinhardt D, Rasche M, von Neuhoff C, Alpermann T, et al. Changes in cytogenetics and molecular genetics in acute myeloid leukemia from childhood to adult age groups. Cancer. (2016) 122:3821–30. doi: 10.1002/cncr.30220
57. Gupta M, Mahapatra M, Saxena R. Cytogenetics' impact on the prognosis of acute myeloid leukemia. J Lab Physicians. (2019) 11:133–7. doi: 10.4103/JLP.JLP_164_18
58. Tang P, Xie M, Wei Y, Xie X, Chen D, Jiang Z. A 10-long non-coding RNA-based expression signature as a potential biomarker for prognosis of acute myeloid leukemia. Med Sci Monit. (2019) 25:4999–5004. doi: 10.12659/MSM.917182
59. Roloff GW, Griffiths EA. When to obtain genomic data in acute myeloid leukemia (AML) and which mutations matter. Blood Adv. (2018) 2:3070–80. doi: 10.1182/bloodadvances.2018020206
60. Roloff GW, Griffiths EA. When to obtain genomic data in acute myeloid leukemia (AML) and which mutations matter. Hematology Am Soc Hematol Educ Program. (2018) 2018:35–44. doi: 10.1182/asheducation-2018.1.35
61. Schlenk RF, Dohner K, Krauter J, Frohling S, Corbacioglu A, Bullinger L, et al. Mutations and treatment outcome in cytogenetically normal acute myeloid leukemia. N Engl J Med. (2008) 358:1909–18. doi: 10.1056/NEJMoa074306
62. Goasguen JE, Bennett JM, Bain BJ, Brunning R, Vallespi MT, Tomonaga M, et al. Dyserythropoiesis in the diagnosis of the myelodysplastic syndromes and other myeloid neoplasms: problem areas. Br J Haematol. (2018) 182:526–33. doi: 10.1111/bjh.15435
63. Zhang L, McGraw KL, Sallman DA, List AF. The role of p53 in myelodysplastic syndromes and acute myeloid leukemia: molecular aspects and clinical implications. Leuk Lymphoma. (2017) 58:1777–90. doi: 10.1080/10428194.2016.1266625
64. Churpek JE. Familial myelodysplastic syndrome/acute myeloid leukemia. Best Pract Res Clin Haematol. (2017) 30:287–9. doi: 10.1016/j.beha.2017.10.002
65. Bejar R. What biologic factors predict for transformation to AML? Best Pract Res Clin Haematol. (2018) 31:341–5. doi: 10.1016/j.beha.2018.10.002
66. Heuser M. Therapy-related myeloid neoplasms: does knowing the origin help to guide treatment? Hematology. Am Soc Hematol Educ Program. (2016) 2016:24–32. doi: 10.1182/asheducation-2016.1.24
67. Ganser A, Heuser M. Therapy-related myeloid neoplasms. Curr Opin Hematol. (2017) 24:152–8. doi: 10.1097/MOH.0000000000000316
68. Irimie AI, Braicu C, Sonea L, Zimta AA, Cojocneanu-Petric R, Tonchev K, et al. A looking-glass of non-coding RNAs in oral cancer. Int J Mol Sci. (2017) 18:E2620. doi: 10.3390/ijms18122620
69. Catana CS, Pichler M, Giannelli G, Mader RM, Berindan-Neagoe I. Non-coding RNAs, the Trojan horse in two-way communication between tumor and stroma in colorectal and hepatocellular carcinoma. Oncotarget. (2017) 8:29519–34. doi: 10.18632/oncotarget.15706
70. Braicu C, Catana C, Calin GA, Berindan-Neagoe I. NCRNA combined therapy as future treatment option for cancer. Curr Pharm Des. (2014) 20:6565–74. doi: 10.2174/1381612820666140826153529
71. Berindan-Neagoe I, Braicu C, Irimie A. Combining the chemotherapeutic effects of epigallocatechin 3-gallate with siRNA-mediated p53 knock-down results in synergic pro-apoptotic effects. Int J Nanomed. (2012) 7:6035–47. doi: 10.2147/IJN.S36523
72. Lajos R, Braicu C, Jurj A, Chira S, Cojocneanu-Petric R, Pileczki V, et al. A miRNAs profile evolution of triple negative breast cancer cells in the presence of a possible adjuvant therapy and senescence inducer. J BUON. (2018) 23:692–705.
73. Calin GA. Preface for GCC special issue on noncoding RNAs, noncoding DNAs, and genome editing. Genes Chromosomes Cancer. (2019) 58:189–90. doi: 10.1002/gcc.22710
74. Ultimo S, Martelli AM, Zauli G, Vitale M, Calin GA, Neri LM. Roles and clinical implications of microRNAs in acute lymphoblastic leukemia. J Cell Physiol. (2018) 233:5642–54. doi: 10.1002/jcp.26290
75. Redis RS, Calin GA. SnapShot: non-coding RNAs and metabolism. Cell Metab. (2017) 25:220.e1. doi: 10.1016/j.cmet.2016.12.012
76. Jurj A, Pop L, Petrushev B, Pasca S, Dima D, Frinc I, et al. Exosome-carried microRNA-based signature as a cellular trigger for the evolution of chronic lymphocytic leukemia into Richter syndrome. Crit Rev Clin Lab Sci. (2018) 55:501–15. doi: 10.1080/10408363.2018.1499707
77. Gulei D, Berindan-Neagoe I, Calin GA. MicroRNas: beating cancer with new powerful weapons. Mol Life. (2017) 1:50–6. doi: 10.26600/MolLife.1.1.6.2017
78. Seles M, Hutterer GC, Kiesslich T, Pummer K, Berindan-Neagoe I, Perakis S, et al. Current insights into long non-coding RNAs in renal cell carcinoma. Int J Mol Sci. (2016) 17:573. doi: 10.3390/ijms17040573
79. Ling H, Vincent K, Pichler M, Fodde R, Berindan-Neagoe I, Slack FJ, et al. Junk DNA and the long non-coding RNA twist in cancer genetics. Oncogene. (2015) 34:5003–11. doi: 10.1038/onc.2014.456
80. Böhmdorfer G, Wierzbicki AT. Control of chromatin structure by long noncoding RNA. Trends Cell Biol. (2015) 25:623–32. doi: 10.1016/j.tcb.2015.07.002
81. Kurokawa R. Promoter-associated long noncoding RNAs repress transcription through a RNA binding protein TLS. Adv Exp Med Biol. (2011) 722:196–208. doi: 10.1007/978-1-4614-0332-6_12
82. Paraskevopoulou MD, Hatzigeorgiou AG. Analyzing MiRNA-LncRNA interactions. Methods Mol Biol. 1402 (2016) 271–86. doi: 10.1007/978-1-4939-3378-5_21
83. Zeng C, Fukunaga T, Hamada M. Identification and analysis of ribosome-associated lncRNAs using ribosome profiling data. BMC Genom. (2018) 19:414. doi: 10.1186/s12864-018-4765-z
84. Zhang H, Liang Y, Han S, Peng C, Li Y. Long noncoding RNA and protein interactions: from experimental results to computational models based on network methods. Int J Mol Sci. (2019) 20:1284. doi: 10.3390/ijms20061284
85. Whitehouse I, Rando OJ, Delrow J, Tsukiyama T. Chromatin remodelling at promoters suppresses antisense transcription. Nature. (2007) 450:1031–5. doi: 10.1038/nature06391
86. Cairns BR. The logic of chromatin architecture and remodelling at promoters. Nature. (2009) 461:193–8. doi: 10.1038/nature08450
87. Murray SC, Mellor J. Using both strands: the fundamental nature of antisense transcription. Bioarchitecture. (2016) 6:12–21. doi: 10.1080/19490992.2015.1130779
88. Tian YJ, Wang YH, Xiao AJ, Li PL, Guo J, Wang TJ, et al. Long noncoding RNA SBF2-AS1 act as a ceRNA to modulate cell proliferation via binding with miR-188-5p in acute myeloid leukemia. Artif Cells Nanomed Biotechnol. (2019) 47:1730–37. doi: 10.1080/21691401.2019.1608221
89. Bill M, Papaioannou D, Karunasiri M, Kohlschmidt J, Pepe F, Walker CJ, et al. Expression and functional relevance of long non-coding RNAs in acute myeloid leukemia stem cells. Leukemia. (2019) 33:2169–82. doi: 10.1038/s41375-019-0429-5
90. Yang Y, Dai W, Sun Y, Zhao Z. Long noncoding RNA linc00239 promotes malignant behaviors and chemoresistance against doxorubicin partially via activation of the PI3K/Akt/mTOR pathway in acute myeloid leukaemia cells. Oncol Rep. (2019) 41:2311–20. doi: 10.3892/or.2019.6991
91. Zhang Y, Huang Z, Sheng F, Yin Z. MYC upregulated LINC00319 promotes human acute myeloid leukemia (AML) cells growth through stabilizing SIRT6. Biochem Biophys Res Commun. (2019) 509:314–21. doi: 10.1016/j.bbrc.2018.12.133
92. Ma L, Kuai WX, Sun XZ, Lu XC, Yuan YF. Long noncoding RNA LINC00265 predicts the prognosis of acute myeloid leukemia patients and functions as a promoter by activating PI3K-AKT pathway. Eur Rev Med Pharmacol Sci. (2018) 22:7867–76. doi: 10.26355/eurrev_201811_16412
93. Congrains-Castillo A, Niemann FS, Santos Duarte AS, Olalla-Saad ST. LEF1-AS1, long non-coding RNA, inhibits proliferation in myeloid malignancy. J Cell Mol Med. (2019) 23:3021–5. doi: 10.1111/jcmm.14152
94. Gan S, Ma P, Ma J, Wang W, Han H, Chen L, et al. Knockdown of ZFAS1 suppresses the progression of acute myeloid leukemia by regulating microRNA-150/Sp1 and microRNA-150/Myb pathways. Eur J Pharmacol. (2019) 844:38–48. doi: 10.1016/j.ejphar.2018.11.036
95. Yang Q, Jia L, Li X, Guo R, Huang Y, Zheng Y, et al. Long noncoding RNAs: new players in the osteogenic differentiation of bone marrow- and adipose-derived mesenchymal stem cells. Stem Cell Rev. (2018) 14:297–308. doi: 10.1007/s12015-018-9801-5
96. Zhang J, Tao Z, Wang Y. Long noncoding RNA DANCR regulates the proliferation and osteogenic differentiation of human bone-derived marrow mesenchymal stem cells via the p38 MAPK pathway. Int J Mol Med. (2018) 41:213–9. doi: 10.3892/ijmm.2017.3215
97. Guan X, Wen X, Xiao J, An X, Yu J, Guo Y. Lnc-SOX6-1 upregulation correlates with poor risk stratification and worse treatment outcomes, and promotes cell proliferation while inhibits apoptosis in pediatric acute myeloid leukemia. Int J Lab Hematol. (2019) 41:234–41. doi: 10.1111/ijlh.12952
98. Qi X, Jiao Y, Cheng C, Qian F, Chen Z, Wu Q. H22954, a novel long non-coding RNA down-regulated in AML, inhibits cancer growth in a BCL-2-dependent mechanism. Cancer Lett. (2019) 454:26–36. doi: 10.1016/j.canlet.2019.03.055
99. Zhang X, Tao W. Long noncoding RNA LINC00152 facilitates the leukemogenesis of acute myeloid leukemia by promoting CDK9 through miR-193a. DNA Cell Biol. (2019) 38:236–42. doi: 10.1089/dna.2018.4482
100. Zhuang MF, Li LJ, Ma JB. LncRNA HOTTIP promotes proliferation and cell cycle progression of acute myeloid leukemia cells. Eur Rev Med Pharmacol Sci. (2019) 23:2908–15. doi: 10.26355/eurrev_201904_17569
101. Rahimi N, Zhang Y, Mina A, Altman JK, Sukhanova M, Frankfurt O, et al. An integrative approach reveals genetic complexity and epigenetic perturbation in acute promyelocytic leukemia: a single institution experience. Hum Pathol. (2019) 91:1–10. doi: 10.1016/j.humpath.2019.05.008
102. Ravandi F, Stone R. Acute promyelocytic leukemia: a perspective. Clin Lymphoma Myeloma Leuk. (2017) 17:543–4. doi: 10.1016/j.clml.2017.06.037
103. Jillella AP, Kota VK. The global problem of early deaths in acute promyelocytic leukemia: a strategy to decrease induction mortality in the most curable leukemia. Blood Rev. (2018) 32:89–95. doi: 10.1016/j.blre.2017.09.001
104. Tsai CH, Yao CY, Tien FM, Tang JL, Kuo YY, Chiu YC, et al. Incorporation of long non-coding RNA expression profile in the 2017 ELN risk classification can improve prognostic prediction of acute myeloid leukemia patients. EBioMedicine. (2019) 40:240–50. doi: 10.1016/j.ebiom.2019.01.022
105. Qin J, Bao H, Li H. Correlation of long non-coding RNA taurine-upregulated gene 1 with disease conditions and prognosis, as well as its effect on cell activities in acute myeloid leukemia. Cancer Biomarkers. (2018) 23:569–77. doi: 10.3233/CBM-181834
106. Wang X, Zhang L, Zhao F, Xu R, Jiang J, Zhang C, et al. Long non-coding RNA taurine-upregulated gene 1 correlates with poor prognosis, induces cell proliferation, and represses cell apoptosis via targeting aurora kinase A in adult acute myeloid leukemia. Ann Hematol. (2018) 97:1375–89. doi: 10.1007/s00277-018-3315-8
107. Li J, Sun CK. Long noncoding RNA SNHG5 is up-regulated and serves as a potential prognostic biomarker in acute myeloid leukemia. Eur Rev Med Pharmacol Sci. (2018) 22:3342–7. doi: 10.26355/eurrev_201806_15154
108. Wang F, Tian X, Zhou J, Wang G, Yu W, Li Z, et al. A threelncRNA signature for prognosis prediction of acute myeloid leukemia in patients. Mol Med Rep. (2018) 18:1473–84. doi: 10.3892/mmr.2018.9139
109. Pashaiefar H, Izadifard M, Yaghmaie M, Montazeri M, Gheisari E, Ahmadvand M, et al. Low expression of long noncoding RNA IRAIN is associated with poor prognosis in non-M3 acute myeloid leukemia patients. Genet Test Mol Biomarkers. (2018) 22:288–94. doi: 10.1089/gtmb.2017.0281
110. Beck D, Thoms JAI, Palu C, Herold T, Shah A, Olivier J, et al. A four-gene LincRNA expression signature predicts risk in multiple cohorts of acute myeloid leukemia patients. Leukemia. (2018) 32:263–72. doi: 10.1038/leu.2017.210
111. Sun MD, Zheng YQ, Wang LP, Zhao HT, Yang S. Long noncoding RNA UCA1 promotes cell proliferation, migration and invasion of human leukemia cells via sponging miR-126. Eur Rev Med Pharmacol Sci. (2018) 22:2233–45. doi: 10.26355/eurrev_201804_14809
112. Zhang Y, Liu Y, Xu X. Knockdown of LncRNA-UCA1 suppresses chemoresistance of pediatric AML by inhibiting glycolysis through the microRNA-125a/hexokinase 2 pathway. J Cell Biochem. (2018) 119:6296–308. doi: 10.1002/jcb.26899
113. Zhang TJ, Zhou JD, Zhang W, Lin J, Ma JC, Wen XM, et al. H19 overexpression promotes leukemogenesis and predicts unfavorable prognosis in acute myeloid leukemia. Clin Epigenet. (2018) 10:47. doi: 10.1186/s13148-018-0486-z
114. Chen S, Nagel S, Schneider B, Dai H, Geffers R, Kaufmann M, et al. A new ETV6-NTRK3 cell line model reveals MALAT1 as a novel therapeutic target - a short report. Cell Oncol. (2018) 41:93–101. doi: 10.1007/s13402-017-0356-2
115. Wu S, Zheng C, Chen S, Cai X, Shi Y, Lin B, et al. Overexpression of long non-coding RNA HOTAIR predicts a poor prognosis in patients with acute myeloid leukemia. Oncol Lett. (2015) 10:2410–4. doi: 10.3892/ol.2015.3552
116. Hao S, Shao Z. HOTAIR is upregulated in acute myeloid leukemia and that indicates a poor prognosis. Int J Clin Exp Pathol. (2015) 8:7223–8.
117. Xing CY, Hu XQ, Xie FY, Yu ZJ, Li HY, Bin Z, et al. Long non-coding RNA HOTAIR modulates c-KIT expression through sponging miR-193a in acute myeloid leukemia. FEBS Lett. (2015) 589:1981–7. doi: 10.1016/j.febslet.2015.04.061
118. Guo H, Wu L, Zhao P, Feng A. Overexpression of long non-coding RNA zinc finger antisense 1 in acute myeloid leukemia cell lines influences cell growth and apoptosis. Exp Ther Med. (2017) 14:647–51. doi: 10.3892/etm.2017.4535
119. Hirano T, Yoshikawa R, Harada H, Harada Y, Ishida A, Yamazaki T. Long noncoding RNA, CCDC26, controls myeloid leukemia cell growth through regulation of KIT expression. Mol Cancer. (2015) 14:90. doi: 10.1186/s12943-015-0364-7
120. Sayad A, Hajifathali A, Hamidieh AA, Esfandi F, Taheri M. Fas-antisense long noncoding RNA and acute myeloid leukemia: is there any relation? Asian Pac J Cancer Prev. (2018) 19:45–48. doi: 10.22034/APJCP.2018.19.1.45
121. Sayad A, Hajifathali A, Hamidieh AA, Roshandel E, Taheri M. HOTAIR long noncoding RNA is not a biomarker for acute myeloid leukemia (AML) in Iranian patients. Asian Pac J Cancer Prev. (2017) 18:1581–4. doi: 10.22034/APJCP.2017.18.6.1581
122. Zhang J, Griffith M, Miller CA, Griffith OL, Spencer DH, Walker JR, et al. Comprehensive discovery of noncoding RNAs in acute myeloid leukemia cell transcriptomes. Exp Hematol. (2017) 55:19–33. doi: 10.1016/j.exphem.2017.07.008
123. Yao H, Sun P, Duan M, Lin L, Pan Y, Wu C, et al. microRNA-22 can regulate expression of the long non-coding RNA MEG3 in acute myeloid leukemia. Oncotarget. (2017) 8:65211–7. doi: 10.18632/oncotarget.18059
124. Yin X, Huang S, Zhu R, Fan F, Sun C, Hu Y. Identification of long non-coding RNA competing interactions and biological pathways associated with prognosis in pediatric and adolescent cytogenetically normal acute myeloid leukemia. Cancer Cell Int. (2018) 18:122. doi: 10.1186/s12935-018-0621-0
125. Wang Y, Zhou Q, Ma JJ. High expression of lnc-CRNDE presents as a biomarker for acute myeloid leukemia and promotes the malignant progression in acute myeloid leukemia cell line U937. Eur Rev Med Pharmacol Sci. (2018) 22:763–70. doi: 10.26355/eurrev_201802_14310
126. Torre D, Lachmann A, Ma'ayan A. BioJupies: automated generation of interactive notebooks for RNA-Seq data analysis in the cloud. Cell Syst. (2018) 7:556–61.e3. doi: 10.1016/j.cels.2018.10.007
127. Dong X, Fang Z, Yu M, Zhang L, Xiao R, Li X, et al. Knockdown of long noncoding RNA HOXA-AS2 suppresses chemoresistance of acute myeloid leukemia via the miR-520c-3p/S100A4 axis. Cell Physiol Biochem. (2018) 51:886–96. doi: 10.1159/000495387
128. Liu B, Ma X, Liu Q, Xiao Y, Pan S, Jia L. Aberrant mannosylation profile and FTX/miR-342/ALG3-axis contribute to development of drug resistance in acute myeloid leukemia. Cell Death Dis. (2018) 9:688. doi: 10.1038/s41419-018-0706-7
129. Redis RS, Vela LE, Lu W, Ferreira de Oliveira J, Ivan C, Rodriguez-Aguayo C, et al. Allele-specific reprogramming of cancer metabolism by the long non-coding RNA CCAT2. Mol Cell. (2016) 61:520–34. doi: 10.1016/j.molcel.2016.01.015
130. Fosselteder J, Calin GA, Pichler M. Long non-coding RNA CCAT2 as a therapeutic target in colorectal cancer. Expert Opin Ther Targets. (2018) 22:973–6. doi: 10.1080/14728222.2018.1541453
131. Ling H, Spizzo R, Atlasi Y, Nicoloso M, Shimizu M, Redis RS, et al. CCAT2, a novel noncoding RNA mapping to 8q24, underlies metastatic progression and chromosomal instability in colon cancer. Genome Res. (2013) 23:1446–61. doi: 10.1101/gr.152942.112
132. Redis RS, Sieuwerts AM, Look MP, Tudoran O, Ivan C, Spizzo R, et al. CCAT2, a novel long non-coding RNA in breast cancer: expression study and clinical correlations. Oncotarget. (2013) 4:1748–62. doi: 10.18632/oncotarget.1292
133. Shen P, Pichler M, Chen M, Calin GA, Ling H. To Wnt or lose: the missing non-coding linc in colorectal cancer. Int J Mol Sci. (2017) 18:2003. doi: 10.3390/ijms18092003
134. Ozawa T, Matsuyama T, Toiyama Y, Takahashi N, Ishikawa T, Uetake H, et al. CCAT1 and CCAT2 long noncoding RNAs, located within the 8q.24.21 ‘gene desert’, serve as important prognostic biomarkers in colorectal cancer. Ann Oncol. (2017) 28:1882–8. doi: 10.1093/annonc/mdx248
135. White NM, Maher CA. The potential use of lncRNAs found in the 8q24 region as biomarkers for colon cancer. Ann Oncol. (2017) 28:1688–9. doi: 10.1093/annonc/mdx337
136. Liu Y, Wang D, Li Y, Yan S, Dang H, Yue H, et al. Long noncoding RNA CCAT2 promotes hepatocellular carcinoma proliferation and metastasis through up-regulation of NDRG1. Exp Cell Res. (2019) 379:19–29. doi: 10.1016/j.yexcr.2019.03.029
137. Cai Y, Li X, Shen P, Zhang D. CCAT2 is an oncogenic long non-coding RNA in pancreatic ductal adenocarcinoma. Biol Res. (2018) 51:1. doi: 10.1186/s40659-017-0149-0
138. Huang JL, Liao Y, Qiu MX, Li J, An Y. Long non-coding RNA CCAT2 promotes cell proliferation and invasion through regulating Wnt/beta-catenin signaling pathway in clear cell renal cell carcinoma. Tumour Biol. (2017) 39:1010428317711314. doi: 10.1177/1010428317711314
139. Lu D, Thum T. RNA-based diagnostic and therapeutic strategies for cardiovascular disease. Nat Rev Cardiol. (2019). doi: 10.1038/s41569-019-0218-x. [Epub ahead of print].
140. Tang W, Tu S, Lee HC, Weng Z, Mello CC. The RNase PARN-1 Trims piRNA 3' ends to promote transcriptome surveillance in C. elegans. Cell. (2016) 164:974–84. doi: 10.1016/j.cell.2016.02.008
141. Fabbri M, Girnita L, Varani G, Calin GA. Decrypting noncoding RNA interactions, structures, and functional networks. Genome Res. (2019) 29:1377–88. doi: 10.1101/gr.247239.118
142. Gulei D, Raduly L, Berindan-Neagoe I, Calin GA. CRISPR-based RNA editing: diagnostic applications and therapeutic options. Expert Rev Mol Diagn. (2019) 19:83–8. doi: 10.1080/14737159.2019.1568242
143. Calin GA. The noncoding RNA revolution-three decades and still going strong! Mol Oncol. (2019) 13:3. doi: 10.1002/1878-0261.12418
144. Grewal R, Cucuianu A, Swanepoel C, Dima D, Petrushev B, Pop B, et al. The role of microRNAs in the pathogenesis of HIV-related lymphomas. Crit Rev Clin Lab Sci. (2015) 52:232–41. doi: 10.3109/10408363.2015.1030063
145. Tomuleasa C, Fuji S, Cucuianu A, Kapp M, Pileczki V, Petrushev B, et al. MicroRNAs as biomarkers for graft-versus-host disease following allogeneic stem cell transplantation. Ann Hematol. (2015) 94:1081–92. doi: 10.1007/s00277-015-2369-0
146. Sachani SS, Landschoot LS, Zhang L, White CR, MacDonald WA, Golding MC, et al. Nucleoporin 107, 62 and 153 mediate Kcnq1ot1 imprinted domain regulation in extraembryonic endoderm stem cells. Nat Commun. (2018) 9:2795. doi: 10.1038/s41467-018-05208-2
147. Cheng P, Lu P, Guan J, Zhou Y, Zou L, Yi X, et al. LncRNA KCNQ1OT1 controls cell proliferation, differentiation and apoptosis by sponging miR-326 to regulate c-Myc expression in acute myeloid leukemia. Neoplasma. (2019). doi: 10.4149/neo_2018_181215N972. [Epub ahead of print].
148. Jiang Y, Du W, Chu Q, Qin Y, Tuguzbaeva G, Wang H, et al. Downregulation of long non-coding RNA Kcnq1ot1: an important mechanism of arsenic trioxide-induced long QT syndrome. Cell Physiol Biochem. (2018) 45:192–202. doi: 10.1159/000486357
149. Xu J, Zhang J. LncRNA TP73-AS1 is a novel regulator in cervical cancer via miR-329-3p/ARF1 axis. J Cell Biochem. (2019). doi: 10.1002/jcb.29181. [Epub ahead of print].
150. Zhu D, Zhou J, Liu Y, Du L, Zheng Z, Qian X. LncRNA TP73-AS1 is upregulated in non-small cell lung cancer and predicts poor survival. Gene. (2019) 710:98–102. doi: 10.1016/j.gene.2019.05.044
151. Isin M, Ozgur E, Cetin G, Erten N, Aktan M, Gezer U, et al. Investigation of circulating lncRNAs in B-cell neoplasms. Clin Chim Acta. (2014) 431:255–9. doi: 10.1016/j.cca.2014.02.010
152. Gasic V, Stankovic B, Zukic B, Janic D, Dokmanovic L, Krstovski N, et al. Expression pattern of long non-coding RNA growth arrest-specific 5 in the remission induction therapy in childhood acute lymphoblastic leukemia. J Med Biochem. (2019) 38:292–8. doi: 10.2478/jomb-2018-0038
153. Sun LY, Li XJ, Sun YM, Huang W, Fang K, Han C, et al. LncRNA ANRIL regulates AML development through modulating the glucose metabolism pathway of AdipoR1/AMPK/SIRT1. Mol Cancer. (2018) 17:127. doi: 10.1186/s12943-018-0879-9
154. Wang SL, Huang Y, Su R, Yu YY. Silencing long non-coding RNA HOTAIR exerts anti-oncogenic effect on human acute myeloid leukemia via demethylation of HOXA5 by inhibiting Dnmt3b. Cancer Cell Int. (2019) 19:114. doi: 10.1186/s12935-019-0808-z
Keywords: myeloid malignancies, non-coding RNAs, diagnostic tool, prognostic tools, clinical impact
Citation: Zimta A-A, Tomuleasa C, Sahnoune I, Calin GA and Berindan-Neagoe I (2019) Long Non-coding RNAs in Myeloid Malignancies. Front. Oncol. 9:1048. doi: 10.3389/fonc.2019.01048
Received: 15 July 2019; Accepted: 26 September 2019;
Published: 18 October 2019.
Edited by:
Abhinav Dhall, Harvard Medical School, United StatesReviewed by:
Ranganatha R. Somasagara, North Carolina Central University, United StatesAlice Mims, The Ohio State University, United States
Copyright © 2019 Zimta, Tomuleasa, Sahnoune, Calin and Berindan-Neagoe. This is an open-access article distributed under the terms of the Creative Commons Attribution License (CC BY). The use, distribution or reproduction in other forums is permitted, provided the original author(s) and the copyright owner(s) are credited and that the original publication in this journal is cited, in accordance with accepted academic practice. No use, distribution or reproduction is permitted which does not comply with these terms.
*Correspondence: Ciprian Tomuleasa, ciprian.tomuleasa@umfcluj.ro; Ioana Berindan-Neagoe, ioana.neagoe@umfcluj.ro