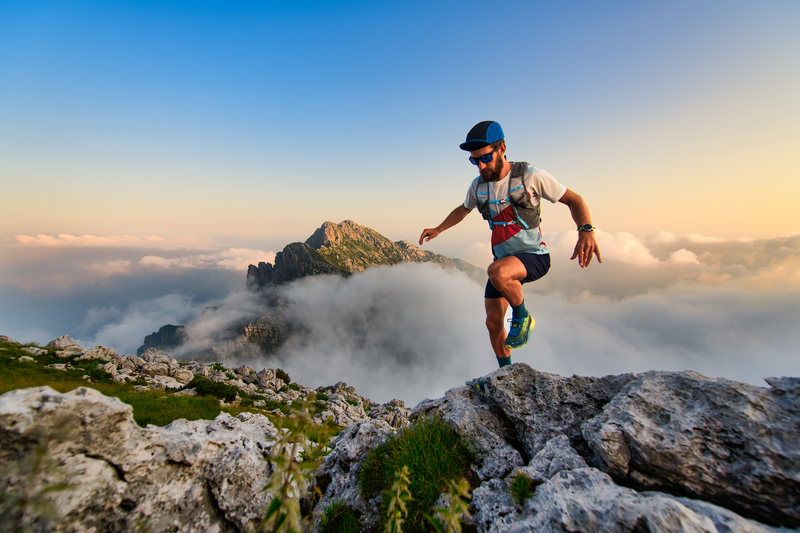
95% of researchers rate our articles as excellent or good
Learn more about the work of our research integrity team to safeguard the quality of each article we publish.
Find out more
ORIGINAL RESEARCH article
Front. Oncol. , 04 October 2019
Sec. Cancer Molecular Targets and Therapeutics
Volume 9 - 2019 | https://doi.org/10.3389/fonc.2019.00956
Mining cancer-omics databases deepens our understanding of cancer biology and can lead to potential breakthroughs in cancer treatment. Here, we propose an integrative analytical approach to reveal across-cancer expression patterns and identify potential clinical impacts for genes of interest from five representative public databases. Using ribonucleotide reductase (RR), a key enzyme in DNA synthesis and cancer-therapeutic targeting, as an example, we characterized the mRNA expression profiles and inter-component associations of three RR subunit genes and assess their differing pathological and prognostic significance across over 30-types of cancers and their related subtypes. Findings were validated by immunohistochemistry with clinical tissue samples (n = 211) collected from multiple cancer centers in China and with clinical follow-up. Underlying mechanisms were further explored and discussed using co-expression gene network analyses. This framework represents a simple, efficient, accurate, and comprehensive approach for cancer-omics resource analysis and underlines the necessity to separate the tumors by their histological or pathological subtypes during the clinical evaluation of molecular biomarkers.
To date, an immense and increasing amount of cancer-omics data and associated clinical annotation has been produced and has become publicly available from diverse repositories. Such cancer-omics data resources include the Catalog of Somatic Mutations in Cancer (COSMIC) (1), the Cancer Genome Atlas (TCGA) (2), the International Cancer Genome Consortium (ICGC) (3), and the Cancer Proteome Atlas (TCPA) (4). In such efforts, researchers have collected and annotated enormous amounts of heterogeneous cancer genomic data and have also created some powerful data-mining tools such as Oncomine (5), cBio Cancer Genomics Portal (cBioPortal) (6), Kaplan-Meier plotter (KM-plotter) (7), and the Human Protein Atlas (HPA) (8). Although these resources have provided an unprecedent opportunity to deepen our understanding of cancer development and progression, the challenge continues to press on toward increasingly efficient and more effective data mining strategies that aim at the identification of key molecular targets for improving cancer treatment.
Ribonucleotide reductase (RR) has been identified as an important anticancer target and its inhibitors, alone or combined with other anticancer drugs, have been successfully used to control multiple solid and hematological malignancies (9–12). However, three genes coding for RR proteins are located in three different chromosomes and their expressions are both varied and diverse in different types of cancers and their histologic variants (9, 10). The whole-genome expressional landscape of different RR subunit genes during cancer development, as well as their underlying molecular regulatory mechanisms and potential clinical applications, still requires considerable clarification. This makes RR an ideal candidate example to use for demonstrating a more integrative analytical approach for the mining of cancer-omics databases.
In this study, we began by analyzing multiple publicly available cancer-omics databases for the expression profiles of a group of RR-related genes and their correlations with patient survival. Findings were further validated using clinical samples from cohorts of lung cancer patients. With this approach, we revealed the expressional patterns of three RR subunit genes and their associations with each other in common types of cancers in Oncomine and TCGA datasets. The differential expression and prognostic significance of the two types of RR (RRM1-RRM2 and RRM1-RRM2B) were further validated in 211 cases of lung squamous cell carcinoma (LUSC) and lung adenocarcinoma (LUAD) patients. Finally, we performed a gene co-expression network analysis to further define possible roles and explore the underlying mechanisms of the RRM2 and RRM2B genes in LUAD and LUSC tumors. Our study provides novel insight in understanding molecular mechanisms of RR in cancer development, which may promote precision RR-targeting for cancer treatment and provides a valuable data-mining approach that could be applied to any gene of interest.
Normalized microarray data used for analysis of RRM1, RRM2, and RRM2B mRNA expressions across 20 types of common human cancers were downloaded from the Oncomine database (5). Expectation-Maximization (RSEM) normalized RNA-sequencing (RNA-seq) data and clinicopathologic data of 31 types of common cancers, including BLCA (n = 408), BRCA (n = 1,093), CESC (n = 304), COAD (n = 285), COADREAD (n = 379), ESCA (n = 184), GBM (n = 153), GBMLGG (n = 669), HNSC (n = 520), KICH (n = 66), KIPAN (n = 889), KIRC (n = 533), KIRP (n = 290), LAML (n = 173), LGG (n = 516), LIHC (n = 371), LUAD (n = 515), LUSC (n = 501), OV (n = 303), PAAD (n = 178), PCPG (n = 179), PRAD (n = 497), READ (n = 94), SARC (n = 259), SKCM (n = 470), STAD (n = 415), STES (n = 599), TGCT (n = 150), THCA (n = 501), THYM (n = 120), and UCEC (n = 176) were downloaded from TCGA via Firehose. TCGA, Oncomine, and KM Plotter datasets were used for pathological survival analyses of LUSC and LUAD.
A total of 211 surgically-excised tumor tissue samples from LUSC and LUAD patients (n = 97 and 114, respectively) were collected between July 2011 and October 2013 in three hospitals in Zhejiang, China, including the First and Second Affiliated Hospitals of Zhejiang University, and Zhejiang Cancer Hospital, and the cohort was named as ZJUC cohort in this study. Prior to the study, all patients gave their written informed consent to allow the tissue samples and clinical information to be used for scientific research. The inclusion criteria were defined as follows: (i) histologically diagnosed as primary LUSC or LUAD; (ii) underwent surgical resection as a primary treatment; (iii) full information available including clinicopathologic characteristics and follow-up information. Patients were excluded if they had incomplete or missing data regarding the American Joint Committee on Cancer (AJCC) staging, survival state, cause of death and survival time. The disease stages were classified based on the 7th edition of AJCC staging manual. This study was approved by the Ethics Committee of each participating hospitals.
The 211 tissue samples were formalin-fixed and paraffin-embedded. The immunohistochemistry was conducted using an Envision Detection System (DAKO, Denmark) according to the manufacturer's instructions as described previously (13). We used the following commercial antibodies against RRM1 (10526-1-AP, Proteintech, 1:500), RRM2 (ab57653, Abcam, 1:200), and RRM2B (ab8105, Abcam, 1:500) for immunohistochemistry. PBS was used as a negative control.
To determine the score of each slide, at least eight individual fields at 200× were selected, and 100 cancer cells were counted in each field. Cells with cytoplasmic and/or nuclear immunoreactivity of RRM1, RRM2, and RRM2B were considered positive. The immunostaining intensity was divided into five grades: 0, negative; 1, weak; 2, moderate; 3, strong; and 4, very strong. The proportion of positive-staining cells was also divided into five grades: 0, <5; 1, 6–25; 2, 26–50; 3, 51–75; and 4, >75%. The IHC scores were generated by multiplying the intensity score and the proportion score. To avoid observer bias, and for consistency, the value of immunostaining intensity and the percentage of positive-staining cells for all the slides were evaluated independently by two different well-trained observers blinded to the clinical data.
Based on RNA-seq data derived from TCGA, we estimated the correlations of gene sets tightly associated with RR subunit genes in different lung cancer types using Pearson's correlation coefficient. Gene-annotation enrichment analysis was next conducted using the DAVID bioinformatics resources version 6.8. Cytoscape 3.0 was used to visualize the topological molecular network structures that were composed of genes highly correlated to RRM2B with their Gene Ontology (GO) terms having p < 0.05 (14).
The definitions of overall survival (OS) and disease-free survival (DFS) followed recommended criteria (15). OS was defined as the interval between first pathological confirmation and death or the last date of follow-up. DFS was defined as the time from random assignment to recurrence, second primary cancer, or death with evidence of disease progression. Within a specific cohort, patients were divided into two groups by the median value of each gene in all samples. Associations with OS were examined using univariate Cox proportional hazard regression models. Survival curves were constructed using the Kaplan–Meier method and compared using the log-rank test. The correlation coefficients across individual gene expressions were calculated using Pearson's correlation coefficient in R 3.1.2 software package. Student t tests were used to compare continuous data. The One-way ANOVA was used to compare means of two or more samples, with the Least Significant Difference (LSD) test used for pair-wise comparisons of different groups. The Kaplan-Meier plot was drawn using GraphPad Software (version 6.0, USA). All tests were two tailed, retaining P < 0.05. Statistical analysis was performed using SAS v9.4 (SAS Institute, Inc., Cary, NC, USA).
We propose a stepwise analytical workflow to investigate the expression patterns and clinical relevance of virtually any genes of interest related to the malignancies of human cancers (Figure 1). This firstly involves collection and integration of genomics, transcriptomics, proteomics, and clinical information from multiple publicly available cancer-omics databases, including COSMIC, TCGA, Oncomine, HPA, and Kaplan-Meier plotter. This data is then validated by routine immunochemical examination of clinical cancer samples collected from multiple archived tissue banks. Finally, the validated results are further explored for their biological relevance, underlying pathological mechanisms, and potential application in diagnosis, treatment, and prevention of cancers. In this manuscript, a multi-subunit enzyme, human RR, was used as an example to demonstrate the use, application and efficiency of this method.
Figure 1. Workflow of the cancer-omics data based integratively analytic strategy. dbs, databases; N/C, Normal vs. Cancerous tissues; TNM, tumor, node, and metastasis classification; OS, Overall survival; DFS, Disease-free survival.
To obtain mRNA expression profiles of three RR subunit genes in the 20 malignancy types, including both solid and blood malignancies, we systematically analyzed all the relevant studies (studies 358, 355 and 209 for RRM1, RRM2, and RRM2B, respectively) collected from Oncomine version 4.5, with the search completed on 15 Mar 2019 (Figure 2). We used following parameters as the filtering criteria to identify differentially expressed genes (DEGs) between cancer and normal tissues: concept filter = cancers vs. normal; data type = mRNA, p < 0.05, and gene rank being ≤10% (in which the genes are ranked by their p-value and a gene rank ≤10% represents those genes with their p-value listed in the top 10% of the up- or down-regulated genes). We found that the mRNA expression levels of RRM1, RRM2, and RRM2B ranked in the top 10% of the up-regulated DEGs in 24.0% (86 of 358), 38.3% (136 of 355), and 10.5% (22 of 209) of the identified cancer studies, respectively. In contrast, RRM1, RRM2, and RRM2B were ranked in the top 10% of the down-regulated DEGs in only 8.4% (30 of 358), 4.5% (16 of 355), and 4.8% (10 of 209) of the cancer studies, respectively. Figure 2A represents the global distribution of RRM1, RRM2, and RRM2B gene expressions across the different types of Oncomine cancer studies. Figure 2B presents the fold changes (FC, cancer vs. normal tissue) of mRNA expression of RRM1, RRM2, and RRM2B genes in each different type of the analyzed cancers. Obviously, RRM2 gene expression levels were higher than that of other two subunits in almost all the studied cancer types, especially in brain and Central Nervous System (CNS) cancers, lymphomas, lung cancer, bladder cancer, head and neck cancer, breast cancer, colorectal cancer, and sarcomas.
Figure 2. RRM1, RRM2, and RRM2B gene expression profiles in 20 common types of cancers. (A) mRNA expression data of RRM1, RRM2, and RRM2B genes in 20 common human cancers across all studies retrieved from Oncomine (version 4.5, search completed on Mar, 2019). DEGs were determined with the screening criteria “concept filter = cancers vs. normal, data type = mRNA, P < 0.05.” The x-axis indicated the proportion of studies with differentially expressed RRM1, RRM2, and RRM2B in all studies according the criteria. Dark red, red, and brick red colors marked the cancers in which the gene of interest had a gene rank of the top 1, 5, or 10% in the elevated-expression of the DEGs, respectively (Gene rank: genes are ranked by the p-value, for example, a gene rank ≤ 10% represents that its p-value lists in top 10% of the up-regulated or down-regulated ones). In contrast, dark blue, blue, and light blue colors marked the cancers in which the interested gene had a p-value rank of the top 1, 5, and 10% in the decreased-expression of the DEGs, respectively. (B) The visualization of fold change (FC) for RRM1, RRM2, and RRM2B mRNA expressions in the studies screened out (Details shown in Supplemental File 1). The FC values of overexpressed genes were calculated from tumor-/normal-tissues expression levels, while those for under-expressed genes were calculated from normal-/tumor-tissue expression levels. The human body parts diagram was adapted and modified from Robinson et al. (16).
To further study associations of RRM1, RRM2, and RRM2B expression in different cancer types and TNM stages, we downloaded and analyzed the RNA-seq and clinicopathologic data of 31 types of cancer from TCGA. Using the Spearman's correlation coefficient analysis, the consistent positive correlations were observed between RRM1 and RRM2 expression in all 31 types of cancer (Figure 3A). This consistency remained after subdividing patients into different TNM stages across almost all types of cancer (Figure 3B). In contrast, the associations between the expression of RRM1 and RRM2B genes were relatively weak and variable.
Figure 3. Associations among RRM1, RRM2, and RRM2B mRNA expressions in 31 types of human cancer. All data were retrieved from TCGA RNA-seq datasets via Firehose and analyzed using Spearman's correlation coefficient. Each cell of the figure contains the correlation coefficient (upper line), p-value and sample size (lower line). The cells were color-coded, red for positive correlations and green for negative correlations. Three different color bars at the top of each column of cells represented the association between RRM1 and RRM2 (yellow), RRM1, and RRM2B (purple), and RRM2 and RRM2B (blue), respectively. (A) The overall mRNA expression relevance of the three RR subunits in 31 cancer types. (B) mRNA expression relevance of the three RR subunits in different TNM stages. NA, represents that the number of cases is <5; Brackets represent that the corresponding information about the TNM stages is not available.
To further characterize the expression pattern of RRM1, RRM2, and RRM2B in different pathological types and stages of cancer, we chosen lung cancer as a representative cancer type as it is the leading cause of cancer death all over the world. We performed differentially expressed genes (DEGs) analyses with the above filtering criteria using the Oncomine data. We found that the mRNA expression of RRM1, RRM2, and RRM2B genes were up-regulated in 57.9% (11 of 19), 78.9% (15 of 19), and 0% (0 of 9), respectively, of the Oncomine cancer studies covering the four subtypes of LUSC, LUAD, large cell lung carcinoma (LCLC), and small cell lung carcinoma (SCLC) (Figure 4A). In contrast, no study showed down-regulated expression of RRM1 (0 of 19) or RRM2 (0 of 19) in all subtypes of lung cancer, 22.2% (2 in 9) of the Oncomine studies demonstrated a down-regulated RRM2B expression in cases of LUSC and LCLC. Figure 4B presented the mRNA expression FC values of RRM1, RRM2, and RRM2B genes in different subtypes of lung cancer, which appeared quite variable across different types of lung cancer and even varied in the same type of tumor from different studies.
Figure 4. Expression changes of RRM1, RRM2, and RRM2B in different pathological types and different TNM stages of lung cancer. (A) mRNA expression data of RRM1, RRM2, and RRM2B in different pathological types of lung cancers across all studies were retrieved from Oncomine. DEGs were determined according to the above screening criteria. The x-axis indicated the proportion of studies with differentially expressed RRM1, RRM2, and RRM2B in all lung cancer studies. Dark red, red, and brick red colors mark the cancers in which the gene of interest had a gene rank of top 1, 5, or 10% among the elevated expression genes, respectively (Gene rank: genes are ranked by the p-value, for example, a gene rank ≤ 10% represents that its P-value lists in top 10% of the up-regulated or down-regulated genes). In contrast, dark blue, blue, and light blue colors mark the cancers in which the gene of interest had a gene rank of top 1, 5, and 10% among the decreased-expressed genes, respectively. (B) The visualization of fold change (FC) values for RRM1, RRM2, and RRM2B mRNA expressions in the studies of different pathological types of lung cancer. The number in each cell represents a FC value from one study. The definition of cell colors is the same as that in (A). The FC value of an overexpressed gene was calculated from tumor-/normal-tissue expression levels; and the under-expressed gene was calculated from normal-/tumor-tissue expression levels. (C) The mRNA expression of RRM1, RRM2, and RRM2B in the different TNM stages of LUSC and LUAD. The RNA-seq and clinicopathological information of LUSC and LUAD patients were downloaded from TCGA through cBioPortal. Five groups of data (Para-cancerous tissues, TNM I, II, IIIA, and IIIB/IV cancer tissues) were analyzed using one-way ANOVA with LSD test for pair-wise comparisons of different groups. ***P < 0.05; ns, not significant.
We then analyzed the mRNA expression of three RR subunit genes in different progression stages of LUSC and LUAD, the two main pathological subtypes of NSCLC, using the RNA-seq data retrieved from TCGA. The expression of RRM1 and RRM2 genes in tumor tissues were both significantly increased in all TNM stages of LUSC and LUAD, compared with their corresponding adjacent normal lung tissue samples. In contrast, the RRM2B gene showed a significantly lower expression in the cancer tissues in all the TNM stages (Figure 4C). RRM2 expression was significantly higher in stages II and IIIA of LUSC and LUAD as well as in stages IIIB/IV of LUAD compared with its expression in stage I tumors (p < 0.05). In addition, RRM1 expression was also significantly higher in stages IIIB/IV LUAD than in stage I tumors (p < 0.05). Interestingly, RRM2B expression was significantly higher in stages IIIB/IV LUSC than in the stages I, II and IIIA tumors (p < 0.05), but was significantly lower in stages IIIB/IV LUAD than in stage I tumors (p < 0.05).
To determine the prognostic values of three RR subunit expression in LUAD and LUSC patients, we analyzed the cancer survival data from TCGA and KM-plotter databases. In the TCGA database, higher RRM1 and RRM2 expression predicted a significantly shorter OS (p = 0.007 and 0.005 for RRM1 and RRM2, respectively, Figure 5A) and DFS (p = 0.009 and 0.011 for RRM1 and RRM2, respectively, Figure 5C) in the LUAD patients, compared with those with lower RRM1 and RRM2 expression. This correlation was not observed in the LUSC patients. Similarly, in the KM-plotter database, higher RRM1 and RRM2 expressions were also significantly associated with a shorter OS (P = 3.3e−10 and 0.006 for RRM1 and RRM2, respectively, Figure 5B) and DFS (P = 1.1e−03 and 2.6e−06 for RRM1 and RRM2, respectively, Figure 5D) in the LUAD patients, but not in the LUSC patients. The poor prognosis of high RRM1 and RRM2 expressions in LUAD patients was also observed in two additional independent studies (9, 10) from Oncomine (Figure S1).
Figure 5. Survival curves for the patients of LUSC and LUAD against the expression levels of RRM1, RRM2, and RRM2B in TCGA and KM Plotter cohorts by using KM survival plotter analysis. The overall survival (OS) and disease free survival (DFS) against RRM1, RRM2, and RRM2B mRNA expressions in TCGA (A,C) and Kaplan-Meier Plotter (B,D) databases are shown, respectively. Vertical hash marks indicate points of censored data. For comparisons, the patients are dichotomized into two groups according to their median expression values.
Interestingly, we observed that a higher RRM2B expression was associated with a significantly better OS (P = 8.2e−12) and DFS (P = 9.3e−04) of the LUAD patients in the KM-plotter cohort (Figures 5B,D). In contrast, a higher RRM2B expression predicted a shorter OS (P = 0.043) and a poor-trend of DFS (P = 0.12) in LUSC patients. The differential prognostic implication of RRM2B expression in the LUSC and LUAD patients appeared to be weaker in the TCGA dataset when the median expression was selected as a cutoff point (Figure 5). However, if the optimal expression was used as a cutoff point, the cancer survivals predicted by RRM2B also trended differentially in the LUAD and LUSC patients, similar to the findings in the KM-plotter cohort (Figure S5). These results showed that RRM2B had opposite prognostic effects in the patients with LUAD and LUSC, which suggested that the RRM2B may function differently in LUAD and LUSC.
To validate the potential implication of RRM1, RRM2, and RRM2B expression in pathology and prognosis of NSCLC, 211 surgically excised tumor specimens were independently collected from the patients with LUSC and LUAD (n = 97 and 114, respectively) from three academic hospitals affiliated to Zhejiang University in China (named the ZJUC cohort hereafter). The demographics and clinicopathological characteristics of the ZJUC cohort are described in Table S1. Among them, 72 percent of the ZJUC cohort were male with a mean age of 60.8 ± 9.6 years, and a median follow-up period of 45 months.
All specimens were examined by immunohistochemical (IHC) staining for three RR subunit expression. The results showed that RRM1 and RRM2 protein mainly localized in the cytosol while RRM2B protein was found in both the cytosol and nucleoplasm of LUSC and LUAD tumor cells (Figure 6A). This was consistent with the subcellular localization observed in an immunofluorescent study of three cancer cell lines (A-431, U-2 OS and U-251 MG) in HPA (Figure S2). Representative IHC pictures of three RR subunit protein expression in the NSCLC tissue samples were shown in Figure 6B. RRM1 and RRM2 protein expressions were significantly increased in both LUSC and LUAD cancer cells as compared to the adjacent normal lung tissues (Figure 6C). RRM2 protein expression was significantly higher in the stage IIIA LUSC or LUAD tumors compared with those in the stage I tumors (P < 0.05). A similar pattern of RRM2 protein expression was also observed in the stage IIIB/IV LUAD tumors compared to their stage I counterparts (P < 0.05). RRM1 expression was also significantly higher in the stage IIIA LUSC and LUAD compared to the stage I tumors (P < 0.05). Interestingly, while RRM2B expression was also higher in the LUSC tumors, as compared to their adjacent normal lung tissues, and was higher in the stage IIIB/IV tumors than the stage II ones (P < 0.05), RRM2B expression appeared to be decreased in LUAD tumors although this level was not statistically significant.
Figure 6. Clinical relevance analyses of RRM1, RRM2, and RRM2B protein expression levels in LUSC and LUAD patients including immunohistochemistry staining and follow-up studies. (A) Localization of RRM1, RRM2, and RRM2B by IHC staining in ZJUC cohort. (B) Protein expression levels by IHC staining in the ZJUC cohort. (C) Association between RRM1, RRM2, and RRM2B protein expression levels and prognosis of LUSC and LUAD patients. ***P < 0.001. (D) The protein expression of RRM1, RRM2, and RRM2B in the different TNM stages of LUSC and LUAD in the ZJUC cohort. IHC staining of RRM1, RRM2, and RRM2B proteins in the clinical tissue samples of LUSC and LUAD patients in the ZJUC cohort (97 LUSC and 114 LUAD). IHC staining was evaluated with a score range from 0 to 16. See Method for scoring criteria.
Follow-up and survival analyses of ZJUC cohort patients suggested that higher RRM1 and RRM2 protein levels were associated with a significantly shorter OS in the LUAD patients (P = 0.003 and 0.023 for RRM1 and RRM2, respectively), but not in the LUSC patients (P > 0.05). Higher RRM2B protein level was associated with a significantly shorter OS (P = 0.005) in the LUSC patients but a significantly prolonged OS (P = 0.001) in the LUAD patients (Figure 6D). These results were similar with what were obtained from data-mining (Figure 5). In conclusion, the IHC analysis of RR subunit protein expressions and the associated survival study in the ZJUC cohort of lung cancer patients demonstrated the usefulness and relevance of our bioinformatic analysis through data mining of multiple cancer-omics databases.
To uncover the underlying mechanisms of the opposite prognostic effects of RRM2B expression in the cases of LUSC as opposed to LUAD, we performed a co-expression gene network analysis of RRM2B in these two subtypes of NSCLCs based on RNA-seq data from TCGA. We used the Pearson's correlation coefficient (CC) with around 0.5 as a cutoff, to identify the RRM2B-associated genes. The resulting networks consisted of 30 genes, 14 in LUSC and 16 in LUAD, with one gene in common. These genes were connected via 166 expressional interactions. The positive or negative correlations and the different correlation intensities are visualized using Cytoscape 3.0 software (Figure 7A and Table S2). Significantly, the results showed two distinct panels of co-expressed genes connected by RRM2B between LUAD and LUSC, with only one overlapping gene.
Figure 7. Gene co-expression networks of RRM2B in LUSC and LUAD. (A) Two co-expressed gene networks of highly correlated genes with RRM2B in LUSC (left panel) and LUAD (right) were constructed using Pearson's correlation coefficient (CC) analysis. Green nodes represent GO terms for gene function, pink nodes denote correlated genes, and lines connect paired co-expressed genes (blue for negative correlation and red for positive correlation). Three correlation intensities (strong: |CC| > 0.9; middle: 0.7 ≤ |CC| ≤ 0.9; weak: |CC| < 0.7) are represented by different line thickness. (B) The mRNA expression associations of RRM2 or RRM2B with 10 RRM2B highly co-expressed genes (MYBL2, PIF1, KIFC1, KIF18B, TROAP, TICRR, CDCA3, PKMYT1, and TACC3) participating in the regulation of the cell cycle in LUAD. R: Pearson correlation coefficient.
A further functional enrichment analysis of two co-expression gene networks using DAVID bioinformatics resources version 6.8 found that the gene set negatively associated with RRM2B expression in LUAD was enriched together with the genes promoting cell cycle progression and malignant aggressiveness, such as those of cell cycle regulation related biological processes and DNA repair. In contrast, the gene set co-expressed with RRM2B in the LUSC consisted of the genes associated with protein destabilization and the ERAD pathway and were not the obligate participants of cell cycle regulation. These results suggested that RRM2B may play double- or multifaceted roles in different types of lung carcinomas using distinct molecular machineries which resulted in its opposite prognostic impacts on LUAD and LUSC. In addition, nine genes involved in cell cycle progression (MYBL2, PIF1, KIFC1, KIF18B, TROAP, TICRR, CDCA3, PKMYT1, and TACC3) were found reversely correlated with RRM2B expression, but tightly positive-correlated with RRM2 expression, in LUAD (Figure 7B). This observation further suggests that the different associations of RRM2B and RRM2 proteins with the members of cell cycle pathway in LUAD may be the underlying mechanism of the opposite prognostic effects of different RR subunits observed in patients with LUAD and LUSC.
Among the current publicly available cancer-omics databases, those genomics and transcriptomics platforms with data-mining tools and data integration functions have been widely used in cancer research and precision medicine. Table S3 shows some popular cancer-omics databases and their main features (1–8, 11, 17–19). However, considering the different specimen sources and the nature of cancer heterogeneity, significant amounts of the data needs to be collected and crossly validated. HPA provides global analysis of how cancer genomes are expressed at the protein level, but its application is limited by a small sample size and lack of quantification. More recently, with the advance of high-throughput single-cell genomics, the Human Cell Atlas (HCA) Project has made progress in defining distinctive molecular profiles of all the human cell types and functions (12) and may soon help promote a profound understanding of the human tumor cellular ecosystem. Although clinical application of cancer-omics is encouraging, a significant challenge remains in terms of how to more efficiently use these rapidly expanding big data sets from multiple sources. In this work, using the study of three RR subunit genes (RRM1, RRM2, and RRM2B) as an example, we proposed a straightforward approach for integrative analysis of multiple cancer omics databases of virtually any genes of interest, which, combined with clinical patients validation, can improve the definition of cancer gene expressional patterns to further elucidate their underlying mechanisms as well as facilitate their clinical application (Figure 1).
RR plays a key role in DNA synthesis and thus is essential for cell proliferation and the development of malignancy (20). In normal human cells, three RR subunit proteins form two-types of holoenzymes (RRM1- RRM2 and RRM1- RRM2B) and their genes are separately distributed in three different chromosomes. Although RRM2 and RRM2B are highly homologous in their gene sequences, their expressional levels and subcellular localizations are differently regulated in cells. The expression and degradation of RRM2 is regulated in a cell cycle-dependent manner (21), while RRM2B is induced in response to DNA damage in a p53-dependent manner (22). Our IHC examination of NSCLC tumor tissue and immunofluorescent images of three cell lines in the HPA have demonstrated that both RRM1 and RRM2 are mainly expressed in the cytoplasm, whereas RRM2B is expressed both in the cytoplasm and the nucleus (Figure 6A and Figure S2). This is consistent with the notion that ribonucleotide reduction with RRM2-containing RR takes place in the cytoplasm and the dNTPs are then transported into the nucleus for DNA synthesis. Conversely, RRM2B-containing RR is more likely to remain in the nucleus, ready for the repair of genetic abnormalities which constantly occur in human cancer cells (23, 24).
In human cancers, the mutation rates of RRM1, RRM2, and RRM2B are all below 0.5%, based on the data from COSMIC and cBioPortal (Table S4). The primary form of mutation is a missense substitution, which appears to be randomly distributed. Only several mutants (e.g., RRM1R499C/H, RRM2BP308L/S, RRM2BF323L) have been identified more than three times. There is almost no copy number variation (CNV) of RRM1 and RRM2 genes in common types of cancers, whereas RRM2B displays CNV gain in more than 3% of breast cancers, liver cancers, stomach cancers and urinary tract cancers. In this study, we found that gene expression dysregulation might be the dominant characteristic of the multi-subunit enzyme RR in human cancers. The expression of three RR genes, especially RRM1 and RRM2, increased significantly across common types of cancers (Figure 2). Typically, RRM2 up-expression occurred in almost all malignancies, and the FCs of RRM2 expression between cancer and normal tissues were much larger than those in either RRM1 or RRM2B. The gene expression of RR in human cancers has been analyzed previously using Oncomine (last updated in Nov, 2013) (25). Here, we further analyzed up to 358, 355, and 209 Oncomine studies for the differential expression of RRM1, RRM2, and RRM2B, respectively (detail comparisons in Table S5). With approximately twice as many studies collected in recent years, we demonstrated a more extensive expressional landscape and increased characteristics of RR genes in common types of cancers. Some additional information was also included, such as the down-regulated expression of RRM2B in lung cancer, leukemia and lymphoma, and the down-regulation of RRM1 expression in bladder, breast and prostate cancers. Importantly, for the first time, we revealed the correlations among RRM1, RRM2, and RRM2B expression during cancer development as well as their individual roles and collaborative relationships during cancer progression together with their prognosis with clinical tumor sample validation.
The differential expressional changes and possible roles of three RR components have been noted previously in different clinical cancer samples, including lung (26), digestive tract (13, 27, 28) and other cancers (29). Among the three RR subunit genes, RRM2 has been proposed to be an oncoprotein (25, 30). Upregulation of RRM2 has been associated with cancer cell proliferation, invasion and metastasis in multiple types of cancers (28, 29, 31, 32). In contrast, although RRM1 expression was also found to be up-regulated in many types of cancers, its role in cancer development remains controversial. Consistent with the role of RR in DNA replication and repair, a high RRM1 expression is known to be associated with a poor response to the DNA-damaging platinum drugs and to the RRM1-targeting drug gemcitabine, and thus led to poor outcomes in these cancer patients (33–35). However, several studies have also shown that a highly expressed RRM1 might be associated with a better outcome for some cancer patients (36, 37), suggestive of a suppressor role of tumor initiation, invasion and metastasis. A recent study demonstrated that RRM1 can negatively regulate ZRANB3 function when in the nucleus, leading to DNA-synthesis reduction (38). The role of RRM2B in tumors is also not clear (25, 30). For example, it has been reported that RRM2B expression was reversely associated with tumor metastasis and was correlated with a better survival in colorectal cancer patients (39, 40). RRM2B expression was also shown to be reversely associated with intrahepatic metastasis in hepatocellular carcinomas (41). Conversely, RRM2B expression has been previously reported to be positively related to the development of esophageal squamous cell carcinoma (27). In this study, by combining RNA-seq and clinicopathologic data from Oncomine and TCGA (Figures 2, 3), we found that expression of RRM1 and RRM2 genes were both significantly increased and correlated with higher TNM stages in most common types of cancers and demonstrated a tumor-promoting role for the RRM1-RRM2 holoenzyme. In contrast, the expression of RRM2B in these cancers varied, and the correlations between the expression of RRM1 and RRM2B were weak and variably dependent on cancer type. Notably, the expression of RRM2 and RRM2B were much weaker or even reversely correlated in some types of cancers. These data suggested that expression of three RR genes, and thus the related enzyme activities, may be dysregulated in the cancer cells. They probably operated under different mechanisms related to aspects of cancer progression or patient survival in different cancer types and stages. Moreover, the differential expression pattern of three RR genes in different types of human cancers suggested that, in addition to forming a RR holoenzyme to implement the classical enzymatic function for cancer cell proliferation, each of the three component proteins may be individually recruited by cancer cells to play a different non-RR enzymatic role during cancer initiation and development.
The clinical significance of different RR expression in cancers have attracted much attention, especially in NSCLC (33, 42). However, discrepancies among different reports remains to be elucidated. For RRM1, some studies showed that high RRM1 expression was associated with better survival in early stage NSCLC (26) or had poor prognosis in advanced NSCLC (43) or lung adenocarcinoma (44), while another study showed that RRM1 protein expression had no significant predictive value for early NSCLC patients (45). For RRM2, some studies suggested that high expression of RRM2 prognosticated a shorter overall survival for NSCLC patients (46, 47), while the others did not find any predictive value (26, 48). For RRM2B, while it was shown that high expression of RRM2B protein was a favorable prognostic factor in early NSCLC patients (49), other authors reported that RRM2B protein expression did not play a prognostic role in NSCLC patients with resected TNM stages I–III tumors (45, 50). Such controversies can be attributed into the following reasons: different expression detection means, sample selection bias and cancer heterogeneity. In this study, we revealed another important reason that there was no distinction of different pathological subtypes. LUSC arises in proximal airways and is more strongly associated with smoking and chronic inflammation than is LUAD, which arises more frequently in the distal airways. By integrative cancer-omics data analysis and clinical sample validation with IHC, we showed that differential expression of three RR components individually and collaboratively impact malignant progression and patient survival in LUSC and LUAD. In addition, we found that LUSC had higher RRM1 and RRM2 expression than LUAD, while RRM2B expressed lower in tumor tissues of LUSC than in those of LUAD (Figure S3). The results implied the different roles of RR subunits in LUSC and LUAD. Recently, Uhlen et al. found high expression of the Endoplasmic Reticulum Oxidoreductase 1 Alpha (ERO1A) gene was correlated with a bad prognosis in NSCLC (including LUSC and LUAD) (51). However, after distinguishing pathological subtypes, we also found opposite prognostic effects of a high expression of ERO1A in LUSC and LUAD (Figure S4). This further supported the necessity to separate the tumors by their histological or pathological subtypes during research and clinical evaluation of molecular biomarkers in NSCLC.
It was unanticipated that a higher expression of RRM2B was significantly associated with a shorter survival in LUSC but a more prolonged survival in LUAD, while higher expressed RRM1 and RRM2 predicted a poorer clinical outcome in LUAD (Figures 5, 6). By co-expression gene network analysis, distinct gene sets were revealed to be associated with RRM2 and RRM2B in LUSC and LUAD, respectively (Figures 7A,B and Table S1). RRM2B expression was reversely correlated with cell cycle-promoting molecules in LUAD but not in LUSC, suggesting that RRM2B may directly or indirectly suppress cell cycle progression, hence cancer cell proliferation, in LUAD. DNA microarray analysis has shown that the gene set regulating cell-cycle progression was significantly enriched in p53R2 (the alias of RRM2B)-silenced human KB oropharyngeal carcinoma cells. RRM2B may suppress cancer cell proliferation partially by upregulation of p21 and downregulation of cyclin D1 in addition to playing a critical role in DNA repair (52). By contrast, RRM2 expression was significantly associated with cell cycle-progression (Figure 7B), consistent with its role as a RR subunit to promote cancer cell proliferation. Thus, the different co-expressed gene networks are compatible with the opposite roles and different underlying mechanisms of RRM2 and RRM2B in different types of cancers, which further stressed the importance of the rationale of the use of RR inhibitors in precision cancer medicine.
Taken together, the systematic translation of cancer-omics data into the fields of tumor biology and cancer therapy remains challenging. Herein, we demonstrated an integrative analytical approach based on mining multiple cancer-omics databases to reveal across-cancer-type expression patterns and their impacts on clinical outcomes of any genes of interest, followed by validation of protein-levels in clinical cancer samples from multiple cancer centers. Using this technique, we depicted a landscape of expression and association of three RR components in different types of common cancers. We further demonstrated a more complex pattern of three RR components in different subtypes and stages of lung cancer and associated these with clinical survival and discussed the possible underlying mechanisms. While the extensive high-expression of RR components in common cancers suggests them to be the targets of a broad-spectrum anti-cancer therapies, the heterogeneous expression pattern of each component of RR in different types of cancers and individual cancer patient supports their important requirement for more specifically targeted aspects and increased precision in cancer therapy.
Herein, we developed an integrative analytical approach to reveal across-cancer expression patterns and identify potential clinical impacts for genes of interest from public databases. Using ribonucleotide reductase (RR) as an example, we characterized the expression profiles and inter-component associations of three RR subunit genes across over 30-types of cancers. In addition, we assessed and validated pathological and prognostic significance of RR in lung cancer and related pathological subtypes. Underlying mechanisms were further explored and discussed.
The datasets generated for this study are available on request to the corresponding author.
The study was conducted according to the principles of the Declaration of Helsinki, and it was approved by the First and Second Affiliated Hospitals of Zhejiang University, and Zhejiang Cancer Hospital, Zhejiang, China (No. 2018-753-2). Written informed consents were obtained from all subjects enrolled in this study.
JS, CL, and LT designed and supervised the research. YD, CL, and TZ analyzed the data. YD, CL, MW, TZ, LL, and XL prepared the figures and tables. TZ, XX, GR, and ZJ constructed the IHC. YD, CL, and TZ wrote the manuscript. JS, CL, LT, YD, DS, HL, LZ, HJ, QLin, QLiu, and TZ revised the manuscript. All authors read and approved the final manuscript.
This work was supported by National Natural Science Foundation of China (81372138, 81572384, and 81771518), National Science and Technology Major Project of China (2018ZX10302206-006-007), National Key R&D Program of China (2016YFC1303401), and Zhejiang Provincial Natural Science Foundation of China (LY17C060003 and LZ16H16000).
The authors declare that the research was conducted in the absence of any commercial or financial relationships that could be construed as a potential conflict of interest.
We would like to thank Mr. Chris Wood and Dr. Yongmei Xi from Zhejiang University for improvement of English writing of the manuscript. We would like to thank the reviewers for their valuable hints and suggestions. The authors are grateful to the research groups for providing the clinical information and microarray data in this study. And we are deeply thankful for the computational resources provided by the Super Computer System, Human Genome Center, Institute of Medical Science, University of Tokyo, Japan.
The Supplementary Material for this article can be found online at: https://www.frontiersin.org/articles/10.3389/fonc.2019.00956/full#supplementary-material
BLCA, Bladder urothelial carcinoma; BRCA, Breast invasive carcinoma; CESC, Cervical and endocervical cancers; COAD, Colon adenocarcinoma; COADREAD, Colorectal adenocarcinoma; DEGs, Differentially expressed genes; ESCA, Esophageal carcinoma; GBM, Glioblastoma multiforme; GBMLGG, Glioma; HNSC, Head and Neck squamous cell carcinoma; KICH, Kidney Chromophobe; KIPAN, Pan-kidney cohort (KICH+KIRC+KIRP); KIRC, Kidney renal clear cell carcinoma; KIRP, Kidney renal papillary cell carcinoma; LAML, Acute Myeloid Leukemia; LCLC, large cell lung carcinoma; LGG, Brain Lower Grade Glioma; LIHC, Liver hepatocellular carcinoma; LUAD, Lung adenocarcinoma; LUSC, Lung squamous cell carcinoma; OV, Ovarian serous cystadenocarcinoma; PAAD, Pancreatic adenocarcinoma; PCPG, Pheochromocytoma and Paraganglioma; PRAD, Prostate adenocarcinoma; READ, Rectum adenocarcinoma; SARC, Sarcoma; SCLC, small cell lung carcinoma; SKCM, Skin Cutaneous Melanoma; STAD, Stomach adenocarcinoma; STES, Stomach and Esophageal carcinoma; TGCT, Testicular Germ Cell Tumors; THCA, Thyroid carcinoma; THYM, Thymoma; UCEC, Uterine Corpus Endometrial Carcinoma.
1. Forbes SA, Beare D, Gunasekaran P, Leung K, Bindal N, Boutselakis H, et al. COSMIC: exploring the world's knowledge of somatic mutations in human cancer. Nucleic Acids Res. (2015) 43: D805–11. doi: 10.1093/nar/gku1075
2. Tomczak K, Czerwinska P, Wiznerowicz M. The Cancer Genome Atlas (TCGA): an immeasurable source of knowledge. Contemp Oncol. (2015) 19:A68–77. doi: 10.5114/wo.2014.47136
3. Hudson TJ, Anderson W, Artez A, Barker AD, Bell C, Bernabé RR, et al. International network of cancer genome projects. Nature. (2010) 464:993–8. doi: 10.1038/nature08987
4. Li J, Lu Y, Akbani R, Ju Z, Roebuck PL, Liu W, et al. TCPA: a resource for cancer functional proteomics data. Nat Methods. (2013) 10:1046–7. doi: 10.1038/nmeth.2650
5. Rhodes DR, Kalyana-Sundaram S, Mahavisno V, Varambally R, Yu J, Briggs BB, et al. Oncomine 3.0: genes, pathways, and networks in a collection of 18,000 cancer gene expression profiles. Neoplasia. (2007) 9:166–80. doi: 10.1593/neo.07112
6. Cerami E, Gao J, Dogrusoz U, Gross BE, Sumer SO, Aksoy BA, et al. The cBio cancer genomics portal: an open platform for exploring multidimensional cancer genomics data. Cancer Discov. (2012) 2:401–4. doi: 10.1158/2159-8290.CD-12-0095
7. Gyorffy B, Surowiak P, Budczies J, Lánczky A. Online survival analysis software to assess the prognostic value of biomarkers using transcriptomic data in non-small-cell lung cancer. PLoS ONE. (2013) 8:e82241. doi: 10.1371/journal.pone.0082241
8. Uhlen M, Fagerberg L, Hallstrom BM, Lindskog C, Oksvold P, Mardinoglu A, et al. Proteomics. Tissue-based map of the human proteome. Science. (2015) 347:1260419. doi: 10.1126/science.1260419
9. Raponi M, Zhang Y, Yu J, Chen G, Lee G, Taylor JM, et al. Gene expression signatures for predicting prognosis of squamous cell and adenocarcinomas of the lung. Cancer Res. (2006) 66:7466–72. doi: 10.1158/0008-5472.CAN-06-1191
10. Shedden K, Taylor JM, Enkemann SA, Tsao MS, Yeatman TJ, Gerald WL, et al. Gene expression-based survival prediction in lung adenocarcinoma: a multi-site, blinded validation study. Nat Med. (2008) 14:822–7. doi: 10.1038/nm.1790
11. Barretina J, Caponigro G, Stransky N, Venkatesan K, Margolin AA, Kim S, et al. The Cancer Cell Line Encyclopedia enables predictive modelling of anticancer drug sensitivity. Nature. (2012) 483:603–7. doi: 10.1038/nature11003
12. Regev A, Teichmann SA, Lander ES, Amit I, Benoist C, Birney E, et al. The human cell atlas. Elife. (2017) 6:e27041. doi: 10.7554/eLife.27041
13. Lou M, Liu Q, Ren G, Zeng J, Xiang X, Ding Y, et al. Physical interaction between human ribonucleotide reductase large subunit and thioredoxin increases colorectal cancer malignancy. J Biol Chem. (2017) 292:9136–49. doi: 10.1074/jbc.M117.783365
14. Shannon P, Markiel A, Ozier O, Baliga NS, Wang JT, Ramage D, et al. Cytoscape: a software environment for integrated models of biomolecular interaction networks. Genome Res. (2003) 13:2498–504. doi: 10.1101/gr.1239303
15. Cheson BD, Bennett JM, Kopecky KJ, Büchner T, Willman CL, Estey EH, et al. Revised recommendations of the international working group for diagnosis, standardization of response criteria, treatment outcomes, and reporting standards for therapeutic trials in acute myeloid leukemia. J Clin Oncol. (2003) 21:4642–9. doi: 10.1200/JCO.2003.04.036
16. Robinson DR, Wu YM, Lonigro RJ, Vats P, Cobain E, Everett J, et al. Integrative clinical genomics of metastatic cancer. Nature. (2017) 548:297–303. doi: 10.1038/nature23306
17. Tang Z, Li C, Kang B, Gao G, Li C, Zhang Z. GEPIA: a web server for cancer and normal gene expression profiling and interactive analyses. Nucleic Acids Res. (2017) 45:W98–102. doi: 10.1093/nar/gkx247
18. Kim MS, Pinto SM, Getnet D, Nirujogi RS, Manda SS, Chaerkady R, et al. A draft map of the human proteome. Nature. (2014) 509:575–81. doi: 10.1038/nature13302
19. Lonsdale J, Thomas J, Salvatore M, Phillips R. The Genotype-Tissue Expression (GTEx) project. Nat Genet. (2013) 45:580–5. doi: 10.1038/ng.2653
20. Nordlund P, Reichard P. Ribonucleotide reductases. Annu Rev Biochem. (2006) 75:681–706. doi: 10.1146/annurev.biochem.75.103004.142443
21. Eriksson S, Gräslund A, Skog S, Thelander L, Tribukait B. Cell cycle-dependent regulation of mammalian ribonucleotide reductase. The S phase-correlated increase in subunit M2 is regulated by de novo protein synthesis. J Biol Chem. (1984) 259:11695–700.
22. Lozano G, Elledge SJ. p53 sends nucleotides to repair DNA. Nature. (2000) 404:24–25. doi: 10.1038/35003670
23. Xue L, Zhou B, Liu X, Qiu W, Jin Z, Yen Y. Wild-type p53 regulates human ribonucleotide reductase by protein-protein interaction with p53R2 as well as hRRM2 subunits. Cancer Res. (2003) 63:980–6.
24. Niida H, Katsuno Y, Sengoku M, Shimada M, Yukawa M, Ikura M, et al. Essential role of Tip60-dependent recruitment of ribonucleotide reductase at DNA damage sites in DNA repair during G1 phase. Genes Dev. (2010) 24:333–8. doi: 10.1101/gad.1863810
25. Aye Y, Li M, Long MJ, Weiss RS. Ribonucleotide reductase and cancer: biological mechanisms and targeted therapies. Oncogene. (2015) 34:2011–21. doi: 10.1038/onc.2014.155
26. Bepler G, Sharma S, Cantor A, Gautam A, Haura E, Simon G, et al. RRM1 and PTEN as prognostic parameters for overall and disease-free survival in patients with non-small-cell lung cancer. J Clin Oncol. (2004) 22:1878–85. doi: 10.1200/JCO.2004.12.002
27. Okumura H, Natsugoe S, Yokomakura N, Kita Y, Matsumoto M, Uchikado Y, et al. Expression of p53R2 is related to prognosis in patients with esophageal squamous cell carcinoma. Clin Cancer Res. (2006) 12:3740–5. doi: 10.1158/1078-0432.CCR-05-2416
28. Morikawa T, Hino R, Uozaki H, Maeda D, Ushiku T, Shinozaki A, et al. Expression of ribonucleotide reductase M2 subunit in gastric cancer and effects of RRM2 inhibition in vitro. Hum Pathol. (2010) 41:1742–8. doi: 10.1016/j.humpath.2010.06.001
29. Morikawa T, Maeda D, Kume H, Homma Y, Fukayama M. Ribonucleotide reductase M2 subunit is a novel diagnostic marker and a potential therapeutic target in bladder cancer. Histopathology. (2010) 57:885–92. doi: 10.1111/j.1365-2559.2010.03725.x
30. Shao J, Liu X, Zhu L, Yen Y. Targeting ribonucleotide reductase for cancer therapy. Expert Opin Ther Targets. (2013) 17:1423–37. doi: 10.1517/14728222.2013.840293
31. Ma XJ, Salunga R, Tuggle JT, Gaudet J, Enright E, McQuary P, et al. Gene expression profiles of human breast cancer progression. Proc Natl Acad Sci USA. (2003) 100:5974–9. doi: 10.1073/pnas.0931261100
32. Aird KM, Zhang G, Li H, Tu Z, Bitler BG, Garipov A, et al. Suppression of nucleotide metabolism underlies the establishment and maintenance of oncogene-induced senescence. Cell Rep. (2013) 3:1252–65. doi: 10.1016/j.celrep.2013.03.004
33. Bepler G, Williams C, Schell MJ, Chen W, Zheng Z, Simon G, et al. Randomized international phase III trial of ERCC1 and RRM1 expression-based chemotherapy versus gemcitabine/carboplatin in advanced non-small-cell lung cancer. J Clin Oncol. (2013) 31:2404–12. doi: 10.1200/JCO.2012.46.9783
34. Akita H, Zheng Z, Takeda Y, Kim C, Kittaka N, Kobayashi S, et al. Significance of RRM1 and ERCC1 expression in resectable pancreatic adenocarcinoma. Oncogene. (2009) 28:2903–9. doi: 10.1038/onc.2009.158
35. Jordheim LP, Sève P, Trédan O, Dumontet C. The ribonucleotide reductase large subunit (RRM1) as a predictive factor in patients with cancer. Lancet Oncol. (2011) 12:693–702. doi: 10.1016/S1470-2045(10)70244-8
36. Fan H, Huang A, Villegas C, Wright JA. The R1 component of mammalian ribonucleotide reductase has malignancy-suppressing activity as demonstrated by gene transfer experiments. Proc Natl Acad Sci USA. (1997) 94:13181–6. doi: 10.1073/pnas.94.24.13181
37. Gautam A, Li ZR, Bepler G. RRM1-induced metastasis suppression through PTEN-regulated pathways. Oncogene. (2003) 22:2135–42. doi: 10.1038/sj.onc.1206232
38. Fu Y, Long MJC, Wisitpitthaya S, Inayat H, Pierpont TM, Elsaid IM, et al. Nuclear RNR-alpha antagonizes cell proliferation by directly inhibiting ZRANB3. Nat Chem Biol. (2018) 14:943–54. doi: 10.1038/s41589-018-0113-5
39. Liu X, Zhou B, Xue L, Shih J, Tye K, Lin W, et al. Metastasis-suppressing potential of ribonucleotide reductase small subunit p53R2 in human cancer cells. Clin Cancer Res. (2006) 12:6337–44. doi: 10.1158/1078-0432.CCR-06-0799
40. Liu X, Lai L, Wang X, Xue L, Leora S, Wu J, et al. Ribonucleotide reductase small subunit M2B prognoses better survival in colorectal cancer. Cancer Res. (2011) 71:3202–13. doi: 10.1158/0008-5472.CAN-11-0054
41. Tian H, Ge C, Li H, Zhao F, Hou H, Chen T, et al. Ribonucleotide reductase M2B inhibits cell migration and spreading by early growth response protein 1-mediated phosphatase and tensin homolog/Akt1 pathway in hepatocellular carcinoma. Hepatology. (2014) 59:1459–70. doi: 10.1002/hep.26929
42. Simon G, Sharma A, Li X, Hazelton T, Walsh F, Williams C, et al. Feasibility and efficacy of molecular analysis-directed individualized therapy in advanced non-small-cell lung cancer. J Clin Oncol. (2007) 25:2741–6. doi: 10.1200/JCO.2006.08.2099
43. Lee JJ, Maeng CH, Baek SK, Kim GY, Yoo JH, Choi CW, et al. The immunohistochemical overexpression of ribonucleotide reductase regulatory subunit M1 (RRM1) protein is a predictor of shorter survival to gemcitabine-based chemotherapy in advanced non-small cell lung cancer (NSCLC). Lung Cancer. (2010) 70:205–10. doi: 10.1016/j.lungcan.2010.02.005
44. Souglakos J, Boukovinas I, Taron M, Mendez P, Mavroudis D, Tripaki M, et al. Ribonucleotide reductase subunits M1 and M2 mRNA expression levels and clinical outcome of lung adenocarcinoma patients treated with docetaxel/gemcitabine. Br J Cancer. (2008) 98:1710–5. doi: 10.1038/sj.bjc.6604344
45. Grossi F, Dal Bello MG, Salvi S, Puzone R, Pfeffer U, Fontana V, et al. Expression of ribonucleotide reductase subunit-2 and thymidylate synthase correlates with poor prognosis in patients with resected stages I-III non-small cell lung cancer. Dis Markers. (2015) 2015:302649. doi: 10.1155/2015/302649
46. Boukovinas I, Papadaki C, Mendez P, Taron M, Mavroudis D, Koutsopoulos A, et al. Tumor BRCA1, RRM1 and RRM2 mRNA expression levels and clinical response to first-line gemcitabine plus docetaxel in non-small-cell lung cancer patients. PLoS ONE. (2008) 3:e3695. doi: 10.1371/journal.pone.0003695
47. Wang L, Meng L, Wang XW, Ma GY, Chen JH. Expression of RRM1 and RRM2 as a novel prognostic marker in advanced non-small cell lung cancer receiving chemotherapy. Tumour Biol. (2014) 35:1899–906. doi: 10.1007/s13277-013-1255-4
48. Xian-Jun F, Xiu-Guang Q, Li Z, Hui F, Wan-Ling W, Dong L, et al. ERCC1 and BRCA1 mRNA expression predicts the clinical outcome of non-small cell lung cancer receiving platinum-based chemotherapy. Pak J Med Sci. (2014) 30:488–92. doi: 10.12669/pjms.303.4187
49. Hsu NY, Wu JY, Liu X, Yen Y, Chen CY, Chou MC, et al. Expression status of ribonucleotide reductase small subunits hRRM2/p53R2 as prognostic biomarkers in stage I and II non-small cell lung cancer. Anticancer Res. (2011) 31:3475–81.
50. Uramoto H, Sugio K, Oyama T, Hanagiri T, Yasumoto K. P53R2, p53 inducible ribonucleotide reductase gene, correlated with tumor progression of non-small cell lung cancer. Anticancer Res. (2006) 26:983–8.
51. Uhlen M, Zhang C. A pathology atlas of the human cancer transcriptome. Science. (2017) 357:eaan2507. doi: 10.1126/science.aan2507
Keywords: cancer-omics, integrative analysis, ribonucleotide reductase, expression characteristics, clinical relevance, gene networks
Citation: Ding Y, Zhong T, Wang M, Xiang X, Ren G, Jia Z, Lin Q, Liu Q, Dong J, Li L, Li X, Jiang H, Zhu L, Li H, Shen D, Teng L, Li C and Shao J (2019) Integrative Analysis Reveals Across-Cancer Expression Patterns and Clinical Relevance of Ribonucleotide Reductase in Human Cancers. Front. Oncol. 9:956. doi: 10.3389/fonc.2019.00956
Received: 24 July 2019; Accepted: 10 September 2019;
Published: 04 October 2019.
Edited by:
Zhi Sheng, Virginia Tech, United StatesReviewed by:
Vijay Pandey, Tsinghua-Berkeley Shenzhen Institute, ChinaCopyright © 2019 Ding, Zhong, Wang, Xiang, Ren, Jia, Lin, Liu, Dong, Li, Li, Jiang, Zhu, Li, Shen, Teng, Li and Shao. This is an open-access article distributed under the terms of the Creative Commons Attribution License (CC BY). The use, distribution or reproduction in other forums is permitted, provided the original author(s) and the copyright owner(s) are credited and that the original publication in this journal is cited, in accordance with accepted academic practice. No use, distribution or reproduction is permitted which does not comply with these terms.
*Correspondence: Lisong Teng, bHN0ZW5nQHpqdS5lZHUuY24=; Chen Li, Y2hlbmxpMjAxMkB6anUuZWR1LmNu; Jimin Shao, c2hhb2ppbWluQHpqdS5lZHUuY24=
Disclaimer: All claims expressed in this article are solely those of the authors and do not necessarily represent those of their affiliated organizations, or those of the publisher, the editors and the reviewers. Any product that may be evaluated in this article or claim that may be made by its manufacturer is not guaranteed or endorsed by the publisher.
Research integrity at Frontiers
Learn more about the work of our research integrity team to safeguard the quality of each article we publish.