- 1Department of Urology, Fudan University Shanghai Cancer Center, Shanghai, China
- 2Department of Oncology, Shanghai Medical College, Fudan University, Shanghai, China
- 3Department of Urology, Affiliated Hospital of Nantong University, Nantong, China
- 4Department of Medical Oncology, Clinical Medical College of Yangzhou University, Northern Jiangsu People's Hospital, Yangzhou, China
Purpose: We aimed to develop and validate a novel gene signature from published data and improve the prediction of survival in muscle-invasive bladder cancer (MIBC).
Methods: We searched the published gene signatures associated with the overall survival (OS) of MIBC and compiled all 274 genes to develop a novel gene signature. RNAseq data of TCGA (the Cancer Genome Atlas) bladder cohort were downloaded. All genes were included in a univariate Cox hazard ratio model. We then used a reduced multivariate Cox regression model, which included only genes achieving P < 0.05 in the univariate model. A total of 172 patients at Fudan University Shanghai Cancer Center (FUSCC) and 61 patients from GEO datasets were used as an external validation set.
Results: A total of 327 patients in the TCGA cohort were enrolled. We identified 274 genes from eight published papers on the OS of MIBC. Using the TCGA database, we identified 12 genes that correlated with OS (P < 0.05 in both univariate and multivariate analyses). By integrating these genes with the RT-qPCR data in our validation dataset and GEO datasets, we confirmed that the power for predicting OS of the 12-gene panel (AUC of 0.741 and 0.727, respectively) was higher than just clinical data (including gender, age, T stage, grade, and N stage) alone in the TCGA and FUSCC cohort (AUC of 0.667 and 0.631, respectively). Additionally, upon combining the clinical data and 12-gene panel together, the AUC increased to 0.768, 0.757, and 0.88 in the TCGA, FUSCC and GSE13507 cohorts, respectively.
Conclusions: Applying published gene signatures and TCGA data, we successfully built and externally validated a novel 12-gene signature for the survival of MIBC.
Brief Explanation
We systemically reviewed all published prognostic gene signatures of muscle-invasive bladder cancer (MIBC) and integrated the genes in the TCGA MIBC cohort. This new gene panel was validated in a newly established MIBC cohort in GEO and FUSCC. This method can help update the previous established panels in a new way.
Introduction
Bladder cancer is the fourth most common cancer, with an incidence of ~7% among all male malignancies, and the eighth most common cause of mortality in men (1). In 2015, a total of 80,500 new bladder cancer cases were expected in China, with 32,900 estimated cancer-related deaths (2). Urothelial carcinoma is the dominant histological subtype of bladder cancer, except in certain parts of Africa and the Middle East (3). Despite the considerable progress in the treatment of bladder cancer, the prognosis of patients with muscle-invasive bladder cancer (MIBC) remains poor, which is partly attributable to the heterogeneity of disease characteristics (4). This indicates the need for an accurate prognostic assessment after radical cystectomy that is essential for treatment decision-making, patient counseling, and most importantly for defining the indication of adjuvant chemotherapy (5).
The American Joint Committee on Cancer (AJCC) TNM staging system, which has been appropriately validated, is the most widely used prognostic model to predict outcome in patients treated with radical cystectomy (6). Although these staging systems provide useful estimates of clinical outcome, their major limitation is the difficulty of incorporating novel clinical information, such as molecular markers or more complex bioinformatics. Furthermore, current staging systems have been shown to be less accurate than some prediction models that incorporate several sets of clinical data in the era of personalized medicine (7). A recently reported, comprehensive molecular analysis of urothelial bladder cancer from the Cancer Genome Atlas (TCGA) (http://cancergenome.nih.gov/) has provided novel insights into molecular subgroups and potential therapeutic targets for this disease (8). A few studies on gene signatures associated with tumor characteristics and outcomes for MIBC had already been reported before the release of the TCGA database. We searched the PubMed database and found eight papers that summarize the gene signature regarding the prediction of overall survival (OS). However, as indicated in the paper by Riester et al. (9), the performance of these eight gene signatures regarding the OS of MIBC was not so robust, as most of their C-indexes were <0.70. Additionally, as the management of MIBC and chemotherapy has changed in recent decades, all the gene signatures need to be updated. Therefore, we planned a study to integrate all of the published genes with TCGA RNAseq data to develop a novel gene panel and to validate the panel in our own cohort by qRT-PCR.
We established a novel 12-gene signature using TCGA data that was well-validated in our cohort and shown to be superior to TNM staging. This signature improved the prediction of survival of MIBC patients when combined with conventional clinical data including gender, age, tumor T and N stages, and tumor grade. Our study has refined the gene signature of MIBC integrated with the RNAseq data of TCGA. These results might reveal new therapeutic targets for bladder cancer and may be helpful during consultations with patients to predict prognosis.
Patients and Methods
Selection of Published Studies
We searched EMBASE (www.embase.com) and MEDLINE (www.ncbi.nlm.nih.gov/pubmed) from their inception to December 2017 and systematically identified gene signature studies predicting the OS of MIBC. No language restrictions were applied. The search terms used were as follows: (“bladder cancer” OR “bladder neoplasm” OR “bladder tumor” OR “bladder urothelial carcinoma”) AND (“gene signature” OR “gene profile” OR “gene model” OR “molecular profile” OR “genomic profile” OR “gene expression”). Irrelevant studies were identified and excluded by scanning their titles and abstracts. The full text of the remaining articles was carefully reviewed to determine whether the articles contained information on the topic of interest. We also scanned the cited references of the retrieved articles and reviews to identify any additional relevant studies. Finally, we retrieved all of the gene panels relevant to MIBC and OS (Figure 1).
Patient Cohorts
Level 3 TCGA RNAseq data from bladder urothelial carcinoma (BLCA) samples were obtained from the TCGA data portal (https://genome-cancer.ucsc.edu/proj/site/hgHeatmap/). Tumor transcriptomic profiles of 20,534 genes were measured in 436 primary bladder cancer patients. Only the 327 patients with intact clinical information, especially follow-up data, were included in this study. The clinical information was retrieved from the “Clinical Biotab” section of the data matrix based on the Biospecimen Core Resource (BCR) identification numbers of the patients. Extended demographic parameters for these patients, characterized by TCGA consortium, are shown in Table 1.
The Fudan University Shanghai Cancer Center (FUSCC) validation cohort consisted of 172 patients with urothelial bladder cancer that was histologically confirmed by an experienced pathologist and treated by radical cystectomy without any pretreatment. These patients were consecutively enrolled from 2008 to 2015 (shown in Table 1). Once resected, tumor tissues were frozen and stored at −80°C. Written informed consent was obtained from all participants of this study. The study protocol was approved by the institutional review board of FUSCC and was carried out in accordance with the approved guidelines (approval ID: 050432-4-1805C).
The GEO dataset GSE13507 was downloaded from the website: www.ncbi.nlm.nih.gov/geo. RNA expression data and metadata of 61 patients were used for external validation of the gene signature. OS data were used for prognosis prediction.
RNA Preparation, cDNA Synthesis, and qRT-PCR Validation
Total RNA from frozen tissue specimens was extracted using TRIzol reagent (Invitrogen, Carlsbad, CA, USA), in accordance with the manufacturer's instructions. RNA quantity and quality were determined using a NanoDrop ND-1000 Spectrophotometer (NanoDrop Technologies, Wilmington, DE, USA). mRNA levels were measured using a RevertAid First Strand cDNA Synthesis Kit (K1622; Thermo Fisher Scientific, Waltham, MA, USA) and qRT-PCR Kit (Invitrogen, Carlsbad, CA, USA). ACTB (β-actin) served as an endogenous control. Primer sets are listed in Supplementary Table 1. qRT-PCR was performed on the Applied Biosystems 7,900 Real-Time PCR system using SYBR Green dye (Applied Biosystems, Foster City, CA, USA), as described by the manufacturer. All determinations were performed in triplicate and in at least three independent experiments. The mean Ct value of each gene minus the mean Ct value of ACTB was calculated as ΔCt. The –ΔCt value of each gene was applied for binary logistic regression and model construction. The details of this experiment are shown in our previous paper (10).
Statistical Analysis
All of the statistical analyses, including gene selection, classification model construction, and independent testing, were performed with R software and packages from the RMS and Bioconductor project (11, 12). For the data obtained by qRT-PCR, univariate and multivariate Cox regression models were used for the selection of genes for the predictive gene signature. All significance tests were two-sided, and a P < 0.05 was considered significant. Area under the ROC curve (AUC) was used as an accuracy index to identify the best combination of multiple markers.
Results
Acquiring Gene Signatures Associated With the OS of MIBC From Literature Analysis
Two reviewers (H.X. and F.W.) conducted the literature search independently. This resulted in the identification of eight studies (9, 13–19) that met our requirements; all genes included in these studies are featured in our candidate signature gene list after de-replication. All eight studies and the 274 genes are shown in Supplementary Tables 2, 3, respectively.
Gene List Discovery Using TCGA Database
In the TCGA cohort, we included 239 male and 88 female patients with a median age of 69 years, ranging from 38 to 90 years. Two-thirds of patients were AJCC stage III and IV patients. Since the gene names in the gene models from the different reports were not consistent, we unified the IDs of all 274 genes into their official gene names to find the corresponding genes in the full TCGA gene list. OS data were retrieved from the TCGA cohort and univariate Cox regression was performed to identify the prognostic value of the 274 genes (Supplementary Table 4). In the univariate analysis, 70 genes reached significance at P < 0.05. We then performed a reduced model of multivariate Cox regression in the TCGA cohort. The results showed that 12 genes (ATIC, C6orf62, CPA4, CYFIP2, EGFR, EHBP1, GRK3, MARCH7, QPRT, SARDH, SUZ12, and YIF1A) were factors independently associated with OS (Tables 2, 3).
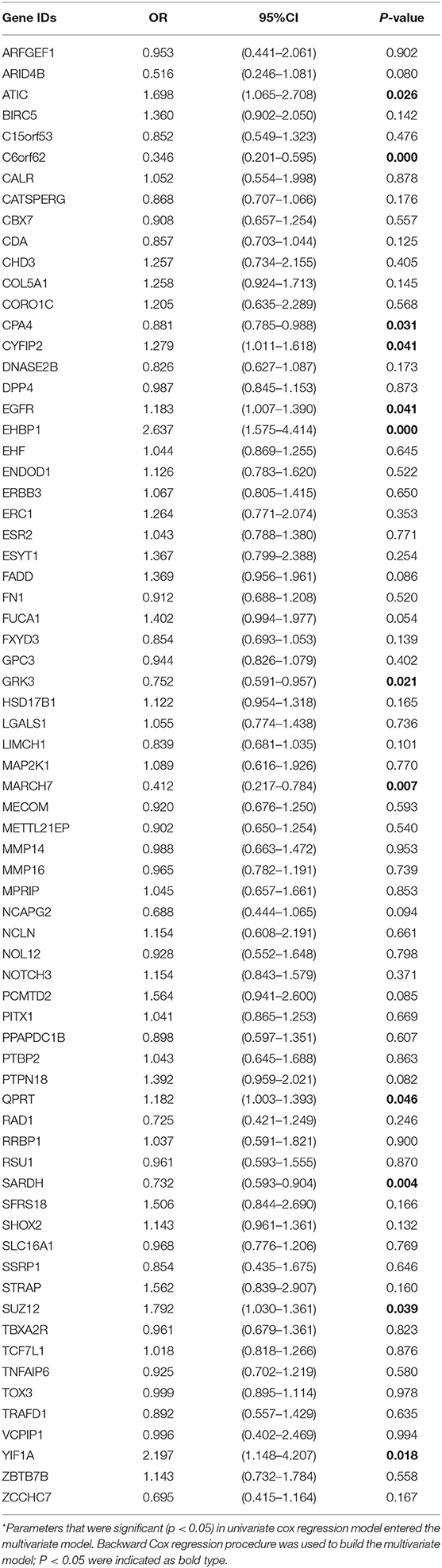
Table 2. Multivariate Cox hazard ratio regression model of integrated gene list in TCGA BLCA cohort.
Validation of the Integrated Gene Signature in the FUSCC Cohort
The FUSCC cohort included 147 male and 25 female patients who underwent cystectomy. The patient age ranged from 31 to 87 years old, with a median of 63 years. We used qRT-PCR to validate all 12 genes in the FUSCC cohort using fresh frozen tissues obtained from cystectomy. The ΔCt value of each gene was normalized by the β-actin Ct value. All 12 genes were significant in the univariate model (all P < 0.05, Table 4). In the multivariate Cox regression model, EHBP1 and SARDH were independent prognostic factors.
Integrated Gene Signature and Validation Using GEO Database
RNA expression data and metadata of 61 MIBC patients from GSE13507 were used for external validation of the gene signature. This cohort included 48 male patients and 13 female patients; the median age was 66 years old with a range from 38 to 87 years. All 12 genes were significant in univariate analysis. C6orf62 and ATIC were independent prognosis factors in the multivariate Cox regression model.
The 12-gene Signature in MIBC Improved the Predictive Value of the Clinical Model
To further assess the prognostic power of the 12-gene signature, we compared this model with a clinical model including gender, age, T stage, tumor grade, and N stage. We used “rms” package in R project to calculate the C-index values of the multivariate cox regression models. The results are shown in Table 5. In TCGA and FUSCC, the 12-gene signature was more accurate than the clinical model with a higher C-index. We then enrolled all the clinical and gene parameters together in a multivariate cox regression model for a combining model. The C-index reached 0.768, 0.757, and 0.88 in the TCGA, FUSCC and GSE13507 cohort, respectively. These results are shown in Table 5.
Discussion
Once diagnosed, the survival of MIBC patients can range from 1 week to a few years. The disease progression is dependent on risk factors such as tobacco smoking history, exposure to chemicals, radiotherapy, chronic urinary infection, gender, and genetic differences. These clinical criteria may not reflect the entire biology of the disease. In this study, we investigated the efficacy of the 12-gene panel to predict the survival of MIBC patients. Although, previous studies have already developed several gene signatures for predicting OS of MIBC, the management of bladder cancer has improved over decades and the models need to be updated as well. An effective gene signature will improve patient counseling after cystectomy and can better identify candidates who need more aggressive management.
In our study, we performed a meta-analysis to systematically review the literature on gene models and attempted to integrate them together with a relatively newly established public cohort. The updated and integrated novel model should be more applicable to recently treated patients. This approach is relatively novel and has not yet been widely used. In this pilot study, gene expression data from the public TCGA cohort of patients with MIBC were analyzed and external validations were performed using the cohort at our center and GEO datasets. Additionally, we randomly selected 5 genes from the 274 genes, and found the predictive superiority of our 12-gene panel (Supplementary Table 5).
Leliveld et al. reported that pathological TNM stage and age were independent prognosis factors for patients with MIBC who underwent radical cystectomy (20). Jin S et al. analyzed lymph node-associated variables [pathological N stage (pN), lymph node ratio (LNR) and log odds (LODDDS)] in the patients with MIBC and found c-index that predicted the survival was 0.6769 (pN), 0.6794 (pN+ LNR), and 0.6855 (pN+LNR+LODDDS) (21). Mitra et al. established clinical and genomic classifiers and the c-index was up to 0.73 (18). However, the c-index of the combination model in our study was 0.88. Thus, our update improved the survival prediction. Compared with conventional clinical data, the genomic-clinicopathologic combination in our study had higher clinical benefit by decision curve analysis (Figure 2). These findings are particularly striking given the relative homogeneity of the population analyzed, as our cohort, in which all patients undergone the most aggressive surgical therapy, was strongly selected for being at high risk of death from disease.
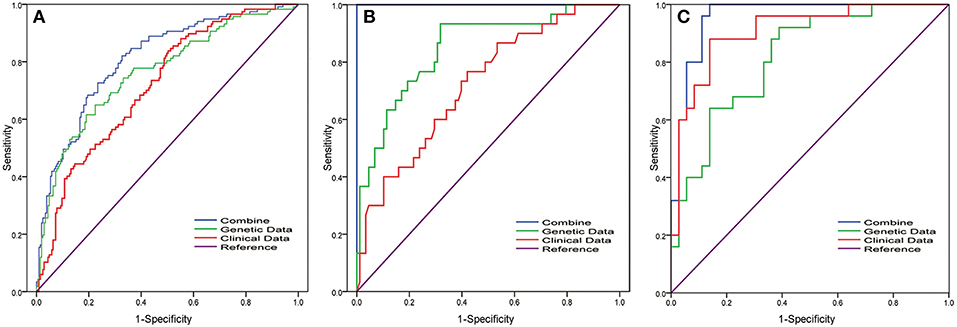
Figure 2. Receiver operating characteristic (ROC) curve analysis of the 12-gene signature in MIBC patients for predicting 5-years overall survival. To compare the prognostic value of the 12-gene signature, we analyzed the ROC curves of the 12-gene signature of 5-years OS in different datasets. ROC plots for the 12-gene signature predicting 5-years OS in the (A) TCGA cohort and (B) FUSCC cohort and (C) GSE13507 dataset. Clinical parameters include gender, age, T stage, grade, and N stage. Combining model was obtained by multivariate regression analysis for the combination of clinical parameter and 12-gene signature.
Among the members of the novel gene panel, C6orf62 may participate in suppressing proliferation and inducing differentiation through regulating the cell cycle (22). YIF1A is involved in the pathway of the transport of proteins to the Golgi and their subsequent modification, as well as the unfolded protein response. Discrete sites in Yif1A that are necessary for the regulation of endoplasmic reticulum structure have also been identified (23). Moreover, in samples from clinical squamous cell cancer, six genes (GAL, GSTP1, MRPL11, MRPL21, SF3B2, and YIF1A) at 11q13.1–13.3 and one gene (GALR1) at 18q23 showed significant differences in expression between normal and tumor samples (24).
Another member of the gene panel is ADRBK2, which encodes the β-adrenergic receptor kinase, a direct target of CREB activation that regulates the neuroendocrine differentiation of prostate cancer cells (25). This kinase is essential for cell metastasis, promotes prostate tumor progression (26), and regulates breast cancer migration, invasion, and metastasis (27). Another gene panel member is CYFIP2, which is involved in T-cell adhesion and p53/TP53-dependent induction of apoptosis. IMP-1 displays cross-talk with K-Ras and modulates colon cancer cell survival through this novel proapoptotic protein (28).
Among the other members of the gene panel, ATIC promotes insulin receptor/INSR autophosphorylation and is involved in INSR internalization (29). The small-molecule inhibitor of ATIC has been shown to suppress the proliferation of breast cancer cells (30). QPRT, which encodes a key enzyme in the catabolism of quinolinate, an intermediate in the tryptophan-nicotinamide adenine dinucleotide pathway, is a potential marker for follicular thyroid carcinoma including the minimally invasive variant (31). EHBP1 encodes a protein that may play a role in endocytic trafficking. The single nucleotide polymorphism rs721048(A>G) in EHBP1 is associated with an aggressive form of prostate cancer (32). EHBP1 is also essential for the anti-invasive effect of atorvastatin in prostate cancer (33).
The panel also includes MARCH7, which is a member of the MARCH family of membrane-bound E3 ubiquitin ligases. E3 ubiquitin ligases accept ubiquitin from an E2 ubiquitin-conjugating enzyme in the form of a thioester and then directly transfer the ubiquitin to targeted substrates. MARCH7 promotes ovarian tumor growth and its expression is correlated with poor prognosis in epithelial ovarian cancer (34, 35). CPA4 is also included in the panel and may be involved in the histone hyperacetylation pathway. CPA4 is imprinted and may be a strong candidate gene for the aggressiveness of prostate cancer (36) as well as a promising diagnostic serum biomarker for both pancreatic cancer and non-small cell lung cancer (37, 38) and an adverse prognostic marker for gastric cancer, NSCLC, and colorectal cancer (37, 39, 40).
The gene panel member SARDH encodes an enzyme localized to the mitochondrial matrix that catalyzes the oxidative demethylation of sarcosine. TMEFF2 and SARDH cooperate to modulate one-carbon metabolism and the invasion of prostate cancer cells (41). Another gene in this list is SUZ12, which is associated with diseases including endometrial stromal sarcoma and endometrial stromal nodules. Among its related pathways are cellular senescence and chromatin organization. SUZ12 promotes proliferation and metastasis in many cancers, including gastric cancer (42), colorectal cancer (43), ovarian cancer (44), bladder cancer (45, 46), and NSCLC (47).
The gene encoding epidermal growth factor receptor (EGFR) is also included in the gene panel. EGFR is a receptor tyrosine kinase of the ErbB family. Several studies have shown that the EGFR family of RTKs is involved in urothelial carcinoma progression and chemoresistance. Many clinical trials using inhibitors of EGFR family RTKs have also been performed or are underway (48).
Although it is lack of novelty and function work in our study and our results require further investigation of the efficacy of the 12-gene signature panel in patients, this panel could be extremely beneficial to identify patients at elevated risk of death that may require adjuvant therapy.
Conclusion
By applying published gene signatures and TCGA data, we successfully built and externally validated a novel 12-gene signature for the survival of MIBC. This model was generated by integration and updating of the existing model. The model improved the prediction of disease progression or survival and may help facilitate doctor-patient consultations and eventually benefit patients.
Data Availability
All datasets generated for this study are included in the manuscript and/or the Supplementary Files.
Ethics Statement
This study protocol was approved by the Institutional Review Board of FUSCC and was carried out in accordance with the approved guidelines (approval ID: 050432-4-1805C). As the data (TCGA and GEO datasets) are publicly available, no ethical approval is required.
Author Contributions
FW, YS, and DY had full access to all the data in the study and take responsibility for the integrity of the data and the accuracy of the data analysis, study concept, design, and supervision. MA and HX acquisition of data and drafting of the manuscript. ZJ, YaZ, and YiZ analysis and interpretation of data. GS, HZ, and BD critical revision of the manuscript for important intellectual content. FW and MA statistical analysis. FW and DY obtaining funding. YaZ and FW administrative, technical, or material support.
Conflict of Interest Statement
The authors declare that the research was conducted in the absence of any commercial or financial relationships that could be construed as a potential conflict of interest.
Acknowledgments
This study was sponsored by the National Natural Science Foundation of China (No. 81472377; No. 81502192; No. 81672544; No. 81872099; No. 81772706; No. 81572531). This article has been accepted as a moderate poster in AUA (American Urological Association) Congress 2017 in Boston in May 2017. We thank Liwen Bianji, Edanz Editing China (www.liwenbianji.cn/ac), for editing the English text of a draft of this manuscript.
Supplementary Material
The Supplementary Material for this article can be found online at: https://www.frontiersin.org/articles/10.3389/fonc.2019.00856/full#supplementary-material
References
1. Siegel RL, Miller KD, Jemal A. Cancer statistics, 2015. CA Cancer J Clin. (2015) 65:5–29. doi: 10.3322/caac.21254
2. Chen W, Zheng R, Baade PD, Zhang S, Zeng H, Bray F, et al. Cancer statistics in China, 2015. CA Cancer J Clin. (2016) 66:115–32. doi: 10.3322/caac.21338
3. Jacobs BL, Lee CT, Montie JE. Bladder cancer in 2010: how far have we come? CA Cancer J Clin. (2010) 60:244–72. doi: 10.3322/caac.20077
4. Kaufman DS, Shipley WU, Feldman AS. Bladder cancer. Lancet. (2009) 374:239–49. doi: 10.1016/S0140-6736(09)60491-8
5. Leow JJ, Martin-Doyle W, Rajagopal PS, Patel CG, Anderson EM, Rothman AT, et al. Adjuvant chemotherapy for invasive bladder cancer: a 2013 updated systematic review and meta-analysis of randomized trials. Eur Urol. (2014) 66:42–54. doi: 10.1016/j.eururo.2013.08.033
6. Edge SB, Compton CC. The American Joint Committee on Cancer: the 7th edition of the AJCC cancer staging manual and the future of TNM. Ann Surg Oncol. (2010) 17:1471–4. doi: 10.1245/s10434-010-0985-4
7. Kluth LA, Black PC, Bochner BH, Catto J, Lerner SP, Stenzl A, et al. Prognostic and prediction tools in bladder cancer: a comprehensive review of the literature. Eur Urol. (2015) 68:238–53. doi: 10.1016/j.eururo.2015.01.032
8. Cancer Genome Atlas Research N. Comprehensive molecular characterization of urothelial bladder carcinoma. Nature. (2014) 507:315–22. doi: 10.1038/nature12965
9. Riester M, Taylor JM, Feifer A, Koppie T, Rosenberg JE, Downey RJ, et al. Combination of a novel gene expression signature with a clinical nomogram improves the prediction of survival in high-risk bladder cancer. Clin Cancer Res. (2012) 18:1323–33. doi: 10.1158/1078-0432.CCR-11-2271
10. Wan F, Zhu Y, Han C, Xu Q, Wu J, Dai B, et al. Identification and validation of an 8-gene expression signature for predicting high Fuhrman grade renal cell carcinoma. Int J Cancer. (2016) 140:1199–208. doi: 10.1002/ijc.30535
11. Reimers M, Carey VJ. Bioconductor: an open source framework for bioinformatics and computational biology. Methods Enzymol. (2006) 411:119–34. doi: 10.1016/S0076-6879(06)11008-3
12. Ihaka R, Gentlemen R. R: a language for data analysis and graphics. J Comput Graph Stat. (1996) 5:299–314. doi: 10.1080/10618600.1996.10474713
13. Smith SC, Baras AS, Dancik G, Ru Y, Ding KF, Moskaluk CA, et al. A 20-gene model for molecular nodal staging of bladder cancer: development and prospective assessment. Lancet Oncol. (2011) 12:137–43. doi: 10.1016/S1470-2045(10)70296-5
14. Lindgren D, Frigyesi A, Gudjonsson S, Sjodahl G, Hallden C, Chebil G, et al. Combined gene expression and genomic profiling define two intrinsic molecular subtypes of urothelial carcinoma and gene signatures for molecular grading and outcome. Cancer Res. (2010) 70:3463–72. doi: 10.1158/0008-5472.CAN-09-4213
15. Kim WJ, Kim EJ, Kim SK, Kim YJ, Ha YS, Jeong P, et al. Predictive value of progression-related gene classifier in primary non-muscle invasive bladder cancer. Mol Cancer. (2010) 9:3. doi: 10.1186/1476-4598-9-3
16. Mitra AP, Pagliarulo V, Yang D, Waldman FM, Datar RH, Skinner DG, et al. Generation of a concise gene panel for outcome prediction in urinary bladder cancer. J Clin Oncol. (2009) 27:3929–37. doi: 10.1200/JCO.2008.18.5744
17. Sanchez-Carbayo M, Socci ND, Lozano J, Saint F, Cordon-Cardo C. Defining molecular profiles of poor outcome in patients with invasive bladder cancer using oligonucleotide microarrays. J Clin Oncol. (2006) 24:778–89. doi: 10.1200/JCO.2005.03.2375
18. Mitra AP, Lam LL, Ghadessi M, Erho N, Vergara IA, Alshalalfa M, et al. Discovery and validation of novel expression signature for postcystectomy recurrence in high-risk bladder cancer. J Natl Cancer Inst. (2014) 106:dju290. doi: 10.1093/jnci/dju290
19. Als AB, Dyrskjot L, von der Maase H, Koed K, Mansilla F, Toldbod HE, et al. Emmprin and survivin predict response and survival following cisplatin-containing chemotherapy in patients with advanced bladder cancer. Clin Cancer Res. (2007) 13(15 Pt 1):4407–14. doi: 10.1158/1078-0432.CCR-07-0109
20. Leliveld AM, Doornweerd BH, Bastiaannet E, Schaapveld M, de Jong IJ. Treatment and outcome in muscle invasive bladder cancer: a population-based survey. World J Urol. (2010) 28:439–44. doi: 10.1007/s00345-010-0546-2
21. Jin S, Wang B, Zhu Y, Dai W, Xu P, Yang C, et al. Log odds could better predict survival in muscle-invasive bladder cancer patients compared with pN and lymph node ratio. J Cancer. (2019) 10:249–56. doi: 10.7150/jca.27399
22. Yoo HN. Expression of C6orf62 in Human Embryonic Stem Cells and Cancer Cells. Reproductive & Developmental Biology. CHA University, Seongnam (2010).
23. Dykstra KM, Ulengin I, Delrose N, Lee TH. Identification of discrete sites in Yip1A necessary for regulation of endoplasmic reticulum structure. PLoS ONE. (2013) 8:e54413. doi: 10.1371/journal.pone.0054413
24. Sugimoto T, Seki N, Shimizu S, Kikkawa N, Tsukada J, Shimada H, et al. The galanin signaling cascade is a candidate pathway regulating oncogenesis in human squamous cell carcinoma. Genes Chrom Cancer. (2009) 48:132–42. doi: 10.1002/gcc.20626
25. Sang M, Hulsurkar M, Zhang X, Song H, Zheng D, Zhang Y, et al. GRK3 is a direct target of CREB activation and regulates neuroendocrine differentiation of prostate cancer cells. Oncotarget. (2016) 7:45171–85. doi: 10.18632/oncotarget.9359
26. Li W, Ai N, Wang S, Bhattacharya N, Vrbanac V, Collins M, et al. GRK3 is essential for metastatic cells and promotes prostate tumor progression. Proc Natl Acad Sci USA. (2014) 111:1521–6. doi: 10.1073/pnas.1320638111
27. Billard MJ, Fitzhugh DJ, Parker JS, Brozowski JM, McGinnis MW, Timoshchenko RG, et al. G protein coupled receptor kinase 3 regulates breast cancer migration, invasion, and metastasis. PLoS ONE. (2016) 11:e0152856. doi: 10.1371/journal.pone.0152856
28. Mongroo PS, Noubissi FK, Cuatrecasas M, Kalabis J, King CE, Johnstone CN, et al. IMP-1 displays cross-talk with K-Ras and modulates colon cancer cell survival through the novel proapoptotic protein CYFIP2. Cancer Res. (2011) 71:2172–82. doi: 10.1158/0008-5472.CAN-10-3295
29. Boutchueng-Djidjou M, Collard-Simard G, Fortier S, Hebert SS, Kelly I, Landry CR, et al. The last enzyme of the de novo purine synthesis pathway 5-aminoimidazole-4-carboxamide ribonucleotide formyltransferase/IMP cyclohydrolase (ATIC) plays a central role in insulin signaling and the Golgi/endosomes protein network. Mol Cell Proteom. (2015) 14:1079–92. doi: 10.1074/mcp.M114.047159
30. Spurr IB, Birts CN, Cuda F, Benkovic SJ, Blaydes JP, Tavassoli A. Targeting tumour proliferation with a small-molecule inhibitor of AICAR transformylase homodimerization. Chembiochem. (2012) 13:1628–34. doi: 10.1002/cbic.201200279
31. Hinsch N, Frank M, Doring C, Vorlander C, Hansmann ML. QPRT: a potential marker for follicular thyroid carcinoma including minimal invasive variant; a gene expression, RNA and immunohistochemical study. BMC Cancer. (2009) 9:93. doi: 10.1186/1471-2407-9-93
32. Ao X, Liu Y, Bai XY, Qu X, Xu Z, Hu G, et al. Association between EHBP1 rs721048(A>G) polymorphism and prostate cancer susceptibility: a meta-analysis of 17 studies involving 150,678 subjects. OncoTargets Therapy. (2015) 8:1671–80. doi: 10.2147/OTT.S84034
33. Ghalali A, Wiklund F, Zheng H, Stenius U, Hogberg J. Atorvastatin prevents ATP-driven invasiveness via P2X7 and EHBP1 signaling in PTEN-expressing prostate cancer cells. Carcinogenesis. (2014) 35:1547–55. doi: 10.1093/carcin/bgu019
34. Zhang L, Wang H, Tian L, Li H. Expression of USP7 and MARCH7 is correlated with poor prognosis in epithelial ovarian cancer. Tohoku J Exp Med. (2016) 239:165–75. doi: 10.1620/tjem.239.165
35. Hu J, Meng Y, Yu T, Hu L, Mao M. Ubiquitin E3 ligase MARCH7 promotes ovarian tumor growth. Oncotarget. (2015) 6:12174–87. doi: 10.18632/oncotarget.3650
36. Ross PL, Cheng I, Liu X, Cicek MS, Carroll PR, Casey G, et al. Carboxypeptidase 4 gene variants and early-onset intermediate-to-high risk prostate cancer. BMC Cancer. (2009) 9:69. doi: 10.1186/1471-2407-9-69
37. Sun L, Wang Y, Yuan H, Burnett J, Pan J, Yang Z, et al. CPA4 is a novel diagnostic and prognostic marker for human non-small-cell lung cancer. J Cancer. (2016) 7:1197–204. doi: 10.7150/jca.15209
38. Sun L, Burnett J, Guo C, Xie Y, Pan J, Yang Z, et al. CPA4 is a promising diagnostic serum biomarker for pancreatic cancer. Am J Cancer Res. (2016) 6:91–6.
39. Sun L, Guo C, Yuan H, Burnett J, Pan J, Yang Z, et al. Overexpression of carboxypeptidase A4 (CPA4) is associated with poor prognosis in patients with gastric cancer. Am J Transl Res. (2016) 8:5071–5.
40. Sun L, Guo C, Burnett J, Yang Z, Ran Y, Sun D. Serum carboxypeptidaseA4 levels predict liver metastasis in colorectal carcinoma. Oncotarget. (2016) 7:78688–97. doi: 10.18632/oncotarget.12798
41. Green T, Chen X, Ryan S, Asch AS, Ruiz-Echevarria MJ. TMEFF2 and SARDH cooperate to modulate one-carbon metabolism and invasion of prostate cancer cells. Prostate. (2013) 73:1561–75. doi: 10.1002/pros.22706
42. Xia R, Jin FY, Lu K, Wan L, Xie M, Xu TP, et al. SUZ12 promotes gastric cancer cell proliferation and metastasis by regulating KLF2 and E-cadherin. Tumour Biol. (2015) 36:5341–51. doi: 10.1007/s13277-015-3195-7
43. Liu YL, Gao X, Jiang Y, Zhang G, Sun ZC, Cui BB, et al. Expression and clinicopathological significance of EED, SUZ12 and EZH2 mRNA in colorectal cancer. J Cancer Res Clin Oncol. (2015) 141:661–9. doi: 10.1007/s00432-014-1854-5
44. Li H, Cai Q, Wu H, Vathipadiekal V, Dobbin ZC, Li T, et al. SUZ12 promotes human epithelial ovarian cancer by suppressing apoptosis via silencing HRK. Mol Cancer Res. (2012) 10:1462–72. doi: 10.1158/1541-7786.MCR-12-0335
45. Lee SR, Roh YG, Kim SK, Lee JS, Seol SY, Lee HH, et al. Activation of EZH2 and SUZ12 regulated by E2F1 predicts the disease progression and aggressive characteristics of bladder cancer. Clin Cancer Res. (2015) 21:5391–403. doi: 10.1158/1078-0432.CCR-14-2680
46. Fan Y, Shen B, Tan M, Mu X, Qin Y, Zhang F, et al. TGF-beta-induced upregulation of malat1 promotes bladder cancer metastasis by associating with suz12. Clin Cancer Res. (2014) 20:1531–41. doi: 10.1158/1078-0432.CCR-13-1455
47. Liu C, Shi X, Wang L, Wu Y, Jin F, Bai C, et al. SUZ12 is involved in progression of non-small cell lung cancer by promoting cell proliferation and metastasis. Tumour Biol. (2014) 35:6073–82. doi: 10.1007/s13277-014-1804-5
Keywords: muscle-invasive bladder cancer, gene signature, overall survival, prognosis, TCGA
Citation: Abudurexiti M, Xie H, Jia Z, Zhu Y, Zhu Y, Shi G, Zhang H, Dai B, Wan F, Shen Y and Ye D (2019) Development and External Validation of a Novel 12-Gene Signature for Prediction of Overall Survival in Muscle-Invasive Bladder Cancer. Front. Oncol. 9:856. doi: 10.3389/fonc.2019.00856
Received: 17 April 2019; Accepted: 19 August 2019;
Published: 06 September 2019.
Edited by:
Ingrid A. Hedenfalk, Lund University, SwedenReviewed by:
Jeongsik Yong, University of Minnesota Twin Cities, United StatesJie Tan, The Johns Hopkins Hospital, Johns Hopkins Medicine, United States
Copyright © 2019 Abudurexiti, Xie, Jia, Zhu, Zhu, Shi, Zhang, Dai, Wan, Shen and Ye. This is an open-access article distributed under the terms of the Creative Commons Attribution License (CC BY). The use, distribution or reproduction in other forums is permitted, provided the original author(s) and the copyright owner(s) are credited and that the original publication in this journal is cited, in accordance with accepted academic practice. No use, distribution or reproduction is permitted which does not comply with these terms.
*Correspondence: Fangning Wan, Zm53YW4wNiYjeDAwMDQwO2Z1ZGFuLmVkdS5jbg==; Yijun Shen, bHVja3lzeWomI3gwMDA0MDtnbWFpbC5jb20=; Dingwei Ye, ZHd5ZWxpJiN4MDAwNDA7MTYzLmNvbQ==
†These authors have contributed equally to this work