- 1Science for Life Laboratory, KTH - Royal Institute of Technology, Stockholm, Sweden
- 2Department of Medical Genomics, King Abdullah International Medical Research Center, Riyadh, Saudi Arabia
- 3King Saud Bin Abdul Aziz University for Health Sciences, Riyadh, Saudi Arabia
- 4Ministry of the National Guard- Health Affairs, Riyadh, Saudi Arabia
- 5Department of Biology and Biological Engineering, Chalmers University of Technology, Gothenburg, Sweden
- 6Centre for Host–Microbiome Interactions, Dental Institute, King's College London, London, United Kingdom
- 7Colorectal Cancer Research Program, King Abdullah International Medical Research Center, Riyadh, Saudi Arabia
Colorectal cancer is the third most incidental cancer worldwide, and the response rate of current treatment for colorectal cancer is very low. Genome-scale metabolic models (GEMs) are systems biology platforms, and they had been used to assist researchers in understanding the metabolic alterations in different types of cancer. Here, we reconstructed a generic colorectal cancer GEM by merging 374 personalized GEMs from the Human Pathology Atlas and used it as a platform for systematic investigation of the difference between tumor and normal samples. The reconstructed model revealed the metabolic reprogramming in glutathione as well as the arginine and proline metabolism in response to tumor occurrence. In addition, six genes including ODC1, SMS, SRM, RRM2, SMOX, and SAT1 associated with arginine and proline metabolism were found to be key players in this metabolic alteration. We also investigated these genes in independent colorectal cancer patients and cell lines and found that many of these genes showed elevated level in colorectal cancer and exhibited adverse effect in patients. Therefore, these genes could be promising therapeutic targets for treatment of a specific colon cancer patient group.
Introduction
Colorectal cancer (CRC) is one of the most common malignant diseases. It has third highest incidence among all cancers worldwide. Due to better screening and early interventions, the mortality of CRC has been decreasing. However, the development of chemotherapy option has seen a marginal improvement. 5-Fluorouracil based chemotherapy is currently the first-line treatment against CRC (1), but the response rate of 5-Fluorouracil is only around 10–15% among patients (2). Several new additions in the form of oxaliplatin, irinotecan, capecitabine, cetuximab, and bevacizumab and other biologicals have become available mostly as combination therapies. The level of success anticipated with the targeted therapeutic agents failed on various parameters (3–7). Therefore, there is still great interest in development of effective treatment strategies for CRC.
While studying colorectal cancer patients locally in Saudi Arabia, we came across the heterogeneity in the somatic cytogenetic and transcriptomic level changes associated with this type of cancer. There were copy number changes that were reported earlier as well as novel events that characterized this patient population (8, 9). The transcription level changes suggest the involvement of different genes in initiation and progression of CRC in these same patients. An integrated analysis with data from cytogenetic and transcriptomic level measurement provided the gene targets that carry higher confidence value than either of them alone (10). The next level in the functional hierarchy is protein and metabolites which are the effector molecules that execute the assigned tasks. Understanding metabolite level changes related to colorectal cancer in an integrated manner would increase the confidence level in finding the biomarkers and therapeutic targets that can be accurate, precise, and clinically useful.
Colon cancer undergoes severe metabolic reprogramming during its transformation and systems biology tools can be employed for identification of the altered metabolic processes. To this end, the reconstruction of genome-scale metabolic models (GEMs) can help to knit high-throughput data (i.e., proteomics and transcriptomics) in the network topology and a variety of methods enable the researchers to predict how these transcriptional perturbations are translated into alterations in distinct and biologically meaningful metabolic subsystems (11–13). GEMs condense information about the known functions of protein-encoding genes, how these genes/proteins interact with other bioactive compounds and associated reactions, allowing robust and reliable analyses to be performed by high-quality bioinformatics pipelines and similar tools (14). These integrative models have been successfully employed to gain further biological and mechanistic understanding of metabolism related disorders (15, 16).
To date, different GEMs for cancer have been reconstructed to characterize the genetic mechanisms of cancer and to reveal how cancer cells benefit from metabolic modifications (17–21). In this report, we reconstructed generic GEM for colon cancer tumors by integrating personalized GEMs that are generated based on the Human Metabolic Reaction 2 (HMR2) database (11, 22) and transcriptomics data (RNA-Seq) in The Cancer Genome Atlas (TCGA). The reconstructed model has been used for the analysis of the gene expression data generated from colon cancer samples and matched normal samples of patients. These analyses revealed the key metabolic alteration around arginine and proline metabolism as well as glutathione metabolism.
Materials and Methods
Patient Samples and mRNA Expression Profiling
Patient sample collection and RNA extraction were performed as previously described (10). Briefly, 46 tumor samples and 44 adjacent normal samples were obtained after the requisite approval of Institutional Review Board at King Abdullah International Medical Research Center by King Abdul Aziz Medical City, Riyadh, Saudi Arabia. The samples were obtained from biopsies as well as surgical resections. All the patients signed the Institutional Review Board informed consent form prior to sample collection. All samples were immediately stored in the RNA later reagent until the extraction of nucleic acids. Methods mentioned herein were performed in accordance with the relevant guidelines and regulations. Homogenization was carried out using a QiaPrep homogenizer (Qiagen, Hilden, Germany) with stainless steel beads (5 mm). Total RNA was isolated using the Macherey-Nagel TripPrep kit (Macherey-Nagel Inc., Bethlehem, PA, USA) with <30 mg of tissue. Human Exon ST 1.0 arrays from Affymetrix (Santa Clara, CA, USA), containing probes set for 22,011 genes, were used as previously described together with the amplification and labeling kit from Ambion (Foster City, CA, USA).
All mRNA expression data has been deposited in the GEO database under the accession number GSE50421 and GSE77434 (90 samples: 44 normal samples and 46 cancer samples). Data were exported as CEL files (which contain data on the intensity of each signal, indicating the expression level of the gene corresponding to each probe) and processed using Expression Console software (Affymetrix). All data was subjected to quantile normalization using Integromics Omics office licensed software available at www.integromics.com. Fold change values for metabolic genes were obtained using Transcriptomics analysis console (Affymetrix, Thermofisher scientific, USA).
Animal Cell Culture
HCT8, HCT 116, SW480, and SW620 colorectal cancer cell lines were obtained from American Type Culture Collection, USA. All cell lines were maintained in 10% Fetal Calf Serum (Gibco) in Dulbecco's modified minimal eagle's media (DMEM) obtained from Thermo Fischer Scientific. Culture conditions were: 5% CO2 at 37°C in a humidified incubator. RNA extraction from these cell lines was done using Macherey-Nagel TripPrep kit (Macherey-Nagel Inc., Bethlehem, PA, USA) followed by cDNA conversion using kit (Roche).
Quantitative Real Time Polymerase Chain Reaction
Gene expression levels for the candidate genes were obtained from matched tumor-normal samples of 15 patients. A Taqman assay from Applied Biosystems (Thermo Fisher Scientific, USA) was used along with the Taqman master mix. 18S rRNA was used as housekeeping gene. All the samples were done in quadruplicate using ABI 7900 instrument. Gene expression analysis software from ABI was used to calculate fold change values using the ΔΔCt method (i.e., RQ = 2−ΔΔCt).
Gene Set Enrichment Analysis and Bioprofiler Analysis
The KEGG and GO enrichment analysis was done with PIANO (23) package in R. The gene-set collections for GO biological process and KEGG pathways was taken from MSigDB (24). GO and KEGG terms with FDR <5% in distinct-directionality class are considered as significantly changed. Distinct-directionality class in PIANO result is using the proportion between significantly up- and down-regulated genes from differential expression to define the statistical value and significance level. Bioprofiler analysis was carried out to know the causal relationship of the six identified genes with CRC. It was done using Ingenuity pathway analysis software (Qiagen biosciences).
Reconstruction of GEMs
The generic human colorectal cancer GEM was reconstructed based on unification of the 374 individual GEMs created in Human Pathology Atlas (downloaded from BioModels Database www.ebi.ac.uk/biomodels) to cover the all individual variations among colorectal cancer patients. The reporter metabolite analysis is performed using RAVEN toolbox implemented in Matlab 2017a.
Kaplan-Meier Analysis
The Kaplan-Meier survival analysis and the log-rank P-value is obtained from pathology atlas (21). We only included data from dead subjects for robustness, and the default best separation cutoff for each gene provided by pathology atlas is used to stratify the patients.
Results
Transcriptomic Comparison Between Colon Cancer Tumor and Normal Tissue
To investigate the transcriptomic changes in colon cancer tumor, we performed differential expression analysis using microarray data from 46 cancer samples and 44 normal samples. Consequently, we identified 2012 up-regulated genes and 1373 down-regulated (FDR < 0.05; Table S1), respectively. Among up-regulated genes, we found key glycolytic enzymes, PFKM, GPI, PGAM5, PKM, and LDHD, and these indicated an enhanced glycolytic process. This is a strong indication for Warburg effect, which is well-known to be present in colorectal cancer cells. We also found FASN, ACLY, ACACA, and SCD are significantly up-regulated, which indicated an increased fatty acid biosynthesis. It is clinically well-known that fatty acid synthesis is increased in colorectal cancer (25), and this could help the cancer cell to produce lipid metabolites crucial to cell membranes, cell signaling, post-translational modifications of proteins, neutralization of toxic reactive oxygen species, and energy storage (26). In addition, we found GLS2 is also significantly up-regulated, which indicated an increased uptake of glutamine and suggested increased glutaminolysis in the cancer cells.
We also performed GO term and KEGG pathway enrichment analysis for these differentially expressed genes (Figure 1; Tables S2, S3). The up-regulated genes were significantly enriched in cell cycle related pathways (cell cycle in KEGG and cell cycle check point in GO), and the down-regulated genes were significantly enriched in the immune related pathways (T cell receptor signaling pathway in KEGG and leukocyte activation in GO), which are expected in cancer and could be treated as good positive controls. In addition, down-regulated genes were also significantly enriched in fatty acid catabolic metabolism pathways in KEGG enrichment analyses, which might be related to normal colon function. Interestingly, we also found several metabolic pathways, for instance oxidative phosphorylation, branch amino acids metabolism, and tricarboxylic acid cycle (TCA) were enriched with down-regulated genes in colon cancer. This motivates further investigation in metabolic shift in colon tumors.
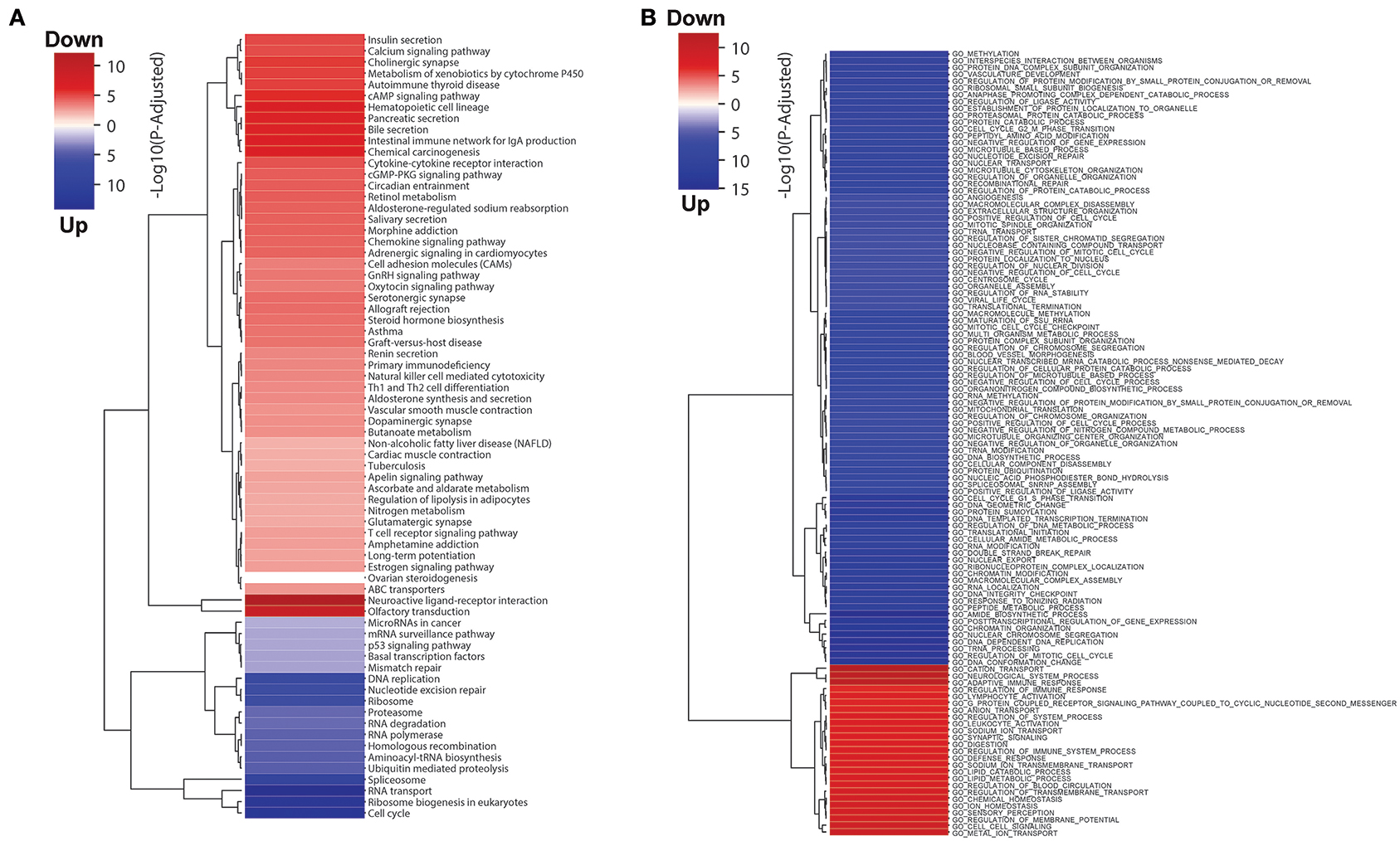
Figure 1. (A) KEGG pathway and (B) GO terms enrichment analysis results for differentially expressed genes in colon tumor. The blue and red colors represent up- and down-regulated in colon tumor compared to normal samples, and the intensity of the color indicates the minus log P-value.
Identification of Key Metabolic Pathways Using Functional GEMs
In order to study the metabolic reprogramming of the colorectal cancer patients accounting for the differences at the individual level, we integrated the personalized colorectal cancer GEMs and generated a generic GEM for colorectal cancer. This GEM includes 2,628 genes, 4,753 metabolites, and 6,716 biochemical reactions that happen in 8 different subcellular compartments. We compared the model with previously published healthy colon tissue model (27). We found that 467 genes, 681 metabolites, and 1,084 reactions are specific to colon cancer model and it is the largest colorectal cancer GEM.
We employed the GEM of colorectal cancer for the analysis of the RNA-Seq data using indigenously generated microarray data and identified the highly perturbed metabolites and modules between the two conditions using Reporter Metabolite and Reporter Subnetworks algorithms, respectively (28). Reporter Metabolite analysis would allow for the identification of metabolites in the network for which there is significant enrichment of associated gene expression changes and the Reporter Subnetwork algorithm would allow for the identification of a set of metabolic reactions that exhibit transcriptional correlation after a perturbation. These algorithms could provide valuable information on transcriptional regulation on changes in cell conditions and allowed for identification of metabolic reprogramming in colorectal cancer metabolism.
The reporter metabolites have been provided in Table S4, and several polyamines appeared as top significant reporter metabolites. We identified putrescine, spermidine, and N1-acetylspermidine are among the top up-regulated reporter metabolites in colon cancer. These are all polyamines and indicate an increased polyamine metabolic activity in colon cancer cells. Polyamines has reported to be natural anti-oxidants that could protect cells from oxidative damage (29). It has also been reported before that patients with malignant disease has higher urine polyamines level (30). Therefore, polyamines metabolism might play a key role in colon cancer. As shown in Figure 2, by mapping the differentially expressed genes and the reporter metabolites, we observed that the metabolic pathway from ornithine to spermine and spermidine is up-regulated in colon cancer cells. We found most of the key enzymes are significantly up-regulated. For instances, ODC1, SRM, and SMOX are significantly up-regulated, and the up-regulation of SAT1 is border line significant (FDR = 0.1). In addition, another key enzyme, SMS, is appeared in the reporter subnetwork analysis even though it is not annotated in the microarray data. These together suggested that polyamine metabolism is essential in colon cancer.
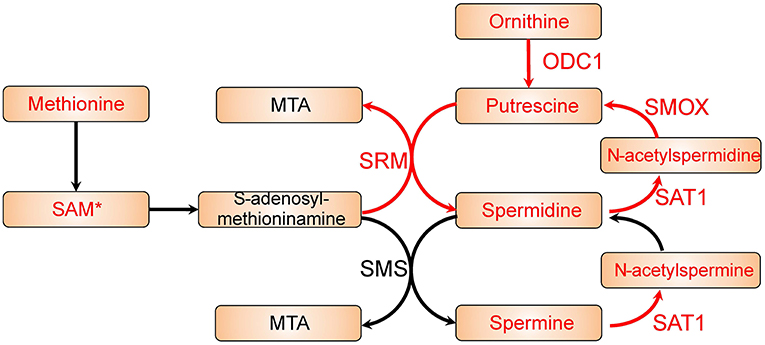
Figure 2. Polyamine metabolic pathway with differential expression genes and reporter metabolites highlighted. Metabolites are shown as bricks and reporter metabolites are highlighted in red. Arrows represent metabolic reactions, and the genes related to the reactions are annotated closely to the arrows. The genes in red are significantly up-regulated in the differential expression analysis, and the reporter metabolites and genes with a star are border line significant (FDR ~ 0.1).
Validation of Candidate Genes
From the results based on the reconstructed functional GEMs, six genes (ODC1, SMS, SRM, RRM2, SMOX, and SAT1) were identified to be playing significant role in causing metabolic changes. The expression level of five of these genes as deduced from patient gene expression microarray data is given in Table 1. For SMS gene we couldn't find the correct annotation in the microarray results. We validated the expression levels of these genes in a subset of 15 patients as well as four colorectal cancer cell lines. While we observed a general pattern exhibiting upregulation of ODC1, RRM2, SAT1, SMOX, SMS, and SRM genes (Figure 3A), heterogeneity among the patient group was conspicuous (Figure 3B). In addition, we also investigated the prognostic roles of these genes in TCGA colorectal cancer cohort and obtained the log-rank P-values from Pathology Atlas (21). As shown in Figure 4, we found higher expression of all six genes to be associated with adverse effect on patients. Among the six genes, the adverse effects of ODC1, SRM, SMOX, and SAT1 exhibited statistical significance (log-rank P < 0.05). This further confirms their key roles in colorectal cancer. As shown in Figure 5, in confirmation with patient sample data, these six genes showed similar expression changes in cell lines. For instance, the prognostic genes ODC1 and SMOX showed upregulation in HCT8, HCT116, SW480, and SW620 cell lines. In addition, SRM was up-regulated in three cell lines, and stayed unchanged in HCT116. SAT1 exhibited downregulation only in SW480 cell line. On the other hand, SMS and RRM2 exhibited heterogeneous expression pattern in cancer cell lines, which is in agreement to the survival analysis results. All values of gene expression fold changes in patients as well as cell lines are given in supplementary data Table S5. In summary, the roles of ODC1, SMOX, SRM, and SAT seems more important and conserved according to both in vivo and in vitro analysis.
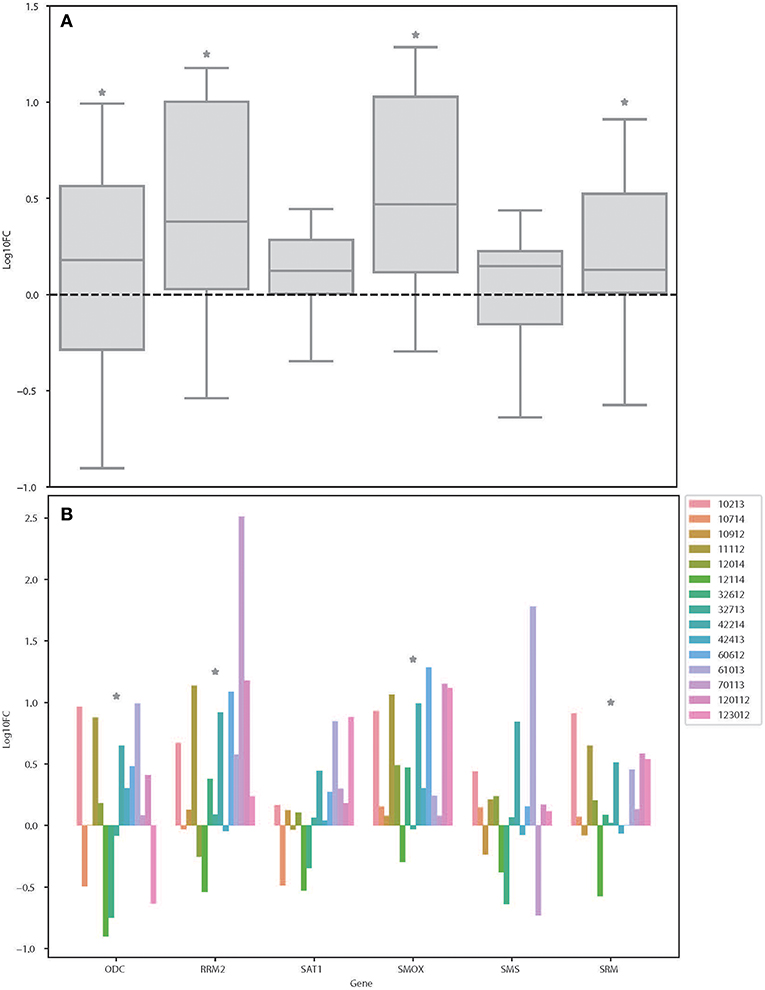
Figure 3. Expression changes of ODC, RRM2, SAT1, SMOX, SMS, and SRM in colorectal cancer patients. (A) Boxplot showing the log fold changes of the indicated genes among the patients. (B) Bar plot showing the log fold changes of each gene in each patient. *Denotes the genes that is significantly differentially expressed between colon cancer and normal samples as shown in Table S1 (FDR < 0.05).
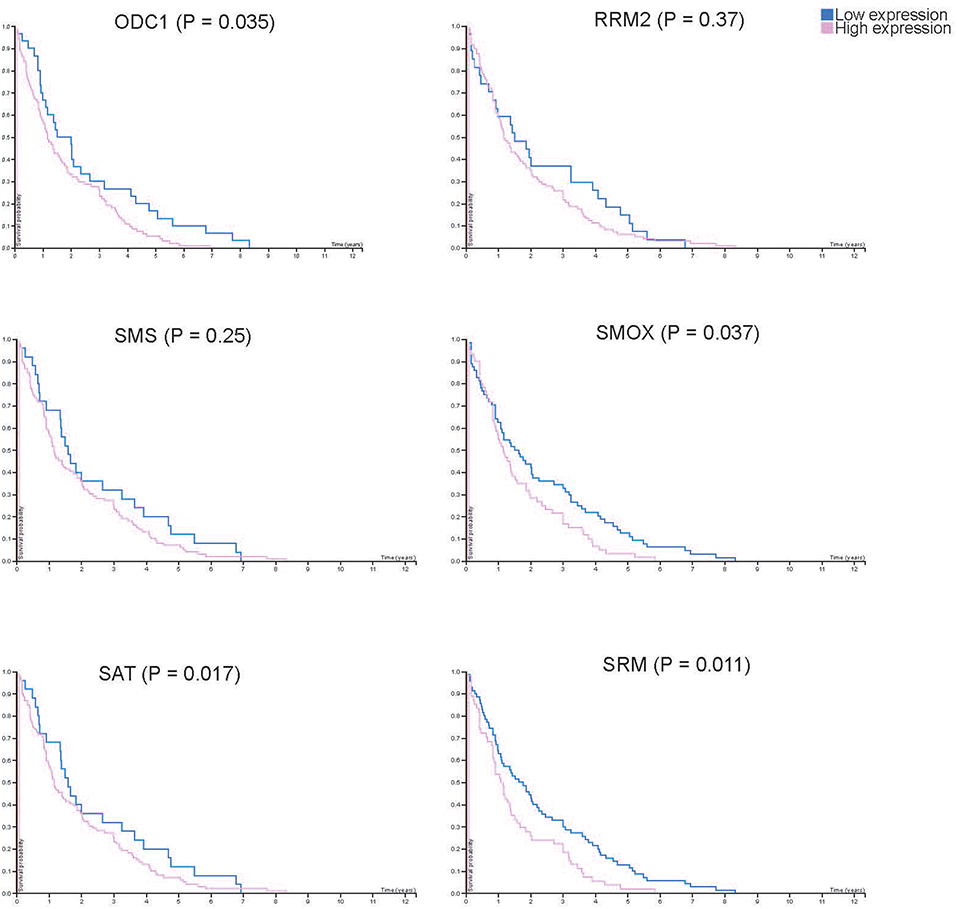
Figure 4. Kaplan-Meier plots showing the survival difference between patient groups with high and low RNA expression of each key gene. Blue and pink lines show the survival of patient group with low and high key gene expression, respectively.
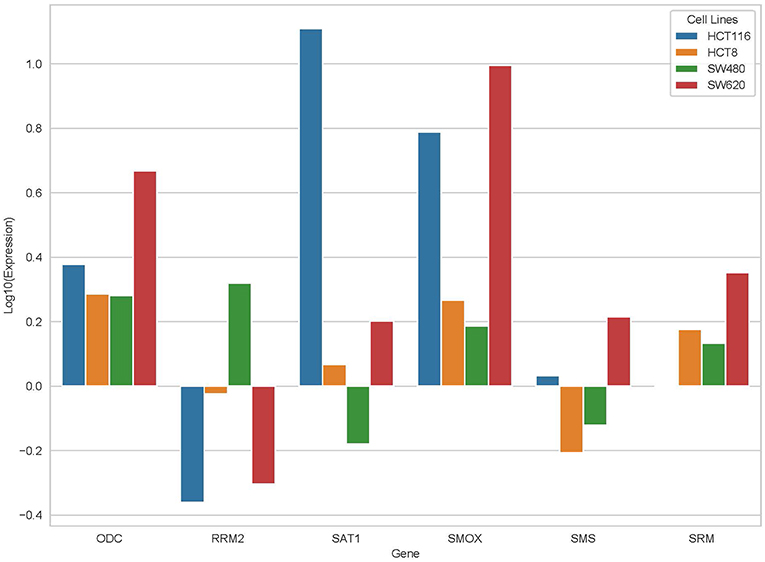
Figure 5. Expression changes of ODC, RRM2, SAT1, SMOX, SMS, and SRM in four cancer cell lines, HCT116, HCT8, SW480, and SW620.
We also conducted the bioprofiler analysis of the six candidate genes using Ingenuity pathway analysis software. These analyses reveal the unknown effect of SMOX, SMS, and SRM genes in colorectal cancer. Other genes including ODC1 were found to affect colorectal cancer but no causal relationship has been reported so far (Table S6).
Discussion
In this report, we have attempted to build genome scale metabolic model for colorectal cancer and identified key metabolic pathways altered in a local patient cohort. Further, we found six genes that could be responsible for these metabolic changes and studied their functional relevance in association with colorectal cancer. Finally, we assessed the prognostic significance of these genes.
Integrated models have recently been successfully used to identify driver genes for colorectal cancer (31–33). However, the patient heterogeneity limits the application of this approach in the clinic. Hence a personalized approach would be desirable for developing appropriate therapeutic regimens. We took a patient wise analysis approach where gene expression profile of colorectal cancer tumor samples was matched with their own normal colon tissue. Glutathione (GSH) metabolism is known to be associated with colorectal cancer pathogenesis. Elevated levels of glutathione and the enzymes responsible for its production have been reported in CRC and this is confirmed by our results. This observation reinforces the hypothesis that rapidly growing cancer cells are under oxidative stress and need more glutathione (34, 35). GSH metabolism could be used as an important biomarker for resistance and treatment response (36). Significant involvement of glutathione metabolism in our patient cohort could be reflective of aggressive tumors since most of the patients were from advanced stages. GSH metabolism could therefore be utilized as a prognostic marker in CRC patients. In addition, polyamine metabolism was identified as elevated in colorectal cancer patients, and it has been associated with tumor growth as well as immune cell function (37). It has been shown that down-regulating one of the key driver of polyamine metabolism, IDO1, could improve the response rate of immunotherapy in cancer (38). Moreover, Hayes et al. reported that by using a “polyamine-blocking therapy,” they could reverse immunosuppression in tumor microenvironment in mice breast cancer model (39). Therefore, the identified polyamine metabolism related genes, especially ODC1 and SMOX, should be tested in in vitro and in vivo models in future studies since they could be promising gene target for CRC treatment.
ODC1 gene polymorphism has been reported to reduce the risk of adenoma recurrence by suppressing synthesis of colonic mucosal polyamines (40). ODC1 protein has been used as a biomarker for measuring the efficacy of colecoxib in the treatment of colorectal cancer. It has also been involved in familial adenomatous polyposis as a manifestation of APC gene mutation (41). However, there is no known correlation of altered ODC1 gene expression levels with CRC.
Our results thus provide added evidence to support the involvement of ODC1 gene in colorectal cancer progression. ODC1, SAT1, SMOX, and SRM genes are known to decrease cell proliferation in several cancer cell lines (42–46). ODC1 and RRM2 have also been implicated in increased cell proliferation in different conditions (47–50). However, our study is the first to report the putative involvement of SAT1, SMOX, SRM, and RR M2 in colorectal cancer. Sulindac has been reported to induce SAT1 gene mediated chemo preventive affect in colorectal cancer in a COX independent mechanism (51). SMS gene is completely novel in this study with no known prior association with any type of cancer.
The importance of proline metabolism in helping cancer cells to grow is being revealed through this report (52). Our study lends credibility to the putative role of proline metabolism in cancer progression. However, current literature is scarce and more definitive studies are needed to substantiate the role of proline metabolism especially in colorectal cancer. Arginine deprivation therapy has been proposed to be a promising way to deal with cancer at metabolic level (53).
Our validation results of expression of six genes in colorectal patients and cell lines corroborate an interesting aspect of cancer—heterogeneity. While some patients show expected pattern of gene expression, there are others who exhibit a reverse pattern. These results suggest and support the notion of personalized medicine especially in colorectal cancer (54). More studies are needed to generate evidence in favor of customizing dosage and therapeutic agents in patients based on their integrated metabolic profile. Integrated evidence from transcriptomics and metabolomics could be more promising in terms of accuracy and specificity in the clinic (55, 56). Evidence is mounting in favor of using multiomics approach toward finding biomarkers and therapeutic targets especially in cancer (57, 58).
Data Availability
The datasets generated for this study can be found in the GEO database: GSE50421/https://www.ncbi.nlm.nih.gov/geo/query/acc.cgi?acc=GSE50421, GEO database: GSE77434/https://www.ncbi.nlm.nih.gov/geo/query/acc.cgi?acc=GSE77434.
Ethics Statement
The studies involving human participants were reviewed and approved by King Abdullah International Medical Research Center. The patients/participants provided their written informed consent to participate in this study.
Author Contributions
CZ analyzed the data and wrote the paper. MAl did the qRT-PCR experiments. MAr analyzed the data. XL helped in data analysis. AM conceived the idea, designed the analyses, and wrote the paper. MAA conceived the idea, generated and analyzed patient gene expression data, and wrote the paper. All authors read the manuscript and approved its contents.
Conflict of Interest Statement
The authors declare that the research was conducted in the absence of any commercial or financial relationships that could be construed as a potential conflict of interest.
Acknowledgments
This study was supported by Grant # RC10/083 awarded to MAA.
Supplementary Material
The Supplementary Material for this article can be found online at: https://www.frontiersin.org/articles/10.3389/fonc.2019.00681/full#supplementary-material
References
1. Pardini B, Kumar R, Naccarati A, Novotny J, Prasad RB, Forsti A, et al. 5-Fluorouracil-based chemotherapy for colorectal cancer and MTHFR/MTRR genotypes. Br J Clin Pharmacol. (2011) 72:162–3. doi: 10.1111/j.1365-2125.2010.03892.x
2. Bloem LT, De Abreu Lourenco R, Chin M, Ly B, Haas M. Factors impacting treatment choice in the first-line treatment of colorectal cancer. Oncol Ther. (2016) 4:103–16. doi: 10.1007/s40487-016-0020-4
3. Brenner H, Kloor M, Pox CP. Colorectal cancer. Lancet. (2014) 383:1490–502. doi: 10.1016/S0140-6736(13)61649-9
4. Cassidy J, Clarke S, Diaz-Rubio E, Scheithauer W, Figer A, Wong R, et al. Randomized phase III study of capecitabine plus oxaliplatin compared with fluorouracil/folinic acid plus oxaliplatin as first-line therapy for metastatic colorectal cancer. J Clin Oncol. (2008) 26:2006–12. doi: 10.1200/JCO.2007.14.9898
5. Saltz LB, Clarke S, Diaz-Rubio E, Scheithauer W, Figer A, Wong R, et al. Bevacizumab in combination with oxaliplatin-based chemotherapy as first-line therapy in metastatic colorectal cancer: a randomized phase III study. J Clin Oncol. (2008) 26:2013–9. doi: 10.1200/JCO.2007.14.9930
6. Tol J, Koopman M, Cats A, Rodenburg CJ, Creemers GJ, Schrama JG, et al. Chemotherapy, bevacizumab, and cetuximab in metastatic colorectal cancer. N Engl J Med. (2009) 360:563–72. doi: 10.1056/NEJMoa0808268
7. Hecht JR, Mitchell E, Chidiac T, Scroggin C, Hagenstad C, Spigel D, et al. A randomized phase IIIB trial of chemotherapy, bevacizumab, and panitumumab compared with chemotherapy and bevacizumab alone for metastatic colorectal cancer. J Clin Oncol. (2009) 27:672–80. doi: 10.1200/JCO.2008.19.8135
8. Aziz MA, Periyasamy S, Yousef Z, Deeb A, AlOtaibi M. Colorectal cancer driver genes identified by patient specific comparison of cytogenetic microarray. Genom Data. (2014) 2:29–31. doi: 10.1016/j.gdata.2014.02.004
9. Eldai H, Periyasamy S, Al Qarni S, Al Rodayyan M, Muhammed Mustafa S, Deeb A, et al. Novel genes associated with colorectal cancer are revealed by high resolution cytogenetic analysis in a patient specific manner. PLoS ONE. (2013) 8:e76251. doi: 10.1371/journal.pone.0076251
10. Aziz MA, Periyasamy S, Al Yousef Z, AlAbdulkarim I, Al Otaibi M, Alfahed A, et al. Integrated exon level expression analysis of driver genes explain their role in colorectal cancer. PLoS ONE. (2014) 9:e110134. doi: 10.1371/journal.pone.0110134
11. Mardinoglu A, Agren R, Kampf C, Asplund A, Nookaew I, Jacobson P, et al. Integration of clinical data with a genome-scale metabolic model of the human adipocyte. Mol Syst Biol. (2013) 9:649. doi: 10.1038/msb.2013.5
12. Bosley J, Boren C, Lee S, Grotli M, Nielsen J, Uhlen M, et al. Improving the economics of NASH/NAFLD treatment through the use of systems biology. Drug Discov Today. (2017) 22:1532–8. doi: 10.1016/j.drudis.2017.07.005
13. Mardinoglu A, Boren J, Smith U, Uhlen M, Nielsen J. Systems biology in hepatology: approaches and applications. Nat Rev Gastroenterol Hepatol. (2018) 15:365–77. doi: 10.1038/s41575-018-0007-8
14. Mardinoglu A, Nielsen J. Systems medicine and metabolic modelling. J Intern Med. (2012) 271:142–54. doi: 10.1111/j.1365-2796.2011.02493.x
15. Mardinoglu A, Gatto F, Nielsen J. Genome-scale modeling of human metabolism - a systems biology approach. Biotechnol J. (2013) 8:985–96. doi: 10.1002/biot.201200275
16. Mardinoglu A, Nielsen J. New paradigms for metabolic modeling of human cells. Curr Opin Biotech. (2015) 34:91–7. doi: 10.1016/j.copbio.2014.12.013
17. Folger O, Jerby L, Frezza C, Gottlieb E, Ruppin E, Shlomi T. Predicting selective drug targets in cancer through metabolic networks. Mol Syst Biol. (2011) 7:501. doi: 10.1038/msb.2011.35
18. Frezza C, Zheng L, Folger O, Rajagopalan KN, MacKenzie ED, Jerby L, et al. Haem oxygenase is synthetically lethal with the tumour suppressor fumarate hydratase. Nature. (2011) 477:225–8. doi: 10.1038/nature10363
19. Agren R, Bordel S, Mardinoglu A, Pornputtapong N, Nookaew I, Nielsen J. Reconstruction of genome-scale active metabolic networks for 69 human cell types and 16 cancer types using INIT. PLoS Comput Biol. (2012) 8:e1002518. doi: 10.1371/journal.pcbi.1002518
20. Agren R, Mardinoglu A, Asplund A, Kampf C, Uhlen M, Nielsen J. Identification of anticancer drugs for hepatocellular carcinoma through personalized genome-scale metabolic modeling. Mol Syst Biol. (2014) 10:721. doi: 10.1002/msb.145122
21. Uhlen M, Zhang C, Lee S, Sjostedt E, Fagerberg L, Bidkhori G, et al. A pathology atlas of the human cancer transcriptome. Science. (2017) 357:eaan2507. doi: 10.1126/science.aan2507
22. Mardinoglu A, Agren R, Kampf C, Asplund A, Uhlen M, Nielsen J. Genome-scale metabolic modelling of hepatocytes reveals serine deficiency in patients with non-alcoholic fatty liver disease. Nat Commun. (2014) 5:3083. doi: 10.1038/ncomms4083
23. Varemo L, Nielsen J, Nookaew I. Enriching the gene set analysis of genome-wide data by incorporating directionality of gene expression and combining statistical hypotheses and methods. Nucleic Acids Res. (2013) 41:4378–91. doi: 10.1093/nar/gkt111
24. Liberzon A, Birger C, Thorvaldsdottir H, Ghandi M, Mesirov JP, Tamayo P. The Molecular Signatures Database (MSigDB) hallmark gene set collection. Cell Syst. (2015) 1:417–25. doi: 10.1016/j.cels.2015.12.004
25. Brown R, Short S, Williams C. Colorectal cancer and metabolism. Curr Colorectal Cancer Rep. (2018) 14:226–41. doi: 10.1007/s11888-018-0420-y
26. Beloribi-Djefaflia S, Vasseur S, Guillaumond F. Lipid metabolic reprogramming in cancer cells. Oncogenesis. (2016) 5:e189. doi: 10.1038/oncsis.2015.49
27. Uhlen M, Fagerberg L, Hallstrom BM, Lindskog C, Oksvold P, Mardinoglu A, et al. Tissue-based map of the human proteome. Science. (2015) 347:1260419. doi: 10.1126/science.1260419
28. Patil KR, Nielsen J. Uncovering transcriptional regulation of metabolism by using metabolic network topology. P Natl Acad Sci USA. (2005) 102:2685–9. doi: 10.1073/pnas.0406811102
29. Ha HC, Sirisoma NS, Kuppusamy P, Zweier JL, Woster PM, Casero RA Jr. The natural polyamine spermine functions directly as a free radical scavenger. Proc Natl Acad Sci USA. (1998) 95:11140–5. doi: 10.1073/pnas.95.19.11140
30. Russell DH, Levy CC, Schimpff SC, Hawk IA. Urinary polyamines in cancer patients. Cancer Res. (1971) 31:1555–8.
31. Auslander N, Cunningham CE, Toosi BM, McEwen EJ, Yizhak K, Vizeacoumar FS, et al. An integrated computational and experimental study uncovers FUT9 as a metabolic driver of colorectal cancer. Mol Syst Biol. (2017) 13:956. doi: 10.15252/msb.20177739
32. Lewis NE, Abdel-Haleem AM. The evolution of genome-scale models of cancer metabolism. Front Physiol. (2013) 4:237. doi: 10.3389/fphys.2013.00237
33. Ghaffari P, Mardinoglu A, Nielsen J. Cancer metabolism: a modeling perspective. Front Physiol. (2015) 6:382. doi: 10.3389/fphys.2015.00382
34. Kim AD, Zhang R, Han X, Kang KA, Piao MJ, Maeng YH, et al. Involvement of glutathione and glutathione metabolizing enzymes in human colorectal cancer cell lines and tissues. Mol Med Rep. (2015) 12:4314–9. doi: 10.3892/mmr.2015.3902
35. Liu Y, Hyde AS, Simpson MA, Barycki JJ. Emerging regulatory paradigms in glutathione metabolism. Adv Cancer Res. (2014) 122:69–101. doi: 10.1016/B978-0-12-420117-0.00002-5
36. Bansal A, Simon MC. Glutathione metabolism in cancer progression and treatment resistance. J Cell Biol. (2018) 217:2291–8. doi: 10.1083/jcb.201804161
37. Hesterberg RS, Cleveland JL, Epling-Burnette PK. Role of polyamines in immune cell functions. Med Sci (Basel). (2018) 6:E22. doi: 10.3390/medsci6010022
38. Liu M, Wang X, Wang L, Ma X, Gong Z, Zhang S, et al. Targeting the IDO1 pathway in cancer: from bench to bedside. J Hematol Oncol. (2018) 11:100. doi: 10.1186/s13045-018-0644-y
39. Hayes CS, Shicora AC, Keough MP, Snook AE, Burns MR, Gilmour SK. Polyamine-blocking therapy reverses immunosuppression in the tumor microenvironment. Cancer Immunol Res. (2014) 2:274–85. doi: 10.1158/2326-6066.CIR-13-0120-T
40. Martinez ME, O'Brien TG, Fultz KE, Babbar N, Yerushalmi H, Qu N, et al. Pronounced reduction in adenoma recurrence associated with aspirin use and a polymorphism in the ornithine decarboxylase gene. Proc Natl Acad Sci USA. (2003) 100:7859–64. doi: 10.1073/pnas.1332465100
41. Plawski A, Banasiewicz T, Borun P, Kubaszewski L, Krokowicz P, Skrzypczak-Zielinska M, et al. Familial adenomatous polyposis of the colon. Hered Cancer Clin Pract. (2013) 11:15. doi: 10.1186/1897-4287-11-15
42. Suzuki T, He Y, Kashiwagi K, Murakami Y, Hayashi S, Igarashi K. Antizyme protects against abnormal accumulation and toxicity of polyamines in ornithine decarboxylase-overproducing cells. Proc Natl Acad Sci USA. (1994) 91:8930–4. doi: 10.1073/pnas.91.19.8930
43. Vujcic S, Halmekyto M, Diegelman P, Gan G, Kramer DL, Janne J, et al. Effects of conditional overexpression of spermidine/spermine N1-acetyltransferase on polyamine pool dynamics, cell growth, and sensitivity to polyamine analogs. J Biol Chem. (2000) 275:38319–28. doi: 10.1074/jbc.M003270200
44. Kee K, Vujcic S, Merali S, Diegelman P, Kisiel N, Powell CT. Metabolic and antiproliferative consequences of activated polyamine catabolism in LNCaP prostate carcinoma cells. J Biol Chem. (2004) 279:27050–8. doi: 10.1074/jbc.M403323200
45. Bluemn EG, Spencer ES, Mecham B, Gordon RR, Coleman I, Lewinshtein D, et al. PPP2R2C loss promotes castration-resistance and is associated with increased prostate cancer-specific mortality. Mol Cancer Res. (2013) 11:568–78. doi: 10.1158/1541-7786.MCR-12-0710
46. Haller DG, Catalano PJ, Macdonald JS, O'Rourke MA, Frontiera MS, Jackson DV, et al. Phase III study of fluorouracil, leucovorin, and levamisole in high-risk stage II and III colon cancer: final report of Intergroup 0089. J Clin Oncol. (2005) 23:8671–8. doi: 10.1200/JCO.2004.00.5686
47. Luk GD, Yang P. Polyamines in intestinal and pancreatic adaptation. Gut. (1987) 28(Suppl):95–101. doi: 10.1136/gut.28.Suppl.95
48. Heidel JD1, Liu JY, Yen Y, Zhou B, Heale BS, Rossi JJ, et al. Potent siRNA inhibitors of ribonucleotide reductase subunit RRM2 reduce cell proliferation in vitro and in vivo. Clin Cancer Res. (2007) 13:2207–15. doi: 10.1158/1078-0432.CCR-06-2218
49. Satow R, Shitashige M, Kanai Y, Takeshita F, Ojima H, Jigami T, et al. Combined functional genome survey of therapeutic targets for hepatocellular carcinoma. Clin Cancer Res. (2010) 16:2518–28. doi: 10.1158/1078-0432.CCR-09-2214
50. Ohhashi S, Ohuchida K, Mizumoto K, Fujita H, Egami T, Yu J, et al. Down-regulation of deoxycytidine kinase enhances acquired resistance to gemcitabine in pancreatic cancer. Anticancer Res. (2008) 28:2205–12.
51. Babbar N, Gerner EW, Casero RA Jr. Induction of spermidine/spermine N1-acetyltransferase (SSAT) by aspirin in Caco-2 colon cancer cells. Biochem J. (2006) 394:317–24. doi: 10.1042/BJ20051298
52. Phang JM, Liu W, Hancock CN, Fischer JW. Proline metabolism and cancer: emerging links to glutamine and collagen. Curr Opin Clin Nutr Metab Care. (2015) 18:71–7. doi: 10.1097/MCO.0000000000000121
53. Delage B, Fennell DA, Nicholson L, McNeish I, Lemoine NR, Crook T, et al. Arginine deprivation and argininosuccinate synthetase expression in the treatment of cancer. Int J Cancer. (2010) 126:2762–72. doi: 10.1002/ijc.25202
54. Aziz MA, Yousef Z, Saleh AM, Mohammad S, Al Knawy B. Towards personalized medicine of colorectal cancer. Crit Rev Oncol Hematol. (2017) 118:70–8. doi: 10.1016/j.critrevonc.2017.08.007
55. Borgan E, Sitter B, Lingjaerde OC, Johnsen H, Lundgren S, Bathen TF, et al. Merging transcriptomics and metabolomics–advances in breast cancer profiling. BMC Cancer. (2010) 10:628. doi: 10.1186/1471-2407-10-628
56. Liesenfeld DB, Grapov D, Fahrmann JF, Salou M, Scherer D, Toth R, et al. Metabolomics and transcriptomics identify pathway differences between visceral and subcutaneous adipose tissue in colorectal cancer patients: the ColoCare study. Am J Clin Nutr. (2015) 102:433–43. doi: 10.3945/ajcn.114.103804
57. Buescher JM, Driggers EM. Integration of omics: more than the sum of its parts. Cancer Metab. (2016) 4:4. doi: 10.1186/s40170-016-0143-y
Keywords: colorectal cancer, genome scale metabolic model, polyamine metabolism, personalized medicine, transcriptomics
Citation: Zhang C, Aldrees M, Arif M, Li X, Mardinoglu A and Aziz MA (2019) Elucidating the Reprograming of Colorectal Cancer Metabolism Using Genome-Scale Metabolic Modeling. Front. Oncol. 9:681. doi: 10.3389/fonc.2019.00681
Received: 29 May 2019; Accepted: 10 July 2019;
Published: 30 July 2019.
Edited by:
Antonio Giuseppe Naccarato, University of Pisa, ItalyReviewed by:
Barbara Marengo, University of Genoa, ItalyPaul Dent, Virginia Commonwealth University, United States
Copyright © 2019 Zhang, Aldrees, Arif, Li, Mardinoglu and Aziz. This is an open-access article distributed under the terms of the Creative Commons Attribution License (CC BY). The use, distribution or reproduction in other forums is permitted, provided the original author(s) and the copyright owner(s) are credited and that the original publication in this journal is cited, in accordance with accepted academic practice. No use, distribution or reproduction is permitted which does not comply with these terms.
*Correspondence: Adil Mardinoglu, YWRpbG0mI3gwMDA0MDtzY2lsaWZlbGFiLnNl; Mohammad Azhar Aziz, YXppem1vJiN4MDAwNDA7bmdoYS5tZWQuc2E=