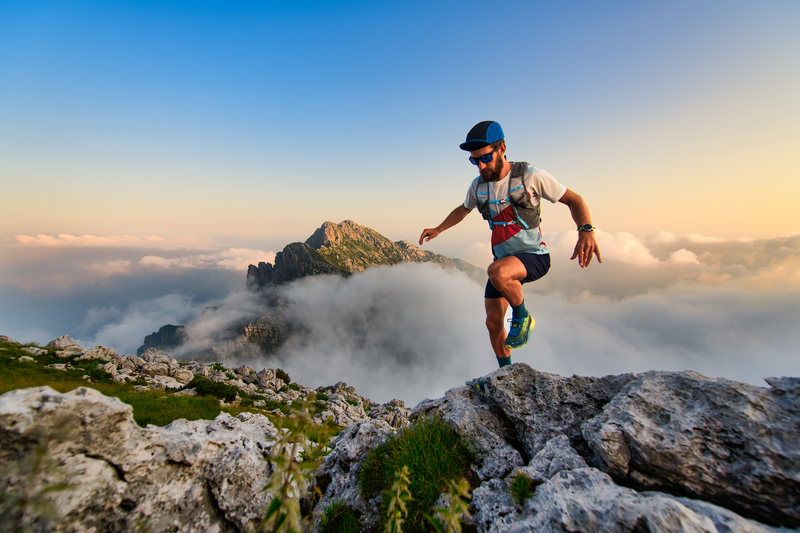
94% of researchers rate our articles as excellent or good
Learn more about the work of our research integrity team to safeguard the quality of each article we publish.
Find out more
ORIGINAL RESEARCH article
Front. Oncol. , 18 July 2019
Sec. Cancer Imaging and Image-directed Interventions
Volume 9 - 2019 | https://doi.org/10.3389/fonc.2019.00589
Purpose: This study assessed the ability of metabolic parameters from 18Fluorodeoxyglucose positron emission tomography/computed tomography (18F-FDG PET/CT) and clinicopathological data to predict epidermal growth factor receptor (EGFR) expression/mutation status in patients with lung adenocarcinoma and to develop a prognostic model based on differences in EGFR expression status, to enable individualized targeted molecular therapy.
Patients and Methods: Metabolic parameters and clinicopathological data from 200 patients diagnosed with lung adenocarcinoma between July 2009 and November 2016, who underwent 18F-FDG PET/CT and EGFR mutation testing, were retrospectively evaluated. Multivariate logistic regression was applied to significant variables to establish a prediction model for EGFR mutation status. Overall survival for both mutant and wild-type EGFR was analyzed to establish a multifactor Cox regression model.
Results: Of the 200 patients, 115 (58%) exhibited EGFR mutations and 85 (42%) were wild-type. Among selected metabolic parameters, metabolic tumor volume (MTV) demonstrated a significant difference between wild-type and mutant EGFR mutation status, with an area under the receiver operating characteristic curve (AUC) of 0.60, which increased to 0.70 after clinical data (smoking status) were combined. Survival analysis of wild-type and mutant EGFR yielded mean survival times of 34.451 (95% CI 28.654–40.249) and 53.714 (95% CI 44.331–63.098) months, respectively. Multivariate Cox regression revealed that mutation type, tumor stage, and thyroid transcription factor-1 (TTF-1) expression status were the main factors influencing patient prognosis. The hazard ratio for mutant EGFR was 0.511 (95% CI 0.303–0.862) times that of wild-type, and the risk of death was lower for mutant EGFR than for wild-type. The risk of death was lower in TTF-1-positive than in TTF-1-negative patients.
Conclusion: 18F-FDG PET/CT metabolic parameters combined with clinicopathological data demonstrated moderate diagnostic efficacy in predicting EGFR mutation status and were associated with prognosis in mutant and wild-type EGFR non-small-cell lung cancer (NSCLC), thus providing a reference for individualized targeted molecular therapy.
Lung cancer is one of the most aggressive malignant tumors, with high rates of morbidity and mortality worldwide, and has recently risen to rank first among malignant tumors (1–3). Among all pathological categories of lung cancer, non-small cell lung cancer (NSCLC) is the most common, accounting for ~85% of lung cancers (4–6). Recent advances in the understanding of the molecular biology of NSCLC (7, 8) have attracted attention for molecular-driven targeted therapy. Tyrosine kinase inhibitors (TKIs), such as gefitinib and erlotinib, have been introduced (9) and have become an effective treatment for patients with mutations in the epidermal growth factor (EGFR) gene (10, 11). Several studies (12–14) have described that the EGFR mutation status is the main factor in predicting the therapeutic effect of EGFR- TKIs. Therefore, it is essential to identify EGFR mutation status before attempts at targeted therapy.
However, tumor biopsies for the detection of EGFR mutations have limitations. The tumor biopsy location may strongly affect the detection result, and patients' general condition may also restrict widespread use of biopsies in clinical practice. Therefore, the use of medical imaging as a non-invasive method to obtain information about the tumor phenotype could provide clues to predict mutation status of the EGFR gene and has been investigated in several studies (15–17) using positron emission tomography with 2-deoxy-2-[fluorine-18]fluoro-D-glucose integrated with computed tomography (18F-FDG PET/CT) to predict the mutation status of EGFR in patients with lung adenocarcinoma; however, the results remain controversial.
Additionally, studies have also described the effects of targeted therapy associated with EGFR mutation status. However, few studies have investigated the effect of various metabolic parameters and clinicopathological information on the prognosis of patients with wild-type and mutant type EGFR. Therefore, the objectives of the present study were to assess the ability of metabolic parameters from 18F-FDG PET/CT and clinicopathological data to predict epidermal growth factor receptor (EGFR) expression/mutation status in patients with lung adenocarcinoma, and to develop a prognostic model based on differences in EGFR mutant status, thereby providing a reference for individualized molecular targeted therapy.
Clinicopathological data from 200 patients diagnosed with lung adenocarcinoma between July 2009 and November 2016, were retrospectively analyzed. Patients who underwent PET/CT examination before treatment with histopathological confirmation, along with EGFR gene test results were included. Individuals with a history of malignant tumors, previous treatment before EGFR gene testing, and insufficient tissue for genetic testing were excluded from this study. Clinicopathological data included age, sex, family history, smoking history/status (Non-smokers were defined as patients who never smoked or smoked <100 cigarettes in their lifetimes), histological grade, regional lymph node metastasis, distant metastasis, tumor, node, and metastasis stage (i.e., I, II/III, and IV), thyroid transcription factor-1 (TTF-1) (– or one + was defined as negative; ≥2 was defined as positive), Ki-67 (≤ 25% was defined as low expression and >25% as high expression), carcinoembryonic antigen, tumor location, the largest diameter of the tumor, and PET/CT metabolism parameters are summarized in Table 1. Overall survival (OS) defined as the time interval between date of PET/CT examination and death or to the date of final follow-up. Statisticed by month. OS of patients with mutant and wild-type EGFR was followed up (July 2009 to January 2019). The starting point for OS was the date of PET/CT examination and the end point was defined as the date of telephonic follow-up or death. And the telephonic follow-up was the last follow-up.
Table 1. Association between clinical characteristics and the epidermal growth factor receptor mutation status in patients with lung adenocarcinoma.
All patients underwent PET/CT before initiation of treatment. PET/CT scans were performed using a PET/CT imager(Biograph 16, Siemens, Knoxville, TN, USA).18F-FDG was produced by EBCO's TR19 medical cyclotron in Canada, and then automatically synthesized using a chemical synthesis system with a radiochemical purity >95%. All patients fasted 6–8 h before the examination. Height, weight, and fasting blood glucose levels were recorded before the examination and blood glucose levels were controlled to levels <6.7 mmol/L. After an intravenous injection of 18F-FDG (3.7–6.66 MBq/kg), patients were asked to consume 500–1,000 ml water and rest for 40–60 min. A whole-body PET/CT examination was performed from the skull base to the upper femur (Generally 6–7 beds location). Both CT and PET scan were performed. CT scan parameters were as follows: tube voltage, 120 kV; tube current, 140 mAs; and layer thickness and layer spacing, 5 mm. The PET acquisition method involved a three-dimensional acquisition, 3 min/bed, and the image was reconstructed by iterative reconstruction. Images of transverse, sagittal, and coronal positions of CT, PET, and PET/CT fusions were acquired.
Two experienced nuclear medicine physicians marked the location, size, and shape of the tumor and its relationship with surrounding tissues on post-processed images. When the opinions of the two physicians are inconsistent, it is decided by the collective discussion of our department. The imaging data were transmitted to the AW4.6 workstation and tumor lesions were identified by visual inspection. Semi-quantitative measurements were based on the high FDG metabolic area of the lesion using the MS viewer software and by manually delineating the region of interest (ROI). ROIs were placed over the primary tumor to measure the maximum standard uptake value (SUVmax) (SUVmax threshold is set to 40%), mean standard uptake value (SUVmean).Calculate the metabolic tumor volume (MTV) (ROI area per layer × layer thickness = volume of each layer, then add the volume of each layer to get MTV). And then calculate tumor-lesion glycolysis (TLG) (TLG = SUVmean × MTV).
EGFR gene testing was performed by the pathology department at the authors' hospital. The tissues for gene detection were obtained from a specimen of tumor resection or biopsy, fixed in formalin, embedded in paraffin, and cut into sections. EGFR gene detection was performed using a real-time fluorescent quantitative nucleotide amplification method.
All data were processed using SPSS version 25.0 (IBM Corporation, Armonk, NY, USA). Quantitative data that were normally distributed are expressed as mean ± standard deviation, and the independent sample t-test was used for comparison between the two groups. Quantitative data that were not normally distributed are expressed as median (interquartile range), and the Mann-Whitney test was used for comparison between the two groups. Qualitative data are expressed as number and percentage (n [%]), and the chi-squared test or Fisher's exact probability method was used for comparison between the two groups. SUVmax, SUVmean, TLG, MTV index, and area under the receiver operating characteristic (ROC) curve (AUC) were calculated from the ROC curve, the identification of wild-type and mutant EGFR status at any threshold, and the maximum Youden's index as the standard for selecting the optimal cut-off limit value to convert the four quantitative indicators into two-category indicators. Covariates were screened using univariate logistic regression (p < 0.10 factor), and further forward likelihood ratio (LR) was used (inclusion test level = 0.05, rejection test level = 0.10) to establish a multivariate logistic step-wise regression model of predictive factors. Multivariate analysis was performed to construct the model; the odds ratio (OR), 95% confidence interval (CI), and AUC were calculated; an optimal cut-off point was identified using Youden's index; the 95% CI values of the cut-off point were calculated. The Delong method was used to compare the AUC values of the different models.
Survival rates for EGFR wild-type and mutant patients were analyzed using the Kaplan-Meier method. Survival time is expressed as mean and median and 95% CI, and compared using the log-rank test. A Cox proportional hazard regression model was used to screen covariates, including variables with p < 0.10 in the univariate analysis, and further forward LR (incorporated with a test level of 0.05 and a rejection test level of 0.10). The optimal multivariate Cox regression model was established and the hazard ratio (HR) and corresponding 95% CI were calculated; p < 0.05 was considered to be statistically significant.
The clinical characteristics of the patient population are summarized in Table 1. Of the 200 patients, 115 (58%) exhibited an EGFR mutation and 85 (42%) exhibited wild-type EGFR. The mean age of the 200 patients with EGFR wild-type and mutant lung adenocarcinoma was 63.61 ± 11.42 and 59.39 ± 9.73 years, respectively. The average age of the EGFR wild-type patients was greater than those with mutant EGFR. Among the 200 patients, 108 (54%) were male, 92 (46%) were female, 132 (66%) were non-smokers, and 68 (32%) were smokers. The EGFR mutation status of poorly differentiated, moderately differentiated, and highly differentiated tumors was 47 (28.1%), 99 (59.3%), and 21 (12.6%), respectively. TNM stages I and II, and III and IV, accounted for 26 (13%) and 174 (87%), respectively. A total of 200 patients were followed-up from July 2009 to January 2019; eight (4%) patients were lost to follow-up. Of the 192 patients who were followed-up, the median survival time was 36.000 months [95% CI 31.284–40.716 (range 1–114 months)] and the mean survival time was 47.635 months (95% CI 40.610–54.661). The 1-, 3-, and the 5-year OS rates were 84.9, 47.7, and 30.5%, respectively. In the follow-up of 123 (64.1%) patients, the median survival time was 21.000 months (range 1–83 months) (95% CI 16.245–25.755), and mean survival time was 24.472 months (95% CI 21.837–27.107). The 1-, 3-, and 5-year OS rates were 76.4, 19.5, and 2.4%, respectively.
EGFR mutations were more common in patients who were female (56.5 vs. 43.5%; p < 0.001), non-smokers (77.4 vs. 22.6%; p < 0.001), patients with moderately differentiated tumors (27.8 vs. 50.0%, and 22.2%; p < 0.015), patients with TTF-1 positive expression (95.5 vs. 4.5%; p < 0.021), and patients with lung adenocarcinoma of the right upper lobe (Table 1).
ROC curves were used to classify a variety of continuous variables that predict the status of EGFR, and the optimal cut-off points were determined (Table 2). Representative PET/CT images of two patients with EGFR mutations are shown in Figure 1.
Table 2. Association between metabolic parameters and epidermal growth factor receptor mutation status.
Figure 1. (A–D) Female, 63 years of age. Positron emission tomography/computed tomography (PET/CT) revealing lung adenocarcinoma of the right upper lobe, ~33 × 25 mm, increased fluorodeoxyglucose (FDG) metabolism, maximum standardized uptake value (SUVmax) 6.8, epidermal growth factor (EGFR) mutation, and overall survival (OS) of 29 months. (E–H) Male, 72 years of age. Positron emission tomography/computed tomography (PET/CT) revealing lung adenocarcinoma of the posterior basal segment of the left lower lobe, ~27 × 19 mm, increased fluorodeoxyglucose (FDG) metabolism, maximum standardized uptake value (SUVmax) 9.4, epidermal growth factor receptor (EGFR) mutation, and OS of 13 months.
Univariate logistic regression analysis revealed a significant association between EGFR mutation status and age, sex, smoking status, histological grade, stage, TTF-1, SUVmax, SUVmean, TLG, and MTV. The multivariate model using forward LR stepwise regression analysis revealed that smoking and MTV remained as independent variables for predicting EGFR mutations [non-smoker (OR,0.288; p < 0.001); MTV (OR, 2.482; p = 0.003)]. Smoking and MTV were independent variables for constructing predictive models. MTV demonstrated a significant difference between wild-type and mutant EGFR status, with an AUC of 0.60, which reached 0.70 after clinical data (smoking status) was combined (Table 3 and Figure 2).
Table 3. Univariate and multivariate analysis of various predictive factors for the EGFR status in lung adenocarcinoma.
Figure 2. The area under the receiver operating characteristic curve (AUC) for metabolic tumor volume (MTV) was 0.60, which increased to 0.70 when clinical data (smoking status) was combined. Sensitivity and specificity were 0.50 and 0.81, respectively. The cut-off value of MTV was 11.55, and was 0.56 when combined with the clinical data (smoking status).
The survival rates of wild-type and mutant EGFR were analyzed using the Kaplan-Meier method. The average survival time of wild-type was 34.451 (95% CI 28.654–40.249), and the median survival time was 27 months (95% CI 20.000–33.000). The mean survival time of the mutant type was 53.714 (95% CI 44.331–63.098) and the median survival time was 44 months (95% CI 36.000–53.000) (Table 4 and Figure 3). The multivariate Cox regression model revealed mutation type, tumor stage, and expression status of TTF-1 as independent risk factors for patient prognosis. The HRs for TTF-1 (++/+++), stage (III/IV), and type (mutant) was 0.325 (95% CI 0.156–0.679; p = 0.003), 7.116 (95% CI 1.710–29.617; p = 0.007), and 0.511 (95% CI 0.303–0.862; p = 0.012), respectively (Table 5).
Table 4. Survival analysis for patients with wild-type and mutant patients epidermal growth factor receptor.
In the present study, we investigated the association between PET/CT metabolic parameters and clinicopathological data and EGFR mutation status, and found a higher frequency of EGFR mutations in females and non-smokers. Previous epidemiological studies have also reported associations between specific features, such as female sex, non-smokers, and Asian origin with mutations in EGFR (18–22), consistent with our results. In addition, results of the present study demonstrated a relationship between EGFR mutation status and age and tumor stage, with the average age of wild-type patients greater than that of patients with mutant EGFR. EGFR mutation was primarily detected in stages III/IV, which has rarely been reported in previous studies. This could be due to race selection bias or insufficient sample size. Our study also found a significantly higher positive expression rate of TTF-1 in mutant patients, which may be explained by the high positive expression rate of TTF-1 in NSCLC and higher adenocarcinoma rate than squamous cell carcinoma, consistent with the literature (23, 24).The principle of applying PET/CT in lung cancer is based on differences in glucose metabolism between tumor and normal tissues (25). Studies have reported that the EGFR gene can affect GLUT-1 through a downstream pathway, thus further affecting tumor glucose metabolism. As such, differences in EGFR mutation status may lead to differences in tumor glucose metabolism (26).
In our study, univariate logistic regression analysis revealed a significant association between EGFR mutations and age, sex, smoking status, histological grade, stage, TTF-1, SUVmax, SUVmean, TLG, and MTV. Further multivariate analysis revealed MTV as a predictor of EGFR mutations, which was not reported in previous studies wherein SUVmax was used as a predictor for EGFR mutations (27–33). As an indicator reflecting the overall metabolic level of the tumor, MTV is suitable for predicting the mutation status of EGFR. In addition, studies have shown that patients with lower MTV have a better prognosis (34–36), while others have reported a significant association between patient prognosis and EGFR mutation status, thus supporting the results of our study. Our research demonstrated that the AUC of MTV was 0.60; further analysis using a combination of MTV with clinical data, including smoking history, resulted in an AUC of 0.70. A previous study involving 849 patients with NSCLC reported similar 18F-FDG PET/CT results to predict EGFR mutation status (32). The results revealed that the AUC was 0.557 when EGFR status was predicted using metabolic parameters alone. After combining clinical data, such as sex and smoking status, the AUC was 0.697. Mei et al. (37) used CT texture features to predict EGFR mutation status in 296 patients. The AUC predicted by histological features alone was 0.575, which increased to 0.664 after clinical data were combined. Zhao et al. (38) used clinical imaging features of CT to predict EGFR mutation status in 471 patients with lung adenocarcinoma and reported an AUC of 0.706 when EGFR mutation status was predicted by clinical features, and 0.784 after the combined CT signatures. In our study, the AUC for predicting EGFR mutation status was similar to that reported in previous studies.
Our study analyzed the survival rates of EGFR wild-type and mutant patients, revealing a shorter mean survival time for EGFR wild-type compared with that for the mutant (34.451 months [95% CI 28.654–40.249] and 53.714 months [95% CI 44.331–63.098], respectively). Median survival of the EGFR wild-type and mutant patients was 27 (95% CI 20.000–33.000) and 44 (95% CI 36.000–53.000) months, respectively. Our results demonstrated a better prognosis for patients with EGFR mutations than for those with EGFR wild-type. Multivariate Cox regression analysis revealed that mutation type, tumor stage, and expression status of TTF-1 were independent factors affecting patient prognosis. The HR for wild-type mutations was 0.511. The risk of death in the mutant type was 0.511 times that of the wild-type; specifically, the risk of death in the mutant type was lower than that of the wild-type, and the prognosis for the mutant type was better than that for the wild-type, which is consistent with previous results (10, 39, 40). The risk of death in stage III/IV was 7.116 times higher than that in stage I/II. The HR for TTF-1 positive expression was 0.325 times that for TTF-1 negative expression, indicating that the risk of death from positive expression was 0.325 times that from negative expression (i.e., the risk of death from positive expression was lower than that from negative expression), which is consistent with previous reports (41–43). The positive expression rate of TTF-1 in EGFR mutant patients was higher, indicating that the prognosis of patients with EGFR mutations was better than those with wild-type EGFR.
There were limitations to the present study, which primarily included its retrospective design, small sample size, and possible patient selection bias. In addition, owing to the small sample size, the exact site of the EGFR mutations was not analyzed. Furthermore, mutant-type and wild-type treatments were not analyzed; therefore, the impact of different treatments on prognosis could not be analyzed and, as such, further research is needed.
In summary, the MTV metabolic parameters of PET/CT had a certain value for the identification of mutant and wild-type EGFR. Combining clinicopathological data with metabolic parameters may further improve diagnostic efficiency and may evaluation prediction of the prognosis of patients with mutant and wild-type EGFR. Our results may provide a reference for the design and implementation of individualized molecular-targeted therapies.
The raw data supporting the conclusions of this manuscript will be made available by the authors, without undue reservation, to any qualified researcher.
The institutional review board of Jinling Hospital, Medical School of Nanjing University approved this retrospective study and waived the need to obtain informed consent from the patients.
Patients consented to publishing their images and clinical information.
BY conceived the idea of the study and wrote the manuscript. BY, QW, and YZ collected the data. HZ and GL performed image analysis. ML performed the statistical analysis. YG and GL edited and reviewed the manuscript. All the authors discussed the results and commented on the manuscript.
This work was supported by the key research and development plan of the Ministry of Science and Technology (2017YFC0113400).
YG was employed by Siemens Healthineers Ltd.
The remaining authors declare that the research was conducted in the absence of any commercial or financial relationships that could be construed as a potential conflict of interest.
We would like to thank all the personnel from the Department of Nuclear Medicine and Department of Radiology, Jinling Hospital, Medical school of Nanjing University for their collective efforts on the image post-processing, clinical management, and follow-up.
CI, confidence interval; HR, hazard ratio; OR, odds ratio; PET/CT, positron emission tomography/computed tomography; MTV, metabolic tumor volume; NSCLC, non-small-cell lung cancer; EGFR, epidermal growth factor receptor; FDG, fluorodeoxyglucose; OS, overall survival; ROI, region of interest; SUVmax, maximum standardized uptake value; SUVmean, mean standardized uptake value; TLG, tumor lesion glycolysis; TKI, tyrosine kinase inhibitor; TTF-1, thyroid transcription factor-1.
1. Siegel RL, Miller KD, Jemal A. Cancer statistics, 2015. CA Cancer J Clin. (2015) 65:5–29. doi: 10.3322/caac.21254
2. Jemal A, Bray F, Center MM, Ferlay J, Ward E, Forman D. Global cancer statistics. CA Cancer J Clin. (2011) 61:69–90. doi: 10.3322/caac.20107
3. Chen W, Zheng R, Baade PD, Zhang S, Zeng H, Bray F, et al. Cancer statistics in China, 2015. CA Cancer J Clin. (2016) 66:115–32. doi: 10.3322/caac.21338
4. Ettinger DS, Wood DE, Akerley W, Bazhenova LA, Borghaei H, Camidge DR, et al. NCCN guidelines insights: non-small cell lung cancer, version 4. 2016. J Natl Compr Canc Netw. (2016) 14:255–64. doi: 10.6004/jnccn.2016.0031
5. Siegel R, Naishadham D, Jemal A. Cancer statistics for hispanics/latinos, 2012. CA Cancer J Clin. (2012) 62:283–98. doi: 10.3322/caac.21153
6. Austin JH, Garg K, Aberle D, Yankelevitz D, Kuriyama K, Lee HJ, et al. Radiologic implications of the 2011 classification of adenocarcinoma of the lung. Radiology. (2013) 266:62–71. doi: 10.1148/radiol.12120240
7. Rosell R, Moran T, Queralt C, Porta R, Cardenal F, Camps C, et al. Screening for epidermal growth factor receptor mutations in lung cancer. N Engl J Med. (2009) 361:958–67. doi: 10.1056/NEJMoa0904554
8. Pao W, Miller V, Zakowski M, Doherty J, Politi K, Sarkaria I, et al. EGF receptor gene mutations are common in lung cancers from “never smokers” and are associated with sensitivity of tumors to gefitinib and erlotinib. Proc Natl Acad Sci USA. (2004) 101:13306–11. doi: 10.1073/pnas.0405220101
9. Pao W, Miller VA. Epidermal growth factor receptor mutations, small-molecule kinase inhibitors, and non-small-cell lung cancer: current knowledge and future directions. J Clin Oncol. (2005) 23:2556–68. doi: 10.1200/JCO.2005.07.799
10. Rosell R, Carcereny E, Gervais R, Vergnenegre A, Massuti B, Felip E, et al. Erlotinib versus standard chemotherapy as first-line treatment for European patients with advanced EGFR mutation-positive non-small-cell lung cancer(EURTAC):a multicentre, open-label, randomised phase 3 trial. Lancet Oncol. (2012) 13:239–46. doi: 10.1016/S1470-2045(11)70393-X
11. Lynch TJ, Bell DW, Sordella R, Gurubhagavatula S, Okimoto RA, Brannigan BW, et al. Activating mutations in the epidermal growth factor receptor underlying responsiveness of non-small-cell lung cancer to gefitinib. N Engl J Med. (2004) 350:2129–39. doi: 10.1056/NEJMoa040938
12. Mok TS, Wu YL, Thongprasert S, Yang CH, Chu DT, Saijo N, et al. Gefitinib or carboplatin-paclitaxel in pulmonary adenocarcinoma. N Engl J Med. (2009) 361:947–57. doi: 10.1056/NEJMoa0810699
13. Maemondo M, Inoue A, Kobayashi K, Sugawara S, Oizumi S, Isobe H, et al. Gefitinib or chemotherapy for non-small-cell lung cancer with mutated EGFR. N Engl J Med. (2010) 362:2380–8. doi: 10.1056/NEJMoa0909530
14. He ZY, Li MF, Lin JH, Lin D, Lin RJ. Comparing the efficacy of concurrent EGFR-TKI and whole-brain radiotherapy vs. EGFR-TKI alone as a first-line therapy for advanced -mutated non-small-cell lung cancer with brain metastases: a retrospective cohort study. Cancer Manag Res. (2019) 11:2129–38. doi: 10.2147/CMAR.S184922
15. Li S, Li L, Zhu Y, Huang C, Qin Y, Liu H, et al. Coexistence of EGFR with KRAS, or BRAF, or PIK3CA somatic mutations in lung cancer: a comprehensive mutation profiling from 5125 Chinese cohorts. Br J Cancer. (2014) 110:2812–20. doi: 10.1038/bjc.2014.210
16. Lozano MD, Zulueta JJ, Echeveste JI, Gurpide A, Seijo LM, Martin-Algarra S, et al. Assessment of epidermal growth factor receptor and K-ras mutation status in cytological stained smears of non-small cell lung cancer patients: correlation with clinical outcomes. Oncologist. (2011) 16:877–85. doi: 10.1634/theoncologist.2010-0155
17. Rekhtman N, Ang DC, Riely GJ, Ladanyi M, Moreira AL. KRAS mutations are associated with solid growth pattern and tumor-infiltrating leukocytes in lung adenocarcinoma. Mod Pathol. (2013) 26:1307–19. doi: 10.1038/modpathol.2013.74
18. Paez JG, Janne PA, Lee JC, Tracy S, Greulich H, Gabriel S, et al. EGFR mutations in lung cancer: correlation with clinical response to gefitinib therapy. Science. (2004) 304:1497–500. doi: 10.1126/science.1099314
19. Kosaka T, Yatabe Y, Endoh H, Kuwano H, Takahashi T, Mitsudomi T. Mutations of the epidermal growth factor receptor gene in lung cancer: biological and clinical implications. Cancer Res. (2004) 64:8919–23. doi: 10.1158/0008-5472.CAN-04-2818
20. Ciardiello F, Tortora G. EGFR antagonists in cancer treatment. N Engl J Med. (2008) 358:1160–74. doi: 10.1056/NEJMra0707704
21. Liu WS, Zhao LJ, Pang QS, Yuan ZY, Li B, Wang P. Prognostic value of epidermal growth factor receptor mutations in resected lung adenocarcinomas. Med Oncol. (2014) 31:771. doi: 10.1007/s12032-013-0771-9
22. Lian W, Ouyang Y. CT-guided aspiration lung biopsy for EGFR and ALK gene mutation analysis of lung cancer. Oncol Lett. (2017) 13:3415–22. doi: 10.3892/ol.2017.5921
23. Maneechawakajorn J, Benjawongsathien D. Prognostic role of thyroid transcription factor-1 (TTF-1) in advanced and inoperable adenocarcinoma subtype of lung cancer among Thai patients. J Med Assoc Thai. (2014) 97 (Suppl 11):S11–17.
24. Gronberg BH, Lund-Iversen M, Strom EH, Brustugun OT, Scott H. Associations between TS, TTF-1, FR-alpha, FPGS, and overall survival in patients with advanced non-small-cell lung cancer receiving pemetrexed plus carboplatin or gemcitabine plus carboplatin as first-line chemotherapy. J Thorac Oncol. (2013) 8:1255–64. doi: 10.1097/JTO.0b013e3182a406a3
25. Shi X, Meng X, Sun X, Xing L, Yu J. PET/CT imaging-guided dose painting in radiation therapy. Cancer Lett. (2014) 355:169–75. doi: 10.1016/j.canlet.2014.07.042
26. Mamede M, Higashi T, Kitaichi M, Ishizu K, Ishimori T, Nakamoto Y, et al. [18F]FDG uptake and PCNA, Glut-1, and Hexokinase-II expressions in cancers and inflammatory lesions of the lung. Neoplasia. (2005) 7:369–79. doi: 10.1593/neo.04577
27. Ko KH, Hsu HH, Huang TW, Gao HW, Shen DH, Chang WC, et al. Value of (1)(8)F-FDG uptake on PET/CT and CEA level to predict epidermal growth factor receptor mutations in pulmonary adenocarcinoma. Eur J Nucl Med Mol Imaging. (2014) 41:1889–97. doi: 10.1007/s00259-014-2802-y
28. Kanmaz ZD, Aras G, Tuncay E, Bahadir A, Kocaturk C, Yasar ZA, et al. Contribution of (1)(8)Fluorodeoxyglucose positron emission tomography uptake and TTF-1 expression in the evaluation of the EGFR mutation in patients with lung adenocarcinoma. Cancer Biomark. (2016) 16:489–98. doi: 10.3233/CBM-160588
29. Lee SM, Bae SK, Jung SJ, Kim CK. FDG uptake in non-small cell lung cancer is not an independent predictor of EGFR or KRAS mutation status: a retrospective analysis of 206 patients. Clin Nucl Med. (2015) 40:950–8. doi: 10.1097/RLU.0000000000000975
30. Choi YJ, Cho BC, Jeong YH, Seo HJ, Kim HJ, Cho A, et al. Correlation between (18)f-fluorodeoxyglucose uptake and epidermal growth factor receptor mutations in advanced lung cancer. Nucl Med Mol Imaging. (2012) 46:169–75. doi: 10.1007/s13139-012-0142-z
31. Na II, Byun BH, Kim KM, Cheon GJ, Choe du H, Koh JS, et al. 18F-FDG uptake and EGFR mutations in patients with non-small cell lung cancer: a single-institution retrospective analysis. Lung Cancer. (2010) 67:76–80. doi: 10.1016/j.lungcan.2009.03.010
32. Lv Z, Fan J, Xu J, Wu F, Huang Q, Guo M, et al. Value of (18)F-FDG PET/CT for predicting EGFR mutations and positive ALK expression in patients with non-small cell lung cancer: a retrospective analysis of 849 Chinese patients. Eur J Nucl Med Mol Imaging. (2018) 45:735–50. doi: 10.1007/s00259-017-3885-z
33. Imyanitov EN, Demidova IA, Gordiev MG, Filipenko ML, Kekeyeva TV, Moliaka YK, et al. Distribution of EGFR mutations in 10,607 russian patients with lung cancer. Mol Diagn Ther. (2016) 20:401–6. doi: 10.1007/s40291-016-0213-4
34. Chung HW, Lee KY, Kim HJ, Kim WS, So Y. FDG PET/CT metabolic tumor volume and total lesion glycolysis predict prognosis in patients with advanced lung adenocarcinoma. J Cancer Res Clin Oncol. (2014) 140:89–98. doi: 10.1007/s00432-013-1545-7
35. Yoo SW, Kim J, Chong A, Kwon SY, Min JJ, Song HC, et al. Metabolic tumor volume measured by F-18 FDG PET/CT can further stratify the prognosis of patients with stage IV non-small cell lung cancer. Nucl Med Mol Imaging. (2012) 46:286–93. doi: 10.1007/s13139-012-0165-5
36. Ho TY, Chou PC, Yang CT, Tsang NM, Yen TC. Total lesion glycolysis determined per RECIST 1.1 criteria predicts survival in EGFR mutation-negative patients with advanced lung adenocarcinoma. Clin Nucl Med. (2015) 40:e295–299. doi: 10.1097/RLU.0000000000000774
37. Mei D, Luo Y, Wang Y, Gong J. CT texture analysis of lung adenocarcinoma: can Radiomic features be surrogate biomarkers for EGFR mutation statuses. Cancer Imaging. (2018) 18:52. doi: 10.1186/s40644-018-0184-2
38. Zhao FN, Zhao YQ, Han LZ, Xie YS, Liu Y, Ye ZX. Clinicoradiological features associated with epidermal growth factor receptor exon 19 and 21 mutation in lung adenocarcinoma. Clin Radiol. (2019) 74:80.e7–80.e17. doi: 10.1016/j.crad.2018.10.001
39. Ciuleanu T, Stelmakh L, Cicenas S, Miliauskas S, Grigorescu AC, Hillenbach C, et al. Efficacy and safety of erlotinib versus chemotherapy in second-line treatment of patients with advanced, non-small-cell lung cancer with poor prognosis (TITAN): a randomised multicentre, open-label, phase 3 study. Lancet Oncol. (2012) 13:300–8. doi: 10.1016/S1470-2045(11)70385-0
40. Wu YL, Lee JS, Thongprasert S, Yu CJ, Zhang L, Ladrera G, et al. Intercalated combination of chemotherapy and erlotinib for patients with advanced stage non-small-cell lung cancer (FASTACT-2):a randomised, double-blind trial. Lancet Oncol. (2013) 14:777–86. doi: 10.1016/S1470-2045(13)70254-7
41. Puglisi F, Aprile G, Bruckbauer M, Barbone F, Damante G, Guerra S, et al. Combined analysis of MIB-1 and thyroid transcription factor-1 predicts survival in non-small cell lung carcinomas. Cancer Lett. (2001) 162:97–103. doi: 10.1016/S0304-3835(00)00625-X
42. Chung KP, Huang YT, Chang YL, Yu CJ, Yang CH, Chang YC, et al. Clinical significance of thyroid transcription factor-1 in advanced lung adenocarcinoma under epidermal growth factor receptor tyrosine kinase inhibitor treatment. Chest. (2012) 141:420–8. doi: 10.1378/chest.10-3149
Keywords: lung adenocarcinoma, PET/CT, metabolic parameters, EGFR, prognosis
Citation: Yang B, Wang Q, Lu M, Ge Y, Zheng Y, Zhu H and Lu G (2019) Correlations Study Between 18F-FDG PET/CT Metabolic Parameters Predicting Epidermal Growth Factor Receptor Mutation Status and Prognosis in Lung Adenocarcinoma. Front. Oncol. 9:589. doi: 10.3389/fonc.2019.00589
Received: 12 April 2019; Accepted: 17 June 2019;
Published: 18 July 2019.
Edited by:
Bo Gao, Affiliated Hospital of Guizhou Medical University, ChinaReviewed by:
Xiaohua Zhu, Huazhong University of Science and Technology, ChinaCopyright © 2019 Yang, Wang, Lu, Ge, Zheng, Zhu and Lu. This is an open-access article distributed under the terms of the Creative Commons Attribution License (CC BY). The use, distribution or reproduction in other forums is permitted, provided the original author(s) and the copyright owner(s) are credited and that the original publication in this journal is cited, in accordance with accepted academic practice. No use, distribution or reproduction is permitted which does not comply with these terms.
*Correspondence: Hong Zhu, emhfenlAMTYzLmNvbQ==; Guangming Lu, Y2pyLmx1Z3VhbmdtaW5nQHZpcC4xNjMuY29t
†These authors have contributed equally to this work
Disclaimer: All claims expressed in this article are solely those of the authors and do not necessarily represent those of their affiliated organizations, or those of the publisher, the editors and the reviewers. Any product that may be evaluated in this article or claim that may be made by its manufacturer is not guaranteed or endorsed by the publisher.
Research integrity at Frontiers
Learn more about the work of our research integrity team to safeguard the quality of each article we publish.