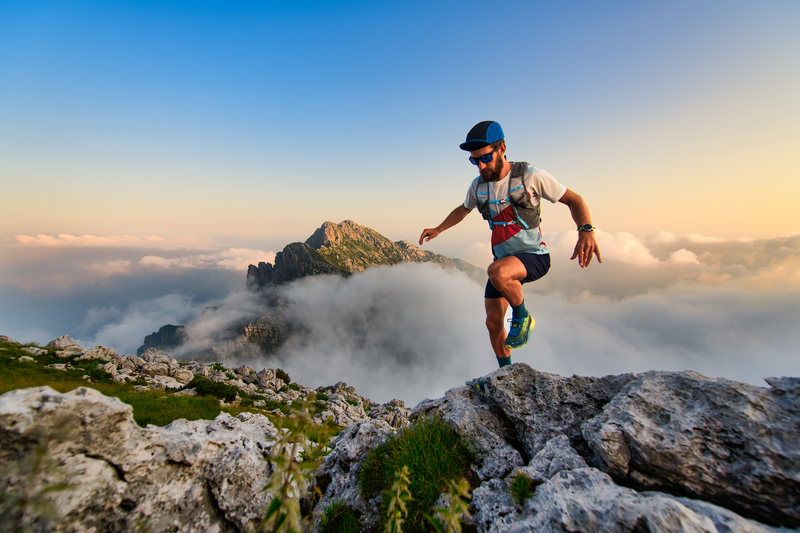
94% of researchers rate our articles as excellent or good
Learn more about the work of our research integrity team to safeguard the quality of each article we publish.
Find out more
ORIGINAL RESEARCH article
Front. Oncol. , 26 June 2019
Sec. Cancer Imaging and Image-directed Interventions
Volume 9 - 2019 | https://doi.org/10.3389/fonc.2019.00552
This article is part of the Research Topic Novel Methods for Oncologic Imaging Analysis: Radiomics, Machine Learning, and Artificial Intelligence View all 38 articles
Background: Conventional methods for predicting treatment response to neoadjuvant chemoradiotherapy (nCRT) in patients with locally advanced rectal cancer (LARC) are limited.
Methods: This study retrospectively recruited 134 LARC patients who underwent standard nCRT followed by total mesorectal excision surgery in our institution. Based on pre-operative axial T2-weighted images, machine learning radiomics was performed. A receiver operating characteristic (ROC) curve was performed to test the efficiencies of the predictive model.
Results: Among the 134 patients, 32 (23.9%) achieved pathological complete response (pCR), 69 (51.5%) achieved a good response, and 91 (67.9%) achieved down-staging. For prediction of pCR, good-response, and down-staging, the predictive model demonstrated high classification efficiencies, with an AUC value of 0.91 (95% CI: 0.83–0.98), 0.90 (95% CI: 0.83–0.97), and 0.93 (95% CI: 0.87–0.98), respectively.
Conclusion: Our machine learning radiomics model showed promise for predicting response to nCRT in patients with LARC. Our predictive model based on the commonly used T2-weighted images on pelvic Magnetic Resonance Imaging (MRI) scans has the potential to be adapted in clinical practice.
Novelty and Impact Statements: Methods for predicting the response of the locally advanced rectal cancer (LARC, T3-4, or N+) to neoadjuvant chemoradiotherapy (nCRT) is lacking. In the present study, we developed a new machine learning radiomics method based on T2-weighted images. As a non-invasive tool, this method facilitates prediction performance effectively. It achieves a satisfactory overall diagnostic accuracy for predicting of pCR, good response, and down-staging show an AUC of 0.908, 0.902, and 0.930 in LARC patients, respectively.
Rectal cancer is a common malignancy worldwide, accounting for ~30–50% of colorectal cancer (1, 2). Moreover, in rectal cancer patients, lesions are usually located in middle-low rectum, which causes increased difficulty in treatment and worse prognosis, especially the locally advanced rectal cancer (LARC, T3-4 or N+) (3, 4). Currently, neoadjuvant chemoradiotherapy (nCRT) followed by total mesorectal excision is the recommended treatment for LARC patients, especially those with lesions located in the middle-low rectum (5). The advantages of nCRT are usually significant (6, 7). However, the response of LARC to nCRT varies widely, ranging from pathological complete response (pCR, ypT0N0M0) with no viable tumor cells left in the surgical specimen, to virtually no tumor regression at all (stable) or even tumor progression in a small group of patients (8, 9). Among these patients, pCR is not only associated with favorable disease-free and overall survival (7, 10), but also motivates the “watch-and-wait” treatment strategy, a non-operative option for patients achieving clinical complete response (11). Therefore, clinicians are motivated to identify ways to accurately predict patients' individual responses to nCRT.
Radiological examination has been considered to be one of the means most likely to accomplish this task (12). Among all modalities, Magnetic Resonance Imaging (MRI) is regarded as the most promising method because it uses no radiation, shows high soft tissue resolution, and has wide routine clinical application for evaluation of rectal cancer. Notably, some conventional and functional MRI methods have been reported to show some advantages in predicting tumor response to nCRT (13–15). Unfortunately, conventional MRI analysis remains limited when predict treatment response in individual patient using experience (16). There is a need to develop new methods.
Quantitative image data analysis, such as texture analysis and radiomics are procedures for converting clinical images into high-dimensional, exploitable, and quantitative imaging features by high-throughput extraction of data-characterization algorithms (17). In addition to clinical outcomes, the biomedical information contained in medical images, such as overall information about phenotype and microenvironment of the tumor, may be vitally important for evidence-based clinical decision support. In theory, all magnetic resonance images in different can be used as a source of analysis. In theory, for quantitative analysis, used features can be extracted from images of all modalities (12, 16, 18–22). However, T2 weighted image is almost the most widely used one, when considering the wide availability of images which can be stably acquired based on different machines. Quantitative image data analysis methods have the potential to reveal such biomedical information, providing an opportunity to improve decision-support in oncology and non-invasively (17, 23). The potential advantage of this kind of method has already been verified in colorectal cancer (24) and a variety of other cancers, including nasopharyngeal carcinoma (25), lung cancer (17), and breast cancer (26). Recently, some independent studies (12, 19–22, 24) reported that a multimodality MRI based radiomics model could predict RC tumor response to nCRT with an improved accuracy for pCR and good response prediction. However, due to the relatively small sample size, or the inclusion of multimodality images with other MRI sequences such as diffusion-weighted imaging, or the lack of integration of important relevant clinical pathological features, there is a need for improving accuracy of the prediction model.
In the present study, we retrospectively collected 134 consecutive surgically and pathologically confirmed LARC patients who received standard nCRT before surgery. We developed a machine learning radiomics model based on imaging data extracted from the T2-weighted images, and validated its prediction efficiency of treatment response to nCRT in patients with LARC.
This retrospective study was approved by our institutional review board (IRB No. 201610070). The written informed consents from patients were waived.
Medical data of consecutive biopsy-proven rectal adenocarcinoma patients with LARC treated with nCRT followed by total mesorectal excision between March 2009 and December 2017 in our institution were retrospectively analyzed. Complete clinical data, including MRI imaging of all patient's performed before radiotherapy, was analyzed. Details about the inclusion and exclusion criteria, clinical and pathological characteristics, and treatments information can be found in Supplementary Files. The patients recruiting process was shown in Figure 1.
Figure 1. Flow-chart. LARC, Locally advanced rectal cancer; nCRT, neoadjuvant chemoradiotherapy; TME, total mesorectal excision.
Each specimen was sampled and evaluated by two experienced dedicated gastrointestinal pathologists. The two pathologists were both blind to the MRI data and clinical data. Criteria for pCRT and non-pCRT were defined as described in previous reports (8). We also classified TRG 3–4 into the good response (GR) group, and TRG 0–2 into the non-GR group according to Dowrak/Rödel's system (27). Changes in TNM staging were recorded by comparing to cTNM before the surgery, and responses were classed as either down-staging or non-down-staging (stability and progression). Details can be found in the Supplementary Files.
All patients underwent an MRI scan in our hospital with either a 1.5 Tesla (Siemens, Erlangen, Germany) or a 3.0 Telsa scanner (GE, Milwaukee, US), using a phased-array body coil, 3–10 days before the start of chemoradiation. To ensure MRI image quality, a quality assurance check was performed biweekly by a hospital radiological physicist and executed bimonthly by the Siemens or GE engineer, as appropriate, according to the maintenance rules for the MRI scanners in our institute. Axial T2-weighted (T2w fast spin echo sequence) images (T2WI) and T1-weighted (T1w spin echo sequence) images (T1WI) were acquired regularly. Subsequently, multiphase T1w images were obtained before and after contrast injection, using a spoiled gradient echo sequence (LAVA/VIBE sequence). Contrast injection and data acquisition were triggered simultaneously. Briefly, a total of four repetitions were acquired, including one before the contrast injection and three after the injection (at 28, 65, and 120 s). For contrast, generally 90–100 ml of the gadolinium-based contrast media dimeglumine gadopentetate (Magnevist; Schering Diagnostics AG, Berlin, Germany) was administrated intravenously at a rate of 2.5 ml/s through a high pressure injector (Optistar LE, Liebel-Flarisheim Company, OH, USA).
Since all patients had at least three kinds of MRI images (T1WI, T2WI, and enhanced T1WI), MRI images from these three serials were included in the present study.
All MRI images of each patient were evaluated independently by two experienced abdominal radiologists (reader 1. C.C with 7 years of experience; reader 2, L.X.Y with 15 years of experience), who were totally blinded to all medical information. Final disagreement was resolved in a panel format including two additional radiologists (L.W.H and Y.X.P). The location and boundary of the tumor were confirmed, tumor size, the distance from the lower edge of the tumor to the anal canal, and the MRI-based TNM stage were recorded. The findings were recorded by consensus.
For each patient, an anonymized representative axial T2WI image in which the lesion had the largest cross-sectional area was selected and retrieved from Picture Archiving and Communication System (PACS, Carestrem, Canada) using Digital Imaging and Communications in Medicine (DICOM) Works software (version 1.3.5). Subsequently, each image was transferred to a personal computer and inputted into the texture analysis software (MaZda Version 4.6, Institute of Electronics, Technical University of Lodz, Poland) (28).
Briefly, the process of texture analysis feature extraction was conducted by 2-steps as follows: (a) selecting and retrieving the suitable MRI images, and then (b) outlining the tumors as the regions of interest (ROI), and extracting quantitative texture analysis features by using the texture analysis software (MaZda Version 4.6, Institute of Electronics, Technical University of Lodz, Poland).
Tumors were outlined as a region of interest (ROI) by performing MaZda on T2WI, while using all other image sequences (especially gadolinium-enhanced images) as references in cases where the margin of the rectal lesion was difficult to define on unenhanced images. Briefly, a ROI was delineated initially by following the tumor outline, with notation that fat and air outside the mass are not included. Then, the ROI was saved for subsequent texture analysis. Contouring was performed carefully to cover the maximum extent of the tumor without exceeding the lesion border, to avoid contamination from adjacent normal rectal tissues or the intestinal lumen. For each ROI, a total of 340 quantitative features were automatically generated using MaZda software, including a gray level histogram, gradient, run-length matrix, co-occurrence matrix, autoregressive model, and wavelet transform analysis according to the software settings.
The reproducibility assessment of the features extracted by the two radiologists from the independent segmentations of T2WI images of all patients was performed. The inter-observer (reader 1 v reader 2) and intra-observer (reader 1 twice) correlation coefficient values were evaluated. The final consistency is evaluated by the following criteria regarding the correlation coefficient values: <0.20 indicates poor reproducibility, 0.21–0.40 fair reproducibility, 0.40–0.60 moderate reproducibility, 0.61–0.80 good reproducibility, and 0.81–1.00 excellent reproducibility. Generally, a correlation coefficient >0.75 is regarded as being in good agreement.
For the Kappa consistency test, excellent, good, and poor agreement were defined as kappa values of >0.81, in the range of 0.61–0.80, and <0.60, respectively.
The Mann-Whitney U-test was used to compare the values of each feature between the two groups. An independent samples t-test or Kruskal-Wallis H test, where appropriate, was used to assess the differences between the features generated by reader 1 (first time) and those generated by reader 2, as well as between the features generated twice by reader 1.
Inter-observer and intra-observer reproducibility of texture feature extraction was initially analyzed with 50 randomly chosen images from all T2WI images selected for evaluation by the two radiologists (reader 1, and reader 2). To assess the intra-observer reproducibility, reader 1 repeated the generation of texture features twice within a 2-week period following the same procedure. Reader 1 completed the workflow for the remaining images.
All statistical analyses were conducted using IBM SPSS version 20.0.0 (IBM Corporation, Armonk, NY, USA). To test the difference between groups, the Wilcoxon rank-sum test was performed for the quantitative features, and the chi-square test or fisher's exact test was performed for the qualitative features.
All data processing, data reduction and feature selection, and model built were performed using MATLAB 2017a (The Mathworks, Inc., Natick, MA, USA). The least absolute shrinkage and selection operator (LASSO) method, was used to select the most useful predictive features from the primary data set, and a radiomics score (Rad-score) was calculated for each patient at the mean time as a linear combination of selected features that were weighted by their respective coefficients. Based on these selected features, another classification model was also constructed by Random forest (RF), and the RF-score was generated. Subsequently, a combined classification model was finally built by the support vector machine (SVM) method (SVM-score), based on Rad-score and RF-score in the previous step. On the basis of two SVM-scores obtained, calculated from TE and TRC features, respectively, a final classification model was generated by using the SVM method again (SVM-score-final). Through the above steps, a total of seven models were generated representing each classification task, considering there are two kinds of data (Texture analysis [TA] features, and Traditional radiological-clinicopathological [TRC] features) that were used to build the model. The seven models include three models generated from TA features (model based on Rad-score, RF model, and first-step SVM model), three models generated from TRC features (model based on Rad-score, RF model, and first-step SVM model), and one combined SVM model.
The basic idea of this algorithm is to consider LASSO and RF as weak regressors and combine them using SVM. For each type of data, i.e., texture feature, we first use LASSO to obtain the Rad-score, and use its side product, i.e., important features, as the feature set of RF to obtain the RF-score. Since Rad-score and RF-score are independently acquired by two different weak regressors, using SVM to regress them in a two- dimensional plane achieves a better result than by them owns. Moreover, data sets Texture feature and Traditional radiological-clinicopathological data are also independent to each other. So for the same reason, we use SVM to regress the scores from Texture feature and Traditional radiological-clinicopathological data to get the final regression score. Finally, the regressed scores can be binarized for further prediction.
To evaluate the performance of the models, all patients were divided into two cohorts: a training cohort and a validation cohort. The models were developed in the training cohort, and tested in the validation cohort. The classification efficiencies of each kind of model mentioned above, including the receiver operating characteristic (ROC) curves, both in the training and validation cohort were calculated. A P-value < 0.05 was considered statistically significant. Details of the flow chart for building the classification model are shown in Figure 2.
Figure 2. Flow chart depicting construction of the classification models. LARC, locally advanced rectal cancer; TE, texture features; TRC Traditional radiological features and clinicopathological features; LASSO, least absolute shrinkage and selection operator; RF Random forest; SVM support vector machine. The nx or ny terms used here indicate the different numbers of selected features used in the LASSO method with three different reduction schemes based on 340 TE features and 31 TRC features, respectively. The texture analysis software used was MaZda Version 4.6 (Institute of Electronics, Technical University of Lodz, Poland).
There were134 patients enrolled in the present study. Patients' characteristics in the training and validation cohorts were summarized in Table 1. Patients were randomly allocated into training cohort and validation cohort in a 3:1 ratio for building the pCR predictive model, the down-staging model, and the good response model. No differences were found between the training and validation cohorts in any of the three models. In addition, the patients' clinicopathological data mostly consisting of laboratory data were also used in building the predictive models.
From a total of 340 features that were extracted from T2-wighted images for each patient, a set of features with corresponding numbers were selected by LASSO and used to calculate the Rad-scores for the pCR, Good Response, and Down-staging models.
On the basis of the selected 10 texture and 8 clinicopathological features, a predictive model was finally constructed with SVM method for pCR prediction. The SVM model yielded an AUC of 90.78% in the training cohort, and 87.45% in the validation cohort (Figure 3 and Supplementary Figure 1).
Figure 3. The efficiencies of machine learning models predicting treatment response in LARC patients receiving nCRT. The distribution of patients with down-staging disease or not (A–D), pCR or not (E–H), and good response or not (I–L), in TRC based model (model 6) (A,E,I), TE based model (model 3) (B,F,J) and the combined TRC and TE based model (Model 7) (C,G,K) were demonstrated by scatter plots. The ROC test (D,H,L) shows that the efficiency of model 7 was significantly higher than that of either model 6 or model 3 in all three missions (all P < 0.05). There is no significant difference in prediction efficiency between model 6 and model 3 in any of the three missions (all P > 0.05).
The predictive model built based on the 10 texture features and 7 clinicopathological features achieved an AUC of 90.17% in the training cohort, and 89.72% in the validation cohort (Figure 3 and Supplementary Figure 1).
The predictive model with 10 texture features and 7 clinicopathological features showed an AUC of 92.97% in the training cohort, and 89.20% in the validation cohort (Figure 3 and Supplementary Figure 1). Details about prediction efficiency of three kinds of models could be found in Table 2. The correlation matrix of the selected features used in the three kinds of models was showed as Figure 4.
Figure 4. Correlation matrix maps show the correlation among all TE and TRC features used in predictive models. (A) Down-staging model. (B) PCR model. (C) Good-response model. TRC features are expressed in bold fonts.
To the best of our knowledge, this was the first cohort studied to date utilizing monosequence-MRI-based machine learning radiomics to predict tumor response to neoadjuvant chemoradiation therapy in patients with locally advanced rectal cancer. Our predictive model constructed with both radiomics features and clinicopathological data achieved higher accuracies than previously reported in the literature, with an AUC of more than 90%. Substantial evidence from prior studies has demonstrated that a number of clinicopathologcial and radiological features may help to predict treatment response (16, 18, 29). Nevertheless, no single factor has stood out to be the most reliable way for clinicians to use in decision making process (6, 16). It is important to distinguish the LARC patients who will likely to respond to nCRT from patients who would not. However, this has not been achieved yet. We introduced here a new imaging oriented strategy for a better prediction, which may have potential for clinical practice.
Our study is in general accord with prior research (19, 30, 31). Nie et al. (12) have reported a relatively satisfactory result by using a radiomics method, with an AUC of 0.84 for pCR and 0.89 for good response prediction. Most recently, Cui et al. (19) reported a further attempt on a bigger group LARC patients by similar methods, which show very high predictive efficiency with an AUC of 0.944. In addition, several LARC studies (20, 21) also perform similar radiomics-based studies with good experimental results, using features extracted from multimodality MR images including T2WI. However, there were obvious advantages in our study when compared to these studies. First, we fully evaluated three aspects of the treatment response: not only pCR and good response, but also down-staging. Our study has the potential to provide more information on the tumor and treatment response. Second, the number of enrolled patients in our study (n = 134), was larger than that in the Nie's (n = 48), and comparable to Cui y's (n = 186), which can ensure the desired prediction results. Third, we also included conventional MRI findings and clinicopathological data which may further improve the prediction. Lastly, our radiomic features were extracted from only one sequence, i.e., the T2-weighted images, other than the multi-sequence MRI images used in previous studies. The T2 weighted images are commonly used in clinical practice, which is familiar to radiologists. In addition, it can be acquired easily and the images are quite stable in appearance, especially when compared with images obtained by special sequence, such as diffusion weighted images. Notably, diffusion weighted images are prone to distortion and susceptibility artifacts, which affect tumor segmentation and data extraction. Similarly, other sequences such as T1-weighted dynamic contrast enhanced images depend on the amount and distribution of the injected contrast-enhancing agent, which might be influenced by variable hemodynamic conditions in the patients.
The exact reason for why quantitative MRI-based texture data appear to be able to predict treatment response is still largely unknown. In theory, the biological phenotype of tumors, including treatment response, is largely determined by their underlying molecular subtypes, whose manifestations may vary. One of the phenotypes may be radiological heterogeneity, including inter- and intra-tumor heterogeneity. A large body of literature indicates that texture based radiomic modeling can evaluate tumor heterogeneity, and can correlate radiological findings with underlying genomic and biological characteristics, including prognosis and treatment response (17, 23). Our study may add into the literature in this regard as we have shown a predictive model for treatment response with high accuracy. From another perspective, the large amount of previous evidence (13, 15), supporting using advanced MRI-based radiomic features to predict different responses to nCRT in patients with rectal cancer.
In addition, we introduced clinicopathological features into the prediction model, which may contribute significantly to the improvement of prediction efficiencies. These features may represent, to some extent, some of the intrinsic properties of the tumor (32, 33). For example, the fecal occult blood test and red cell counts may indicate oxygen status of tumor. Neutrophil counts, Monocyte counts, globulin, or platelet counts, may actually reflect the immune status of LARC patients to some extent. The hypoxia and immune status of the tumor can influence tumor treatment response and mediate radiotherapy resistance (34, 35). Pathology type and distance from the anal verge also influence the tumor response, as has been shown in previous studies (36, 37). Our study results suggest that these clinicopathological data may play an important role in treatment response.
There were several limitations in our study. First, as a retrospective study, there may be a selection bias. Second, the sample size in our study was modest, which may affect the accuracy and stability of the predictive models. Third, both the building and validation of the models were conducted in our institution with a single dataset. A multicenter prospective study might be helpful to further validate and optimize our prediction models. The texture features were extracted from the largest cross-sectional area of the tumor rather than from the entire tumor, which may raise questions as to whether these features were optimally representative of the characteristics of the entire tumor. Lastly, the MRI images used in the texture feature extraction were obtained from three different MRI scanners (Siemens and GE) in our hospital, and differences among the scanners may potentially influence the texture features and the subsequent model building. Future research is needed to standardize the signal intensity among different MRI scanners.
Our study showed a predictive model built with radiomic features and clinicopathological data was promising to predict tumor response to neoadjuvant chemoradiation in patients with locally advanced rectal cancer. In addition, our method developed with information from the clinically obtained T2-weighted sequence may be used as a complimentary tool to assist clinical decision making. Nevertheless, future prospective multicenter studies with larger samples will be needed to validate our study result and to optimize the prediction models for clinical practice.
XY, QP, HP, and WeiL conceived and designed the experiments. XY, QP, and BC wrote the paper. All authors analyzed the data and contributed to reagents, materials, analysis tools, and also read and approved the final manuscript.
This study was supported by the following: the Nature Scientific Foundations of China (81702956, 81602399), the Postdoctoral Science Foundation of Central South University (No.185705), the Strategy-Oriented Special Project of Central South University in China (ZLXD2017003), and the Beijing CSCO Fund (Y-MX2014-002).
The authors declare that the research was conducted in the absence of any commercial or financial relationships that could be construed as a potential conflict of interest.
We thank Doctors Hongling Yin and Guanghui Gong, from the Department of Pathology (Xiangya Hospital, Central South University) for the detailed pathological analysis.
The Supplementary Material for this article can be found online at: https://www.frontiersin.org/articles/10.3389/fonc.2019.00552/full#supplementary-material
LARC, locally advanced rectal cancer; nCRT, neoadjuvant chemoradiotherapy; MRI, magnetic resonance imaging; RC, rectal cancer; CRC, colorectal cancer; pCR, pathological complete response; cCR, clinical complete response; AUC, area under curve; DWI, diffusion-weighted imaging; GR, good response; ROI, region of interest; ROC, receiver operating characteristic.
1. Bailey CE, Hu CY, You YN, Bednarski BK, Rodriguez-Bigas MA, Skibber JM, et al. Increasing disparities in the age-related incidences of colon and rectal cancers in the United States, 1975-2010. JAMA Surg. (2015) 150:17–22. doi: 10.1001/jamasurg.2014.1756
2. Gu J, Chen N. Current status of rectal cancer treatment in China. Colorectal Dis. (2013) 15:1345–50. doi: 10.1111/codi.12269
3. Liu Z, Xiong L, Ouyang G, Ma L, Sahi S, Wang K, et al. Investigation of copper cysteamine nanoparticles as a new type of radiosensitiers for colorectal carcinoma treatment. Sci Rep. (2017) 7:9290. doi: 10.1038/s41598-017-09375-y
4. Sun PL, Li B, Ye QF. Effect of neoadjuvant cetuximab, capecitabine, and radiotherapy for locally advanced rectal cancer: results of a phase II study. Int J Colorectal Dis. (2012) 27:1325–32. doi: 10.1007/s00384-012-1446-2
5. Schmoll HJ, Van Cutsem E, Stein A, Valentini V, Glimelius B, Haustermans K, et al. ESMO Consensus Guidelines for management of patients with colon and rectal cancer. a personalized approach to clinical decision making. Ann Oncol. (2012) 23:2479–516. doi: 10.1093/annonc/mds236
6. Dayde D, Tanaka I, Jain R, Tai MC, Taguchi A. Predictive and prognostic molecular biomarkers for response to neoadjuvant chemoradiation in rectal cancer. Int J Mol Sci. (2017) 18: E573. doi: 10.3390/ijms18030573
7. Zorcolo L, Rosman AS, Restivo A, Pisano M, Nigri GR, Fancellu A, et al. Complete pathologic response after combined modality treatment for rectal cancer and long-term survival: a meta-analysis. Ann Surg Oncol. (2012) 19:2822–32. doi: 10.1245/s10434-011-2209-y
8. Park IJ, You YN, Agarwal A, Skibber JM, Rodriguez-Bigas MA, Eng C, et al. Neoadjuvant treatment response as an early response indicator for patients with rectal cancer. J Clin Oncol. (2012) 30:1770–6. doi: 10.1200/JCO.2011.39.7901
9. Ryan JE, Warrier SK, Lynch AC, Heriot AG. Assessing pathological complete response to neoadjuvant chemoradiotherapy in locally advanced rectal cancer: a systematic review. Colorectal Dis. (2015) 17:849–61. doi: 10.1111/codi.13081
10. Maas M, Nelemans PJ, Valentini V, Das P, Rödel C, Kuo LJ, et al. Long-term outcome in patients with a pathological complete response after chemoradiation for rectal cancer: a pooled analysis of individual patient data. Lancet Oncol. (2010) 11:835–44. doi: 10.1016/S1470-2045(10)70172-8
11. Renehan AG, Malcomson L, Emsley R, Gollins S, Maw A, Myint AS, et al. Watch-and-wait approach versus surgical resection after chemoradiotherapy for patients with rectal cancer (the OnCoRe project): a propensity-score matched cohort analysis. Lancet Oncol. (2016) 17:174–83. doi: 10.1016/S1470-2045(15)00467-2
12. Nie K, Shi L, Chen Q, Hu X, Jabbour SK, Yue N, et al. Rectal cancer: assessment of neoadjuvant chemoradiation outcome based on radiomics of multiparametric MRI. Clin Cancer Res. (2016) 22:5256–64. doi: 10.1158/1078-0432.CCR-15-2997
13. Barbaro B, Vitale R, Valentini V, Illuminati S, Vecchio FM, Rizzo G, et al. Diffusion-weighted magnetic resonance imaging in monitoring rectal cancer response to neoadjuvant chemoradiotherapy. Int J Radiat Oncol Biol Phys. (2012) 83:594–9. doi: 10.1016/j.ijrobp.2011.07.017
14. Lu W, Jing H, Ju-Mei Z, Shao-Lin N, Fang C, Xiao-Ping Y, et al. Intravoxel incoherent motion diffusion-weighted imaging for discriminating the pathological response to neoadjuvant chemoradiotherapy in locally advanced rectal cancer. Sci Rep. (2017) 7:8496. doi: 10.1038/s41598-017-09227-9
15. Martens MH, Subhani S, Heijnen LA, Lambregts DM, Buijsen J, Maas M, et al. Can perfusion MRI predict response to preoperative treatment in rectal cancer. Radiother Oncol. (2015) 114:218–23. doi: 10.1016/j.radonc.2014.11.044
16. Ryan JE, Warrier SK, Lynch AC, Ramsay RG, Phillips WA, Heriot AG. Predicting pathological complete response to neoadjuvant chemoradiotherapy in locally advanced rectal cancer: a systematic review. Colorectal Dis. (2016) 18:234–46. doi: 10.1111/codi.13207
17. Aerts HJ, Velazquez ER, Leijenaar RT, Parmar C, Grossmann P, Carvalho S, et al. Decoding tumour phenotype by noninvasive imaging using a quantitative radiomics approach. Nat Commun. (2014) 5:4006. doi: 10.1038/ncomms5644
18. Al-Sukhni E, Attwood K, Mattson DM, Gabriel E, Nurkin SJ. Predictors of pathologic complete response following neoadjuvant chemoradiotherapy for rectal cancer. Ann Surg Oncol. (2016) 23:1177–86. doi: 10.1245/s10434-015-5017-y
19. Cui Y, Yang X, Shi Z, Yang Z, Du X, Zhao Z, et al. Radiomics analysis of multiparametric MRI for prediction of pathological complete response to neoadjuvant chemoradiotherapy in locally advanced rectal cancer. Eur Radiol. (2019) 29:1211–20. doi: 10.1007/s00330-018-5683-9
20. Giannini V, Mazzetti S, Bertotto I, Chiarenza C, Cauda S, Delmastro E, et al. Predicting locally advanced rectal cancer response to neoadjuvant therapy with 18F-FDG PET and MRI radiomics features. Eur J Nucl Med Mol Imaging. (2019) 46:878–88. doi: 10.1007/s00259-018-4250-6
21. Bibault JE, Giraud P, Housset M, Durdux C, Taieb J, Berger A, et al. Deep Learning and Radiomics predict complete response after neo-adjuvant chemoradiation for locally advanced rectal cancer. Sci Rep. (2018) 8:12611. doi: 10.1038/s41598-018-35359-7
22. Wang G, He L, Yuan C, Huang Y, Liu Z, Liang C. Pretreatment MR imaging radiomics signatures for response prediction to induction chemotherapy in patients with nasopharyngeal carcinoma. Eur J Radiol. (2018) 98:100–6. doi: 10.1016/j.ejrad.2017.11.007
23. Gillies RJ, Kinahan PE, Hricak H. Radiomics: images are more than pictures, they are data. Radiology. (2016) 278:563–77. doi: 10.1148/radiol.2015151169
24. Horvat N, Veeraraghavan H, Khan M, Blazic I, Zheng J, Capanu M, et al. MR Imaging of rectal cancer: radiomics analysis to assess treatment response after neoadjuvant therapy. Radiology. (2018) 287:833–43. doi: 10.1148/radiol.2018172300
25. Zhang B, He X, Ouyang F, Gu D, Dong Y, Zhang L, et al. Radiomic machine-learning classifiers for prognostic biomarkers of advanced nasopharyngeal carcinoma. Cancer Lett. (2017) 403:21–7. doi: 10.1016/j.canlet.2017.06.004
26. Valdora F, Houssami N, Rossi F, Calabrese M, Tagliafico AS. Rapid review: radiomics and breast cancer. Breast Cancer Res Treat. (2018) 169:217–29. doi: 10.1007/s10549-018-4675-4
27. Rödel C, Martus P, Papadoupolos T, Füzesi L, Klimpfinger M, Fietkau R, et al. Prognostic significance of tumor regression after preoperative chemoradiotherapy for rectal cancer. J Clin Oncol. (2005) 23:8688–96. doi: 10.1200/JCO.2005.02.1329
28. Szczypiński PM, Strzelecki M, Materka A, Klepaczko A. MaZda–a software package for image texture analysis. Comput Methods Programs Biomed. (2009) 94:66–76. doi: 10.1016/j.cmpb.2008.08.005
29. Das P, Skibber JM, Rodriguez-Bigas MA, Feig BW, Chang GJ, Wolff RA, et al. Predictors of tumor response and downstaging in patients who receive preoperative chemoradiation for rectal cancer. Cancer. (2007) 109:1750–5. doi: 10.1002/cncr.22625
30. Zhang S, Shi L, Mao D, Peng W, Sheng C, Ding C, et al. Use of jianpi jiedu herbs in patients with advanced colorectal cancer: a systematic review and meta-analysis. Evid Based Complement Alternat Med. (2018) 2018:6180810. doi: 10.1155/2018/6180810
31. Rossi L, Bijman R, Schillemans W, Aluwini S, Cavedon C, Witte M, et al. Texture analysis of 3D dose distributions for predictive modelling of toxicity rates in radiotherapy. Radiother Oncol. (2018) 129:548–53. doi: 10.1016/j.radonc.2018.07.027
32. Song X, Zhu H, Pei Q, Tan F, Li C, Zhou Z, et al. Significance of inflammation-based indices in the prognosis of patients with non-metastatic colorectal cancer. Oncotarget. (2017) 8:45178–89. doi: 10.18632/oncotarget.16774
33. Tan X, Wen Q, Wang R, Chen Z. Chemotherapy-induced neutropenia and the prognosis of colorectal cancer: a meta-analysis of cohort studies. Expert Rev Anticancer Ther. (2017) 17:1077–85. doi: 10.1080/14737140.2017.1380521
34. Formenti SC, Demaria S. Effects of chemoradiation on tumor-host interactions: the immunologic side. J Clin Oncol. (2008) 26:1562–3. doi: 10.1200/JCO.2007.15.5499
35. Ostheimer C, Gunther S, Bache M, Vordermark D, Multhoff G. Dynamics of heat shock protein 70 serum levels as a predictor of clinical response in non-small-cell lung cancer and correlation with the hypoxia-related marker osteopontin. Front Immunol. (2017) 8:1305. doi: 10.3389/fimmu.2017.01305
36. McCawley N, Clancy C, O'Neill BD, Deasy J, McNamara DA, Burke JP. Mucinous rectal adenocarcinoma is associated with a poor response to neoadjuvant chemoradiotherapy: a systematic review and meta-analysis. Dis Colon Rectum. (2016) 59:1200–8. doi: 10.1097/DCR.0000000000000635
Keywords: locally advanced rectal cancer (LARC), neoadjuvant chemoradiotherapy (nCRT), treatment response, magnetic resonance imaging (MRI), machine learning radiomics
Citation: Yi X, Pei Q, Zhang Y, Zhu H, Wang Z, Chen C, Li Q, Long X, Tan F, Zhou Z, Liu W, Li C, Zhou Y, Song X, Li Y, Liao W, Li X, Sun L, Pei H, Zee C and Chen BT (2019) MRI-Based Radiomics Predicts Tumor Response to Neoadjuvant Chemoradiotherapy in Locally Advanced Rectal Cancer. Front. Oncol. 9:552. doi: 10.3389/fonc.2019.00552
Received: 07 December 2018; Accepted: 06 June 2019;
Published: 26 June 2019.
Edited by:
Xuelei Ma, Sichuan University, ChinaReviewed by:
Ana Paula Candiota, Centre for Biomedical Network Research (CIBER), SpainCopyright © 2019 Yi, Pei, Zhang, Zhu, Wang, Chen, Li, Long, Tan, Zhou, Liu, Li, Zhou, Song, Li, Liao, Li, Sun, Pei, Zee and Chen. This is an open-access article distributed under the terms of the Creative Commons Attribution License (CC BY). The use, distribution or reproduction in other forums is permitted, provided the original author(s) and the copyright owner(s) are credited and that the original publication in this journal is cited, in accordance with accepted academic practice. No use, distribution or reproduction is permitted which does not comply with these terms.
*Correspondence: Haiping Pei, cGVpaGFpcGluZ3h5QDE2My5jb20=; Weihua Liao, ZG9jdG9ybGlhb3dlaWh1YUAxNjMuY29t
†These authors have contributed equally to this work
Disclaimer: All claims expressed in this article are solely those of the authors and do not necessarily represent those of their affiliated organizations, or those of the publisher, the editors and the reviewers. Any product that may be evaluated in this article or claim that may be made by its manufacturer is not guaranteed or endorsed by the publisher.
Research integrity at Frontiers
Learn more about the work of our research integrity team to safeguard the quality of each article we publish.