- 1Department of Botany and Plant Sciences, University of California, Riverside, Riverside, CA, United States
- 2Department of Urology, Guizhou Provincial People's Hospital, Guangzhou, China
- 3Department of Urology, Guangdong Key Laboratory of Clinical Molecular Medicine and Diagnostics, Guangzhou First People's Hospital, School of Medicine, South China University of Technology, Guangzhou, China
- 4Department of Mathematics, Bowdoin College, Brunswick, ME, United States
- 5Department of Pathology and Urology, Massachusetts General Hospital and Harvard Medical School, Boston, MA, United States
Diagnosis of the presence of tumors and subsequent prognosis based on tumor microenvironment becomes more clinically practical because tumor-adjacent tissues are easy to collect and they are more genetically homogeneous. The purpose of this study was to identify new prognostic markers in prostate stroma that are near the tumor. We have demonstrated the prognostic features of FGFR1, FRS2, S6K1, LDHB, MYPT1, and P-LDHA in prostate tumors using tissue microarrays (TMAs) which consist of 241 patient samples from Massachusetts General Hospital (MGH). In this study, we investigated these six markers in the tumor microenvironment using an Aperio Imagescope system in the same TMAs. The joint prognostic power of markers was further evaluated and classified using a new algorithm named Weighted Dichotomizing. The classifier was verified via rigorous 10-fold cross validation. Statistical analysis of the protein expression indicated that in tumor-adjacent stroma FGFR1 and MYPT1 were significantly correlated with patient outcomes and LDHB showed the outcome-association tendency. More interestingly, these correlations were completely opposite regarding tumor tissue as previously reported. The results suggest that prognostic testing should utilize either tumor-enriched tissue or stroma with distinct signature profiles rather than using mixture of both tissue types. The new classifier based on stroma tissue has potential value in the clinical management of prostate cancer patients.
Introduction
After decades of research, prostate cancer remains one of the leading worldwide concerns in male health (1, 2). Three major challenges need to be better addressed through biomarker studies to improve the management of the disease and save lives—early detection (3, 4), accurate prediction of patients' outcomes (5, 6), and development of effective personalized treatments for different types of prostate tumors (7). In the current study, we focused on the identification and verification of biomarkers that are associated with patients' outcomes, i.e., whether biochemical recurrence (BCR) will occur after prostatectomy, or how soon the disease will come back (e.g., time to BCR) if the disease does recur. Such biomarkers, once identified and validated, may be used in clinical applications to distinguish the patients who require surgery or/and adjuvant therapy from the patients who only need active surveillance (8–10).
Gene expression profiles have been widely scrutinized for years in order to develop expression signatures for the prediction of BCR status (3). Nevertheless, very few clinically applicable expression signatures have been developed, including Prolaris (46-gene test) from Myriad Genetics Inc., 22-gene test from Decipher Inc., and Genomic Prostate Score (17-gene test) from Oncotype DX, all of which still leave great room for improvement. The difficulty for development of prognostic markers from prostate tumor tissue could be due to the heterogenic nature of the prostate tumors and also because of the offsetting expression of signature genes in the tumor vs. its microenvironment, which makes it difficult to utilize these gene markers in a mixture of tissues (a new discovery of this study). A statistic-relevant explanation could be owing to the relatively small sample size in individual studies such that in each study the sample did not fully represent the heterogeneous population of prostate tumors (3, 6, 11–13). Another possibility could be that gene expression is controlled by many complicated biological systems, such as subtle gene networks (interaction among genes), epigenetic modification, small RNA interference, and transcriptional modification such as alternative splicing (14, 15). Multiple biochemical steps are involved from DNA to protein through the Central Dogma. It has been well-assumed that proteins (products of gene transcription) directly reflect genes' functions which play critical roles in biological processes in vivo. However, there is not always a correlation between protein expression and mRNA expression (16, 17). Therefore, the quantification of protein might be better correlated with the phenotypes of interest, such as aggressiveness of the cancer. In many studies, the expression of a protein has been used as a measure of a disease phenotype, for example, prostate-specific antigen (PSA), prostate-specific membrane antigen (PSMA), prostatic acid phosphatase (PAP) and prostate stem cell antigen (PSCA)/ alpha-fetoprotein (AFP), carcino-embryonic antigen (CEA), and cancer antigen (CA-125) (18–23). Therefore, predictive models based on the progression-associated antigens (protein markers) may potentially have increased prognostic power compared to the models that are solely based upon the gene expression profiles.
In our previous studies, we have demonstrated the prognostic potential for six proteins (FGFR1, FRS2, S6K1, LDHB, MYPT1, and P-LDHA) using a tissue microarray (TMA) system that was developed in-house at Massachusetts General Hospital (MGH) (5, 24–26). All the protein expression data were obtained through manual evaluation of the TMAs by pathologists, which may have led to possibly inconsistent readings and an artificially enlarged variation of the data (27). These six proteins showed differentially expressed levels between relapsed tumors and non-relapsed tumors. Independent RT-PCR experiments demonstrated similar expression patterns in mRNA levels for these proteins. In the current study, we explored the possibility of extending the application of these prognostic markers to the tumor microenvironment. It is difficult to diagnose the presence of prostate tumors, especially in their early stage, with biopsies due to their small volumes. Thus, methods have been developed to detect prostate tumors using surrounding tissues (with significant larger effective volumes) based on the assumption that tumor-adjacent tissues are restructured by the nearby tumor via paracrine (3, 28–30). We hypothesized that tumor microenvironments respond differentially to aggressive tumors vs. indolent tumors, and such differences, including differentially expressed proteins, may be used as signatures for prognosis.
To test this hypothesis, we reevaluated these MGH TMAs by only selecting the non-tumor regions that are adjacent to tumors. The array data of these six proteins were individually analyzed using an automated Aperio Imagescope system, as described in our previous study in PCa diagnosis with tumor-free cells (30), rather than manual evaluation. The Aperio system utilized a single standard to read the TMA image and calculated the average immunohistochemistry reaction intensity for each sample on the TMA, yielding reliable expression data. Additional within array normalization was carried out to remove potential systematic differences between various batches of array reactions. The objective data were then analyzed using various statistical methods to evaluate the association between each of the protein markers and BCR status or time to BCR. We found in tumor-adjacent stroma that FGFR1 was negatively associated with the risk of BCR (p-value = 0.005), MYPT1 was positively associated with the risk of BCR (p-value = 0.008), and LDHB showed a positive-association tendency (p-value = 0.164); however, such association patterns, either positive or negative, were completely opposite to what had been reported in tumor tissues (5, 24–32). We refer to “opposite” the distinct association patterns between the protein expression and risk of recurrence in the tumor site versus the tumor-adjacent stroma site. This interesting phenomenon may reflect the intricate interaction between tumor and microenvironment, the understanding of which may benefit clinical diagnosis and prognosis of the disease. Moreover, the results suggested prognosis of prostate cancer should be based on either highly enriched tumor tissue or stroma tissue (close to tumor) with distinct signature profiles rather than a mixture of both tissue types.
A newly developed approach, named Weighted Dichotomizing, was used to train a predictive classifier using these three prognostic markers. The results showed an accuracy of 71% in predicting the BCR status for the patients using the classifier. The model has been verified using 10-fold cross validation. The properties of the new predictive classifier, its clinical potential, and the potential for improvement have been discussed.
Materials and Methods
Prostate Tissues and Tissue Microarray (TMA) Assays
TMAs include formalin-fixed and paraffin-embedded (FFPE) specimens from 241 patients who were confirmed to have PCa and received radical prostatectomy at MGH from September 1993 to March 1995 (5, 24–27).
The study was approved by the human study ethics committees at Massachusetts General Hospital (Boston, MA) and the Ministry of Public Health of the People's Republic of China. All specimens in this study were anonymously handled according to ethical and legal standards.
The clinicopathological characteristics of all cases represented on TMAs are summarized in Table S1, and the data for the 105 cases (36 aggressive cases and 69 indolent cases) are summarized in Table 1. Clinicopathological data including pre-operation PSA, Gleason scores (GS, reassigned based on the current grading recommendation provided by the International Society of Urological Pathology), American Joint Committee on Cancer (AJCC) T stage, surgical margin status, time to biochemical recurrence (BCR) or PSA failure, time to metastasis, and overall survival time have been collected. The time to BCR was defined as the time interval between initial operation and first appearance of two consecutive rises of PSA. The time to metastasis was defined as the time interval between the initial operation and the detection of metastatic sites. The overall survival time was calculated from the date of surgery to the data of the last follow-up or death. None of the patients or subjects recruited for the study had chemotherapy or radiotherapy before the surgery. All tissues were reconfirmed by HE staining.
Immunohistochemical staining of formalin-fixed and paraffin-embedded sections was performed using a standard immunohistochemistry (IHC) protocol. Briefly, after deparaffinization and rehydration using a Leica autostainer XL ST5010 system, the TMA slides were pretreated with 10 mM sodium citrate buffer (pH 6.0) for 5–10 min in a microwave for antigen retrieval. The endogenous peroxidase was quenched by adding the hydrogen peroxide (3% H2O2 in 70% methanol) at room temperature for 15 min. After washing, the slides were blocked for 30 min. The blocking buffer was removed and the slides were then incubated for 1 h with primary antibodies (FGFR1, FRS2, LDHB, MYPT1, P-LDHA, and S6K1), respectively, with the optimized dilutions at room temperature. The catalog numbers for these six antibodies are ab10646 (Abcam Co Ltd, USA), sc-8318 (Santa Cruz, CA, USA), ab85319, (Abcam Co Ltd, USA), ab59235 (Abcam Co Ltd, USA), 8176 (Cell Signaling Technology, CA, USA), and ab32359 (Abcam Co Ltd, USA), respectively. The optimized dilutions for the six antibodies in the TMA reactions are 1:200, 1:200, 1:200, 1:300, 1:200, and 1:50 respectively.
Slides were washed with the 1×PBS solution and further incubated with DAKO Envision+/HRP for 30 min at room temperature. Detection was based on the use of the 3, 3′-diaminobenzidene as instructed (DAB kit, DAKO, Denmark). Slides were counterstained with hematoxylin before microscopic analysis. An H-Score was initially calculated based on scoring of stained cells according to published method (33).
Image Analysis
The expressions of each protein in a TMA were measured by analyzing the staining signal intensity using Aperio image scope v11 (Aperio, USA). Briefly, in Aperio Imagescope windows, epithelial cancer cells and tumor-free micro-environmental area were compartmentalized by an experienced pathologist using pen tool, based on typical pathological features. The brown staining (positive) in the intensely stained image and the blue staining (negative) in the least intensely stained area were selected for further data processing. The subsequent staining intensity was measured as the densitometry of the digital image (×400), and the counted positive pixels were transformed to three intensity bins.
A total of 181 tumor-bearing cases were considered in the study. For each case, tumor areas were first identified by an experienced pathologist with the aid of the Aperio Imagescope system. The pen tool in the Aperio Imagescope system was then used to select stroma that were close to tumor regions from each IHC image (shown in Figure 1), and the image data were then translated to numerical data, such as intensities of positive signal, intensities of negative signal, number of positive signals, and number of negative signals. The average intensity, which is the ratio of the sum of the intensities of positive signals (weak positive, positive, and strong positive) and the sum of the number of positive signals (weak positive, positive, and strong positive), is calculated and used for further statistical analysis.
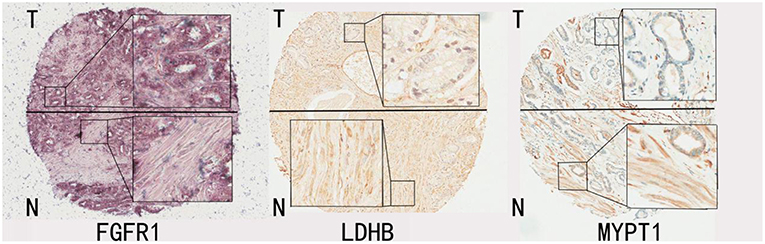
Figure 1. Representative IHC images for FGFR1, LDHB, MYPT1. The tissue regions labeled with T mainly represent tumor enriched area from patients' prostate glands; the tissue regions labeled with N represent tumor stroma areas of the prostate glands from the same patients.
Redefinition of Study Cases
From the 181 tumor-bearing cases, we redefined 36 aggressive cases as the patients who had experienced BCR within 3 years after surgery removal of the gland and 69 indolent cases as the patients who did not show BCR for 6 or more years after the surgery.
Basic Statistical Analyses
Pearson's Correlation Analysis was used to evaluate the relationship between the protein markers and the clinical variables. Survival analyses including Cox regression model and Kaplan-Meier were used to assess the association between protein markers and time to BCR. ROC curve and area under the curve (AUC) were used for the evaluation of the classification model. A p-value ≤ 0.05 was used for claiming a significant result for the statistical tests. Box plot and density plot were used for examining the distribution of the expression levels of each protein based on the patient's disease phenotype variables.
Weighted Dichotomizing Algorithm
We proposed to develop a composite predictive classifier using a multi-marker signature when different markers have various levels of predictability. The training set was first divided into a few subgroups based on a certain observed criterion, for example the binary clinical outcome of BCR status in the current study (aggressive group indicated by 1 and indolent group indicated by 0). For each marker, we sorted the patient cases based on the marker's expression level from lowest to highest. We sequentially used each of the sorted expression values of the marker as a cutoff to define predicted aggressive cases and predicted indolent cases. If the marker represents a protein product of an oncogene (i.e., higher expression levels are associated with more aggressive cases), the training cases with expression levels less than the cutoff were defined as predicted indolent cases and the training cases with expression levels greater than the cutoff were defined as predicted aggressive cases. If the marker is a product of a tumor suppressor gene, we defined the predicted indolent cases and predicted aggressive cases in the opposite way. The predicted indolent/aggressive classifications were then compared to the observed indolent/aggressive classifications to calculate the classification accuracy. Note that each cutoff was associated with a classification accuracy rate. In the process of developing a composite classifier, the cutoff with the highest classification accuracy was selected as the optimal cutoff for the marker, and the highest classification accuracy was used to calculate the weight for the marker in the composite classifier. A composite multi-marker classifier consisted of two components for classification calculation, i.e., the optimal cutoffs and the weights for the markers. Two steps were involved in the classification calculation when the composite classifier was applied to a test patient case. Suppose the composite classifier was composed of k markers or proteins (for example, k = 3 in the study because 3 proteins were analyzed). First, for the ith marker, we used the optimal cutoff to predict the patient outcome Si, with Si = 1 for aggressive case and Si = 0 for indolent case, where i = 1 to k. The weighed score for the outcome was calculated using the following formula:
where wi was the weight for the ith marker which was calculated as:
where ai was the highest classification accuracy that was achieved for the ith marker in the training process. The test patient was predicted as an aggressive case if S ≥ 0.5 and predicted as indolent case if S < 0.5.
Results
Quantifying Expression Levels of Protein Markers Using an Aperio System
The images of selected tumor-adjacent stroma regions on all the tissue microarrays were analyzed and transformed to numerical expression values (average intensity I) using an Aperio System (see Figure 1). Normalization had been carried out for each sample within a TMA using the following formula
where I and I' are the original and adjusted intensities, respectively, for a sample, M is the median intensity value for all the samples on the TMA, and R is the range of the intensity values for all the samples on the TMA. We only selected tumor-bearing tissues from a total of 181 patients who did not receive any adjuvant therapies after surgery for the study.
Statistical Analysis of the Association Between Markers and Clinical Variables
Pairwise Pearson's correlation was first examined among the six proteins and with four clinical variables including pre-operation PSA, Gleason score, time to biochemical recurrence (BCR), and time to metastasis. No significant correlation was detected between any protein marker and the four important clinical variables which have been widely utilized in disease management (Figure 2).
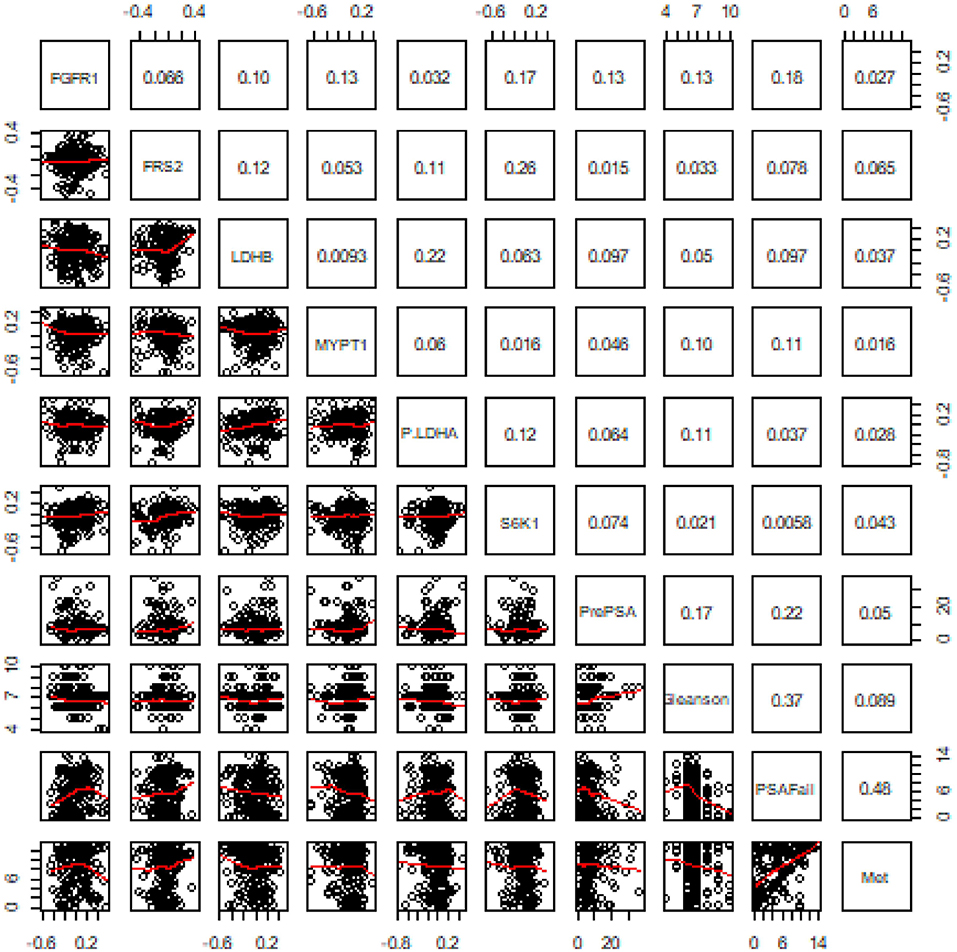
Figure 2. Results of pairwise correlation between six proteins and four clinical variables. The numbers in the grids are correlation coefficients in the pairwise correlation analysis.
We then analyzed the association between each of these 6 proteins and patients' biochemical recurrence (BCR) time using survival analysis. The patient cases were divided into two groups (H and L) based on the median expression value for the protein (5, 25, 34–41); the cases with expression levels greater than the median expression value were placed in group H and the cases with expression levels less than the median expression value were placed in group L. Kaplan-Meier curves were plotted in Figure 3 and p-values indicating the level of difference in survival between H and L groups were calculated using a Cox regression model (42). The results showed that FGFR1 (p-value = 0.005) and MYPT1 (p-value = 0.008) are significantly associated with the time to BCR, and LDHB (p-value = 0.164) is also relevant to the aggressiveness of the disease (Figure 3), which is consistent with the boxplots in Figure 4. However, the outcome-associations for these three proteins in tumor associated stroma are completely opposite to what we have observed in tumor tissues.
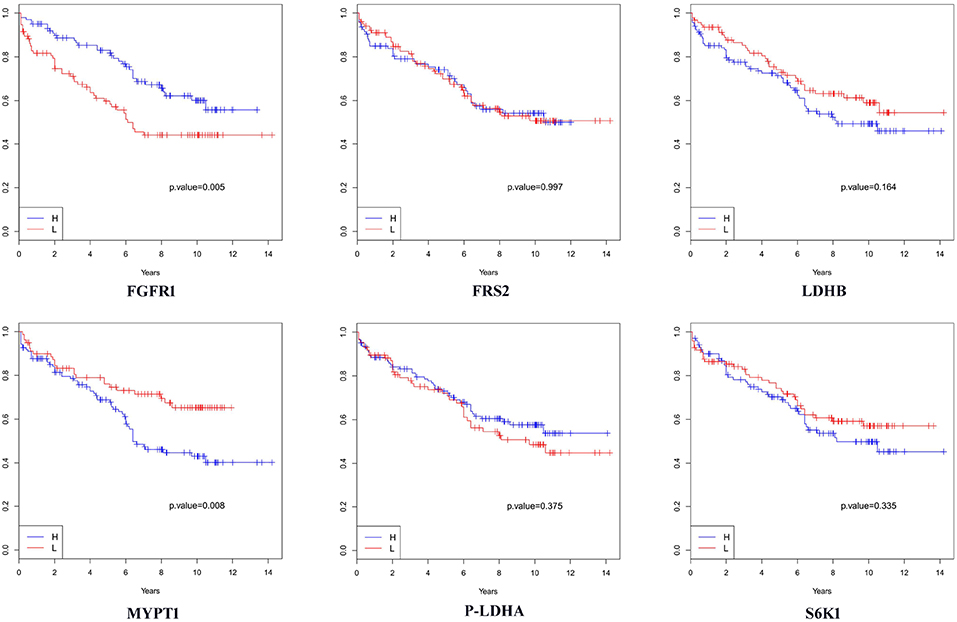
Figure 3. Survival analysis in terms of biochemical recurrence when tumor-adjacent stroma tissues were used. H: the subgroup of cases with expression levels greater than the median value. L: the subgroup of cases with expression levels less than the median value.
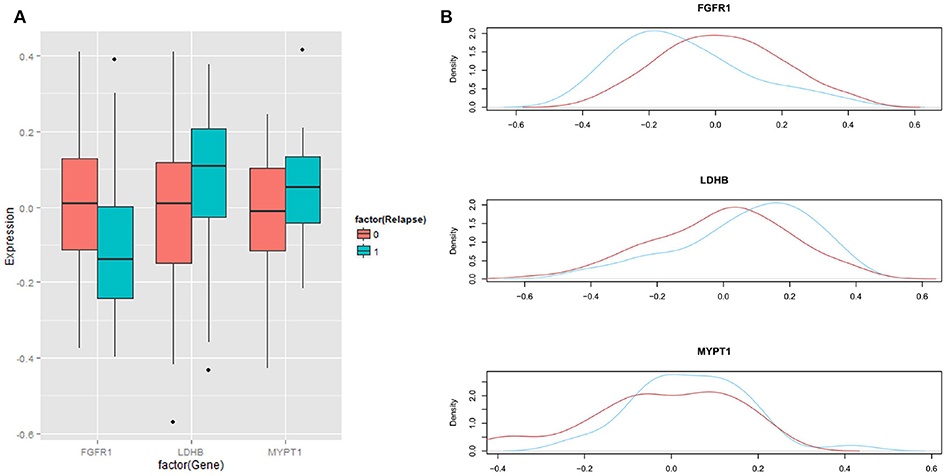
Figure 4. Distribution of expression levels in regard with BCR status. (A) Boxplots showing the differences in expression of three proteins between aggressive cases (labeled as 1 or blue) and indolent cases (labeled as 0 or red). (B) Distributions of expression of three proteins between aggressive cases (blue) and indolent cases (red).
Evaluation of Three Markers Jointly Using Weighted Dichotomizing Method
In order to evaluate the prognostic potential for these three protein markers simultaneously, we developed a novel algorithm, named Weighted Dichotomizing (WD), for developing a multi-marker predictive classifier. There is a total of 36 aggressive cases and 69 indolent cases in the training set (see Materials and Methods). For each of the three proteins, we sorted the patient cases based on the expression level of the protein and then systematically searched for the threshold expression value for this protein that optimally distinguished the aggressive cases from the indolent cases, i.e., the cutoff expression value with the highest classification accuracy (Figure 5).
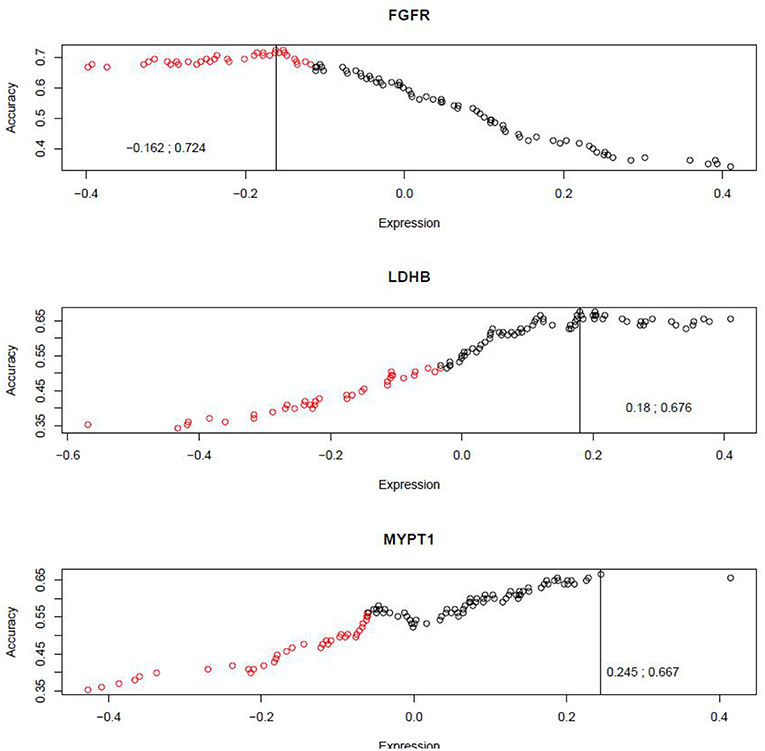
Figure 5. Systematic search for the optimal cutoffs. The x-axis represents the expression scores for protein markers, and the y-axis represents the classification accuracies achieved by various cutoff expression values.
A composite classifier was developed based on the calculation of the sum of the weighted classification using the three selected threshold values (as described in Materials and Methods). The weights used for the three markers were the highest classification accuracies (0.724, 0.676 and 0.667) that were achieved by the individual threshold expression values for the markers. When the classifier was applied to the training set, the classification accuracy or area under the curve (AUC) was 0.71 (see Figure 6 for ROC curve), with positive predictive value (PPV) and negative predictive value (NPV) being 0.70 and 0.69, respectively. We also verified the method using 10-fold cross validation as described below. The 105 patients (36 aggressive cases + 69 indolent cases) were arbitrarily divided into ten portions which were roughly of equal size with about 3 aggressive cases and 6 indolent cases in each portion. In each cross-validation step, we developed a classifier (as described above) using nine portions (~90%) of the cases and tested the classifier on the remaining one portion (~10%) which was not used for classifier development. We iteratively repeated this cross validation until each of the ten portions had been used exactly once for testing. Thus, we have predicted outcomes for each patient which can be compared with the observed outcomes to evaluate the performance of the classifier. The overall accuracy for the 10-fold cross validation was 0.66.
Comparison of Prognostic Factors Using Survival Analysis
Univariate and multivariate analyses using the Cox Proportional-Hazards Model were performed to compare prognostic factors including the profiles the three 3 proteins (FGFR, LDHB, and MYPT1), the composite scores (WD Score) calculated from these protein profiles using the WD algorithm, and four primary clinical variables, i.e., Pre-OP PSA, Gleason score, Margin, and Stage (Table 2). The results of univariate analysis showed that the profiles of each of three proteins are significantly correlated with the time to BCR, however, the composite scores (WD Score) with the combined protein profiles demonstrated substantially stronger association with BCR, indicating an increase in prognostic power by combining prognostic biomarkers. The univariate analysis also showed that all four well-known pathological variables were individually associated with the time to BCR, with the Gleason score being the strongest predictor.
When the WD Score was combined with the four pathological variables in the multivariate analysis, Gleason, WD Score and pre-OP PSA were still statistically significantly associated with the time to BCR (in a descending order), whereas the previous associations of Margin or Stage with the time to BCR in the univariate analysis had vanished. These results indicated (1) the prognostic information provided by Margin or Stage in the univariate analysis may be well-represented by the data of either protein profiles or other clinical variables, and (2) combining protein profiles with nomograms based on clinical characteristics will potentially increase prognostic accuracy.
Discussion
We investigated the profiles for six protein markers, FGFR1, FRS2, S6K1, LDHB, MYPT1, and P-LDHA, in tumor-adjacent stroma. The results indicated that FGFR1, MYPT1, and LDHB are relevant to disease progression, i.e., FGFR1 is negatively correlated with tumor progression whereas MYPT1 and LDHB are positively correlated with tumor progression in tumor-adjacent stroma. However, these correlations in tumor-adjacent stroma (in the current study) are completely opposite those in tumor tissue (as previously reported (5, 24–27, 31, 32)). Such a difference may reflect the intricate interaction between tumors and their associated stroma tissues. More importantly, the results suggested that prognosis of prostate cancer should be based on either highly enriched tumor tissue or stroma tissue (close to tumor) with distinct signature profiles rather than a mixture of both tissue types. This is because the expression of these marker genes in two tissue types may be offset in the mixture samples, yielding ambiguous test results.
Due to the heterogenic nature of the prostate tumors, it has been difficult to develop clinically useful biomarkers for prognosis. Compared to tumor tissues, tumor-adjacent stroma is much more genetically stable and homogenous. As the cancer develops, grows, and progresses, the stroma tissue in the surrounding microenvironment co-evolves into an activated state through continuous paracrine communication. Studies have suggested that tumors restructure surrounding stroma tissues such that these tumor-adjacent stroma tissues become quite different from the remote stroma, and such differences depend on the tumors' properties, i.e., aggressive tumors and indolent tumors (43, 44). However, research on prognostic markers in tumor-adjacent stroma has been inadequate. In this study, we mainly focused on the exploitation of prognostic markers in tumor-adjacent stroma using existing TMA data.
FGFR1 has been intensively studied in prostate cancer (45–47). In the normal human prostate gland, expression of FGFR1 is restricted to stroma and is not expressed in epithelial cells (48). Nevertheless, prostate tumors exhibit aberrant expression of FGFR1 in epithelial carcinoma cells (49, 50), and increased expression of FGFR1 seemed to be associated with aggressive tumors. However, contradictory results have been reported for the FGFR family—for example, down-regulation of FGFR2 is associated with neoplastic progression (49–52). Moreover, previous studies found that cloned FGF family epithelial cells from the non-malignant PCa, when implanted in rat hosts in the absence of stromal cells, can progress to malignant PCa (53). As a key regulator of vascular smooth muscle, MYPT1 is a member of the myosin phosphatase targeting protein (MYPT) family, which is most abundant in smooth muscle cells (54). Expression of MYPT1 is associated with many human diseases, including cancers (55–57). A previous study also revealed that MYPT1 could affect the cell cycle, migration, and adhesion processes of cancer cells (55). Our previous study indicated that MYPT1 stimulation could antagonize the pro-tumor effect induced by Mir 30d-upregulation in PCa epithelial cells (25). Nevertheless, our current study showed that MYPT1 in tumor stroma was positively correlated with tumor progression. As for LDHB, it is one of the subunits of LDH which catalyzes the reversible conversion of pyruvate to lactate. It has been reported that reduced expression of LDHB is associated with progression of PCa and other forms of cancer (58–60). A previous study showed that loss of LDHB expression in prostate cancer was due to promoter hypermethylation (32). However, our results indicated that LDHB was positively correlated with tumor progression in tumor-adjacent stroma, which was also opposite to the previous study in tumor tissues. As discussed above, studies are needed to disclose tumor-stroma interactions.
It has been routine that pathologists or specially trained personnel read the TMA image file to provide scores for evaluating the expression levels for the protein of interest. These scores often take ordinal form, for example, 0 for negative signal, 1 for weak signal, 2 for moderate signal, and 3 for strong signal. The ordinal scale does not provide precise measurement because the intermediate levels between the ordinal numbers are not represented. Moreover, different pathologists may use different standards which are based on individual experiences, sometime yielding discordant data. The Pearson's correlation test between the scores provided by three pathologists showed poor concordance between them (Figure S1). In the study, we evaluated the TMAs to provide quantitative data using an automated way through the image software Aperio image scope v11 (Aperio, USA), avoiding potential bias or erroneous scoring due to manual work. Additional normalization was used to remove systematical errors between different batches of the arrays to achieve reliable data. Thus, TMAs analyzed using different antibodies with different batches may be analyzed at the same time to increase analytic power and reduce manpower.
Simple Pearson's correlation could not identify any substantial association between protein markers or between any marker and clinical variables. The results indicated that the markers, if they are relevant to a certain clinical outcome, may not be linked to the clinical variable in a linearly related manner. For example, a threshold expression level may exist for a protein marker such that patients with protein expression higher than that threshold exhibit one phenotype whereas patients with protein expression lower than that threshold have another phenotype; however, no correlation can be detected if we simply calculate the Pearson's correlation coefficient based on all the patients. This was also true when we used the median expression level as a cutoff to subdivide the training set into two equal groups and checked the survival in regard to BCR. It is also in agreement with the various dominance-recessive relations between phenotypes that are due to the gene dosage, i.e., haplo-sufficient or haplo-insufficient (61). The survival analysis indicated that FGFR1 (tumor suppressor gene), LDHB (oncogene), and MYPT1 (oncogene) are all relevant to the time to BCR; however, the lack of correlation among these three markers themselves well-support the “threshold” theory. When we check the distribution of expression levels for these three proteins between BCR status (0 for non-relapse and 1 for relapse), there was a substantial overlap between the non-relapse group and the relapse group. The results suggested that to distinguish aggressive cases from indolent cases, we need to use both the BCR status (relapse/non-relapse) and the time to BCR to deal with the censored data. From a total of 181 tumor-bearing cases, we redefined 36 aggressive cases as the patients who had experienced BCR within 3 years after surgical removal of the gland, and redefined 69 indolent cases as the patients who did not show BCR for 6 or more years after the surgery. Indeed, the aggressive and indolent cases here refer to the early relapse cases and non-relapse cases with long follow-up period, respectively. The censored data in clinical studies can also be properly analyzed by the Cox Proportional-Hazards Model. Table 2 showed that the Cox regression can detect the associations between protein profiles or well-known pathological variables with the time to BCR which had been missed by Pearson's correlation analysis. The multivariate Cox regression analysis suggested that protein markers and clinical variables may correlate with disease outcomes in different manners; thus, combining different types of predictors has potential to increase prognostic power, which warrants future research.
We developed a new algorithm, named Weighted Dichotomizing, to uncover the association between the protein markers and the aggressiveness of the tumors that are embodied by the BCR status and the time to BCR. For each marker, we first identify the threshold (or cutoff value) that optimally separates aggressive cases from indolent cases with the highest classification accuracy. We then combined the markers to form a composite classifier by considering the different predictive potentials for the markers, i.e., the combined model put more weight on the protein markers that had achieved higher classification accuracies in training. The prognostic classifier trained using the proposed method achieved an overall accuracy of 71% based on only three protein markers, and the approach was verified by 10-fold cross validation, with an overall accuracy of 66%. Note that only 3 protein marks and 105 patient samples have been used for development of the model. The performance of the classifier will increase if more markers and more patient samples are used to develop the composite model.
The in-house TMAs by MGH may be used to identify and validate more potential protein markers which could be identified by mining the publicly available databases, such as The Cancer Genome Atlas (TCGA) (62). However, in order to translate to clinical use, other platforms for easily analyzing proteins are needed, for example Quantitative Infrared Westerns (63), reverse phase protein microarrays (64), or isobaric tags for relative and absolute quantitation (65). Multicolor staining technology should be applied to future study. In our own future study, we will identify and validate more protein markers using the MGH TMAs and develop a composite classifier with improved accuracy. Also, we will use the MGH TMAs to analyze a few proteins of house-keeping genes to outline the basal expressions for these reference proteins. Normalization based on these reference proteins, which are assumed to have stable expressions across disease statuses, will be used to refine the classifier algorithm. A fluorescent multiplex immunohistochemistry (mIHC) protocol may be developed to reduce the potential systematic bias (66–68). The well-established classifier algorithm can be used to calculate risk score based on the data that are generated from other lab-friendly platforms. TMAs with improved quality and with more patients being included will certainly help develop a classifier with improved performance in clinic.
The potential limitation of the study is that different scoring methods were used for tumor assay and stroma assay, i.e., tumor samples in the previous studies were scored manually by pathologists (5, 24–27, 34–36), whereas the stromal regions in the current study were digitally scored using the Aperio Imagescope system. Further validation is needed to employ the same scoring method (i.e., digital scoring) and assay to analyze both tumor tissues and stroma tissues in new patient cases. Compared to tumors, stroma appears to be more homogenous. There are multiple cell-types in stroma; however, predominant cells in the tumorous stroma are fibroblasts/myofibroblasts (69). In the current study, we used the pen tool in the Aperio Imagescope system to select tumor-adjacent stroma for the analysis. Advanced methods, such as microdissection, may be used to collect more homogeneous samples for verification.
Conclusions
The results suggest that prognosis of prostate cancer should utilize either tumor-enriched tissue or stroma (close to tumor) tissue with distinct signature profiles rather than using a mixture of both tissue types. This is because the expression of these marker genes in the two tissue types may offset in the mixture samples, yielding ambiguous test results. More importantly, the new classifier based on stroma tissue has potential value in clinical management of prostate cancer patients.
Statement of Translational Relevance
Our study indicated that the protein expression of three markers (FGFR1, LDHB, and MYPT1) were correlated with prostate cancer patients' outcomes (biochemical recurrence) in tumor-adjacent stroma, and such correlations were surprisingly opposite to those in tumor tissues. The results suggested prognosis of prostate cancer should be based on either highly enriched tumor tissue or stroma tissue (adjacent to tumor) with distinct signature profiles, rather than a mixture of both tissue types. This is because the expression of these marker genes in two tissue types may offset in the mixture samples, yielding ambiguous test results. We further evaluated the joint prognostic power of these three protein markers in tumor-adjacent stroma by using a composite classifier developed through a new algorithm, named Weighted Dichotomizing, which was verified by rigorous 10-fold cross validation. The new classifier demonstrated potential value in clinical management of prostate cancer patients.
Ethics Statement
The study was approved by the human study ethics committees at Massachusetts General Hospital (Boston, MA) and the Ministry of Public Health of PR China. All specimens in this study were anonymously handled according to the ethical and legal standards.
Author Contributions
W-DZ supervised the whole project and study and participated in study design and coordination. ZJ, JZ, and YZ analyzed data and wrote and revised the paper. RL, HQ, SW, MW, JC, and RM revised the figures and verified the results. ZL, ZC, JL, YW, FJ, HH, and C-LW collected the samples information and evaluated the score. All authors read and approved the final manuscript.
Conflict of Interest Statement
The authors declare that the research was conducted in the absence of any commercial or financial relationships that could be construed as a potential conflict of interest.
Acknowledgments
This work was supported by grants from International Scientific and Technological Cooperation Projects of Guizhou Province (QKHW-G [2014]7004), National Natural Science Foundation of China (81571427; 81660426), National Key Basic Research Program of China (2015CB553706), Guangzhou Municipal Science and Technology Project (201803040001, 201707010291).
Supplementary Material
The Supplementary Material for this article can be found online at: https://www.frontiersin.org/articles/10.3389/fonc.2019.00539/full#supplementary-material
Table S1. Characteristics of all cases represented on the MGH TMAs.
Figure S1. Comparison of expression scores of three proteins manually evaluated by 3 pathologists.
References
1. Siegel RL, Miller KD, Jemal A. Cancer Statistics, 2017. CA Cancer J Clin. (2017) 67:7–30. doi: 10.3322/caac.21387
2. Attard G, Parker C, Eeles RA, Fritz Schröder P, Tomlins SA, Tannock I, et al. Prostate cancer. Lancet. (2016) 387:70–82. doi: 10.1016/S0140-6736(14)61947-4
3. Jia Z, Wang Y, Sawyers A, Yao H, Rahmatpanah F, Xia XQ, et al. Diagnosis of prostate cancer using differentially expressed genes in stroma. Cancer Res. (2011) 71:2476–87. doi: 10.1158/0008-5472.CAN-10-2585
4. Cuzick J, Thorat MA, Andriole G, Brawley OW, Brown PH, Culig Z, et al. Prevention and early detection of prostate cancer. Lancet Oncol. (2014) 15:e484–92. doi: 10.1007/978-3-642-45195-9
5. Cai C, Chen QB, Han ZD, Zhang YQ, He HC, Chen JH, et al. miR-195 inhibits tumor progression by targeting RPS6KB1 in human prostate cancer. Clin Cancer Res. (2015) 21:4922–34. doi: 10.1158/1078-0432.CCR-15-0217
6. Jia Z, Rahmatpanah FB, Chen X, Lernhardt W, Wang Y, Xia XQ, et al. Expression changes in the stroma of prostate cancer predict subsequent relapse. PLoS ONE. (2012) 7:e41371. doi: 10.1371/journal.pone.0041371
7. Lazzeri M. Prostate cancer claims for a personalized medicine. N Am J Med Sci. (2015) 7:436–7. doi: 10.4103/1947-2714.168661
8. Zheng SL, Sun J, Wiklund F, Smith S, Stattin P, Li G, et al. Cumulative association of five genetic variants with prostate cancer. N Engl J Med. (2008) 358:910–9. doi: 10.1056/NEJMoa075819
9. Ganz PA, Barry JM, Burke W, Col NF, Corso PS, Dodson E, et al. National institutes of health state-of-the-science conference: role of active surveillance in the management of men with localized prostate cancer. Ann Inter Med. (2012) 156:591–5. doi: 10.7326/0003-4819-156-8-201204170-00010
10. van den Bergh RC, Albertsen PC, Bangma CH, Freedland SJ, Graefen M, Vickers A, et al. Timing of curative treatment for prostate cancer: a systematic review. Eur Urol. (2013) 64:204–15. doi: 10.1016/j.eururo.2013.02.024
11. Chen X, Xu S, McClelland M, Rahmatpanah F, Sawyers A, Jia Z, et al. An accurate prostate cancer prognosticator using a seven-gene signature plus gleason score and taking cell type heterogeneity into account. PLoS ONE. (2012) 7:e45178. doi: 10.1371/journal.pone.0045178
12. Stuart RO, Wachsman W, Berry CC, Wang-Rodriguez J, Wasserman L, Klacansky I, et al. In silico dissection of cell type associated patterns of gene expression in prostate cancer. Proc Natl Acad Sci USA. (2004) 101:615–20. doi: 10.1073/pnas.2536479100
13. Wang Y, Xia XQ, Jia Z, Sawyers A, Yao H, Wang-Rodriquez J, et al. In silico estimates of tissue components in surgical samples based on expression profiling data. Cancer Res. (2010) 70:6448–55. doi: 10.1158/0008-5472.CAN-10-0021
14. Ren S, Peng Z, Mao JH, Yu Y, Yin C, Gao X, et al. RNA-seq analysis of prostate cancer in the Chinese population identifies recurrent gene fusions, cancer-associated long noncoding RNAs and aberrant alternative splicings. Cell Res. (2012) 22:806–21. doi: 10.1038/cr.2012.30
15. Ren S, Wang F, Shen J, Sun Y, Xu W, Lu J, et al. Long non-coding RNA metastasis associated in lung adenocarcinoma transcript 1 derived miniRNA as a novel plasma-based biomarker for diagnosing prostate cancer. Eur J Cancer. (2013) 49:2949–59. doi: 10.1016/j.ejca.2013.04.026
16. Guo Y, Xiao P, Lei S, Deng F, Xiao GG, Liu Y., et al. How is mRNA expression predictive for protein expression? A correlation study on human circulating monocytes. Acta Biochimica Et Biophysica Sinica. (2008) 40:426. doi: 10.1111/j.1745-7270.2008.00418.x
17. de Sousa Abreu R, Penalva LO, Marcotte EM, Vogel C. Global signatures of protein and mRNA expression levels. Mol Biosyst. (2009) 5:1512–26. doi: 10.1039/b908315d
18. Hara N, Kasahara T, Kawasaki T, Bilim V, Obara K, Takahashi K, et al. Reverse transcription polymerase chain reaction detection of prostate-specific antigen, prostate-specific membrane antigen, and prostate stem cell antigen in one milliliter of peripheral blood. Clin Cancer Res. (2002) 6:1794–9.
19. Matera L. The choice of the antigen in the dendritic cell-based vaccine therapy for prostate cancer. Cancer Treat Rev. (2010) 36:131–41. doi: 10.1016/j.ctrv.2009.11.002
20. Heijnsdijk E, Wever E, Auvinen A, Hugosson J, Ciatto S, Nelen V, et al. Quality-of-life effects of prostate specific antigen screening. N Engl J Med. (2012) 367:595–605. doi: 10.1056/NEJMc1211071
21. Zamcheck N, Pusztaszeri G. CEA, AFP and other potential tumor markers. CA Cancer J Clin. (1975) 25:204–14. doi: 10.3322/canjclin.25.4.204
22. Webb A, Scott-Mackie P, Cunningham D, Norman A, Andreyev J, O'Brien M, et al. The prognostic value of serum and immunohistochemical tumour markers in advanced gastric cancer. Eur J Cancer. (1996) 32A:63–8. doi: 10.1016/0959-8049(95)00504-8
23. Webb A, Scott-Mackie P, Cunningham D, Norman A, Andreyev J, O'Brien M, et al. The prognostic value of CEA, beta HCG, AFP, CA125, CA19-9 and C-erb B-2, beta HCG immunohistochemistry in advanced colorectal cancer. Ann Oncol. (1995) 6:581–7. doi: 10.1093/oxfordjournals.annonc.a059248
24. Liu J, You P, Chen G, Fu X, Zeng X, Wang C, et al. Hyperactivated FRS2α-mediated signaling in prostate cancer cells promotes tumor angiogenesis and predicts poor clinical outcome of patients. Oncogene. (2016) 35:1750–9. doi: 10.1038/onc.2015.239
25. Lin ZY, Chen G, Zhang YQ, He HC, Liang YX, Ye JH., et al. MicroRNA-30d promotes angiogenesis and tumor growth via MYPT1/c-JUN/VEGFA pathway and predicts aggressive outcome in prostate cancer. Mol Cancer. (2017) 16:48. doi: 10.1186/s12943-017-0615-x
26. Liu J, Chen G, Liu Z, Liu S, Cai Z, You P, et al. Aberrant FGFR tyrosine kinase signaling enhances the warburg effect by reprogramming LDH isoform expression and activity in prostate cancer. Cancer Res. (2018) 78:4459–70. doi: 10.1158/0008-5472.CAN-17-3226
27. Zhong WD, Liang YX, Lin SX, Li L, He HC, Bi XC, et al. Expression of CD147 is associated with prostate cancer progression. Int J Cancer. (2012) 130:300–8. doi: 10.1002/ijc.25982
28. Otte A, Yang Y, von der Ohe J, Melzer C, Hillemanns P, Feuerhake F, et al. SCCOHT tumors acquire chemoresistance and protection by interacting mesenchymal stroma/stem cells within the tumor microenvironment. Int J Oncol. (2016) 49:3735. doi: 10.3892/ijo.2016.3735
29. Erdogan B, Webb DJ. Cancer-associated fibroblasts modulate growth factor signaling and extracellular matrix remodeling to regulate tumor metastasis. Biochem Soc Trans. 2017:229–36. doi: 10.1042/BST20160387
30. Zhu J, Pan C, Jiang J, Deng M, Gao H, Men B, et al. Six stroma-based RNA markers diagnostic for prostate cancer in European-Americans validated at the RNA and protein levels in patients in China. Oncotarget. (2015) 6:16757–65. doi: 10.18632/oncotarget.4430
31. Yang F, Zhang Y, Ressler SJ, Ittmann MM, Ayala GE, Dang TD, et al. FGFR1 is essential for prostate cancer progression and metastasis. Cancer Res. (2013) 73:3716–24. doi: 10.1158/0008-5472.CAN-12-3274
32. Leiblich A, Catto JWF, Cross S, Phillips J, Leung HY, Hamdy FC, et al. Lactate dehydrogenase-B is silenced by promoter hypermethylation in human prostate cancer. Oncogene. (2006) 25:2953–60. doi: 10.1038/sj.onc.1209262
33. Ishibashi H, Suzuki T, Suzuki S, Moriya T, Kaneko C, Takizawa T, et al. Sex steroid hormone receptors in human thymoma. J Clin Endocrinol Metab. (2003) 88:2309–17. doi: 10.1210/jc.2002-021353
34. Lin ZY, Huang YQ, Zhang YQ, Han ZD, He HC, Ling XH, et al. roRNA-224 inhibits progression of human prostate cancer by downregulating TRIB1. Int J Cancer. (2014) 135:541–50. doi: 10.1002/ijc.28707
35. Zhuo YJ, Liu ZZ, Wan S, Cai ZD, Xie JJ, Cai ZD, et al. Enhanced expression of SRPK2 contributes to aggressive progression and metastasis in prostate cancer. Biomed Pharmacother. (2018) 102:531–8. doi: 10.1016/j.biopha.2018.03.079
36. Mo RJ, Han ZD, Liang YK, Ye JH, Wu SL, Lin SX, et al. Expression of PD-L1 in tumor-associated nerves correlates with reduced CD8+ tumor-associated lymphocytes and poor prognosis in prostate cancer. Int J Cancer. (2019) 144:3099–110. doi: 10.1002/ijc.32061
37. Reichard CA, Nyame YA, Sundi D, et al. Does time from diagnosis to treatment of high- or very-high-risk prostate cancer affect outcome? BJU Int. (2019). doi: 10.1111/bju.14671. [Epub ahead of print].
38. Leapman MS, Nguyen HG, Cowan JE, et al. Comparing prognostic utility of a single-marker immunohistochemistry approach with commercial gene expression profiling following radical prostatectomy. Eur Urol. (2018) 74:668–75. doi: 10.1016/j.eururo.2018.08.020
39. Jiang Y, Mei W, Gu Y, Lin X, He L, Zeng H, et al. Construction of a set of novel and robust gene expression signatures predicting prostate cancer recurrence. Mol Oncol. (2018) 12:1559–78. doi: 10.1002/1878-0261.12359
40. McKay RR, Montgomery B, Xie W, Zhang Z, Bubley GJ, Lin DW, et al. Post prostatectomy outcomes of patients with high-risk prostate cancer treated with neoadjuvant androgen blockade. Prostate Cancer Prostatic Dis. (2018) 21:364–72. doi: 10.1038/s41391-017-0009-6
41. Gandaglia G, Fossati N, Karnes RJ, Boorjian SA, Colicchia M, Bossi A, et al. Use of concomitant androgen deprivation therapy in patients treated with early salvage radiotherapy for biochemical recurrence after radical prostatectomy: long-term results from a large, multi-institutional series. Eur Urol. (2018) 73:512–8. doi: 10.1016/j.eururo.2017.11.020
42. Cox DR. Regression models and life-tables (With Discussion). J R Stat Soc Series B. (1972) 34:187–220. doi: 10.1111/j.2517-6161.1972.tb00899.x
43. Keely PJ. Mechanisms by which the extracellular matrix and integrin signaling act to regulate the switch between tumor suppression and tumor promotion. J Mammary Gland Biol Neoplasia. (2011) 16:205–19. doi: 10.1007/s10911-011-9226-0
44. Noël A, Foidart JM. The role of stroma in breast carcinoma growth in vivo. J Mammary Gland Biol Neoplasia. (1998) 3:215–25. doi: 10.1023/A:1018703208453
45. Kwabi-Addo B, Ozen M, Ittmann M. The role of fibroblast growth factors and their receptors in prostate cancer. Endocrine Related Cancer. (2004) 11:709. doi: 10.1677/erc.1.00535
46. Schwertfeger KL. Fibroblast growth factors in development and cancer: insights from the mammary and prostate glands. Curr Drug Targets. (2009) 10:632–44. doi: 10.2174/138945009788680419
47. Feng S, Shao L, Yu W, Gavine P, Ittmann M. Targeting fibroblast growth factor receptor signaling inhibits prostate cancer progression. Clin Cancer Res. (2012) 18:3880–8. doi: 10.1158/1078-0432.CCR-11-3214
48. Ittman M, Mansukhani A. Expression of fibroblast growth factors (FGFs) and FGF receptors in human prostate. J Urol. (1997) 157:351–6. doi: 10.1016/S0022-5347(01)65376-X
49. Giri D, Ropiquet F, Ittmann M. Alterations in expression of basic fibroblast growth factor (FGF) 2 and its receptor FGFR-1 in human prostate cancer. Clin Cancer Res. (1999) 5:1063–71.
50. Sahadevan K, Darby S, Leung HY, Mathers ME, Robson CN, Gnanapragasam VJ. Selective over-expression of fibroblast growth factor receptors 1 and 4 in clinical prostate cancer. J Pathol. (2007) 213:82–90. doi: 10.1002/path.2205
51. Yasumoto H, Matsubara A, Mutaguchi K, Usui T, McKeehan W. Restoration of fibroblast growth factor receptor 2 suppresses growth and tumorigenicity of malignant human prostate carcinoma PC-3 cells. Prostate. (2004) 61:236–42. doi: 10.1002/pros.20093
52. Feng S, Wang F, Matsubara A, Kan M, McKeehan WL. Fibroblast growth factor receptor 2 limits and receptor 1 accelerates tumorigenicity of prostate epithelial cells. Cancer Res. (1997) 57:5369–78.
53. Yan G, Fukabori Y, McBride G, Nikolaropolous S, McKeehan WL. Exon switching and activation of stromal and embryonic libroblast growth factor (FGF)-FGF receptor genes in prostate epithelial cells accompany stromal independence and malignancy. Mol Cell Biol. (1993) 13:4513–22. doi: 10.1128/MCB.13.8.4513
54. Feng J, Ito M, Ichikawa K, Isaka N, Nishikawa M, Hartshorne DJ, et al. Inhibitory phosphorylation site for Rho-associated kinase on smooth muscle myosin phosphatase. J Biol Chem. (1999) 274:37385–90. doi: 10.1074/jbc.274.52.37385
55. Grassie ME, Moffat LD, Walsh MP, MacDonald JA. The myosin phosphatase targeting protein (MYPT) family: a regulated mechanism for achieving substrate specificity of the catalytic subunit of protein phosphatase type 1δ. Arch Biochem Biophys. (2011) 510:147. doi: 10.1016/j.abb.2011.01.018
56. Cho HS, Suzuki T, Dohmae N, Hayami S, Unoki M, Yoshimatsu M, et al. Demethylation of RB regulator MYPT1 by histone demethylase LSD1 promotes cell cycle progression in cancer cells. Cancer Res. (2011) 71:655–60. doi: 10.1158/0008-5472.CAN-10-2446
57. Chirino YI, García-Cuellar CM, García-García C, Soto-Reyes E, Osornio-Vargas ÁR, Herrera LA, et al. Airborne particulate matter in vitro exposure induces cytoskeleton remodeling through activation of the ROCK-MYPT1-MLC pathway in A549 epithelial lung cells. Toxicol Lett. (2017) 272:29–37. doi: 10.1016/j.toxlet.2017.03.002
58. McCleland ML, Adler AS, Deming L, Cosino E, Lee L, Blackwood EM, et al. Lactate dehydrogenase B is required for the growth of KRAS-dependent lung adenocarcinomas. Clin Cancer Res. (2013) 19:773. doi: 10.1158/1078-0432.CCR-12-2638
59. McCleland ML, Adler AS, Shang Y, Hunsaker T, Truong T, Peterson D, et al. An integrated genomic screen identifies LDHB as an essential gene for triple-negative breast cancer. Cancer Res. (2012) 72:5812. doi: 10.1158/0008-5472.CAN-12-1098
60. Zha X, Wang F, Wang Y, He S, Jing Y, Wu X, et al. Lactate dehydrogenase B is critical for hyperactive mTOR-mediated tumorigenesis. Cancer Res. (2011) 71:13–8. doi: 10.1158/0008-5472.CAN-10-1668
61. Berger AH, Pandolfi PP. Haplo-insufficiency: a driving force in cancer. J Pathol. (2011) 223:137–46. doi: 10.1002/path.2800
62. Weinstein JN, Collisson EA, Mills GB, Shaw KR, Ozenberger BA, et al. The cancer genome atlas pan-cancer analysis project. Nat Genet. (2013) 45:1113–20. doi: 10.1038/ng.2764
63. Loebke C, Sueltmann H, Schmidt C, Henjes F, Wiemann S, Poustka A, et al. Infrared-based protein detection arrays for quantitative proteomics. Proteomics. (2007) 7:558–64. doi: 10.1002/pmic.200600757
64. Paweletz CP, Charboneau L, Bichsel VE, Simone NL, Chen T, Gillespie JW, et al. Reverse phase protein microarrays which capture disease progression show activation of pro-survival pathways at the cancer invasion front. Oncogene. (2001) 20:1981–9. doi: 10.1038/sj.onc.1204265
65. Wiese S, Reidegeld KA, Meyer HE, et al. Protein labeling by iTRAQ: a new tool for quantitative mass spectrometry in proteome research. Proteomics. (2007) 7:340–50. doi: 10.1002/pmic.200600422
66. Stack EC, Wang C, Roman KA, Hoyt CC. Multiplexed immunohistochemistry, imaging, and quantitation: a review, with an assessment of Tyramide signal amplification, multispectral imaging and multiplex analysis. Methods. (2014) 70:46–58. doi: 10.1016/j.ymeth.2014.08.016
67. Bethmann D, Feng Z, Fox BA. Immunoprofiling as a predictor of patient's response to cancer therapy—promises and challenges. Curr. Opin. Immunol. (2017) 45:60–72. doi: 10.1016/j.coi.2017.01.005
68. Dixon AR, Bathany C, Tsuei M, White J, Barald KF, Takayama S. Recent developments in multiplexing techniques for immunohistochemistry. Expert Rev Mol Diagn. (2015) 15:1171–86. doi: 10.1586/14737159.2015.1069182
Keywords: prostate cancer, microenvironment, biochemical recurrence, tissue microarray, prognosis, offsetting expression
Citation: Jia Z, Zhu J, Zhuo Y, Li R, Qu H, Wang S, Wang M, Lu J, Chater JM, Ma R, Liu Z, Cai Z, Wu Y, Jiang F, He H, Zhong W-D and Wu C-L (2019) Offsetting Expression Profiles of Prognostic Markers in Prostate Tumor vs. Its Microenvironment. Front. Oncol. 9:539. doi: 10.3389/fonc.2019.00539
Received: 06 January 2019; Accepted: 03 June 2019;
Published: 26 June 2019.
Edited by:
Fabio Grizzi, Humanitas Research Hospital, ItalyReviewed by:
Francesca Sanguedolce, Azienda Ospedaliero-Universitaria Ospedali Riuniti di Foggia, ItalyJames Koziol, The Scripps Research Institute, United States
Copyright © 2019 Jia, Zhu, Zhuo, Li, Qu, Wang, Wang, Lu, Chater, Ma, Liu, Cai, Wu, Jiang, He, Zhong and Wu. This is an open-access article distributed under the terms of the Creative Commons Attribution License (CC BY). The use, distribution or reproduction in other forums is permitted, provided the original author(s) and the copyright owner(s) are credited and that the original publication in this journal is cited, in accordance with accepted academic practice. No use, distribution or reproduction is permitted which does not comply with these terms.
*Correspondence: Wei-De Zhong, emhvbmd3ZDIwMDlAbGl2ZS5jbg==
†These authors have contributed equally to this work and are co-first authors