- 1Department of Preventive Medicine, Institute of Biomedical Informatics, Joint National Laboratory for Antibody Drug Engineering, Cell Signal Transduction Laboratory, Bioinformatics Center, School of Software, School of Basic Medical Sciences, Henan University, Kaifeng, China
- 2Department of Anesthesia, Stanford University, Stanford, CA, United States
- 3Henan Bioengineering Research Center, Zhengzhou, China
- 4Division of Biostatistics, Dan L. Duncan Cancer Center Department of Molecular and Cellular Biology Baylor College of Medicine, Houston, TX, United States
The availability of transcriptome data and clinical annotation offers the opportunity to identify prognosis biomarkers in cancer. However, efficient online prognosis analysis tools are still lacking. Herein, we developed a user-friendly web server, namely Online consensus Survival analysis of leiomyosarcoma (OSlms), to centralize published gene expression data and clinical datasets of leiomyosarcoma (LMS) patients from The Cancer Genome Atlas (TCGA) and Gene Expression Omnibus (GEO). OSlms comprises of a total of 268 samples from three independent datasets, and employs the Kaplan Meier survival plot with hazard ratio (HR) and log rank test to estimate the prognostic potency of genes of interests for LMS patients. Using OSlms, clinicians and basic researchers could determine the prognostic significance of genes of interests and get opportunities to identify novel potential important molecules for LMS. OSlms is free and publicly accessible at http://bioinfo.henu.edu.cn/LMS/LMSList.jsp.
Introduction
Leiomyosarcoma (LMS) is a rare malignant soft tissue tumor. It occurs primarily in the uterus and retroperitoneum and accounts for ~24% of soft tissue sarcomas. LMS has been reported to be difficult for clinical management with poor clinical outcome.
So far, several factors or genes have been found to associate with poor outcomes for LMS patients, including DNA aneuploidy (1), vascular invasion (2), ARL4C (3, 4) and c-Myc expression (5). In particular, high c-Myc expression was reported to be an unfavorable prognosis biomarker in terms of overall survival (OS) in LMS (5). PRUNE2 (prune homolog 2, Drosophila) regulates differentiation, proliferation, and invasiveness of neuroblastoma cell and the up-regulated expression of this protein is a favorable prognosis biomarker in human neuroblastomas (6). Recently, Zhao et al. demonstrated that increased PRUNE2 protein expression is significantly associated with tumor size (p = 0.03) and hemorrhage/cyst (p = 0.01), and is an independent favorable prognostic factor for OS in LMS (p < 0.05) (7). Since identification of prognostic factors will greatly improve cancer diagnosis and treatment, more efforts should be spent on discovering novel prognostic factors for LMS.
With the development in high-throughput sequencing and gene microarray, one way to facilitate the discovery of novel prognostic factors is by studying gene expression profiles of tumors in conjunction with the review of patients' clinical information. However, certain obstacles exist that hinders the use of such data. First, raw microarray datasets are typically buried in the supplementary data files of publications or in the public datasets that are deposited into GEO (8), TCGA or ArrayExpress (9). Second, professionals, who have specialized knowledge and backgrounds to curate the data, are needed to dig into public datasets for prognostic analysis. Third, the clinical follow-up information in the publication or supplied as supporting information is often missing (10). Therefore, user friendly online tools are extremely valuable to provide intuitive curation of these datasets and offer toolkits to quickly visualize and analyze the datasets. Previous studies have reported five pioneer online prognosis analysis tools including KM Plotter (11–14), ITTACA (10), PrognoScan (15), OncoLnc (http://www.oncolnc.org/), and GEPIA (16) for gastric cancer, lung cancer, ovarian cancer, glioma and breast cancer, respectively. However, there is no such tool for LMS. Therefore, an effective analysis tool to access and analyze the prognosis for LMS needs to be established.
In current studies, we have collected public available LMS expression profiling datasets with clinical information and developed a “OSlms” web server, a user-friendly web tool for assessing the relationship between gene expression and LMS patient prognosis using Kaplan Meier plot approach. This web server will help clinicians and basic researchers to determine the prognostic significance of genes of interests and will offer opportunities to identify novel potential important molecules for LMS studies.
Materials and Methods
Data Collection
Gene expression profiling datasets of LMS were collected from PubMed, GEO and TCGA (Table 1) according to the following criteria: (1) contains patient follow-up information, (2) includes ≥ 50 LMS samples to enable valid survival analysis, (3) derives from a platform having public probe annotation.
Development of OSlms
The OSlms server is deployed in a tomcat server. Front end application was developed in HTML and JSP, which can retrieve user inputs and display the results on the web page. The server application was developed in Java and R, which controls the analysis request and returns the results. The SQL Server database was used to store and manage the gene expression profiles and clinical data. The R package, “RODBC” and “JDBC,” were used as middleware to connect R and SQL Server, and to connect Java and SQL Server. The R packages “survminer” and “survival” generate Kaplan Meier (KM) survival curves and calculates the hazard ratio (HR) with 95% confidence intervals and log rank p-value. OSlms can be accessed at http://bioinfo.henu.edu.cn/LMS/LMSList.jsp. System flow diagram is presented in Figure 1.
Results
Establishment of the OSlms Web Server
The OSlms web server employs the Kaplan Meier plot to show the association between the investigated gene and survival rate. Prior to the analysis, the users have the options to narrow the analysis in a subgroup of LMS patients based on age, local recurrence status, gender, residual tumor extent and radiotherapy response. Next, when a query is submitted, the expression level of a selected gene will be demonstrated as median, quartile and trichotomy of the expression level. In addition, clinical outcomes, which include overall survival (OS), metastasis free survival (MFS), progression free survival (PFS), disease free interval (DFI), and progression free interval (PFI), can be evaluated based on the selected samples. Finally, prognostic results of selected genes or probe sets targeting selected genes can be output to assist the evaluation of the validity and reliability of a biomarker.
Functional Validation of Previous Reported Prognostic Biomarkers in the OSlms Web Server
OSlms is a user-friendly tool to assess the prognostic value of genes of interests for LMS patients. As a demonstration, we used the tool to validate the published prognostic biomarker PRUNE2 (7), and found that LMS patients with high levels of PRUNE2 have better survival (HR: 0.435, 95%CI: 0.228-0.831, p = 0.0117), while patients who exhibited low PRUNE2 expression have a lower chance of survival (Figure 2A). This result not only supports that elevated PRUNE2 expression correlates with good prognosis in LMS, but also shows the validity and reliability of this web server.
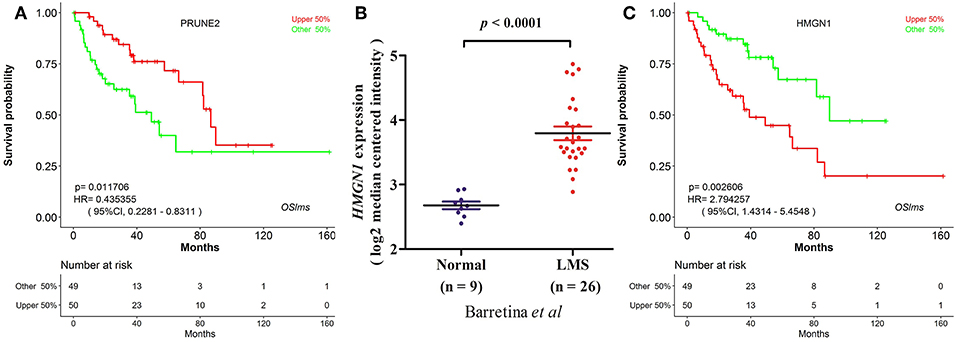
Figure 2. Prognostic test of gene PRUNE2 and HMGN1 in LMS. (A) Kaplan Meier plot for gene PRUNE2. The LMS patients with high and low expression levels are designated as red and green colors. Confidence intervals (CI, 95%) and log rank p-values are as shown. The X-axis represents survival time and the Y-axis represents survival rate. (B) Significant high expression of HMGN1 was found in LMS (20). (C) Kaplan Meier plot for HMGN1 shows that high (red) and low (green) expression of HMGN1 is significantly associated with patient survival.
Identification of Two Putative New LMS Prognostic Biomarkers in OSlms
To test the predictive power of OSlms, a known prognostic biomarker of lung and breast cancer, HMGN1 (Alarmin high-mobility group nucleosome-binding protein 1), was analyzed in the OSlms web server. HMGN1 is a member of the high-mobility group protein family and a non-histone DNA-binding protein that regulates gene transcription and affects chromatin structure by direct binding to nucleosomes (20). In 2015, Wei et al. demonstrated that HMGN1 is a novel clinical biomarker in early-stage patients of non-small cell lung cancer (21). Meanwhile, Lee et al. showed that HMGB1 (high mobility group B1) and HMGN1 are related with tumor-infiltrating lymphocytes in HER2-positive breast cancers (22). Compared with adipose tissue, HMGN1 mRNA expression was significantly increased in LMS (p < 0.0001, Figure 2B) (23). Since the prognostic significance of HMGN1 in lung cancer and other cancers, we examined the prognostic potency of HMGN1 for LMS patients. Using OSlms, we found that LMS patients with high HMGN1 expression have significantly worse OS than the low HMGN1 expression group (HR: 2.794, 95%CI: 1.431-5.455, p = 0.0026), suggesting that HMGN1 could be a novel prognostic indicator of poor overall survival for LMS (Figure 2C).
To further test the server, another known prognostic biomarker in breast cancer, PIGX (Phosphatidylinositol glycan anchor biosynthesis class X), was selected. PIGX plays an important role in the biosynthetic pathway of glycosylphosphatidylinositol (GPI)-anchor motif and is found to be highly upregulated in breast cancer cells (24). Nakakido et al. showed that PIGX promotes cancer cell proliferation by suppressing putative tumor suppressors, including EHD2 and ZIC1 (24). Using OSlms, the high PIGX expressing LMS group has significantly worse MFS, while low PIGX expressing group has better MFS in both the GSE21050 (HR: 2.762, 95%CI: 1.408–5.419, p = 0.0031) and the GSE71118 datasets (HR: 2.328, 95%CI: 1.230–4.403, p = 0.0093) (Figures 3A,B). Not surprisingly, in the combined datasets of GSE21050 and GSE71118, high PIGX expressing group also showed poor MFS (HR: 2.589, 95%CI: 1.628–4.119, p = 5.9e-05), suggesting that PIGX is likely an unfavorable prognostic biomarker for MFS in LMS (Figure 3C).
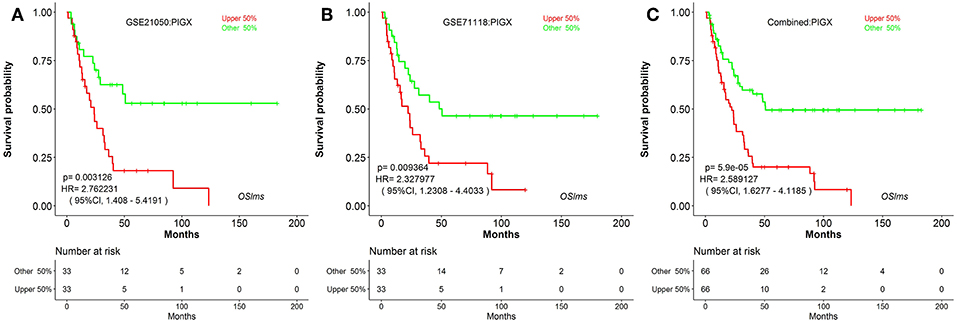
Figure 3. Kaplan Meier plots for high (red) and low (green) PIGX-expressing LMS groups in GSE21050 (A), GSE71118 (B) and combined datasets (C). Confidence intervals (CI, 95%) and log rank p-values are as shown. The X-axis represents survival time and the Y-axis represents survival rate.
Discussion
OSlms is the first online analysis tool to perform prognosis tests and investigations for LMS patient. This online tool provides a platform for clinicians, biologists and other researchers to evaluate the reliability and prognostic significance of genes of interests in LMS. A limitation of this web tool is the patient sample size, for which only 268 samples are currently available in OSlms. When more gene expression datasets of LMS patients become available, we will add new datasets into the OSlms database to improve the power and reliability of this tool.
Data Availability
Publicly available datasets were analyzed in this study. This data can be found online at: “http://bioinfo.henu.edu.cn/LMS/LMSList.jsp.”
Author Contributions
XG: study concept and design; LX, YD, XG, XS, TX, ZY, WZ, YW, WL: acquisition of data; QW, LX, YD, XG, XS, TX, ZY, WZ, YW, WL: analysis and interpretation of data. QW, LX, JG, YH, XG: draft of the manuscript; QW, LX, WZ, XG, WL: critical revision of the manuscript for intellectual content.
Funding
This study is supported by Innovative National Natural Science Foundation of China (No.81602362), Projects for College Students in Henan University, China Postdoctoral Science Foundation (No.2017M62237) and Henan Post-doctoral Foundation (No. 001702052), Program for Science and Technology Development in Henan Province (No.162102310391, No.172102210187), Program for Scientific and Technological Research of Henan Education Department (No.14B520022), Program for Young Key Teacher of Henan Province (2016GGJS-214), Kaifeng Science and Technology Major Project (18ZD008), Supporting grants of Henan University (No.2015YBZR048; No.B2015151), Yellow River Scholar Program (No.H2016012), and Program for Innovative Talents of Science and Technology in Henan Province (No. 18HASTIT048).
Conflict of Interest Statement
The authors declare that the research was conducted in the absence of any commercial or financial relationships that could be construed as a potential conflict of interest.
References
1. Gustafson P, Rydholm A, Willén H, Åkerman M, Baldetorp B, Fernö M. Soft tissue leiomyosarcoma. A population-based epidemiologic and prognostic study of 48 patients, including cellular dna content. Cancer. (2015) 70:114–9. doi: 10.1002/1097-0142(19920701)70:1<114::AID-CNCR2820700119>3.0.CO;2-U
2. Svarvar C, Böhling T, Berlin O, Gustafson P, Follerås G, Bjerkehagen B, et al. Clinical course of nonvisceral soft tissue leiomyosarcoma in 225 patients from the Scandinavian Sarcoma Group. Cancer. (2010) 109:282–91. doi: 10.1002/cncr.22395
3. Guo X, Jo VY, Mills AM, Zhu SX, Lee C-H, Espinosa I, et al. Clinically relevant molecular subtypes in leiomyosarcoma. Clin Cancer Res. (2015) 21:3501–11. doi: 10.1158/1078-0432.CCR-14-3141
4. An Y, Wang S, Li S, Zhang L, Wang D, Wang H, et al. Distinct molecular subtypes of uterine leiomyosarcoma respond differently to chemotherapy treatment. BMC Cancer. (2017) 17:639. doi: 10.1186/s1288-017-3568-y
5. Tsiatis AC, Herceg ME, Keedy VL, Halpern JL, Holt GE, Schwartz HS, et al. Prognostic significance of c-Myc expression in soft tissue leiomyosarcoma. Modern Pathol. (2009) 22:1432. doi: 10.1038/modpathol. 2009.113
6. Machida T, Fujita T, Ooo ML, Ohira M, Isogai E, Mihara M, et al. Increased expression of proapoptotic BMCC1, a novel gene with the BNIP2 and Cdc42GAP homology (BCH) domain, is associated with favorable prognosis in human neuroblastomas. Oncogene. (2006) 25:1931–42. doi: 10.1038/sj.onc.1209225
7. Zhao LR, Wei T, Wang GW, Chen KX, Yang JL. The prognostic role of PRUNE2 in leiomyosarcoma. Chin J Cancer. (2013) 32:648–52. doi: 10.5732/cjc.013.10069
8. Barrett T, Suzek TO, Troup DB, Wilhite SE, Ngau WC, Ledoux P, et al. editors. NCBI GEO: mining millions of expression profiles—database and tools. Nucleic Acids Res. (2005) 33(Database issue):D562–6. doi: 10.1093/nar/gki022
9. Parkinson H, Kapushesky M, Shojatalab M, Abeygunawardena N, Coulson R, Farne A, et al. ArrayExpress–a public database of microarray experiments and gene expression profiles. Nucl Acids Res. (2007) 35(Database issue):747–50. doi: 10.1093/nar/gkl995
10. Elfilali A, Lair S, Verbeke C, La RP, Radvanyi F, Barillot E. ITTACA: a new database for integrated tumor transcriptome array and clinical data analysis. Nucl Acids Res. (2006) 34(Database issue):D613–6. doi: 10.1093/nar/gkj022
11. Szász AM, Lánczky A, Nagy Á, Förster S, Hark K, Green JE, et al. Cross-validation of survival associated biomarkers in gastric cancer using transcriptomic data of 1,065 patients. Oncotarget. (2016) 7:49322–33. doi: 10.18632/oncotarget.10337
12. Gyorffy B, Surowiak P, Budczies J, Lánczky A. Online survival analysis software to assess the prognostic value of biomarkers using transcriptomic data in non-small-cell lung cancer. PLoS ONE. (2013) 8:e82241. doi: 10.1371/journal.pone.0082241
13. Gyorffy B, Lánczky A, Szállási Z. Implementing an online tool for genome-wide validation of survival-associated biomarkers in ovarian-cancer using microarray data from 1287 patients. Endocr Relat Cancer. (2012) 19:197–208. doi: 10.1530/ERC-11-0329
14. Györffy B, Lanczky A, Eklund AC, Denkert C, Budczies J, Li Q, et al. An online survival analysis tool to rapidly assess the effect of 22,277 genes on breast cancer prognosis using microarray data of 1,809 patients. Breast Cancer Res Treat. (2010) 123:725–31. doi: 10.1007/s10549-009-0674-9
15. Mizuno H, Kitada K, Nakai K, Sarai A. PrognoScan: a new database for meta-analysis of the prognostic value of genes. Bmc Med Genomics. (2009) 2:18. doi: 10.1186/1755-8794-2-18
16. Tang Z, Li C, Kang B, Gao G, Li C, Zhang Z. GEPIA: a web server for cancer and normal gene expression profiling and interactive analyses. Nucl Acids Res. (2017) 45:W98–102. doi: 10.1093/nar/gkx247
17. Abeshouse A, Adebamowo C, Adebamowo SN, Akbani R, Akeredolu T, Ally A, et al. Comprehensive and Integrated Genomic Characterization of Adult Soft Tissue Sarcomas. Cell. (2017) 171:950. doi: 10.1016/j.cell.2017.10.014
18. Chibon F, Lagarde P, Salas S, Pérot G, Brouste V, Tirode F, et al. Validated prediction of clinical outcome in sarcomas and multiple types of cancer on the basis of a gene expression signature related to genome complexity. Nat Med. (2010) 16:781–7. doi: 10.1038/nm.2174
19. Lesluyes T, Pérot G, Largeau MR, Brulard C, Lagarde P, Dapremont V, et al. RNA sequencing validation of the Complexity INdex in SARComas prognostic signature. Eur J Cancer. (2016) 57:104–11. doi: 10.1016/j.ejca.2015.12.027
20. Bianchi ME, Agresti A. HMG proteins: dynamic players in gene regulation and differentiation. Curr Opin Genet Dev. (2005) 15:496–506. doi: 10.1016/j.gde.2005.08.007
21. Wei F, Yang F, Jiang X, Yu W, Ren X. High-mobility group nucleosome-binding protein 1 is a novel clinical biomarker in non-small cell lung cancer. Tumour Biol J Int Soc Oncodev Biol Med. (2015) 36:9405. doi: 10.1007/s13277-015-3693-7
22. Lee HJ, Kim JY, Song IH, Park IA, Yu JH, Ahn JH, et al. High mobility group B1 and N1 (HMGB1 and HMGN1) are associated with tumor-infiltrating lymphocytes in HER2-positive breast cancers. Virchows Archiv. (2015) 467:701–9. doi: 10.1007/s00428-015-1861-1
23. Barretina J, Taylor BS, Banerji S, Ramos AH, Lagos-Quintana M, Decarolis PL, et al. Subtype-specific genomic alterations define new targets for soft tissue sarcoma therapy. Nat Genet. (2010) 42:715–21. doi: 10.1038/ng.619
24. Nakakido M, Tamura K, Chung S, Ueda K, Fujii R, Kiyotani K, et al. Phosphatidylinositol glycan anchor biosynthesis, class X containing complex promotes cancer cell proliferation through suppression of EHD2 and ZIC1, putative tumor suppressors. Int J Oncol. (2016) 49:868–76. doi: 10.3892/ijo.2016.3607
Keywords: leiomyosarcoma, prognostic, web server, database, survival analysis
Citation: Wang Q, Xie L, Dang Y, Sun X, Xie T, Guo J, Han Y, Yan Z, Zhu W, Wang Y, Li W and Guo X (2019) OSlms: A Web Server to Evaluate the Prognostic Value of Genes in Leiomyosarcoma. Front. Oncol. 9:190. doi: 10.3389/fonc.2019.00190
Received: 31 January 2019; Accepted: 05 March 2019;
Published: 29 March 2019.
Edited by:
Jianguang Ji, Lund University, SwedenCopyright © 2019 Wang, Xie, Dang, Sun, Xie, Guo, Han, Yan, Zhu, Wang, Li and Guo. This is an open-access article distributed under the terms of the Creative Commons Attribution License (CC BY). The use, distribution or reproduction in other forums is permitted, provided the original author(s) and the copyright owner(s) are credited and that the original publication in this journal is cited, in accordance with accepted academic practice. No use, distribution or reproduction is permitted which does not comply with these terms.
*Correspondence: Xiangqian Guo, xqguo@henu.edu.cn
†These authors have contributed equally to this work