Corrigendum: Analgesic Effects of Compression at Trigger Points Are Associated With Reduction of Frontal Polar Cortical Activity as Well as Functional Connectivity Between the Frontal Polar Area and Insula in Patients With Chronic Low Back Pain: A Randomized Trial
- 1Department of System Emotional Science, Faculty of Medicine, University of Toyama, Toyama, Japan
- 2Department of Sports and Health Sciences, Faculty of Human Sciences, University of East Asia, Shimonoseki, Japan
Background: Compression of myofascial trigger points (MTrPs) in muscles is reported to reduce chronic musculoskeletal pain. Although the prefrontal cortex (PFC) is implicated in development of chronic pain, the mechanisms of how MTrP compression at low back regions affects PFC activity remain under debate. In this study, we investigated effects of MTrP compression on brain hemodynamics and EEG oscillation in subjects with chronic low back pain.
Methods: The study was a prospective, randomized, parallel-group trial and an observer and subject-blinded clinical trial. Thirty-two subjects with chronic low back pain were divided into two groups: subjects with compression at MTrPs (n = 16) or those with non-MTrPs (n = 16). Compression at MTrP or non-MTrP for 30 s was applied five times, and hemodynamic activity (near-infrared spectroscopy; NIRS) and EEGs were simultaneously recorded during the experiment.
Results: The results indicated that compression at MTrPs significantly (1) reduced subjective pain (P < 0.05) and increased the pressure pain threshold (P < 0.05), (2) decreased the NIRS hemodynamic activity in the frontal polar area (pPFC) (P < 0.05), and (3) increased the current source density (CSD) of EEG theta oscillation in the anterior part of the PFC (P < 0.05). CSD of EEG theta oscillation was negatively correlated with NIRS hemodynamic activity in the pPFC (P < 0.05). Furthermore, functional connectivity in theta bands between the medial pPFC and insula cortex was significantly decreased in the MTrP group (P < 0.05). The functional connectivity between those regions was positively correlated with subjective low back pain (P < 0.05).
Discussion: The results suggest that MTrP compression at the lumbar muscle modulates pPFC activity and functional connectivity between the pPFC and insula, which may relieve chronic musculoskeletal pain.
Trial registration: This trial was registered at University Hospital Medical Information Network Clinical Trials Registry (UMIN000033913) on 27 August 2018, at https://upload. umin.ac.jp/cgi-open-bin/ctr/ctr_view.cgi?recptno=R000038660.
Introduction
Chronic musculoskeletal pain is a substantial health concern, which may lead to worsening of daily living activities over time (Nakamura et al., 2011; Sugai et al., 2017). Chronic musculoskeletal low back pain is the most frequently reported chronic musculoskeletal pain (Nakamura et al., 2011). The myofascial trigger point (MTrP) is a painful spot in a taut band of skeletal muscle fibers. When compressed, the MTrP can produce referred pain, and it has been reported to be a main cause of chronic musculoskeletal pain (Simons et al., 1999a, b). An epidemiological study reported that ratios of the presence of MTrPs in the lumbar muscles in patients with chronic low back pain were higher than in subjects without low back pain (Iglesias-González et al., 2013). Compression at MTrPs has been reported to be an effective massage technique for acute and chronic musculoskeletal pain (Hou et al., 2002; Hains et al., 2010; Cagnie et al., 2013; Takamoto et al., 2015; Morikawa et al., 2017). It is noted that prolonged nociceptive inputs from MTrPs induce plastic changes in the brain, resulting in development and maintenance of chronic musculoskeletal pain (Niddam, 2009; Hocking, 2013; Shah et al., 2015). These findings suggest that analgesic effects of MTrP compression may be mediated through its effects on the central nervous system.
Recent neuroimaging studies have reported that chronic musculoskeletal pain induces morphological and functional activity alteration in the brain regions involved in somatosensory, emotional, and cognitive processing of pain including the prefrontal cortex (PFC), insula, anterior cingulate cortex, and somatosensory cortex (Coppieters et al., 2016; Niddam et al., 2017, 2008). Chronic pain was also shown to induce alteration of functional connectivity between these brain areas (Coppieters et al., 2016; Niddam et al., 2017, 2008). Brain oscillation, measured using electroencephalograms (EEGs), has been implicated in pain information processing in these brain areas (Sarnthein et al., 2005; Stern et al., 2006; Ploner et al., 2017). Various therapies for chronic pain have been shown to affect pain-related brain activity. MTrP compression and thermotherapy in the neck in patients with chronic neck pain was shown to suppress neck pain, as well as hemodynamic activity in the frontal polar PFC (Yasui et al., 2010; Morikawa et al., 2017). Furthermore, other treatments, such as acupuncture, physical therapies, and pharmacological therapies, have been shown to induce structural and functional activity changes, especially in the PFC, and also induce changes in functional connectivity among the pain-related brain areas in patients with chronic musculoskeletal pain (Baliki et al., 2008; Napadow et al., 2012; Li et al., 2014; Kregel et al., 2017). However, the neural mechanisms underlying the analgesic effects of MTrP compression in chronic low back pain remain unknown.
In the present study, we hypothesized that compression at lumbar MTrPs may affect hemodynamic and oscillatory activity in the PFC, as well as functional connectivity between the PFC and other pain-related brain areas, in patients with chronic musculoskeletal low back pain. To analyze these parameters, we used simultaneous EEG and near infrared spectroscopy (NIRS) recording.
Materials and Methods
Experimental Design and Subjects
The study was a prospective, randomized, parallel-group trial and an observer- and subject-blinded clinical trial. This trial was performed from 27 August 2018 to 18 January 2019 in university of Toyama. The experiment was carried out in accordance with the guidelines set by the Helsinki Declaration and was approved by the ethics committee of the University of Toyama. Before commencement, the study was registered in UMIN Clinical Trials Registry as UMIN 000033913. We obtained written informed consent from the all subjects. The structure of the present study was shown in Supplementary Table S1 based on The Consolidated Standards of Reporting Trials (CONSORT) statement1.
Thirty-two subjects with chronic low back pain (mean age 24.0 ± 0.9 years old; 14 males and 18 females) were enrolled in University of Toyama in the present study. The inclusion criteria for the participants were as follows: (1) persistent pain in the lumbar quadrate muscle for more than 3 months, (2) presence of active MTrP(s) in the lumbar quadrate muscle, (3) baseline lumbago scores over 40 mm in the visual analog scale (VAS), and (4) no neurological signs or peripheral nerve complaints. One therapist with over 6 years of clinical experience, who had the license for Japanese Judo therapy, identified active MTrPs according to the criteria for active MTrP diagnosis (Gerwin et al., 1997): (1) presence of hypersensitive spot(s) in taut band(s) in the muscle, and (2) reproduction of the same low back pain with the same referred pain as subjects usually felt when active MTrP(s) were stimulated by compression. The therapist detected the active MTrP in the lumbar quadrate muscle from all subjects (see below). The subjects were randomly assigned to two groups: the MTrP (compression at active MTrP) or non-MTrP (compression at non-MTrP) groups (Figure 1).
Active MTrPs in the lumbar quadrate muscle were identified according to the previous studies (Simons et al., 1999b; Joseph, 2009). First, the erector muscles of spine, iliac crest, and 12th rib were identified by palpation while the subjects lay on the bed in a prone position. Second, the lumbar quadrate muscle was identified by palpating the external edge of the erector muscles of spine, where the lumbar quadrate muscle is located. Finally, the therapist palpated the lumbar quadrate muscle in the area between the 12th rib and its iliac attachment to identify active MTrPs. The therapist usually identified active MTrPs in the lumbar quadrate muscle below the 12th rib in the present study.
Sample Size
The sample size was computed on the basis of the previous clinical experiment that investigated effects of compression at active MTrPs and non-MTrPs on chronic musculoskeletal pain (Morikawa et al., 2017). In the previous study, the standard deviation of VAS score of pain at baseline was 9.8. The difference in VAS pain changes before and after treatment between the MTrP and non-MTrP groups was 14.2. Based on a significance level of 5% (two-tailed) and a statistical power of 95%, 14 subjects would be required in each group. With consideration of drop out and withdrawal rate, the adequate sample size was determined as 16 subjects in each group for the present study.
Experimental Procedures
The subjects rated low back pain before experiment using the VAS score [from 0 (no pain) to 100 (maximum tolerable pain)] (primary outcome). Then, the subjects sat in a massage chair, were instructed to close their eyes, and the heads of the subjects were fitted with a combined NIRS/EEG head cap for simultaneous measurement of NIRS and EEG (Takeuchi et al., 2009) (secondary outcomes). The NIRS method allows hemodynamic measurements under a situation similar to clinical practice where subjects sit on a comfortable chair, and physicians can easily and exactly manipulate the low back muscle by hand. Then, 32 EEG electrodes and 61 NIRS probes were attached to the head cap. The pain pressure threshold (PPT) (secondary outcome) and maximal pressure pain threshold (MPT) in the stimulation site were measured using a digital algometer prior to the compression experiment (see the section “Muscle Compression (Intervention)” in detail).
The compression experiment was performed after a 5 min rest period while EEGs and NIRS were recorded as baseline activity. Then, active MTrP or non-MTrP compression was applied for 30 s, followed by a rest period of 60 s. This cycle was repeated five times (five cycles). The therapist kept predetermined constant pressure at an intensity midway between the PPT and MPT according to monitoring of the pressure intensity on the thumb. After the end of the experiment, the EEG and NIRS were further measured for 5 min without muscle compression. Then, PPT was similarly assessed three times, and the head cap was removed. Finally, comfort/discomfort score and pain intensity score during compression, as well as present low back pain after compression experiment, were assessed using the VAS. For the comfort/discomfort score, the participants were instructed to rate pleasantness of the compression after each cycle based on a scale of −10 to 10, where −10 and 10 represented the most unpleasant and pleasant experiences, respectively. For the pain intensity score, the participants were asked to rate the intensity of compression after each cycle based on a scale of 0 to 100 [from 0 (no pain) to 100 (maximum tolerable pain)].
Muscle Compression (Intervention)
Before the experiment, the therapist identified each point as MTrP or non-MTrP in the lumbar quadrate muscle stimulation site. Non-MTrP was located at the point 3 cm away from the MTrP, where compression did not elicit local or referred pain, and no taut band was detected.
To determine compression intensity at each stimulation site, the therapist measured PPT and MPT by compression at the MTrP or non-MTrP using a digital pressure sensor (6 mm diameter) (PDA-2MPA: Tokyo Sokki Kenkyujo, Japan) that was attached on the therapist’s thumb and connected to a data collection hardware device (PCD-301B: Kyowa Dengyo, Japan). Pressure intensity was observed online via a monitor (DCS-100A: Kyowa Dengyo, Japan) that neither the therapist nor the subject could look at. An experimenter (therapist, Exp 1), who could not observe the monitor recording, determined the PPT and MTP according to the reports of the subject. Another experimenter (Exp 2) recorded compression intensity from the monitor. While Exp 1 gradually increased compression intensity at an MTrP or non-MTrP, the subjects were instructed to say “yes” when they initially felt pain [score 4 (pain threshold: PPT); score 0 (no pain)], and also when they felt severe pain [score 10 (severe pain)] (i.e., MTP). Exp 2 recorded compression intensity at PPT and MTP. The PPT and MTP were measured three times in each stimulation site, and midway, intensities between the mean PPT and mean MTP were determined for each subject.
Randomization and Blinding
The subjects were randomly assigned to two groups (MTrP and non-MTrP). An independent operator, who was different from the therapist and experimenter, generated random allocation sequence (block randomization; block size, 2; allocation ratio, 1:1) using R software (ver. 3.43) before starting the trial. The operator sealed the allocation code in sequentially numbered opaque envelopes. The envelopes were opened in numerical order, and the subjects were automatically assigned to one of the two groups based on the allocation code. After the trial was completed, the operator confirmed that the subjects were exactly assigned according to the random allocation sequence.
The subjects were not informed whether MTrP or non-MTrP was compressed. An experimenter, who was unaware of the treatment groups, assessed VAS, PPT, MTP, comfort/discomfort score, and pain intensity score during compression. The experimenter was away from the experimental room while the therapist detected the stimulation sites (MTrPs and non-MTrPs).
NIRS Recording
Cerebral hemodynamics were measured using two NIRS systems (OMM3000, Shimadzu Inc., Kyoto) in the present study. We used a head-cap for simultaneous recording of EEG and NIRS (FLASH-PLUS, Shimadzu Co. Ltd., Kyoto) (Takeuchi et al., 2009). The head cap was placed so that the bottom horizontal line of the NIRS optodes was positioned at the FP1-FP2 line of the 10–20 EEG system (Jurcak et al., 2007; Takeuchi et al., 2009). The head cap contained 26 source probes and 32 detector probes (Figure 2A). The hemodynamic response was detected by the near-infrared wave in the different three wavelengths (708, 805, and 830 nm) with 5 ms pulse. Hemoglobin concentration [oxy-Hb, deoxy-Hb, and total-Hb (Oxy-Hb + Deoxy-Hb)] was measured based on the modified Lambert-Beer law (Seiyama et al., 1988; Wray et al., 1988).
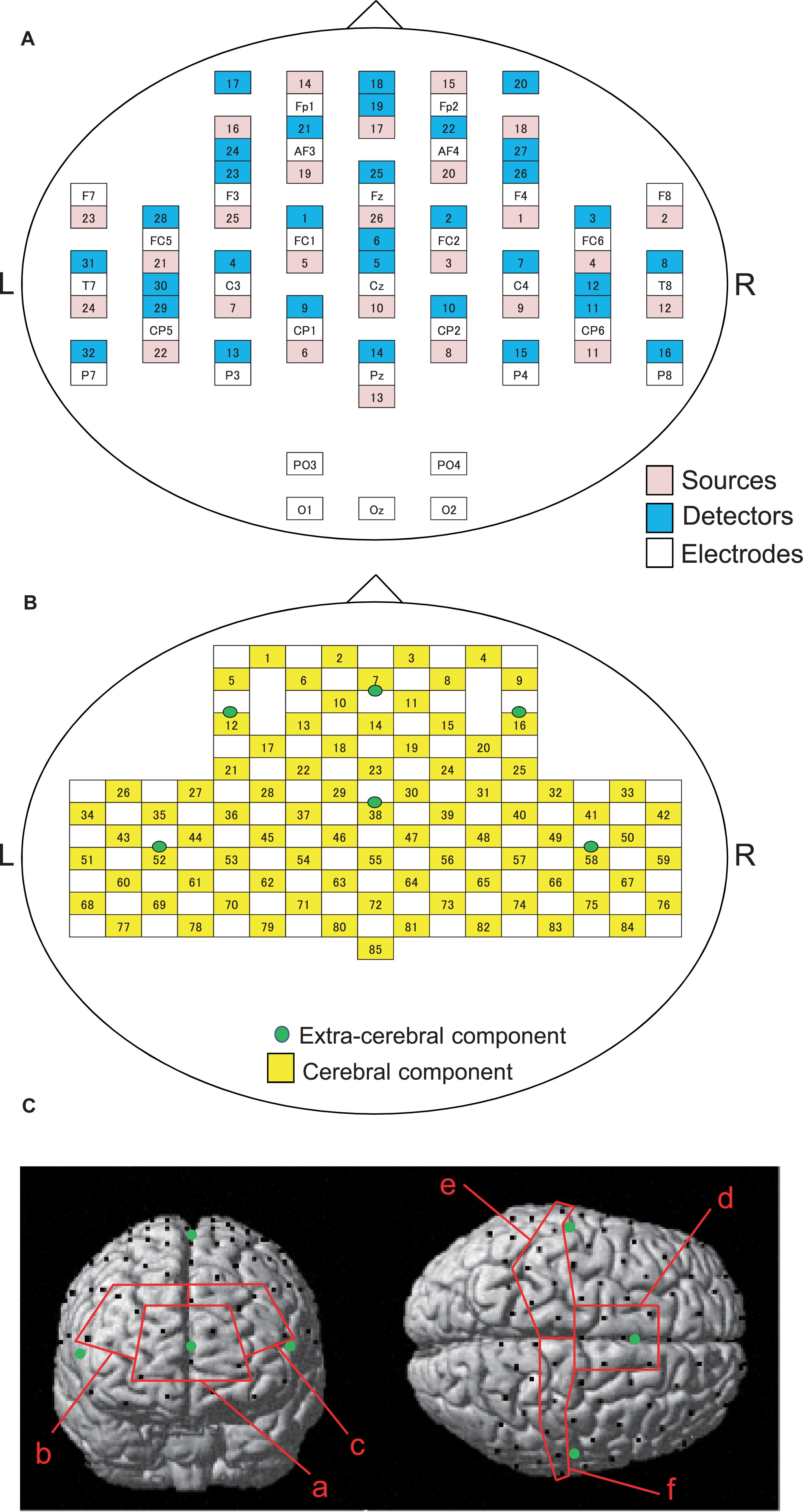
Figure 2. Arrangements of NIRS probes and EEG electrodes. (A) Arrangement of NIRS probes (source and detectors) and EEG electrodes. (B) Locations of NIRS channels. Green cycles indicate extra-cerebral component channels. (C) Spatial registration of NIRS channel locations. Black circles, NIRS channels; green circles, extra-cerebral component channels. (a) frontal polar area in the prefrontal cortex (pPFC); (b) right dorsolateral prefrontal cortex (R-dlPFC); (c) left dorsolateral prefrontal cortex (L-dlPFC); (d) supplementary motor area (SMA); (e) left primary sensorimotor cortex (L-SMC); (f) right primary sensorimotor cortex (R-SMC).
The information of the NIRS signals consists of both the cerebral and extra-cerebral hemodynamic components. The proportion of the two components depends on the distance between the source and detector probes (Fukui et al., 2003; Niederer et al., 2008). Hemodynamic signals from optodes with a distance over 3 cm reflect both cerebral and extra-cerebral (e.g., scalp, skull, etc.) components of the signals. When the distance of optodes is shorter than 1.5 cm, hemodynamic signals include mainly extra-cerebral components. Therefore, the optode arrangement with multi-distance was used to eliminate the interference from extra-cerebral hemodynamic components, including systemic changes of blood flow in the scalp layer and head motion artifacts, to the hemodynamic NIRS signals (Yamada et al., 2009, 2015; Saager et al., 2011; Ishikuro et al., 2014; Sato et al., 2016; Nakamichi et al., 2018).
In the present study, 26 detectors were placed 3 cm away from each source optode (Figure 2B). The midpoints between the source and detector optodes were defined as the “whole component channels,” and the signals from those optodes were defined as “whole signals.” This arrangement of the optodes resulted in 85 channels (and corresponding signals). In addition, another 6 detector optodes were placed 1.5 cm away from source optodes. The midpoints between the source and those detector probes were defined as “extra-cerebral component channels,” and the signals from those optodes were “extra-cerebral signals.” A total of six extra-cerebral signals were recorded from the corresponding extra-cerebral component channels (Figure 2B).
After the experiment in each subject, the three-dimensional coordinate positions of the optodes and channels was measured by a digitizer (Fastrak, Polhemus Inc., United States) with reference to the bilateral external auditory meatus and nasion.
NIRS Data Analysis
The cerebral components of the NIRS signals (oxy-Hb, deoxy-Hb, total-Hb) were estimated using simple-subtraction methods (Schytz et al., 2009; Nakamichi et al., 2018). The cerebral components of the signals were estimated by subtraction of the extra-cerebral signals, located closest to corresponding whole signals, from the whole signals. Next, the resultant NIRS signals were band-pass filtered (0.01–0.1 Hz) to eliminate the baseline drift and physiological noises due to respiratory and cardiac activities (Tong et al., 2011; Yasumura et al., 2014).
Then, the NIRS signals during the five experimental cycles were summed and averaged to analyze the temporal changes of hemodynamics during the compression. The averaged hemodynamic responses were corrected for the mean baseline activity from −10 to 0 s before the compression. The change in Oxy-Hb concentration, which is the index most reflecting the neuronal activity (Hoshi et al., 2001), was converted to the effect size to compare the changes in hemodynamics between the two groups. The effect size of each channel in each cycle was calculated as [(averaged oxy-Hb concentration during compression for 30 s) - (averaged oxy-Hb concentration in the rest period from −10 to 0 s before the compression onset)]/the standard deviation of mean oxy-Hb concentration in the rest period. Then, the effect size from all five cycles was summed and averaged.
To identify the anatomical location of each channel, the three-dimensional coordinates of the channels in each subject were normalized to the Montreal Neurological Institute (MNI) coordinates by virtual registration (Tsuzuki et al., 2007). The anatomical locations [Brodmann’s areas (BA)] of the NIRS channels was estimated using the Talairach Daemon program2. We analyzed a total of six regions of interest (ROIs): frontal polar area in the PFC (pPFC; BA10), left and right dorsolateral prefrontal cortex (dlPFC; BA9,46), medial part of BA 6 that corresponds to the supplementary motor area (SMA), left sensorimotor cortex (L-SMC; BA1, 2, 3, and 4), and right sensorimotor cortex (R-SMC; BA1, 2, 3, and 4) (Figure 2C). Then, the effect sizes of the NIRS signals in the all channels in each ROI were summed and averaged.
EEG Recording
EEG signals were recorded using a BioSemi ActiveTwo System (BioSemi CO. Ltd., Netherlands). After the head cap was placed on the head, 32 high-impedance electrodes, 2 reference electrodes, and grand were slotted into the holes in the head cap. Extra-electrodes for re-referencing were attached on the nose and both earlobes. To detect blinking and eye movements, electrooculograms (EOGs) were also recorded. It is noted that the EEG electrodes were placed at the midpoint between the NIRS source and detector optodes (Figure 2B), indicating that the locations of the EEG electrodes match those of the NIRS channels (Takeuchi et al., 2009). Electrode offset, which corresponds to input impedance, of all the electrodes were set to less than ±5 mV (Metting van Rijin et al., 1990). EEG signals were digitized using a 24-bit A/D converter at 2000 Hz sampling rate.
EEG Data Analysis
The EEG data were preprocessed by using MATLAB (Mathworks Inc.) and the EEGLAB toolbox (Delorme and Makeig, 2004). The EEG data were down sampled from 2000 to 500 Hz and band-pass filtered in the range of 1–50 Hz. Then, EEG signals were removed automatically or by visual inspection if they were above ±50 μV or contained motion artifacts. Furthermore, EEG artifacts caused by eye movements and blinking were removed by using independent component analysis (ICA; Makeig et al., 1997, 1999; Jung et al., 2000; Delorme and Makeig, 2004). The artifact-free data of the 28 s period during each compression and 10 s period during rest after each compression were extracted from the EEG data.
Current source density (CSD) of neuronal oscillations was estimated from the EEG data using sLORETA (Pascual-Marqui, 2002). The solution space was confined to cortical gray matter, which consists of 6239.5 mm3 voxels. The artifact-free EEG data during compression (28 s for each cycle) and rest (10 s for each cycle) were segmented into 2 s epochs. A total of 70 and 25 of the 2 s epochs during compression and rest, respectively, in each subject were subjected to sLORETA analysis. Cross-spectra of the EEG epochs for each subject in each condition were computed using the sLORETA software in eight frequency bands: delta, 1.5–6.0 Hz; theta, 6.5–8.0 Hz; alpha1, 8.5–10.0 Hz; alpha2, 10.5–12.0 Hz; beta1, 12.5–18.0 Hz; beta2, 18.5–21.0 Hz; beta3, 21.5–30.0 Hz; and gamma, 31.0–50.0 Hz. The sLORETA computed the cortical distribution of CSD of neuronal oscillations in 6239 voxels from the averaged cross-spectra in each band for each subject in each condition. The CSD data were normalized with subject-wise normalization; CSD at each voxel was normalized with power density averaged across all frequencies and all 6239 voxels (Babiloni et al., 2007). Then, subtracted CSD (compression – rest) (defined as “CSD responses” during compression) were compared between the two groups by voxel-by-voxel in each band using the t-statistical non-parametric mapping (SnPM) implemented in sLORETA.
The significant voxels were counted in each brain region defined in sLORETA (see Table 3 in section “Results”). Since Brodmann area (BA) 10 includes a large cortical region, it was divided into two subregions in each side based on the map in sLORETA: mpPFC (a medial part of BA 10; i.e., BA 10 area in the medial frontal gyrus in sLORETA) and the remaining lateral part of BA 10 (lpPFC; i.e., BA10 area in the superior, middle and inferior frontal gyri, and subgyrus). BA 11 (OPFC in sLORETA) was also divided into two subregions: superior OPFC (an anterior part of BA 11; i.e., BA11 area in the superior frontal gyrus in sLORETA) and the remaining other region (rOPFC) in BA11 (BA 11 area in the inferior frontal; medial frontal, middle frontal, orbital, rectal, and subcallosal gyri in sLORETA). The dlPFC included BA 9 and 46 in sLORETA. The primary somatosensory cortex included BA 1, 2, and 3 in sLORETA. The insula cortex was divided into two regions: the insula in BA 13 (INS13) and the remaining other regions (oINS). The remaining other regions (oINS) included right BA22, right BA40, right BA41, left BA41, left BA45, and right BA47.
Relationships Between EEGs and Hemodynamics
Relationships between CSD responses and effect sizes of hemodynamic responses (Oxy-Hb concentration) during compression were analyzed. First, brain regions that showed more than 30 voxels with significant differences in CSD responses between the two groups were identified in each frequency band in sLORETA (see above). In the present study, significant differences in CSD responses between the two groups were found only in theta bands (see section “Results”). Since spatial resolution of the NIRS is low, the right and left sides of each medial prefrontal region (mpPFC and lpPFC; superior OPFC and rOPFC) were each combined into one region. Then, mean CSD responses in theta bands in each brain region were computed by averaging CSD responses in the all voxels in each selected brain region. Then, mean theta CSD responses were subjected to natural logarithmic transformation to obtain a normal distribution. Finally, relationships between mean CSD responses and mean effect sizes of hemodynamic responses were analyzed in each selected brain region using a simple linear regression.
Functional Connectivity
The 22 pain-related brain regions were selected as ROIs based on the map in sLORETA (Table 1). The ROIs included the right and left primary somatosensory cortex (S1) (BA 1, 2, and 3), right and left primary motor cortex (M1) (BA 4), right and left SMA, right and left dlPFC (BA 9, 46), right and left mpPFC, right and left lpPFC, right and left superior OPFC (BA11), right and left rOPFC (BA 11), right and left INS13 (BA 13 in the insula cortex), right and left v-ACC (BA 24), and right and left d-ACC (BA 32). According to a previous study (Cui et al., 2014), a single voxel in the center of each ROI was selected as a representative voxel in each ROI to compute functional connectivity since the spatial resolution of sLORETA was low.
To estimate functional connectivity between all possible pairs of ROIs based on sLORETA, lagged phase synchronization was used (Pascual-Marqui, 2007a, b; Canuet et al., 2011). This measure (lagged phase synchronization) evaluates similarity (nonlinear functional connectivity) between signals in the frequency domain according to normalized Fourier transforms and is corrected with the instantaneous zero-lag contribution. The instantaneous zero-lag contribution often includes non-physiological components such as volume conduction or physical artifact. This measure (lagged phase synchronization) is thought to contain only connectivity information without non-physiological components (Pascual-Marqui, 2007a, b; Canuet et al., 2011).
The independent sample t test and non-parametric randomizations (number of randomizations = 5000) were used to analyze significant difference in functional connectivity between all the possible pairs of ROIs in eight frequency bands between the MTrP and non-MTrP groups (Nichols and Holmes, 2002).
Statistical Analysis
All data in the present study were shown as the average ± standard error. The Kolmogorov–Smirnov test was used to assess normality distribution for each continuous variable. Comparison of the baseline characteristics (age, VAS, PPT, and intensity of compression) and sensations evoked by compression (comfort/discomfort scores and pain intensity score during compression) between the two groups were performed by Student’s t test. The gender and stimulation site between the two groups were compared by Fisher’s exact test. Comparisons of changes in VAS and PPT before and after compression between the MTrP and non-MTrP groups were performed using Mann–Whitney U test. The effect sizes of hemodynamic responses during compression were compared by a repeated-measures two-way ANOVA (group × ROI). Relationships between effect sizes of hemodynamic responses (Oxy-Hb) and mean CSD in each band in each ROI were analyzed using the simple regression test. All the statistical analyses, except those implemented in sLORETA, were conducted using SPSS 19.0 (IBM Inc., New York, United States). Statistically significant levels were set to values of P < 0.05.
Results
Baseline Characteristics
Table 2 shows baseline clinical characteristics, stimulus condition, and sensations evoked by compression in each group. There were no significant differences between the two groups in age, VAS, PPT, intensity of stimulation, comfort/discomfort scores, or pain intensity scores during compression (Student’s t test, P > 0.05) and gender (Fisher’s exact test, P > 0.05). Furthermore, there was no significant difference in laterality of stimulation sites between the two groups (Fisher’s exact test, P > 0.05).
Changes in VAS Pain and PPT by Compression
Compression significantly affected PTT and VAS pain. Figure 3A shows a PPT increment after compression in the two groups. Changes of PPT before and after compression was significantly increased after compression in the MTrP group [18.35 ± 4.69, mean ± SEM; 95% confidence interval (CI), 8.03 to 28.68] than the non-MTrP group (0.42 ± 6.78; CI, -14.50 to 15.34) [Mann–Whitney U test, P < 0.05; effect size (r) = 0.36]. Figure 3B shows VAS pain reduction after compression in the two groups. Compression significantly decreased changes of VAS pain before and after compression in the MTrP group (−23.69 ± 3.21; CI, −30.75 to −16.62) than the non-MTrP group (−8.88 ± 3.47; CI, −14.50 to 15.34) [Mann–Whitney U test, P < 0.05; effect size (r) = 0.55]. No adverse incidents were observed in the two groups.
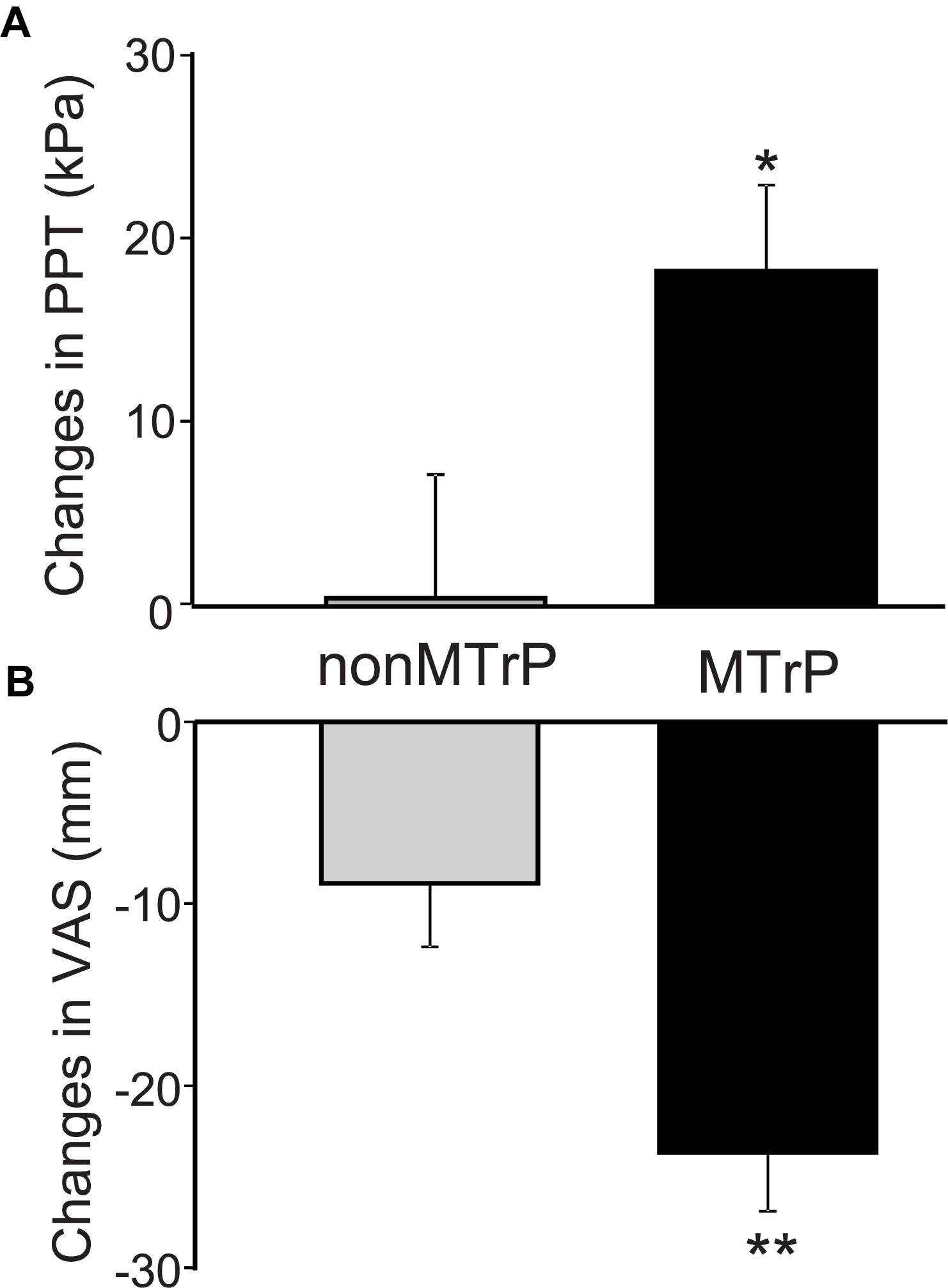
Figure 3. Changes of PPT (A) and VAS scores of low back pain (B) before and after compression in the MTrP and non-MTrP groups. Note that compression significantly increased PPT (A) and decreased VAS pain (B) in the MTrP group compared with the non-MTrP group (P < 0.05).
Hemodynamic Responses
Compression significantly affected cerebral hemodynamic activity. Figure 4 shows typical examples of hemodynamic responses during compression for 30 s at MTrPs and non-MTrPs, shown as effect sizes of Oxy-Hb concentration. The topographical maps of effect sizes indicated that hemodynamic activity increased in the pPFC during compression at a non-MTrP (left panel in A), while hemodynamic activity decreased in the pPFC during compression at an MTrP (left panel in B). Temporal patterns of Oxy-Hb signals showed similar changes; oxy-Hb signals gradually increased in the pPFC during compression at non-MTrPs (right panel in A), while Oxy-Hb concentration gradually decreased in the pPFC during MTrP compression (right panel in B).
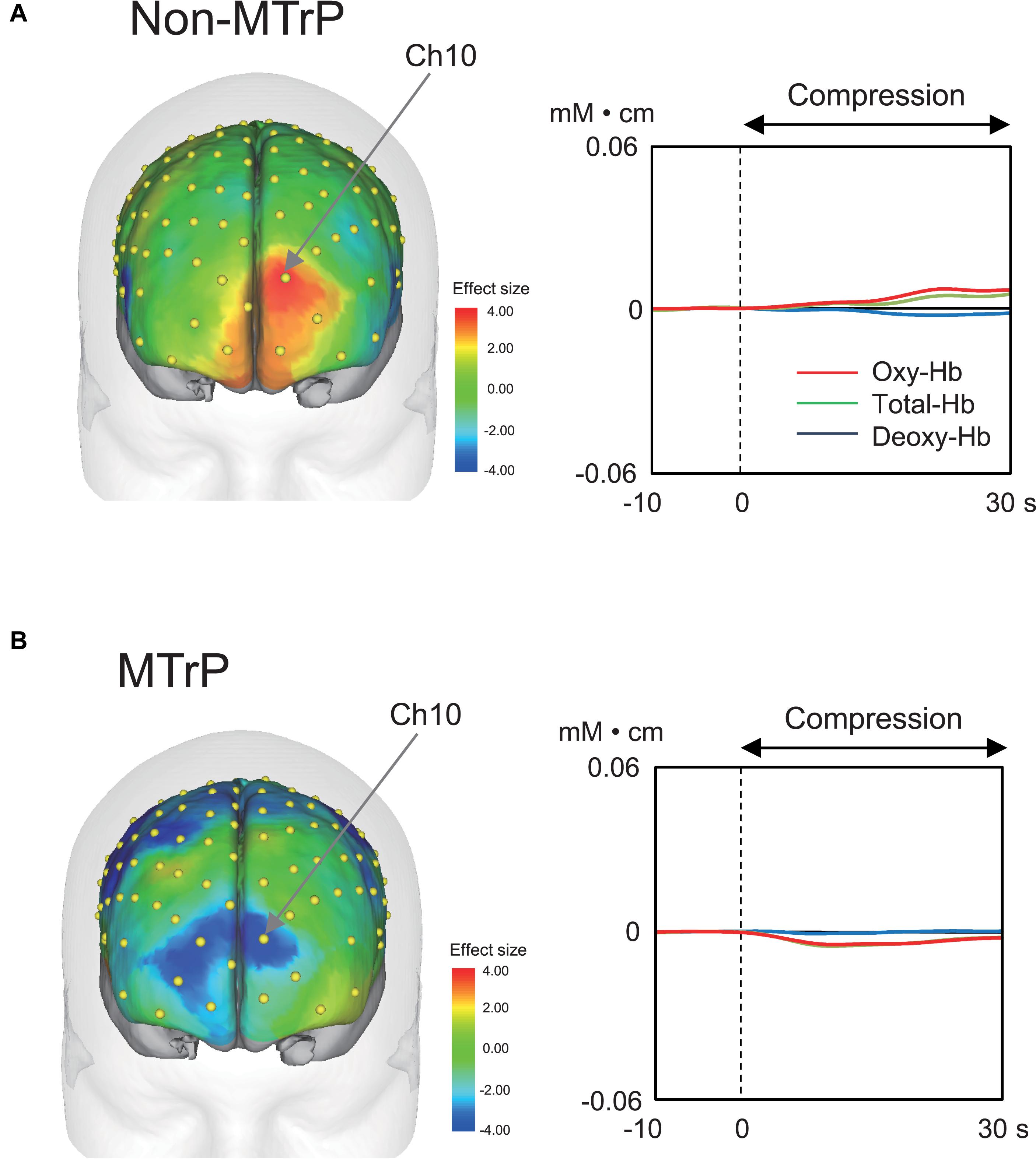
Figure 4. Examples of hemodynamic responses during compression in the non-MTrP (A) and MTrP (B) groups. Topographical maps in the left indicate effect sizes of hemodynamic responses (change in Oxy-Hb concentration) during compression. Yellow dots indicate NIRS channels. The right graphs indicate temporal changes in hemodynamic activity during compression.
Figure 5 shows the mean effect sizes of hemodynamic responses in the six ROIs in the MTrP and non-MTrP groups. The analysis by repeated-measures two-way ANOVA with “group” and “ROI” as factors showed no significant main effects of group (MTrP vs. non-MTrP) [F(1,30) = 1.61, P > 0.05] or ROI [F(2.96,88.77) = 2.02, P > 0.05], but showed a significant interaction between group and ROI [F(2.96,88.77) = 3.50, P < 0.05]. Post hoc comparisons revealed that Oxy-Hb concentration in the pPFC during compression was significantly decreased in the MTrP group compared to the non-MTrP group (Bonferroni test, P < 0.01).
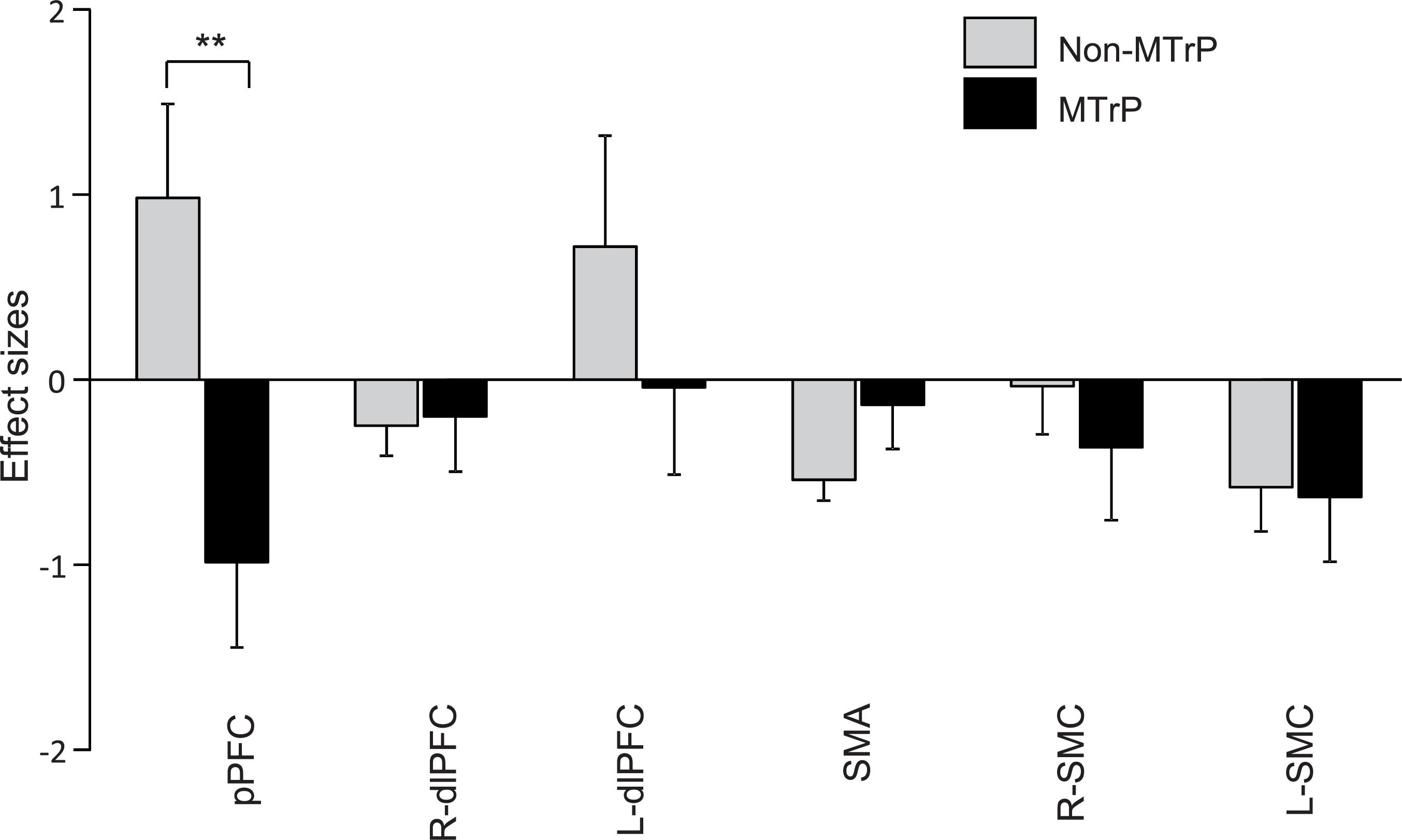
Figure 5. Comparison of hemodynamic responses in each ROI during compression between the MTrP and non-MTrP groups. Effect sizes of hemodynamic responses during compression was significantly decreased in the MTrP group compared with non-MTrP. pPFC, frontal polar area in the PFC; dlPFC, dorsolateral PFC; SMA, supplementary motor area; L-SMC, left primary sensorimotor cortex; R-SMC, right primary sensorimotor cortex.
Brain CSD Activity
Among the eight frequency bands, we found significant differences only in CSD in theta bands between the MTrP and non-MTrP groups. Figure 6 shows significant differences in CSD between the MTrP and non-MTrP groups during compression. CSD in the theta bands increased mainly in the anterior part of the PFC during compression in the MTrP group than the non-MTrP group. Significant voxels were observed in the right primary somatosensory cortex (S1; BA 1–3), right primary motor cortex (M1;BA 4), bilateral supplementary motor cortex (SMA;BA 6), left frontal eye field (FEF;BA 8), bilateral dlPFC (BA 9, 46), bilateral mpPFC (BA 10), bilateral lpPFC (BA10), bilateral superior OPFC (BA 11), bilateral OPFC (BA 11), left middle temporal gyrus (MT; BA 21), bilateral subgenual cortex (BA 25), bilateral d-ACC (BA 32), left temporal pole (TP;BA 38), left opercular part of inferior frontal gyrus (OpIFG; BA 44), left triangular part of inferior frontal gyrus (TrIFG; BA 45), and left inferior frontal cortex (IFC) (BA 47) (Table 3).
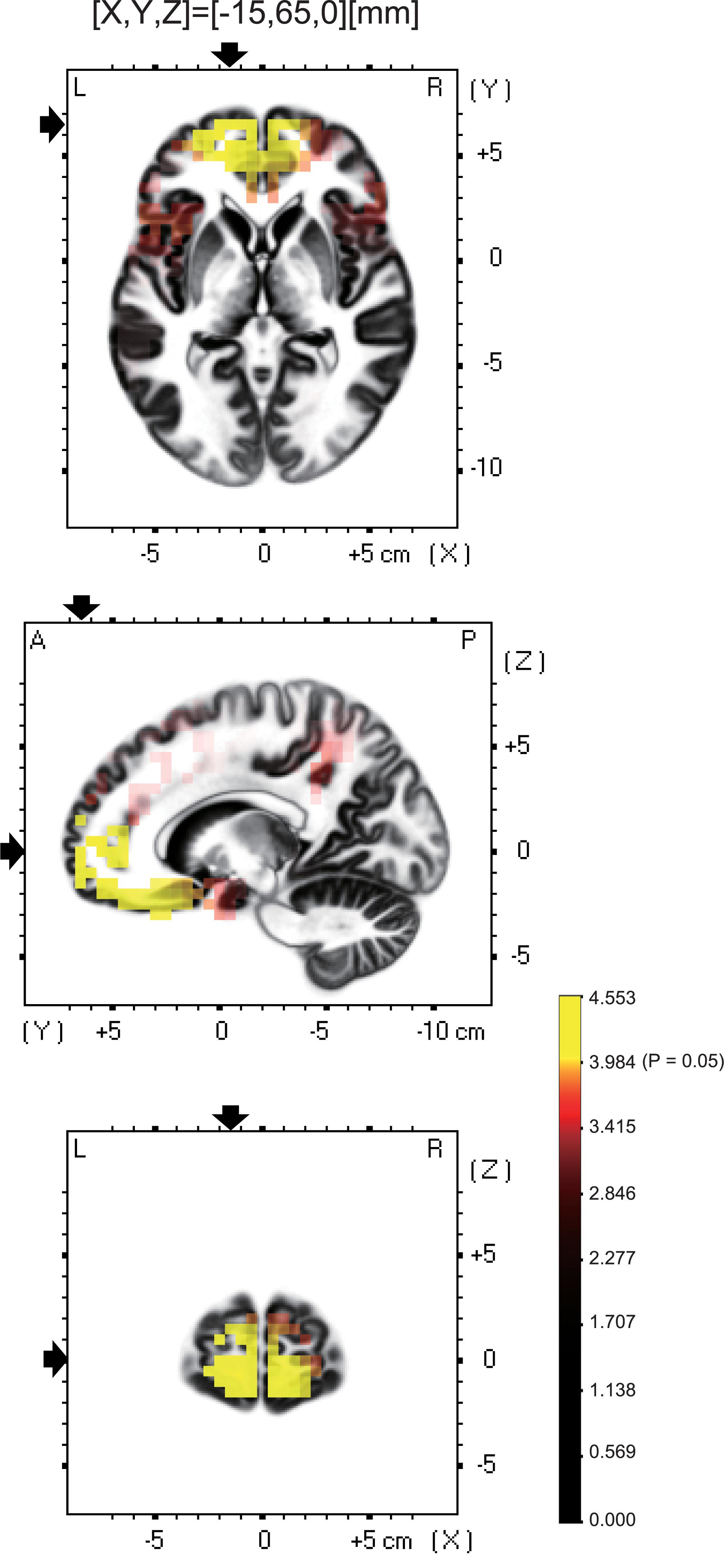
Figure 6. Brain maps showing significant changes in CSD of theta oscillations during compression between the MTrP and non-MTrP groups. Yellow areas show brain voxels with significantly higher theta CSD in the MTrP group compared with the non-MTrP group (P < 0.05). The black arrows indicate the 3D coordinates of the voxel with the highest CSD.
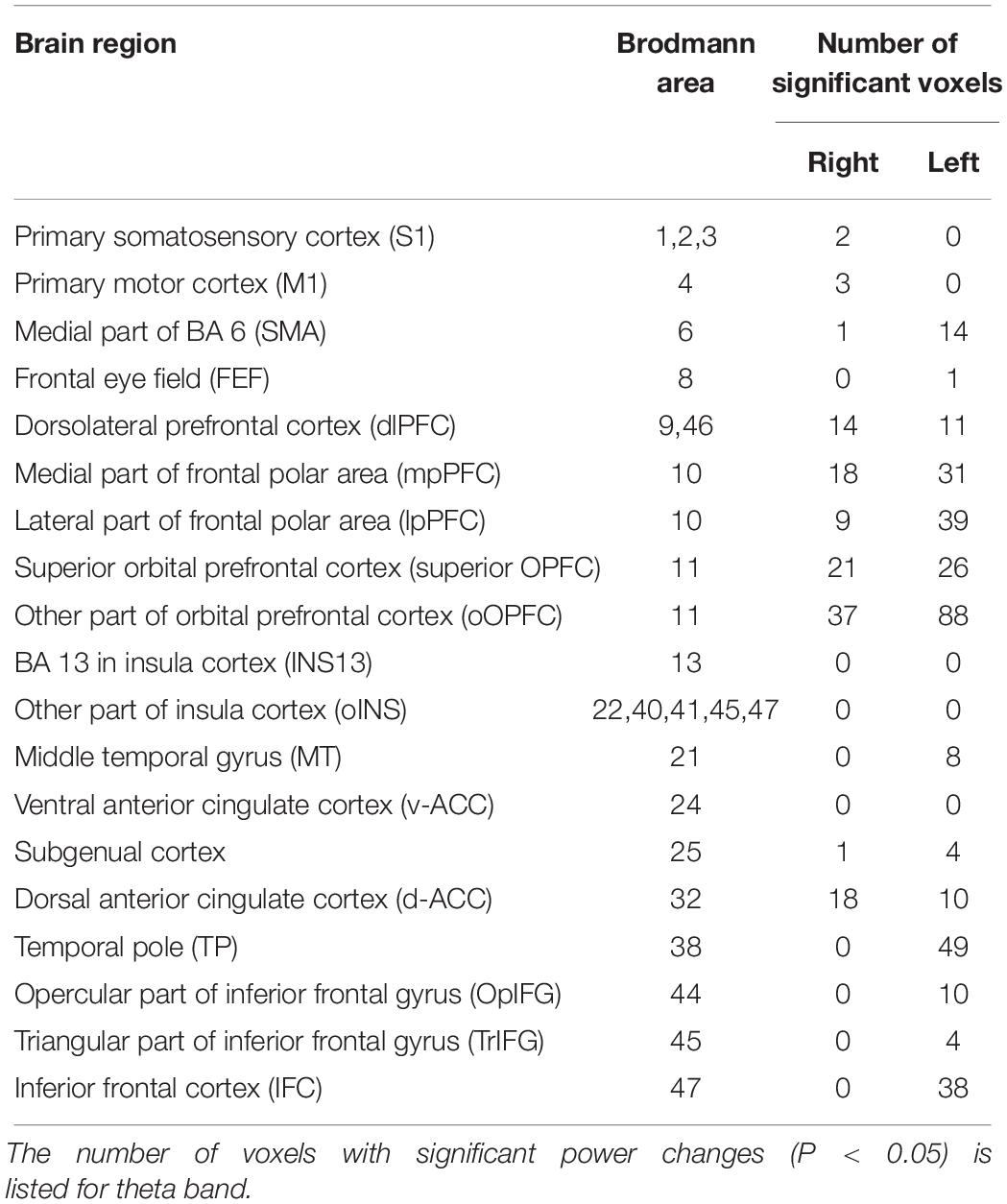
Table 3. Number of voxels with significant differences in CSD of theta oscillations between the MTrP and non-MTrP groups in each brain area.
Among these brain regions, the bilateral mpPFC, bilateral lpPFC, bilateral superior OPFC, bilateral OPFC, left TP, and left IFC had more than 30 voxels with significant differences in CSD responses between the two groups.
Relationship Between CSD and Hemodynamic Responses
Figure 7 shows the relationship between effects sizes of hemodynamic responses and logarithmic theta CSD responses during compression. The pPFC, OPFC, left TP, and IFC were selected as ROIs (see above section “Brain CSD Activity”). Statistical analyses by simple regression analysis revealed that the effect sizes of NIRS hemodynamic activity in the pPFC significantly and negatively correlated with the logarithmic theta CSD responses during compression in the pPFC [r = 0.41, F(1,30) = 5.88, P < 0.05]. In the other brain regions, there was no significant correlation between effect sizes of hemodynamic responses and logarithmic theta CSD responses (data not shown).
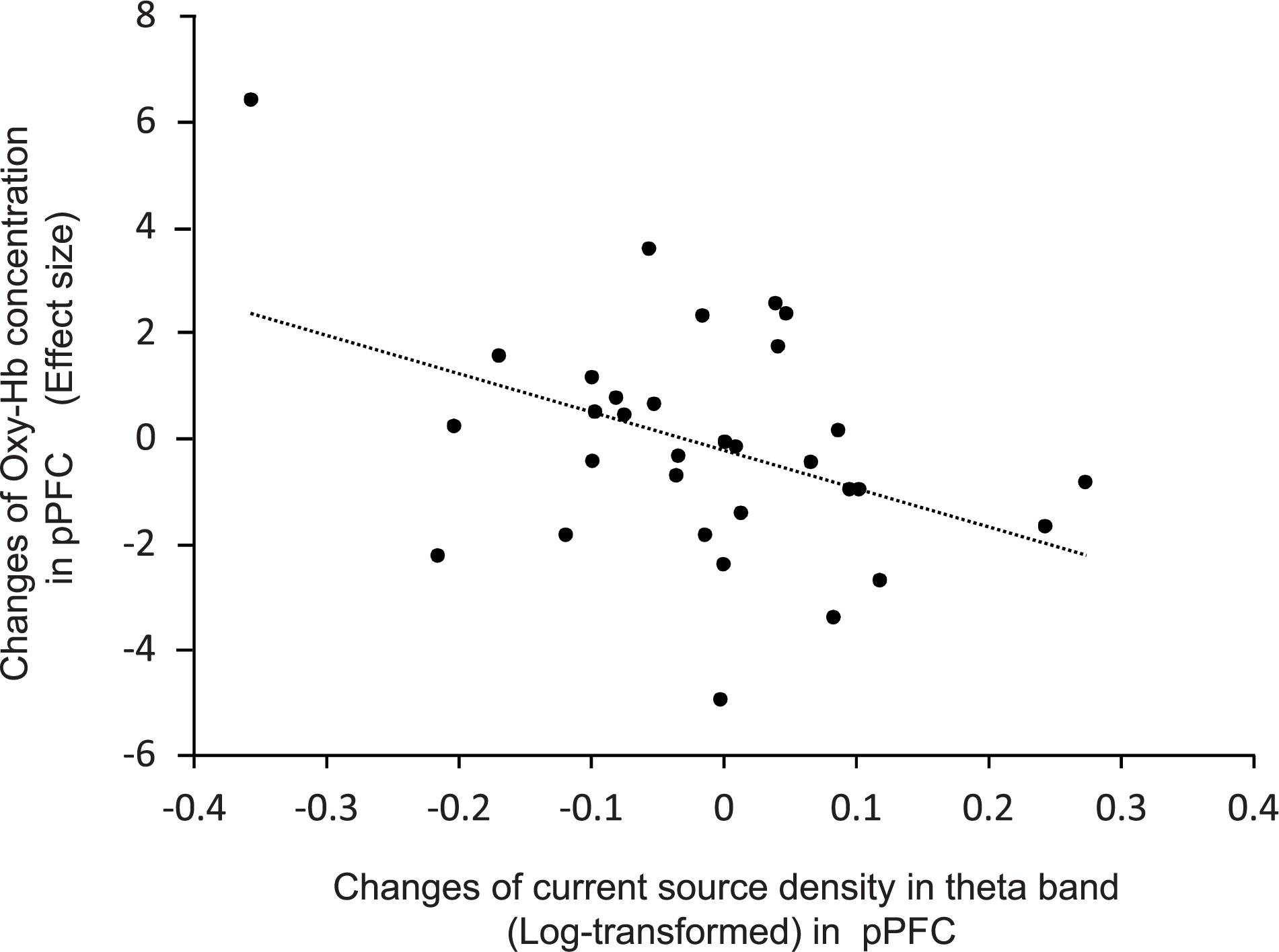
Figure 7. Relationships between changes in effect sizes of NIRS hemodynamic responses and changes in log-transformed CSD of EEG theta oscillations in the pPFC during compression.
Functional Connectivity During Compression
Although all possible pairs of ROIs were investigated in six frequency bands, there was only a significant difference in functional connectivity between the left mpPFC and left insula cortex in theta band between the MTrP and non-MTrP groups. Figure 8A shows functional connectivity in theta bands, which was significantly modulated by compression at the MTrP and non-MTrP. The statistical results in sLORETA indicated that only the functional connectivity between the left mpPFC and left INS13 was significantly different between the MTrP and non-MTrP groups (Figure 8A), and that this connectivity was significantly reduced during compression in the MTrP group than the non-MTrP group (Figure 8B). Furthermore, changes in functional connectivity in theta band between the left mpPFC and left INS13 by compression were significantly correlated with changes in VAS pain after compression [r = 0.41, F(1,30) = 5.97, P < 0.05] (Figure 8C).
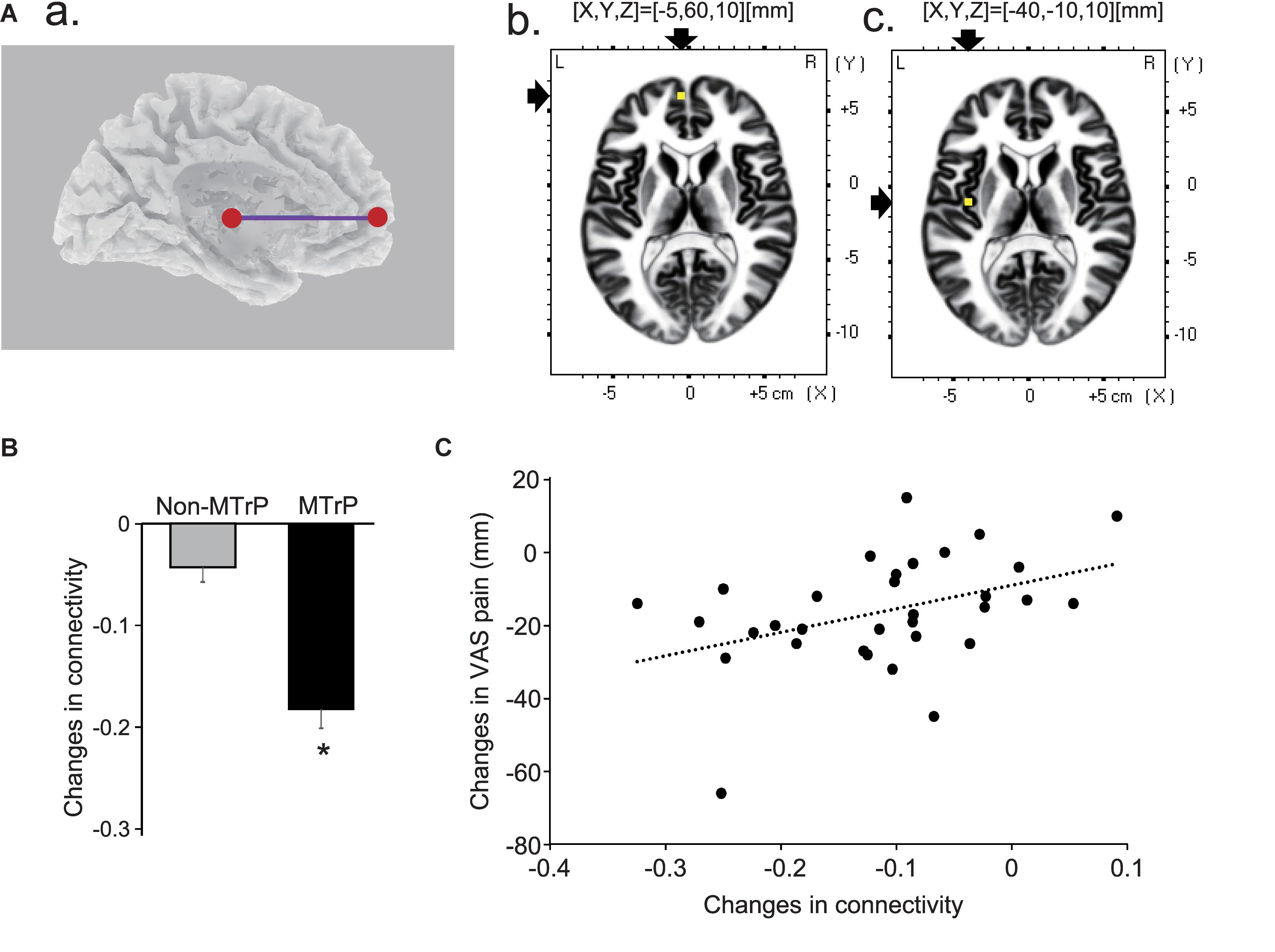
Figure 8. Changes in functional connectivity between the MTrP and non-MTrP groups during compression. (A) A purple line indicates a functional connectivity between the left mpPFC and left insula cortex (INS13) with significant reduction during compression in the MTrP group compared with the non-MTrP group. (a) Right two panels show the locations of the left mpPFC (b) and left insula cortex (c) in the MNI coordinates. (B) Comparison of changes in functional connectivity between the left mpPFC and insula cortex during compression between the non-MTrP and MTrP groups. ∗P < 0.05. (C) Relationships between functional connectivity changes shown in panel B and VAS pain changes during compression.
Discussion
Effects of Compression on Cerebral Hemodynamics
In the present study, compression in the quadratus lumborum muscle improved low back pain and hyperalgesia and decreased hemodynamic activity in the pPFC in the MTrP group than the non-MTrP group. These results corroborate a previous NIRS study, in which compression at MTrPs in the trapezius muscle decreased hemodynamic activity in the pPFC in patients with chronic neck pain (Morikawa et al., 2017). Simultaneous NIRS and fMRI recording showed that the decrease in hemodynamic activity (Oxy-Hb concentration) was correlated with a decrease in blood oxygenation level-dependent (BOLD) signals in fMRI (Seiyama et al., 2004; Maggioni et al., 2015). It is reported that a decrease in BOLD signals reflects inhibition of neuronal activity (Huber et al., 2014; Sten et al., 2017). These findings suggest that compression at MTrPs inhibits neuronal activity in the pPFC regardless of locations of MTrPs involved in musculoskeletal pain.
The medial PFC including the pPFC (BA 10) is involved in sensory, emotional, and cognitive processing of pain, regulation of the autonomic nervous system, and pain chronification (McKlveen et al., 2015; Peng et al., 2018). Overactivation in the medial PFC was observed in patients with chronic low back pain (Baliki et al., 2006). PFC activity is correlated with cardiovascular sympathetic nervous activity during cognitive stress (Umeno et al., 2003) and muscle sympathetic nervous activity during acute tonic muscle pain (Kobuch et al., 2018). Taken together, the findings suggest that activity in the medial PFC, including the pPFC, increases in response to chronic pain, which in turn increases sympathetic nervous activity. We previously reported that MTrP compression in the neck decreased hemodynamic responses in the pPFC, the activity of which positively correlated with sympathetic nervous activity in patients with chronic neck pain (Morikawa et al., 2017). Furthermore, sympathetic nervous activity was positively correlated with subjective neck pain (Morikawa et al., 2017). It has been hypothesized that hyperactivity of sympathetic nervous activity induces the sustained muscle contraction and release of substances induced by muscle ischemia, which in turn develops MTrPs in the muscles (Gerwin, 2008). Thus, reduction of sympathetic nervous activity by MTrP compression through suppression of the medial PFC might induce a decrease in muscle tone and removal of algesic substances due to blood flow improvement, which, in turn, ameliorates chronic pain and hyperalgesia (Morikawa et al., 2017). The present results suggest that the same mechanisms are involved in reduction of chronic low back pain during compression at MTrPs in the quadratus lumborum muscle.
Effects of Compression on EEG Oscillation
In this study, CSD of EEG theta oscillation increased more strongly in the anterior part of the brain including the pPFC in the MTrP group than the non-MTrP group. An EEG study reported that CSD of theta oscillations in the medial PFC was decreased during nociceptive stimulation and negatively correlated with pain intensity (Shao et al., 2012), whereas gamma oscillations in the PFC were positively correlated with subjective pain intensity in patients with chronic low back pain (May et al., 2019). Furthermore, some analgesic agents increased theta power (Malver et al., 2014). These results suggest that theta oscillations in the medial PFC are related to reduction in subjective pain.
In addition, CSD of theta oscillations in the medial PFC was negatively correlated with NIRS hemodynamic activity in the present study. Consistently, human non-invasive studies with simultaneous recordings of fMRI and EEG reported an inverse correlation between EEG theta oscillations and BOLD signals in the medial PFC (Mizuhara et al., 2004; Meltzer et al., 2007; Scheeringa et al., 2009; Michels et al., 2010; White et al., 2013). A theoretical study also suggests that neuronal activation increases BOLD signals and shifts the spectral profile of EEGs toward higher frequencies (Kilner et al., 2005). Taken together, the present EEG results also suggest that MTrP compression suppresses activity in the medial PFC including the pPFC, which may induce pain reduction.
Simultaneous EEG and ECG studies reported that an increase in theta oscillations in the PFC was associated with a reduction of sympathetic nervous activity or increment of parasympathetic nervous activity (Kubota et al., 2001; Takahashi et al., 2005; Tang et al., 2009). These results support the hypothesis that MTrP compression inhibits activity in the medial PFC, including the pPFC, which reduces sympathetic nervous activity. This reduction may in turn relieve chronic low back pain (see the above section “Effects of Compression on Cerebral Hemodynamics”).
Effects of Compression on Brain Functional Connectivity
In the present study, functional connectivity based on theta coherence between the pPFC and insula cortex was significantly decreased in the MTrP group. Furthermore, functional connectivity between the pPFC and insula cortex positively correlated with subjective low back pain. It is suggested that the insula cortex plays an essential role in pain information processing including perception/sensation of pain, affective aspects of pain, maintenance, and progress of chronic pain, etc. (Benison et al., 2011; Cauda et al., 2011; Deen et al., 2011; Chang et al., 2013; Segerdahl et al., 2015), while the pPFC is also implicated in chronic pain (Apkarian et al., 2001; Baliki et al., 2006; Morikawa et al., 2017). Consistent with the current study, previous fMRI studies reported that functional connectivity between the insula cortex and default mode network (DMN) including the medial PFC increased in patients with chronic pain including chronic low back pain and fibromyalgia and correlated with subjective pain intensity (Cauda et al., 2009; Napadow et al., 2010; Loggia et al., 2013). Furthermore, pharmacological and physical interventions to reduce pain decreased such connectivity, while maneuvers to increase pain increased the functional connectivity (Napadow et al., 2012; Harris et al., 2013; Loggia et al., 2013). The current results, along with the previous fMRI studies, suggest that functional connectivity between the insula and other brain regions including the pPFC is one of the important determinants for development of chronic pain.
The pathway from the insula to the medial PFC is involved in information processing of uncontrollable pain (Bräscher et al., 2016), and the medial PFC may be involved in facilitation of pain sensation (Pastoriza et al., 1996; Lin et al., 2014). The present results indicated that coherence in the theta bands was decreased by manual compression. Synchronized activity between distant brain regions indicated by EEG coherence, especially in theta bands, reflects information transfer or memory retrieval between such distant brain regions (Jacobs et al., 2006; Saalmann, 2014). Furthermore, chronic pain is hypothesized to be associated with formation and activation of nociceptive memory (Yi and Zhang, 2011). Taken together, these findings suggest that manual compression at MTrPs may interfere with information flow of nociceptive memory from the insula to the pPFC by decreasing theta coherence. Consistent with this idea, functional connectivity between the medial PFC and insula cortex was involved in persistence of chronic low back pain (Hashmi et al., 2012).
The mechanisms of reduction of theta coherence are uncertain in the present study. However, changes in theta coherence could be attributed to changes in oscillations in the thalamus due to manual compression at MTrPs. Acupuncture stimulation of acupoints, 71% of which match trigger points anatomically (Melzack et al., 1977), activates Aβ-, Aδ-, and C-fibers (Chen et al., 2014). Most thalamic nuclei respond to stimuli activating these fibers (Ren et al., 2009; Cardoso-Cruz et al., 2013; Whitt et al., 2013; Chen et al., 2014; Bastuji et al., 2016). These findings suggest that compression at MTrPs modulates thalamic activity. On the other hand, the thalamus is proposed to coordinate cortical coherence (Saalmann, 2014), and the thalamic nuclei modulate cortical theta oscillations differently depending on the subnuclei of the thalamus (Sarnthein and Jeanmonod, 2008; LeBlanc et al., 2014, 2017). These findings further suggest that compression at MTrPs may reduce theta coherence between the pPFC and insula through its effects on the thalamus.
Limitation
In the present study, we investigated effects of compression at active MTrPs on chronic low back pain. However, effects of compression at latent MTrPs are unknown in the present study. MTrPs are classified into active and latent MTrPs. Latent MTrPs are defined as follows: (1) presence of a hypersensitive spot in a taut band in a given muscle and (2) production of pain or referred pain that is not related to a given patient’s clinical pain complaint when latent MTrP(s) are stimulated by compression (Simons et al., 1999a, b). Levels of algesic substances and muscle stiffness were higher in active MTrPs than latent MTrPs (Shah and Gilliams, 2008; Shah et al., 2008; Calvo-Lobo et al., 2017). The number of active and latent MTrPs was greater in patients with chronic low back pain compared with healthy subjects (Iglesias-González et al., 2013). Furthermore, a previous study using patients with low back pain reported that muscle stiffness was higher while PPT was lower in lumbar areas with active and latent MTrPs compared with those with non-MTrP (Calvo-Lobo et al., 2017). These findings suggest that latent MTrPs could be also involved in low back pain. Further studies are required to investigate effects of compression at latent MTrPs in patients with chronic low back pain.
Second, palpation of the lumbar quadrate muscle is relatively difficult, compared with other muscles that are located superficially, since it could be palpated directly from the skin or indirectly below other muscles depending on its position (Simons et al., 1999b). Future studies such as those combined with elastography could more precisely diagnose active MTrPs in the lumbar quadrate muscle.
Conclusion
The present study investigated the neural mechanisms of analgesic effects of MTrP compression in chronic low back pain using NIRS and EEG measurement. Both NIRS and EEG results indicated that MTrP compression significantly decreased activity in the pPFC, as well as decreased functional connectivity between the pPFC and insula cortex, the strength of which between those regions was negatively correlated with subjective low back pain. The results, along with the previous studies, suggest that MTrP compression at the lumbar muscle reduces coherent activity in the pPFC and insula cortex, which may decrease sympathetic activity involved in muscle pain.
MTrP compression did not affect gamma oscillations in the present study. However, previous human studies reported that an increase in gamma oscillations, especially in higher-frequency bands (50–120 Hz), was associated with pain perception in the PFC and somatosensory cortex (Zhang et al., 2012; Schulz et al., 2015; Li et al., 2016; Zhou et al., 2018; Hu and Iannetti, 2019; May et al., 2019). Thus, the absence of gamma oscillation changes could be ascribed to the low-frequency band (31–50 Hz) of gamma oscillations analyzed in this study. Furthermore, cross-frequency coupling between theta and gamma oscillations was increased during painful sensory stimulation or in a state with chronic inflammatory pain (Liu et al., 2015; Wang et al., 2016). The current results, along with these previous studies, suggest that high-frequency gamma oscillations coupled with theta oscillations is transferred from the insula cortex to the PFC, which may be involved in the perception of chronic pain. Reduction of functional connectivity in theta bands by MTrP compression may decrease this transfer of high-frequency gamma oscillation. Further studies to investigate higher-frequency gamma oscillations are required to address the role of functional connectivity between the pPFC and insula in chronic pain perception.
Data Availability Statement
The data that support the findings in this study are available from the corresponding author HisN, upon reasonable request.
Ethics Statement
The studies involving human participants were reviewed and approved by the ethics committee of the University of Toyama. The patients/participants provided their written informed consent to participate in this study.
Author Contributions
HisN and KT designed the experiment. KK performed the experiment. KK, KT, and HisN analyzed the data and wrote the manuscript. HisN, HirN, JM, YT, SS, and TO revised the manuscript. All authors discussed the results, and approved the final manuscript.
Funding
This work was supported partly by Japan Society for the Promotion of Science KAKENHI (Grant nos. 16K01499 and 19K11341) and research funds from University of Toyama.
Conflict of Interest
The authors declare that the research was conducted in the absence of any commercial or financial relationships that could be construed as a potential conflict of interest.
Acknowledgments
We would like to thank Editage (www.editage.com) for English language editing.
Supplementary Material
The Supplementary Material for this article can be found online at: https://www.frontiersin.org/articles/10.3389/fnsys.2019.00068/full#supplementary-material
Footnotes
References
Apkarian, A. V., Thomas, P. S., Krauss, B. R., and Szeverenyi, N. M. (2001). Prefrontal cortical hyperactivity in patients with sympathetically mediated chronic pain. Neurosci. Lett. 311, 193–197. doi: 10.1016/S0304-3940(01)02122-X
Babiloni, C., Squitti, R., Del Percio, C., Cassetta, E., Ventriglia, M. C., Ferreri, F., et al. (2007). Free copper and resting temporal EEG rhythms correlate across healthy, mild cognitive impairment, and Alzheimer’s disease subjects. Clin. Neurophysiol. 118, 1244–1260. doi: 10.1016/j.clinph.2007.03.016
Baliki, M. N., Chialvo, D. R., Geha, P. Y., Levy, R. M., Harden, R. N., Parrish, T. B., et al. (2006). Chronic pain and the emotional brain: specific brain activity associated with spontaneous fluctuations of intensity of chronic back pain. J. Neurosci. 26, 12165–12173. doi: 10.1523/jneurosci.3576-06.2006
Baliki, M. N., Geha, P. Y., Jabakhanji, R., Harden, N., Schnitzer, T. J., and Apkarian, A. V. (2008). A preliminary fMRI study of analgesic treatment in chronic back pain and knee osteoarthritis. Mol. Pain 4:47. doi: 10.1186/1744-8069-4-47
Bastuji, H., Frot, M., Mazza, S., Perchet, C., Magnin, M., and Garcia-Larrea, L. (2016). Thalamic responses to nociceptive-specific input in humans: functional dichotomies and thalamo-cortical connectivity. Cereb. Cortex 26, 2663–2676. doi: 10.1093/cercor/bhv106
Benison, A. M., Chumachenko, S., Harrison, J. A., Maier, S. F., Falci, S. P., Watkins, L. R., et al. (2011). Caudal granular insular cortex is sufficient and necessary for the long-term maintenance of allodynic behavior in the rat attributable to mononeuropathy. J. Neurosci. 31, 6317–6328. doi: 10.1523/JNEUROSCI.0076-11.2011
Bräscher, A. K., Becker, S., Hoeppli, M. E., and Schweinhardt, P. (2016). Different brain circuitries mediating controllable and uncontrollable pain. J. Neurosci. 36, 5013–5025. doi: 10.1523/JNEUROSCI.1954-15.2016
Cagnie, B., Dewitte, V., Coppieters, I., Van Oosterwijck, J., Cools, A., and Danneels, L. (2013). Effect of ischemic compression on trigger points in the neck and shoulder muscles in office workers: a cohort study. J. Manipulative Physiol. Ther. 36, 482–489. doi: 10.1016/j.jmpt.2013.07.001
Calvo-Lobo, C., Diez-Vega, I., Martínez-Pascual, B., Fernández-Martínez, S., de la Cueva-Reguera, M., Garrosa-Martin, G., et al. (2017). Tensiomyography, sonoelastography, and mechanosensitivity differences between active, latent, and control low back myofascial trigger points: a cross-sectional study. Medicine 96:e6287. doi: 10.1097/MD.0000000000006287
Canuet, L., Ishii, R., Pascual-Marqui, R. D., Iwase, M., Kurimoto, R., Aoki, Y., et al. (2011). Resting-state EEG source localization and functional connectivity in schizophrenia-like psychosis of epilepsy. PLoS One 6:e27863. doi: 10.1371/journal.pone.0027863
Cardoso-Cruz, H., Sousa, M., Vieira, J. B., Lima, D., and Galhardo, V. (2013). Prefrontal cortex and mediodorsal thalamus reduced connectivity is associated with spatial working memory impairment in rats with inflammatory pain. Pain 154, 2397–2406. doi: 10.1016/j.pain.2013.07.020
Cauda, F., D’Agata, F., Sacco, K., Duca, S., Geminiani, G., and Vercelli, A. (2011). Functional connectivity of the insula in the resting brain. Neuroimage 55, 8–23. doi: 10.1016/j.neuroimage.2010.11.049
Cauda, F., Sacco, K., Duca, S., Cocito, D., D’Agata, F., Geminiani, G. C., et al. (2009). Altered resting state in diabetic neuropathic pain. PLoS One 4:e4542. doi: 10.1371/journal.pone.0004542
Chang, L. J., Yarkoni, T., Khaw, M. W., and Sanfey, A. G. (2013). Decoding the role of the insula in human cognition: functional parcellation and large-scale reverse inference. Cereb. Cortex 23, 739–749. doi: 10.1093/cercor/bhs065
Chen, S., Wang, S., Rong, P., Liu, J., Zhang, H., and Zhang, J. (2014). Acupuncture for refractory epilepsy: role of thalamus. Evid. Based Complement. Alternat. Med. 2014:950631. doi: 10.1155/2014/950631
Coppieters, I., Meeus, M., Kregel, J., Caeyenberghs, K., De Pauw, R., Goubert, D., et al. (2016). Relations between brain alterations and clinical pain measures in chronic musculoskeletal pain: a systematic review. J. Pain 17, 949–962. doi: 10.1016/j.jpain.2016.04.005
Cui, D., Liu, J., Bian, Z., Li, Q., Wang, L., and Li, X. (2014). Cortical source multivariate EEG synchronization analysis on amnestic mild cognitive impairment in type 2 diabetes. Sci. World J. 2014:52316. doi: 10.1155/2014/523216
Deen, B., Pitskel, N. B., and Pelphrey, K. A. (2011). Three systems of insular functional connectivity identified with cluster analysis. Cereb. Cortex 21, 1498–1506. doi: 10.1093/cercor/bhq186
Delorme, A., and Makeig, S. (2004). EEGLAB: an open source toolbox for analysis of single-trial EEG dynamics including independent component analysis. J. Neurosci. Methods 134, 9–21. doi: 10.1016/j.jneumeth.2003.10.009
Fukui, Y., Ajichi, Y., and Okada, E. (2003). Monte Carlo prediction of near-infrared light propagation in realistic adult and neonatal head models. Appl. Opt. 42, 2881–2887. doi: 10.1364/AO.42.002881
Gerwin, R. D. (2008). The taut band and other mysteries of the trigger point: an examination of the mechanisms relevant to the development and maintenance of the trigger point. J. Musculoskelet. Pain. 16, 115–121. doi: 10.1080/10582450801960081
Gerwin, R. D., Shannon, S., Hong, C. Z., Hubbard, D., and Gevirtz, R. (1997). Interrater reliability in myofascial trigger point examination. Pain 69, 65–73. doi: 10.1016/S0304-3959(96)03248-3244
Hains, G., Descarreaux, M., and Hains, F. (2010). Chronic shoulder pain of myofascial origin: a randomized clinical trial using ischemic compression therapy. J. Manip. Physiol. Ther. 33, 362–369. doi: 10.1016/j.jmpt.2010.05.003
Harris, R. E., Napadow, V., Huggins, J. P., Pauer, L., Kim, J., Hampson, J., et al. (2013). Pregabalin rectifies aberrant brain chemistry, connectivity, and functional response in chronic pain patients. Anesthesiology 119, 1453–1464. doi: 10.1097/ALN.0000000000000017
Hashmi, J. A., Baria, A. T., Baliki, M. N., Huang, L., Schnitzer, T. J., and Apkarian, A. V. (2012). Brain networks predicting placebo analgesia in a clinical trial for chronic back pain. Pain 153, 2393–2402. doi: 10.1016/j.pain.2012.08.008
Hocking, M. J. (2013). Exploring the central modulation hypothesis: do ancient memory mechanisms underlie the pathophysiology of trigger points? Curr. Pain Headache Rep. 17:347. doi: 10.1007/s11916-013-0347-346
Hoshi, Y., Kobayashi, N., and Tamura, M. (2001). Interpretation of near-infrared spectroscopy signals: a study with a newly developed perfused rat brain model. J. Appl. Physiol. 90, 1657–1662. doi: 10.1152/jappl.2001.90.5.1657
Hou, C. R., Tsai, L. C., Cheng, K. F., Chung, K. C., and Hong, C. Z. (2002). Immediate effects of various physical therapeutic modalities on cervical myofascial pain and trigger-point sensitivity. Arch. Phys. Med. Rehabil. 83, 1406–1414. doi: 10.1053/apmr.2002.34834
Hu, L., and Iannetti, G. D. (2019). Neural indicators of perceptual variability of pain across species. Proc. Natl. Acad. Sci. U.S.A. 116, 1782–1791. doi: 10.1073/pnas.1812499116
Huber, L., Goense, J., Kennerley, A. J., Ivanov, D., Krieger, S. N., Lepsien, J., et al. (2014). Investigation of the neurovascular coupling in positive and negative BOLD responses in human brain at 7 T. Neuroimage 97, 349–362. doi: 10.1016/j.neuroimage.2014.04.022
Iglesias-González, J. J., Muñoz-García, M. T., Rodrigues-de-Souza, D. P., Alburquerque-Sendín, F., and Fernández-de-Las-Peñas, C. (2013). Myofascial trigger points, pain, disability, and sleep quality in patients with chronic nonspecific low back pain. Pain Med. 14, 1964–1970. doi: 10.1111/pme.12224
Ishikuro, K., Urakawa, S., Takamoto, K., Ishikawa, A., Ono, T., and Nishijo, H. (2014). Cerebral functional imaging using near-infrared spectroscopy during repeated performances of motor rehabilitation tasks tested on healthy subjects. Front. Hum. Neurosci. 8:292. doi: 10.3389/fnhum.2014.00292
Jacobs, J., Hwang, G., Curran, T., and Kahana, M. J. (2006). EEG oscillations and recognition memory: theta correlates of memory retrieval and decision making. Neuroimage 32, 978–987. doi: 10.1016/j.neuroimage.2006.02.018
Joseph, E. (2009). The Muscle and Bone Palpation Manual With Trigger Points, Referral Patterns and Stretching. St. Louis: Mosby Elsevier.
Jung, T. P., Makeig, S., Humphries, C., Lee, T. W., McKeown, M. J., Iragui, V., et al. (2000). Removing electroencephalographic artifacts by blind source separation. Psychophysiology 37, 163–178. doi: 10.1017/s0048577200980259
Jurcak, V., Tsuzuki, D., and Dan, I. (2007). 10/20, 10/10, and 10/5 systems revisited: their validity as relative head-surface-based positioning systems. Neuroimage 34, 1600–1611. doi: 10.1016/j.neuroimage.2006.09.024
Kilner, J. M., Mattout, J., Henson, R., and Friston, K. J. (2005). Hemodynamic correlates of EEG: a heuristic. Neuroimage 28, 280–286. doi: 10.1016/j.neuroimage.2005.06.008
Kobuch, S., Fazalbhoy, A., Brown, R., Macefield, V. G., and Henderson, L. A. (2018). Muscle sympathetic nerve activity-coupled changes in brain activity during sustained muscle pain. Brain Behav. 8:e00888. doi: 10.1002/brb3.888
Kregel, J., Coppieters, I., DePauw, R., Malfliet, A., Danneels, L., Nijs, J., et al. (2017). Does conservative treatment change the brain in patients with chronic musculoskeletal pain? a systematic review. Pain Physician. 20, 139–154.
Kubota, Y., Sato, W., Toichi, M., Murai, T., Okada, T., Hayashi, A., et al. (2001). Frontal midline theta rhythm is correlated with cardiac autonomic activities during the performance of an attention demanding meditation procedure. Brain Res. Cogn. Brain Res. 11, 281–287. doi: 10.1016/s0926-6410(00)00086-0
LeBlanc, B. W., Cross, B., Smith, K. A., Roach, C., Xia, J., Chao, Y. C., et al. (2017). Thalamic bursts down-regulate cortical theta and nociceptive behavior. Sci. Rep. 7:2482. doi: 10.1038/s41598-017-02753-2756
LeBlanc, B. W., Lii, T. R., Silverman, A. E., Alleyne, R. T., and Saab, C. Y. (2014). Cortical theta is increased while thalamocortical coherence is decreased in rat models of acute and chronic pain. Pain 155, 773–782. doi: 10.1016/j.pain.2014.01.013
Li, J., Zhang, J. H., Yi, T., Tang, W. J., Wang, S. W., and Dong, J. C. (2014). Acupuncture treatment of chronic low back pain reverses an abnormal brain default mode network in correlation with clinical pain relief. Acupunct. Med. 32, 102–108. doi: 10.1136/acupmed-2013-010423
Li, L., Liu, X., Cai, C., Yang, Y., Li, D., Xiao, L., et al. (2016). Changes of gamma-band oscillatory activity to tonic muscle pain. Neurosci. Lett. 627, 126–131. doi: 10.1016/j.neulet.2016.05.067
Lin, H. C., Huang, Y. H., Chao, T. H., Lin, W. Y., Sun, W. Z., and Yen, C. T. (2014). Gabapentin reverses central hypersensitivity and suppresses medial prefrontal cortical glucose metabolism in rats with neuropathic pain. Mol. Pain 10:63. doi: 10.1186/1744-8069-10-63
Liu, C. C., Chien, J. H., Kim, J. H., Chuang, Y. F., Cheng, D. T., Anderson, W. S., et al. (2015). Cross-frequency coupling in deep brain structures upon processing the painful sensory inputs. Neuroscience 303, 412–421. doi: 10.1016/j.neuroscience.2015.07.010
Loggia, M. L., Kim, J., Gollub, R. L., Vangel, M. G., Kirsch, I., Kong, J., et al. (2013). Default mode network connectivity encodes clinical pain: an arterial spin labeling study. Pain 154, 24–33. doi: 10.1016/j.pain.2012.07.029
Maggioni, E., Molteni, E., Zucca, C., Reni, G., Cerutti, S., Triulzi, F. M., et al. (2015). Investigation of negative BOLD responses in human brain through NIRS technique. A visual stimulation study. Neuroimage 108, 410–422. doi: 10.1016/j.neuroimage.2014.12.074
Makeig, S., Jung, T. P., Bell, A. J., Ghahremani, D., and Sejnowski, T. J. (1997). Blind separation of auditory event-related brain responses into independent components. Proc. Natl. Acad. Sci. U.S.A. 94, 10979–10984. doi: 10.1073/pnas.94.20.10979
Makeig, S., Westerfield, M., Jung, T. P., Covington, J., Townsend, J., Sejnowski, T. J., et al. (1999). Functionally independent components of the late positive event-related potential during visual spatial attention. J. Neurosci. 19, 2665–2680. doi: 10.1523/jneurosci.19-07-02665.1999
Malver, L. P., Brokjaer, A., Staahl, C., Graversen, C., Andresen, T., and Drewes, A. M. (2014). Electroencephalography and analgesics. Br. J. Clin. Pharmacol. 77, 72–95. doi: 10.1111/bcp.12137
May, E. S., Nickel, M. M., Ta Dinh, S., Tiemann, L., Heitmann, H., Voth, I., et al. (2019). Prefrontal gamma oscillations reflect ongoing pain intensity in chronic back pain patients. Hum. Brain Mapp. 40, 293–305. doi: 10.1002/hbm.24373
McKlveen, J. M., Myers, B., and Herman, J. P. (2015). The medial prefrontal cortex: coordinator of autonomic, neuroendocrine and behavioural responses to stress. J. Neuroendocrinol. 27, 446–456. doi: 10.1111/jne.12272
Meltzer, J. A., Negishi, M., Mayes, L. C., and Constable, R. T. (2007). Individual differences in EEG theta and alpha dynamics during working memory correlate with fMRI responses across subjects. Clin. Neurophysiol. 118, 2419–2436. doi: 10.1016/j.clinph.2007.07.023
Melzack, R., Stillwell, D. M., and Fox, E. J. (1977). Trigger points and acupuncture points for pain: correlations and implications. Pain 3, 3–23. doi: 10.1016/0304-3959(77)90032-x
Metting van Rijin, A. C., Peper, A., and Grimbergen, C. A. (1990). High-quality recording of bioelectric events. Part 1. Interference reduction, theory and practice. Med. Biol. Eng. Comput. 25, 389–397. doi: 10.1007/bf02441961
Michels, L., Bucher, K., Lunchinger, R., Klaver, P., Martin, E., Jeanmonod, D., et al. (2010). Simultaneous EEG-fMRI during a working memory task: modulations in low and high frequency bands. PLoS One 5:e10298. doi: 10.1371/journal.pone.0010298
Mizuhara, H., Wang, L. Q., Kobayashi, K., and Yamaguchi, Y. (2004). A long-range cortical network emerging with theta oscillation in a mental task. Neuroreport 15, 1233–1238. doi: 10.1097/01.wnr.0000126755.09715.b3
Morikawa, Y., Takamoto, K., Nishimaru, H., Taguchi, T., Urakawa, S., Sakai, S., et al. (2017). Compression at myofascial trigger point on chronic neck pain provides pain relief through the prefrontal cortex and autonomic nervous system: a pilot study. Front. Neurosci. 11:186. doi: 10.3389/fnins.2017.00186
Nakamichi, N., Takamoto, K., Nishimaru, H., Fujiwara, K., Takamura, Y., Matsumoto, J., et al. (2018). Cerebral hemodynamics in speech-related cortical areas: articulation learning involves the inferior frontal gyrus, ventral sensory-motor cortex, and parietal-temporal sylvian area. Front. Neurol. 9:939. doi: 10.3389/fneur.2018.00939
Nakamura, M., Nishiwaki, Y., Ushida, T., and Toyama, Y. (2011). Prevalence and characteristics of chronic musculoskeletal pain in Japan. J. Orthop. Sci. 16, 424–432. doi: 10.1007/s00776-011-0102-y
Napadow, V., Kim, J., Clauw, D. J., and Harris, R. E. (2012). Decreased intrinsic brain connectivity is associated with reduced clinical pain in fibromyalgia. Arthritis Rheum. 64, 2398–2403. doi: 10.1002/art.34412
Napadow, V., LaCount, L., Park, K., As-Sanie, S., Clauw, D. J., and Harris, R. E. (2010). Intrinsic brain connectivity in fibromyalgia is associated with chronic pain intensity. Arthritis Rheum. 62, 2545–2555. doi: 10.1002/art.27497
Nichols, T. E., and Holmes, A. P. (2002). Nonparametric permutation tests for functional neuroimaging: a primer with examples. Hum. Brain Mapp. 15, 1–25. doi: 10.1002/hbm.1058
Niddam, D. M. (2009). Brain manifestation and modulation of pain from myofascial trigger points. Curr. Pain Headache Rep. 13, 370–375. doi: 10.1007/s11916-009-0060-7
Niddam, D. M., Chan, R. C., Lee, S. H., Yeh, T. C., and Hsieh, J. C. (2008). Central representation of hyperalgesia from myofascial trigger point. Neuroimage 39, 1299–1306. doi: 10.1016/j.neuroimage.2007.09.051
Niddam, D. M., Lee, S. H., Su, Y. T., and Chan, R. C. (2017). Brain structural changes in patients with chronic myofascial pain. Eur. J. Pain 21, 148–158. doi: 10.1002/ejp.911
Niederer, P., Mudra, R., and Keller, E. (2008). Monte carlo simulation of light propagation in adult brain: influence of tissue blood content and indocyanine green. Opto. Electron Rev. 16, 124–130. doi: 10.2478/s11772-008-0012-15
Pascual-Marqui, R. D. (2002). Standardized low-resolution brain electromagnetic tomography (sLORETA): technical details. Methods Find. Exp. Clin. Pharmacol. 24(Suppl. D), 5–12.
Pascual-Marqui, R. D. (2007a). Coherence and phase synchronization: generalization to pairs of multivariate time series, and removal of zero-lag contributions. rXiv. [Preprint]. Available at: https://arxiv.org/abs/0706.1776 (accessed September 1, 2019).
Pascual-Marqui, R. D. (2007b). Instantaneous and lagged measurements of linear and nonlinear dependence between groups of multivariate time series: frequency decomposition. arXiv. [Preprint]. Available at: https://arxiv.org/abs/0711.1455 (accessed September 1, 2019).
Pastoriza, L. N., Morrow, T. J., and Casey, K. L. (1996). Medial frontal cortex lesions selectively attenuate the hot plate response: possible nocifensive apraxia in the rat. Pain 64, 11–17. doi: 10.1016/0304-3959(95)00070-4
Peng, K., Steele, S. C., Becerra, L., and Borsook, D. (2018). Brodmann area 10: collating, integrating and high level processing of nociception and pain. Prog. Neurobiol. 161, 1–22. doi: 10.1016/j.pneurobio.2017.11.004
Ploner, M., Sorg, C., and Gross, J. (2017). Brain rhythms of pain. Trends Cogn. Sci. 21, 100–110. doi: 10.1016/j.tics.2016.12.001
Ren, Y., Zhang, L., Lu, Y., Yang, H., and Westlund, K. N. (2009). Central lateral thalamic neurons receive noxious visceral mechanical and chemical input in rats. J. Neurophysiol. 102, 244–258. doi: 10.1152/jn.90985.2008
Saager, R. B., Telleri, N. L., and Berger, A. J. (2011). Two-detector corrected near infrared spectroscopy (C-NIRS) detects hemodynamic activation responses more robustly than single-detector NIRS. Neuroimage 55, 1679–1685. doi: 10.1016/j.neuroimage.2011.01.043
Saalmann, Y. B. (2014). Intralaminar and medial thalamic influence on cortical synchrony, information transmission and cognition. Front. Syst. Neurosci. 8:83. doi: 10.3389/fnsys.2014.00083
Sarnthein, J., and Jeanmonod, D. (2008). High thalamocortical theta coherence in patients with neurogenic pain. Neuroimage 39, 1910–1917. doi: 10.1016/j.neuroimage.2007.10.019
Sarnthein, J., Stern, J., Aufenberg, C., Rousson, V., and Jeanmonod, D. (2005). Increased EEG power and slowed dominant frequency in patients with neurogenic pain. Brain 129, 55–64. doi: 10.1093/brain/awh631
Sato, T., Nambu, I., Takeda, K., Aihara, T., Yamashita, O., Isogaya, Y., et al. (2016). Reduction of global interference of scalp-hemodynamics in functional near-infrared spectroscopy using short distance probes. Neuroimage 141, 120–132. doi: 10.1016/j.neuroimage.2016.06.054
Scheeringa, R., Petersonn, K. M., Oostenveld, R., Norris, D. G., Hagoort, P., and Bastiaansen, M. C. (2009). Trial-by-trial coupling between EEG and BOLD identifies networks related to alpha and theta EEG power increases during working memory maintenance. Neuroimage 44, 1224–1238. doi: 10.1016/j.neuroimage.2008.08.041
Schulz, E., May, E. S., Postorino, M., Tiemann, L., Nickel, M. M., Witkovsky, V., et al. (2015). Prefrontal gamma oscillations encode tonic pain in humans. Cereb. Cortex 25, 4407–4414. doi: 10.1093/cercor/bhv043
Schytz, H. W., Wienecke, T., Jensen, L. T., Selb, J., Boas, D. A., and Ashina, M. (2009). Changes in cerebral blood flow after acetazolamide: an experimental study comparing near-infrared spectroscopy and SPECT. Eur. J. Neurol. 16, 461–467. doi: 10.1111/j.1468-1331.2008.02398.x
Segerdahl, A. R., Mezue, M., Okell, T. W., Farrar, J. T., and Tracey, I. (2015). The dorsal posterior insula subserves a fundamental role in human pain. Nat. Neurosci. 18, 499–500. doi: 10.1038/nn.3969
Seiyama, A., Hazeki, O., and Tamura, M. (1988). Noninvasive quantitative analysis of blood oxygenation in rat skeletal muscle. J. Biochem. 103, 419–424. doi: 10.1093/oxfordjournals.jbchem.a122285
Seiyama, A., Seki, J., Tanabe, H. C., Sase, I., Takatsuki, A., Miyauchi, S., et al. (2004). Circulatory basis of fMRI signals: relationship between changes in the hemodynamic parameters and BOLD signal intensity. Neuroimage 21, 1204–1214. doi: 10.1016/j.neuroimage.2003.12.002
Shah, J. P., Danoff, J. V., Desai, M. J., Parikh, S., Nakamura, L. Y., Phillips, T. M., et al. (2008). Biochemicals associated with pain and inflammation are elevated in sites near to and remote from active myofascial trigger points. Arch. Phys. Med. Rehabil. 89, 16–23. doi: 10.1016/j.apmr.2007.10.018
Shah, J. P., and Gilliams, E. A. (2008). Uncovering the biochemical milieu of myofascial trigger points using in vivo microdialysis: an application of muscle pain concepts to myofascial pain syndrome. J. Bodyw. Mov. Ther. 12, 371–384. doi: 10.1016/j.jbmt.2008.06.006
Shah, J. P., Thaker, N., Heimur, J., Aredo, J. V., Sikdar, S., and Gerber, L. (2015). Myofascial trigger points then and now: a historical and scientific perspective. PM R 7, 746–761. doi: 10.1016/j.pmrj.2015.01.024
Shao, S., Shen, K., Yu, K., Wilder-Smith, E. P., and Li, X. (2012). Frequency-domain EEG source analysis for acute tonic cold pain perception. Clin. Neurophysiol. 123, 2042–2049. doi: 10.1016/j.clinph.2012.02.084
Simons, D. G., Travell, J. G., and Simons, L. S. (1999a). Myofascial Pain and Dysfunction, The Trigger Point Manual, The Upper Extremities, 2nd Edn, Vol. 1, Baltimore: Williams and Wilkins.
Simons, D. G., Travell, J. G., and Simons, L. S. (1999b). Myofascial Pain and Dysfunction, The Trigger Point Manual, The Lower Extremities, 2nd Edn, Vol. 2, Baltimore: Williams and Wilkins.
Sten, S., Lundengard, K., Witt, S., Cedersund, G., Elinder, F., and Engstorm, M. (2017). Neural inhibition can explain negative BOLD responses: a mechanistic modelling and fMRI study. Neuroimage 158, 219–231. doi: 10.1016/j.neuroimage.2017.07.002
Stern, J., Jeanmonod, D., and Sarnthein, J. (2006). Persistent EEG overactivation in the cortical pain matrix of neurogenic pain patients. Neuroimage 31, 721–731. doi: 10.1016/j.neuroimage.2005.12.042
Sugai, K., Tsuji, O., Matsumoto, M., Nishiwaki, Y., and Nakamura, M. (2017). Chronic musculoskeletal pain in Japan (the final report of the 3-year longitudinal study): association with a future decline in activities of daily living. J. Orthop. Surg. 25:2309499017727945. doi: 10.1177/2309499017727945
Takahashi, T., Murata, T., Hamada, T., Omori, M., Kosaka, H., Kikuchi, M., et al. (2005). Changes in EEG and autonomic nervous activity during meditation and their association with personality traits. Int. J. Psychophysiol. 55, 199–207. doi: 10.1016/j.ijpsycho.2004.07.004
Takamoto, K., Bito, I., Urakawa, S., Sakai, S., Kigawa, M., Ono, T., et al. (2015). Effects of compression at myofascial trigger points in patients with acute low back pain: a randomized controlled trial. Eur. J. Pain 19, 1186–1196. doi: 10.1002/ejp.694
Takeuchi, M., Hori, E., Takamoto, K., Tran, A. H., Satoru, K., Ishikawa, A., et al. (2009). Brain cortical mapping by simultaneous recording of functional near infrared spectroscopy and electroencephalograms from the whole brain during right median nerve stimulation. Brain Topogr. 22, 197–214. doi: 10.1007/s10548-009-0109-102
Tang, Y. Y., Ma, Y., Fan, Y., Feng, H., Wang, J., Feng, S., et al. (2009). Central and autonomic nervous system interaction is altered by short-term meditation. Proc. Natl. Acad. Sci. U.S.A. 106, 8865–8870. doi: 10.1073/pnas.0904031106
Tong, Y., Lindsey, K. P., and deB Frederick, B. (2011). Partitioning of physiological noise signals in the brain with concurrent near-infrared spectroscopy and fMRI. J. Cereb. Blood Flow Metab. 31, 2352–2362. doi: 10.1038/jcbfm.2011.100
Tsuzuki, D., Jurcak, V., Singh, A., Okamoto, M., Watanabe, E., and Dan, I. (2007). Virtual spatial registration of stand-alone fNIRS data to MNI space. Neuroimage 34, 1506–1518. doi: 10.1016/j.neuroimage.2006.10.043
Umeno, K., Hori, E., Tabuchi, E., Takakura, H., Miyamoto, K., Ono, T., et al. (2003). Gamma-band EEGs predict autonomic responses during mental arithmetic. Neuroreport 14, 477–480. doi: 10.1097/00001756-200303030-00036
Wang, J., Wang, J., Xing, G. G., Li, X., and Wan, Y. (2016). Enhanced gamma oscillatory activity in rats with chronic inflammatory pain. Front. Neurosci. 10:489. doi: 10.3389/fnins.2016.00489
White, T. P., Jansen, M., Doege, K., Mullinger, K. J., Park, S. B., Liddle, E. B., et al. (2013). Theta power during encoding predicts subsequent-memory performance and default mode network deactivation. Hum. Brain Mapp. 34, 2929–2943. doi: 10.1002/hbm.22114
Whitt, J. L., Masri, R., Pulimood, N. S., and Keller, A. (2013). Pathological activity in mediodorsal thalamus of rats with spinal cord injury pain. J. Neurosci. 33, 3915–3926. doi: 10.1523/JNEUROSCI.2639-12.2013
Wray, S., Cope, M., Delpy, D. T., Wyatt, J. S., and Reynolds, E. O. (1988). Characterization of the near infrared absorption spectra of cytochrome aa3 and haemoglobin for the non-invasive monitoring of cerebral oxygenation. Biochim. Biophys. Acta 933, 184–192. doi: 10.1016/0005-2728(88)90069-90062
Yamada, T., Umeyama, S., and Matsuda, K. (2009). Multidistance probe arrangement to eliminate artifacts in functional near-infrared spectroscopy. J. Biomed. Opt. 14:064034. doi: 10.1117/1.3275469
Yamada, T., Umeyama, S., and Ohashi, M. (2015). Removal of motion artifacts originating from optode fluctuations during functional near-infrared spectroscopy measurements. Biomed. Opt. Express 6, 4632–4649. doi: 10.1364/BOE.6.004632
Yasui, H., Takamoto, K., Hori, E., Urakawa, S., Nagashima, Y., Yada, Y., et al. (2010). Significant correlation between autonomic nervous activity and cerebral hemodynamics during thermotherapy on the neck. Auton. Neurosci. 156, 96–103. doi: 10.1016/j.autneu.2010.03.011
Yasumura, A., Kokubo, N., Yamamoto, H., Yasumura, Y., Nakagawa, E., Kaga, M., et al. (2014). Neurobehavioral and hemodynamic evaluation of stroop and reverse stroop interference in children with attention-deficit/hyperactivity disorder. Brain Dev. 36, 97–106. doi: 10.1016/j.braindev.2013.01.005
Yi, M., and Zhang, H. (2011). Nociceptive memory in the brain: cortical mechanisms of chronic pain. J. Neurosci. 31, 13343–13345. doi: 10.1523/JNEUROSCI.3279-11.2011
Zhang, Z. G., Hu, L., Hung, Y. S., Mouraux, A., and Iannetti, G. D. (2012). Gamma-band oscillations in the primary somatosensory cortex–a direct and obligatory correlate of subjective pain intensity. J. Neurosci. 32, 7429–7438. doi: 10.1523/JNEUROSCI.5877-11.2012
Keywords: chronic low back pain, myofascial trigger point, prefrontal cortex, hemodynamic activity, oscillation, functional connectivity
Citation: Kodama K, Takamoto K, Nishimaru H, Matsumoto J, Takamura Y, Sakai S, Ono T and Nishijo H (2019) Analgesic Effects of Compression at Trigger Points Are Associated With Reduction of Frontal Polar Cortical Activity as Well as Functional Connectivity Between the Frontal Polar Area and Insula in Patients With Chronic Low Back Pain: A Randomized Trial. Front. Syst. Neurosci. 13:68. doi: 10.3389/fnsys.2019.00068
Received: 11 September 2019; Accepted: 28 October 2019;
Published: 13 November 2019.
Edited by:
James W. Grau, Texas A&M University, United StatesReviewed by:
César Calvo Lobo, Universidad de León, Ponferrada, SpainCésar Fernández-de-las-Peñas, Rey Juan Carlos University, Spain
Noureddin Nakhostin Ansari, Tehran University of Medical Sciences, Iran
Copyright © 2019 Kodama, Takamoto, Nishimaru, Matsumoto, Takamura, Sakai, Ono and Nishijo. This is an open-access article distributed under the terms of the Creative Commons Attribution License (CC BY). The use, distribution or reproduction in other forums is permitted, provided the original author(s) and the copyright owner(s) are credited and that the original publication in this journal is cited, in accordance with accepted academic practice. No use, distribution or reproduction is permitted which does not comply with these terms.
*Correspondence: Hisao Nishijo, nishijo@med.u-toyama.ac.jp