- Department of Molecular, Cellular and Developmental Biology, University of Michigan, Ann Arbor, MI, United States
Measurements of population activity in alert animals have demonstrated that the intrinsic response state of the cortex has profound effects on the neuronal representation of sensory inputs, raising the possibility that cortical state could influence the behavioral performance in perceptual learning (PL). PL is a process by which sensory experience leads to gradual and semi-permanent improvements in perceptual judgment, and it is generally agreed that these improvements are modulated by sensory cortical areas. Although the precise neural mechanisms underlying the improved perceptual judgment remain unclear, cortical state has been shown to impact the behavioral outcome of PL. We discuss several ways in which cortical state might influence PL based on the recent evidence for state-dependent modulation of sensory encoding. Conversely, training in a certain perceptual task feeds back to modulate cortical state, suggesting a bi-directional relationship between cortical state and behavioral outcomes of PL. We highlight the recent studies that shed light on the mechanism of the interplay between cortical state and PL.
Introduction
Cortical state classically refers to the dynamics of neuronal population activity in a cortical network (Harris and Thiele, 2011). Transitions of cortical states have been typically described as changes of spontaneous ongoing network activity. Early studies using electroencephalogram (EEG) and local field potential (LFP) recordings have revealed two fundamental states that exist in the neocortex: a synchronized state characterized by large-amplitude, low-frequency (0.2–10 Hz) spontaneous fluctuations between the up and down phases, and a desynchronized state characterized by small-amplitude, high-frequency (25–100 Hz) fluctuations whereby neighboring neurons spike more independently (Steriade et al., 2001). Conventionally, the synchronized state has been associated with sleeping or anesthetized brain, and the desynchronized state with alert brain (Steriade et al., 2001). The transition between these two activity states plays a central role in modulating sensory-evoked population activity in the cortex. Recent studies in head-fixed awake rodents have demonstrated that waking state actually consists of distinct “substates,” including a state similar to the synchronized state usually observed during sleep (Figure 1; Poulet and Petersen, 2008; Sachidhanandam et al., 2013; Reimer et al., 2014; Tan et al., 2014; McGinley et al., 2015a,b; Vinck et al., 2015). These substates exhibit rapid and spontaneous transitions, which reflect global fluctuation in the levels of arousal and movement, and have a major impact on sensory processing and behavioral performance. Another line of investigation has been focused on the local changes in cortical states during tasks that involve selective attention, studied in non-human primates (Desimone and Duncan, 1995; Fries et al., 2001; Cohen and Maunsell, 2009; Gregoriou et al., 2009; Mitchell et al., 2009; Chalk et al., 2010). Shifts in local cortical state during selective attention appear to have similar effects on sensory encoding to those elicited by the global state changes. Both selective attention and global arousal enhance the representation of sensory information by increasing evoked firing rate, decreasing response variability and reducing correlated noise (Luck et al., 1997; Cohen and Maunsell, 2009; Mitchell et al., 2009; Reimer et al., 2014; Vinck et al., 2015).This suggests that the effects of both global and local state transitions might be mediated by overlapping mechanisms.
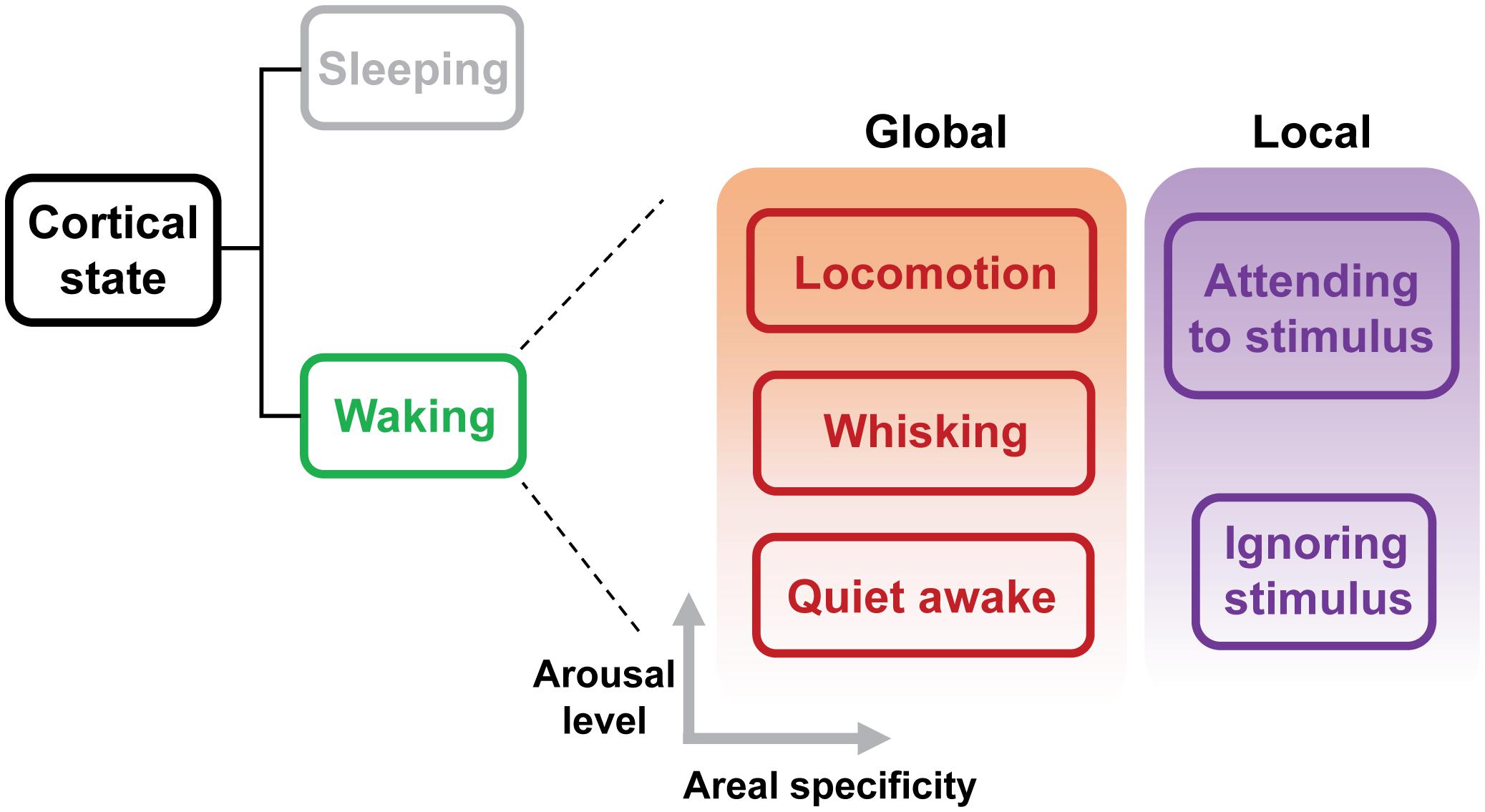
FIGURE 1. Global and local substates of wakefulness. Sleep and wakefulness are the two fundamental cortical states. Recent studies have revealed that, even during wakefulness, the cortical state exhibits transitions between different sub-states characterized as different levels of arousal (Gervasoni et al., 2004; Harris and Thiele, 2011; McGinley et al., 2015b). Substate transitions may occur globally or within specific areas depending on behavioral demands. A hallmark of a globally aroused state such as locomotion is cortical desynchronization. Selective attention leads to a local state transition, which manifests as the desynchronization in cortical areas responsive to the attended stimuli. Therefore, both global and local state transitions appear to use similar mechanisms. Advances in recording techniques and behavioral approaches will provide an increasingly more elaborate taxonomy of cortical state.
How these cortical states are orchestrated to modulate neural and behavioral plasticity during learning is a fundamental question in neuroscience. Recent findings of functional MRI (fMRI) studies in human subjects show that cortical state, measured as the resting state network activity of the brain has a significant impact on the performance in perceptual learning (PL) (Baldassarre et al., 2012; Freyer et al., 2013). Here, we review how cortical state has been defined in the latest studies, highlight ways in which cortical state may influence PL and vice-versa, and discuss an integrative framework that could guide future investigation.
Definitions of Cortical State in the Awake Brain
Cortical state has been defined operationally on different spatial scales (from a specific area to the entire cortex) and temporal scales (tens of milliseconds to seconds) even in the awake brain. At the neuronal level, shifts in cortical state manifest as a transition between up (depolarized) and down (hyperpolarized) phases of membrane potential, which arises from fluctuations in the synaptic input. Cortical state has also been defined based on population-level fluctuations in synchrony or the LFP power during spontaneous activity (Harris and Thiele, 2011). Simultaneous measurements of membrane potential, LFP and behavioral state (e.g., movement, muscle tone, pupil diameter) in head-fixed mice have revealed that neuronal and brain-wide state fluctuations are generally correlated and that pupil dilation is indicative of desynchronized or “aroused” state (Reimer et al., 2014; McGinley et al., 2015a; Vinck et al., 2015). Cortical state monitored in these studies is associated with fluctuations in the global behavioral state such as arousal and locomotion. In studies that used ensemble spiking activity recording, cortical state has been defined based on fluctuations of the synchrony (Luczak et al., 2013; Pachitariu et al., 2015; Scholvinck et al., 2015) and the strength (Arandia-Romero et al., 2016; Engel et al., 2016; Beaman et al., 2017; Gutnisky et al., 2017) of population activity in a local cortical area. Attempts have been made to correlate trial-to-trial fluctuation of spontaneous and evoked population activity to sensory encoding and behavioral responses. This line of work shows that cortical state can exhibit rapid trial-to-trial shifts, modulate sensory encoding and impact perceptual behavior (Arandia-Romero et al., 2016; Engel et al., 2016; Beaman et al., 2017; Gutnisky et al., 2017). Despite the differences in experimental settings and ways in which cortical state was defined (e.g., synchrony-based vs. strength-based definitions), these studies agree on a general point: certain patterns of ongoing network activity reflecting a cortical state enhance sensory encoding, and transitions in cortical state correlate well with fluctuations in encoded sensory information and the behavioral report. Thus, cortical response is not exclusively determined by external stimulus but by the interaction between the sensory stimulus and internal state reflected in the spontaneous population activity. How does cortical state impact learning? Here, we will attempt to answer this question by focusing on PL, in which sensory cortex has been shown to play an important role (Caras and Sanes, 2017).
Cortical State and Perceptual Learning
Perceptual learning is a form of implicit learning and distinct from declarative learning that requires the use of the medial temporal lobe (Gilbert and Sigman, 2007; Watanabe and Sasaki, 2015). PL is characterized by a gradual, long-term improvement in perceptual judgement. Neural plasticity mechanisms associated with PL are thought to be specific to the trained stimulus and task. However, recent studies demonstrated that perceptual improvement in one task can transfer to other tasks (Xiao et al., 2008; Zhang et al., 2010). Therefore it is still under debate whether and under what circumstances PL generalizes to other tasks and sensory features. Many studies have reported cortical changes associated with PL, including prominent map expansion, increases in receptive field size, sharpening and amplification of tuning curves to the trained feature and reduction of correlated variability among neurons in early sensory cortex (Recanzone et al., 1993; Schoups et al., 2001; Ni et al., 2018). However, these changes are not always observed in early sensory cortex (Ghose et al., 2002) but rather in the intermediate sensory cortex or the sensory-motor area (Yang and Maunsell, 2004; Law and Gold, 2008) and do not necessarily correlate with performance improvement. Moreover, the involvement of early sensory cortex in itself does not explain the context-specificity of PL. Performance improvement within one task does not transfer to another task that uses the same set of stimuli in a different context, suggesting that the outcome of PL is under “top-down” influence (Ahissar and Hochstein, 1993; Gilbert and Sigman, 2007).
Neural mechanisms that underlie behavioral improvement associated with PL remain undefined. Depending on the task design, species and sensory modality, different mechanisms have been put forward. In tasks that require discrimination or detection of primary features of stimulus, the performance improvement has been associated with enhanced representation of the relevant features. On the other hand, tasks that are based on emergent properties of cortex such as context-specific sensory coding, remarkable changes in the top-down influence have been observed while the neural plasticity at the level of individual sensory neurons appeared to be modest (Crist et al., 2001). Another potential mechanism for the neural plasticity during PL involves reweighting connections between sensory and decision cortical areas via trial-by-trial feedback signals (Dosher and Lu, 1998; Seitz and Watanabe, 2005; Law and Gold, 2009). This leads to an improved “read-out” of sensory evidence by a decision unit and a reduced internal noise (Dosher and Lu, 1998). A similar model applied to feedforward connections between thalamus and early sensory cortex also captures behavioral improvements during PL (Bejjanki et al., 2011). Therefore cortical state may impact PL via enhancing the representation of task-relevant sensory input, improving context-dependent recruitment of relevant circuits and reducing internal noise. In the following section, we will discuss the impact of cortical state on sensory encoding as a potential mechanism for PL. Importantly cortical state is subject to various forms of modulation. We will review the evidence supporting that top-down feedback signals from “higher” cortical areas modulate cortical state and how such modulation might occur during PL (Gilbert and Sigman, 2007; Law and Gold, 2009).
The Impact of Cortical State on Stimulus Encoding
An intuitive way by which cortical state might affect PL is through modulating neural activity in the cortical area that encodes task-relevant features. Typical PL involves training subjects to discriminate perceptually demanding stimuli that are repeatedly presented for extended periods of time. Neuronal responses to repetitive sensory stimuli are not static but dynamically modulated by the ongoing spontaneous activity. Recent studies using population recording techniques have revealed that spontaneous activity is structured in spatial and temporal domains. The amplitude and variability of sensory-evoked responses are correlated with the intrinsic response state in the sensory cortex to such an extent that the evoked responses can be predicted from the pre-stimulus on-going activity (Curto et al., 2009; Scholvinck et al., 2015). Stimulus statistics is another factor that affects the internal cortical state during the presentation of a sensory input. For example, unexpected brief stimuli evoke robust responses regardless of cortical states, although the response amplitude is smaller in desynchronized states (Castro-Alamancos, 2004; Otazu et al., 2009). Responses to stimuli with high repetition frequencies show a more complex state-dependence. While the response to the first stimulus in a train with a high repetition frequency is elevated in synchronized states compared to desynchronized states, responses adapt more strongly in a synchronized state (Castro-Alamancos, 2004). These observations suggest that sensory stimuli presented with repetition may lead to enhanced neuronal representation in a desynchronized state. Across modalities, temporally extended stimuli are more faithfully represented in desynchronized than synchronized states, which might be beneficial for animals with behavioral needs to analyze fine details of continuous sensory inputs (Goard and Dan, 2009; Marguet and Harris, 2011).
State-dependent improvement in sensory encoding can be observed at the population level. Network-level changes have been measured recently using population recording techniques, which demonstrated that the amount of sensory information encoded in population activity was significantly higher in desynchronized states (Pachitariu et al., 2015; Beaman et al., 2017). A potential explanation for the improved population decoding in these studies is a decrease in the correlated variability among neuronal population during desynchronized state. In vivo measurements in different cortical areas show that the pairwise correlated variability is on average about 0.1–0.2, although it can vary significantly depending on internal cortical state (Cohen and Kohn, 2011; Ecker et al., 2014). Recurrent network models of anatomically inspired connectivity yield a similar value (Shadlen et al., 1996). This correlated variability severely limits the amount of information encoded by neuronal population as it cannot be removed by simply pooling responses across neurons. Although the relationship between cortical state and the correlated variability is still under debate, many studies have observed that the correlated variability is reduced in a desynchronized state, which would lead to improved population coding (Pachitariu et al., 2015; Scholvinck et al., 2015; Gutnisky et al., 2017). An elegant study has revealed that the reduction in correlated variability is a common mechanism for improved performance during PL and attention, although these processes occur on different time scales (Ni et al., 2018).
Circuit mechanisms by which state-dependent changes in pairwise correlations are implemented during PL remain poorly understood. Globally desynchronized states, such as arousal and locomotion, enhance sensory-evoked responses and reduce the correlated noise among neurons, leading to increases in encoded sensory information. However, such global states do not explain the neural changes that occur locally within relevant brain areas during some PL tasks. Moreover, it is unclear whether desynchronization leads directly to decorrelation, because the relationship between spiking synchrony and the correlated variability is not straightforward. It is possible to have a decorrelated cortical network with two highly synchronized groups of neurons if the two populations cancel each other out, as would be in the case of populations of excitatory and inhibitory neurons (Harris and Thiele, 2011). Indeed, theoretical models support that inhibition might play a critical role in active decorrelation of cortical neurons (Renart et al., 2010; Tetzlaff et al., 2012; Kanashiro et al., 2017). Long-range cortico-cortical and neuromodulatory projections have been shown to provide synaptic inputs to local inhibitory interneurons in target sensory cortical areas (Letzkus et al., 2011; Lee et al., 2013; Pi et al., 2013; Zhang et al., 2014). These interneurons are likely to be recruited in PL, and may serve to mediate state-dependent modulation of neuronal correlations during this learning process. Accumulating experimental evidence supports the roles of the interneurons in different forms of learning. Whether inhibitory interneurons are indeed important for decorrelation of cortical network over the course of PL is an important avenue for future investigation.
Modulation of Cortical State by Perceptual Learning
As we explore our surroundings, we are constantly bombarded with sensory stimuli. Depending on the behavioral context and outcome, some sensory stimuli are filtered out while others are registered for further processing, suggesting that cortical state is not static but dynamically modulated by ongoing behavioral demands. While, as discussed above, certain cortical states have been shown to enhance sensory encoding and facilitate PL, it is important to note that PL in turn modulates cortical state in a context-specific manner. How does the brain assess behavioral context and update cortical state accordingly? To understand the context-dependent modulation of cortical state during PL, it would be necessary to determine at what time point in a trial such modulation occurs in relation to the switching of the context. In a pioneering study, animals were trained for two different visual PL tasks that involved the same set of stimuli (Li et al., 2004). It is clear from this work that when cued to the task to be performed before the stimulus onset, the difference in the response arises at the outset of the neurons’ responses, indicating that the top-down information conveying the task instruction sets the cortex to a state that then enables analysis of the stimulus in a task-relevant fashion. In a recent study, the cortical state defined by the population activity during a delay period prior to the presentation of the test stimulus, switches to a “low activity” mode, and this shift in state showed trial-by-trial correlation with encoded sensory information and behavioral performance in an orientation discrimination task (Gutnisky et al., 2017). These studies suggest that signals carrying information about behavioral outcome propagate back to early sensory cortex, and that this “top-down” influence (e.g., expectation or prediction) sets cortical state in preparation for the ongoing behavioral demand. Studies using two-photon calcium imaging have revealed diverse sources of long-range projections to early sensory cortex, including those from higher cortical areas, higher thalamic nuclei and amygdala, which carry information about context, behavioral outcome and physiologically relevant sensory cues (Makino and Komiyama, 2015; Burgess et al., 2016; Kwon et al., 2016; Roth et al., 2016).
A widely studied instance of top-down influence is spatial attention. In the majority of PL tasks that involve active engagement of subjects, attention is required for behavioral improvement. It is generally agreed that attention enhances performance in perceptual tasks by increasing the neuronal gain and reducing the correlated noise shared among similarly tuned neurons, thereby leading to improved sensory coding and enhanced performance (Cohen and Maunsell, 2009; Rabinowitz et al., 2015). It has also been reported that attention modulates network oscillation. Attention decreases low frequency fluctuation while increasing the fluctuation at gamma frequencies (25–100 Hz) in task-relevant brain areas, reflecting local cortical desynchronization (Fries et al., 2001; Gregoriou et al., 2009; Mitchell et al., 2009). A recent study investigated changes in synchronous spiking in a visual cortex during a visual task that required spatial attention (Engel et al., 2016). By modeling the transition between vigorous and faint spiking states, this study demonstrated that the impacts of spatial attention on firing rate and the transition dynamics can be separated. Importantly, the local modulation of the state transition predicted the behavioral performance in the task.
These observations suggest that various forms of the top-down influence set cortical state into a mode that enables efficient sensory processing and perceptual improvement through desynchronizing cortical network and reducing the correlated variability. As a potential mechanism of top-down influence, enhanced selective attention during PL modulates cortical state such that population coding of relevant sensory input is improved, which leads to behavioral improvements (Byers and Serences, 2014; Ni et al., 2018). In addition to this “permissive” role, it is possible that some cortical states may play a causal role by actively mediating or driving the neural plasticity underlying PL. Theoretical works suggest that shifts in network oscillations such as the increase in gamma power might organize neurons into a cell assembly that is capable of registering and evaluating sensory inputs during an ongoing task (Borgers et al., 2008). The increase in gamma power has been shown to correlate with enhanced coupling between cortical areas during attention (Gregoriou et al., 2009). Therefore, it still remains to be determined whether cortical state plays a direct causal role or merely allows performance improvements during PL. In order to establish the necessity of a certain cortical state for behavioral outcome of PL, one needs to manipulate cortical state in behaving subjects and test its effect on sensory encoding and performance during PL. This is an important area of ongoing and future investigation.
Future Outlook
A tremendous progress has been made in our understanding of cortical state and how cortical state and PL impact each other. However, there are important gaps to be filled. While recent studies have revealed fine spatiotemporal structures in cortical state, it remains poorly understood how neurons of different cell-types orchestrate their activity during shifts in cortical state. As discussed above, inhibitory interneurons are thought to be important effectors during cortical state changes. In addition, excitatory neurons in the neocortex have specific projection targets. How are neurons of different cell-types, defined by neurotransmitter and projection patterns, involved in cortical state changes? To fill this important gap, experimental efforts need to be made to measure cell type-specific activity patterns during cortical state transitions.
Many labs have demonstrated a tight correlation between cortical state and sensory processing during various perceptual tasks. More attempts need to be made to establish a causal relationship between changes in cortical state and behavioral changes during PL. Optogenetic manipulation to add or suppress activity during a certain cortical state in behaving animals would be a powerful approach to test the causality of cortical state for sensory processing and perception on a trial-by-trial basis.
It is important to note that PL involves plasticity in several different brain areas. Although it is debatable which brain area(s) causes perceptual improvement, interaction between different cortical areas is likely to be critical for PL. Therefore, studies examining the impact of cortical state on PL and vice versa should consider the long-range pathways that connect different cortical areas. To this end, genetic tools for directly manipulating specific long-range pathways may be combined with recording of cortical state during perceptual tasks. Advances in multi-areal, single-neuron imaging in behaving animals would allow us to define how cortical state transitions are coordinated across brain areas and whether a specific coordination is important for PL.
Author Contributions
SK wrote the paper.
Funding
Support for this work was provided by the Simons Foundation Autism Research Initiative Bridge to Independence Award.
Conflict of Interest Statement
The author declares that the research was conducted in the absence of any commercial or financial relationships that could be construed as a potential conflict of interest.
References
Ahissar, M., and Hochstein, S. (1993). Attentional control of early perceptual learning. Proc. Natl. Acad. Sci. U.S.A. 90, 5718–5722. doi: 10.1073/pnas.90.12.5718
Arandia-Romero, I., Tanabe, S., Drugowitsch, J., Kohn, A., and Moreno-Bote, R. (2016). Multiplicative and additive modulation of neuronal tuning with population activity affects encoded information. Neuron 89, 1305–1316. doi: 10.1016/j.neuron.2016.01.044
Baldassarre, A., Lewis, C. M., Committeri, G., Snyder, A. Z., Romani, G. L., and Corbetta, M. (2012). Individual variability in functional connectivity predicts performance of a perceptual task. Proc. Natl. Acad. Sci. U.S.A. 109, 3516–3521. doi: 10.1073/pnas.1113148109
Beaman, C. B., Eagleman, S. L., and Dragoi, V. (2017). Sensory coding accuracy and perceptual performance are improved during the desynchronized cortical state. Nat. Commun. 8:1308. doi: 10.1038/s41467-017-01030-4
Bejjanki, V. R., Beck, J. M., Lu, Z. L., and Pouget, A. (2011). Perceptual learning as improved probabilistic inference in early sensory areas. Nat. Neurosci. 14, 642–648. doi: 10.1038/nn.2796
Borgers, C., Epstein, S., and Kopell, N. J. (2008). Gamma oscillations mediate stimulus competition and attentional selection in a cortical network model. Proc. Natl. Acad. Sci. U.S.A. 105, 18023–18028. doi: 10.1073/pnas.0809511105
Burgess, C. R., Ramesh, R. N., Sugden, A. U., Levandowski, K. M., Minnig, M. A., Fenselau, H., et al. (2016). Hunger-dependent enhancement of food cue responses in mouse postrhinal cortex and lateral amygdala. Neuron 91, 1154–1169. doi: 10.1016/j.neuron.2016.07.032
Byers, A., and Serences, J. T. (2014). Enhanced attentional gain as a mechanism for generalized perceptual learning in human visual cortex. J. Neurophysiol. 112, 1217–1227. doi: 10.1152/jn.00353.2014
Caras, M. L., and Sanes, D. H. (2017). Top-down modulation of sensory cortex gates perceptual learning. Proc. Natl. Acad. Sci. U.S.A. 114, 9972–9977. doi: 10.1073/pnas.1712305114
Castro-Alamancos, M. A. (2004). Absence of rapid sensory adaptation in neocortex during information processing states. Neuron 41, 455–464. doi: 10.1016/S0896-6273(03)00853-5
Chalk, M., Herrero, J. L., Gieselmann, M. A., Delicato, L. S., Gotthardt, S., and Thiele, A. (2010). Attention reduces stimulus-driven gamma frequency oscillations and spike field coherence in V1. Neuron 66, 114–125. doi: 10.1016/j.neuron.2010.03.013
Cohen, M. R., and Kohn, A. (2011). Measuring and interpreting neuronal correlations. Nat. Neurosci. 14, 811–819. doi: 10.1038/nn.2842
Cohen, M. R., and Maunsell, J. H. (2009). Attention improves performance primarily by reducing interneuronal correlations. Nat. Neurosci. 12, 1594–1600. doi: 10.1038/nn.2439
Crist, R. E., Li, W., and Gilbert, C. D. (2001). Learning to see: experience and attention in primary visual cortex. Nat. Neurosci. 4, 519–525. doi: 10.1038/87470
Curto, C., Sakata, S., Marguet, S., Itskov, V., and Harris, K. D. (2009). A simple model of cortical dynamics explains variability and state dependence of sensory responses in urethane-anesthetized auditory cortex. J. Neurosci. 29, 10600–10612. doi: 10.1523/JNEUROSCI.2053-09.2009
Desimone, R., and Duncan, J. (1995). Neural mechanisms of selective visual attention. Annu. Rev. Neurosci. 18, 193–222. doi: 10.1146/annurev.ne.18.030195.001205
Dosher, B. A., and Lu, Z. L. (1998). Perceptual learning reflects external noise filtering and internal noise reduction through channel reweighting. Proc. Natl. Acad. Sci. U.S.A. 95, 13988–13993. doi: 10.1073/pnas.95.23.13988
Ecker, A. S., Berens, P., Cotton, R. J., Subramaniyan, M., Denfield, G. H., Cadwell, C. R., et al. (2014). State dependence of noise correlations in macaque primary visual cortex. Neuron 82, 235–248. doi: 10.1016/j.neuron.2014.02.006
Engel, T. A., Steinmetz, N. A., Gieselmann, M. A., Thiele, A., Moore, T., and Boahen, K. (2016). Selective modulation of cortical state during spatial attention. Science 354, 1140–1144. doi: 10.1126/science.aag1420
Freyer, F., Becker, R., Dinse, H. R., and Ritter, P. (2013). State-dependent perceptual learning. J. Neurosci. 33, 2900–2907. doi: 10.1523/JNEUROSCI.4039-12.2013
Fries, P., Reynolds, J. H., Rorie, A. E., and Desimone, R. (2001). Modulation of oscillatory neuronal synchronization by selective visual attention. Science 291, 1560–1563. doi: 10.1126/science.291.5508.1560
Gervasoni, D., Lin, S. C., Ribeiro, S., Soares, E. S., Pantoja, J., and Nicolelis, M. A. (2004). Global forebrain dynamics predict rat behavioral states and their transitions. J. Neurosci. 24, 11137–11147. doi: 10.1523/JNEUROSCI.3524-04.2004
Ghose, G. M., Yang, T., and Maunsell, J. H. (2002). Physiological correlates of perceptual learning in monkey V1 and V2. J. Neurophysiol. 87, 1867–1888. doi: 10.1152/jn.00690.2001
Gilbert, C. D., and Sigman, M. (2007). Brain states: top-down influences in sensory processing. Neuron 54, 677–696. doi: 10.1016/j.neuron.2007.05.019
Goard, M., and Dan, Y. (2009). Basal forebrain activation enhances cortical coding of natural scenes. Nat. Neurosci. 12, 1444–1449. doi: 10.1038/nn.2402
Gregoriou, G. G., Gotts, S. J., Zhou, H., and Desimone, R. (2009). High-frequency, long-range coupling between prefrontal and visual cortex during attention. Science 324, 1207–1210. doi: 10.1126/science.1171402
Gutnisky, D. A., Beaman, C., Lew, S. E., and Dragoi, V. (2017). Cortical response states for enhanced sensory discrimination. eLife 6:e29226. doi: 10.7554/eLife.29226
Harris, K. D., and Thiele, A. (2011). Cortical state and attention. Nat. Rev. Neurosci. 12, 509–523. doi: 10.1038/nrn3084
Kanashiro, T., Ocker, G. K., Cohen, M. R., and Doiron, B. (2017). Attentional modulation of neuronal variability in circuit models of cortex. eLife 6:e23978. doi: 10.7554/eLife.23978
Kwon, S. E., Yang, H., Minamisawa, G., and O’Connor, D. H. (2016). Sensory and decision-related activity propagate in a cortical feedback loop during touch perception. Nat. Neurosci. 19, 1243–1249. doi: 10.1038/nn.4356
Law, C. T., and Gold, J. I. (2008). Neural correlates of perceptual learning in a sensory-motor, but not a sensory, cortical area. Nat. Neurosci. 11, 505–513. doi: 10.1038/nn2070
Law, C. T., and Gold, J. I. (2009). Reinforcement learning can account for associative and perceptual learning on a visual-decision task. Nat. Neurosci. 12, 655–663. doi: 10.1038/nn.2304
Lee, S., Kruglikov, I., Huang, Z. J., Fishell, G., and Rudy, B. (2013). A disinhibitory circuit mediates motor integration in the somatosensory cortex. Nat. Neurosci. 16, 1662–1670. doi: 10.1038/nn.3544
Letzkus, J. J., Wolff, S. B., Meyer, E. M., Tovote, P., Courtin, J., Herry, C., et al. (2011). A disinhibitory microcircuit for associative fear learning in the auditory cortex. Nature 480, 331–335. doi: 10.1038/nature10674
Li, W., Piech, V., and Gilbert, C. D. (2004). Perceptual learning and top-down influences in primary visual cortex. Nat. Neurosci. 7, 651–657. doi: 10.1038/nn1255
Luck, S. J., Chelazzi, L., Hillyard, S. A., and Desimone, R. (1997). Neural mechanisms of spatial selective attention in areas V1, V2, and V4 of macaque visual cortex. J. Neurophysiol. 77, 24–42. doi: 10.1152/jn.1997.77.1.24
Luczak, A., Bartho, P., and Harris, K. D. (2013). Gating of sensory input by spontaneous cortical activity. J. Neurosci. 33, 1684–1695. doi: 10.1523/JNEUROSCI.2928-12.2013
Makino, H., and Komiyama, T. (2015). Learning enhances the relative impact of top-down processing in the visual cortex. Nat. Neurosci. 18, 1116–1122. doi: 10.1038/nn.4061
Marguet, S. L., and Harris, K. D. (2011). State-dependent representation of amplitude-modulated noise stimuli in rat auditory cortex. J. Neurosci. 31, 6414–6420. doi: 10.1523/JNEUROSCI.5773-10.2011
McGinley, M. J., David, S. V., and McCormick, D. A. (2015a). Cortical membrane potential signature of optimal states for sensory signal detection. Neuron 87, 179–192. doi: 10.1016/j.neuron.2015.05.038
McGinley, M. J., Vinck, M., Reimer, J., Batista-Brito, R., Zagha, E., Cadwell, C. R., et al. (2015b). Waking state: rapid variations modulate neural and behavioral responses. Neuron 87, 1143–1161. doi: 10.1016/j.neuron.2015.09.012
Mitchell, J. F., Sundberg, K. A., and Reynolds, J. H. (2009). Spatial attention decorrelates intrinsic activity fluctuations in macaque area V4. Neuron 63, 879–888. doi: 10.1016/j.neuron.2009.09.013
Ni, A. M., Ruff, D. A., Alberts, J. J., Symmonds, J., and Cohen, M. R. (2018). Learning and attention reveal a general relationship between population activity and behavior. Science 359, 463–465. doi: 10.1126/science.aao0284
Otazu, G. H., Tai, L. H., Yang, Y., and Zador, A. M. (2009). Engaging in an auditory task suppresses responses in auditory cortex. Nat. Neurosci. 12, 646–654. doi: 10.1038/nn.2306
Pachitariu, M., Lyamzin, D. R., Sahani, M., and Lesica, N. A. (2015). State-dependent population coding in primary auditory cortex. J. Neurosci. 35, 2058–2073. doi: 10.1523/JNEUROSCI.3318-14.2015
Pi, H. J., Hangya, B., Kvitsiani, D., Sanders, J. I., Huang, Z. J., and Kepecs, A. (2013). Cortical interneurons that specialize in disinhibitory control. Nature 503, 521–524. doi: 10.1038/nature12676
Poulet, J. F., and Petersen, C. C. (2008). Internal brain state regulates membrane potential synchrony in barrel cortex of behaving mice. Nature 454, 881–885. doi: 10.1038/nature07150
Rabinowitz, N. C., Goris, R. L., Cohen, M., and Simoncelli, E. P. (2015). Attention stabilizes the shared gain of V4 populations. eLife 4:e08998. doi: 10.7554/eLife.08998
Recanzone, G. H., Schreiner, C. E., and Merzenich, M. M. (1993). Plasticity in the frequency representation of primary auditory cortex following discrimination training in adult owl monkeys. J. Neurosci. 13, 87–103. doi: 10.1523/JNEUROSCI.13-01-00087.1993
Reimer, J., Froudarakis, E., Cadwell, C. R., Yatsenko, D., Denfield, G. H., and Tolias, A. S. (2014). Pupil fluctuations track fast switching of cortical states during quiet wakefulness. Neuron 84, 355–362. doi: 10.1016/j.neuron.2014.09.033
Renart, A., de la Rocha, J., Bartho, P., Hollender, L., Parga, N., Reyes, A., et al. (2010). The asynchronous state in cortical circuits. Science 327, 587–590. doi: 10.1126/science.1179850
Roth, M. M., Dahmen, J. C., Muir, D. R., Imhof, F., Martini, F. J., and Hofer, S. B. (2016). Thalamic nuclei convey diverse contextual information to layer 1 of visual cortex. Nat. Neurosci. 19, 299–307. doi: 10.1038/nn.4197
Sachidhanandam, S., Sreenivasan, V., Kyriakatos, A., Kremer, Y., and Petersen, C. C. (2013). Membrane potential correlates of sensory perception in mouse barrel cortex. Nat. Neurosci. 16, 1671–1677. doi: 10.1038/nn.3532
Scholvinck, M. L., Saleem, A. B., Benucci, A., Harris, K. D., and Carandini, M. (2015). Cortical state determines global variability and correlations in visual cortex. J. Neurosci. 35, 170–178. doi: 10.1523/JNEUROSCI.4994-13.2015
Schoups, A., Vogels, R., Qian, N., and Orban, G. (2001). Practising orientation identification improves orientation coding in V1 neurons. Nature 412, 549–553. doi: 10.1038/35087601
Seitz, A., and Watanabe, T. (2005). A unified model for perceptual learning. Trends Cogn. Sci. 9, 329–334. doi: 10.1016/j.tics.2005.05.010
Shadlen, M. N., Britten, K. H., Newsome, W. T., and Movshon, J. A. (1996). A computational analysis of the relationship between neuronal and behavioral responses to visual motion. J. Neurosci. 16, 1486–1510. doi: 10.1523/JNEUROSCI.16-04-01486.1996
Steriade, M., Timofeev, I., and Grenier, F. (2001). Natural waking and sleep states: a view from inside neocortical neurons. J. Neurophysiol. 85, 1969–1985. doi: 10.1152/jn.2001.85.5.1969
Tan, A. Y., Chen, Y., Scholl, B., Seidemann, E., and Priebe, N. J. (2014). Sensory stimulation shifts visual cortex from synchronous to asynchronous states. Nature 509, 226–229. doi: 10.1038/nature13159
Tetzlaff, T., Helias, M., Einevoll, G. T., and Diesmann, M. (2012). Decorrelation of neural-network activity by inhibitory feedback. PLoS Comput. Biol. 8:e1002596. doi: 10.1371/journal.pcbi.1002596
Vinck, M., Batista-Brito, R., Knoblich, U., and Cardin, J. A. (2015). Arousal and locomotion make distinct contributions to cortical activity patterns and visual encoding. Neuron 86, 740–754. doi: 10.1016/j.neuron.2015.03.028
Watanabe, T., and Sasaki, Y. (2015). Perceptual learning: toward a comprehensive theory. Annu. Rev. Psychol. 66, 197–221. doi: 10.1146/annurev-psych-010814-015214
Xiao, L. Q., Zhang, J. Y., Wang, R., Klein, S. A., Levi, D. M., and Yu, C. (2008). Complete transfer of perceptual learning across retinal locations enabled by double training. Curr. Biol. 18, 1922–1926. doi: 10.1016/j.cub.2008.10.030
Yang, T., and Maunsell, J. H. (2004). The effect of perceptual learning on neuronal responses in monkey visual area V4. J. Neurosci. 24, 1617–1626. doi: 10.1523/JNEUROSCI.4442-03.2004
Zhang, J. Y., Zhang, G. L., Xiao, L. Q., Klein, S. A., Levi, D. M., and Yu, C. (2010). Rule-based learning explains visual perceptual learning and its specificity and transfer. J. Neurosci. 30, 12323–12328. doi: 10.1523/JNEUROSCI.0704-10.2010
Keywords: brain state, population activity, perceptual learning, attention, sensory processing
Citation: Kwon SE (2018) The Interplay Between Cortical State and Perceptual Learning: A Focused Review. Front. Syst. Neurosci. 12:47. doi: 10.3389/fnsys.2018.00047
Received: 31 May 2018; Accepted: 24 September 2018;
Published: 09 October 2018.
Edited by:
Keith B. Hengen, Washington University in St. Louis, United StatesReviewed by:
Victor de Lafuente, Universidad Nacional Autónoma de México, MexicoMichael A. Silver, University of California, Berkeley, United States
Copyright © 2018 Kwon. This is an open-access article distributed under the terms of the Creative Commons Attribution License (CC BY). The use, distribution or reproduction in other forums is permitted, provided the original author(s) and the copyright owner(s) are credited and that the original publication in this journal is cited, in accordance with accepted academic practice. No use, distribution or reproduction is permitted which does not comply with these terms.
*Correspondence: Sung Eun Kwon, c2Fta3dvbkB1bWljaC5lZHU=