- Florey Institute of Neuroscience and Mental Health, University of Melbourne, Parkville, VIC, Australia
The cortex is crucial for many behaviors, ranging from sensory-based behaviors to working memory and social behaviors. To gain an in-depth understanding of the contribution to these behaviors, cellular and sub-cellular recordings from both individual and populations of cortical neurons are vital. However, techniques allowing such recordings, such as two-photon imaging and whole-cell electrophysiology, require absolute stability of the head, a requirement not often fulfilled in freely moving animals. Here, we review and compare behavioral paradigms that have been developed and adapted for the head-fixed preparation, which together offer the needed stability for live recordings of neural activity in behaving animals. We also review how the head-fixed preparation has been used to explore the function of primary sensory cortices, posterior parietal cortex (PPC) and anterior lateral motor (ALM) cortex in sensory-based behavioral tasks, while also discussing the considerations of performing such recordings. Overall, this review highlights the head-fixed preparation as allowing in-depth investigation into the neural activity underlying behaviors by providing highly controllable settings for precise stimuli presentation which can be combined with behavioral paradigms ranging from simple sensory detection tasks to complex, cross-modal, memory-guided decision-making tasks.
Introduction
Our behavior, the way one acts or conducts oneself, is key to survival. Animals must behave in an appropriate manner to successfully navigate and interact with their surroundings. This involves billions of neurons working together to formulate a cohesive motor output. Therefore, due simply to the sheer numbers involved, understanding the neural basis of behavior is complicated. It is further confounded by the diversity of behaviors, ranging from perception to social interactions to navigation, which involve different classes of neurons, neural interactions as well as brain regions. The activity of individual neurons within the cortex are often highlighted as being crucial for many behaviors, such as sensory-based (Xu et al., 2012; Takahashi et al., 2016) and social behaviors (Rao et al., 2014; Lenschow and Brecht, 2015), as well as anticipation (Erlich et al., 2011; Guo et al., 2014b) and decision making (Harvey et al., 2012). However, it is difficult to clearly state the overall role of the cortex, or a class of cortical neurons, during behavior as the underlying neural activity is dependent on many factors, such as changes in feedforward and feedback information, as well as overall brain state (Poulet and Petersen, 2008).
To understand the contribution of individual neurons during behavior, recordings must be performed from an individual or population of identified neurons, during an active behavioral task. Recording action potential firing in a population of neurons in freely-behaving rodents can be achieved using extracellular probes with a high density of recording sites (Buzsáki, 2004). However, extracellular recordings are fraught with analysis considerations (Buzsáki et al., 2012; Higley, 2012) and cannot typically measure sub-threshold and sub-cellular activity (although see Suzuki and Larkum, 2017). The mere act of behaving makes recording the activity of individual neurons challenging, as most techniques used to measure the activity of individual neurons, such as calcium imaging and patch-clamp electrophysiology, requires absolute stability of the recording preparation. Although miniaturized equipment can record sub-cellular calcium (Helmchen et al., 2001; Ghosh et al., 2011; Cai et al., 2016) and voltage (Burgalossi et al., 2011; Tang et al., 2014) activity from single neurons in animals as they physically move through an environment, these are highly specialized and difficult to implement. Therefore, most studies delving into the sub-cellular neural activity associated with behavior are performed in the head-fixed condition. Unlike the freely moving animal models, the head-fixed preparation allows for highly controllable settings, such as stimulus presentation and motor output. Whether it requires precise stimuli for studying perception, discrimination, decision making or other cognitive tasks, the head-fixed preparation provides the opportunity to precisely control the stimulus delivered to the animal. Head-fixed behavioral models also typically provide high repeatability and employment of standardized techniques (Guo et al., 2014a). This review article brings together studies investigating the neural activity during various behaviors developed for head-fixed preparation. Here, we focus primarily on the role of the cortex in sensory-based behaviors. Historically, research into the role of the cortex during sensory perception and decision making was typically performed in primates (for review see Parker and Newsome, 1998), however, due to recent advances in molecular and optical techniques, rodents are now a crucial animal model and will be the focus of this review.
Behavioral Paradigms
Since there is no one-fits-all behavioral model or task that will highlight the role of all brain regions, it is crucial to consider which model or task will be the most appropriate to engage a particular brain region and how to pair this with an appropriate recording technique. In the head-fixed preparation, the stimulus typically needs to be brought to the animal, as opposed to the animal moving to the stimulus in the freely moving scenario. Therefore, various behavioral tasks have been specifically developed for the head-fixed preparation. These tasks range in complexity, from presenting specific sensory stimuli to the animals to reconstructing an entire sensory environment.
Go/NoGo
Perhaps the easiest way to explore perception and decision making is by presenting one stimulus associated with a single response. This is the basis of the Go/NoGo task (Figure 1A), where an animal is presented with a stimulus and trained to report the perception of the stimulus, by either responding or withholding an action. This creates a Hit/Miss scenario for Go trials and a Correct Rejection/False Alarm scenario for NoGo trials. The Go/NoGo task can be used to address simple questions such as sensory perception and modulation (Petreanu et al., 2012; Xu et al., 2012; Takahashi et al., 2016; Micallef et al., 2017). In general, this paradigm takes only a few days to implement (1–3 days; Guo et al., 2014a; Micallef et al., 2017), however, a common weakness of the task, is a potential deviation in motivation leading to erroneous reporting. To overcome the decreased motivation towards obtaining a reward, the Go/NoGo task can be further advanced by applying the signal detection theory, as reviewed by Carandini and Churchland, (2013). By introducing a stimulus-based NoGo signal that is of same stimulus type but of different character (rather than the absence of a stimulus as a NoGo signal, commonly referred to as “Catch trials”), the animal has to discriminate between two stimuli, reporting only one of them, instead of simply reporting whenever the stimulus is detected. Tasks using this paradigm are often referred to as discrimination tasks (Figure 1B; Gilad et al., 2018; Helmchen et al., 2018) and in general take longer to learn than the simple Go/NoGo task (1–3 weeks; Guo et al., 2014a; Gilad et al., 2018; Helmchen et al., 2018). When water-deprived, animals are typically highly motivated to perform the task (Guo et al., 2014a). Since the instinctive reaction is to lick as soon as the stimuli has been detected, a short delay can be incorporated between stimulus and response period to temporally separate the different epochs (Guo et al., 2014a), however implementing a delay requires further training (Helmchen et al., 2018).
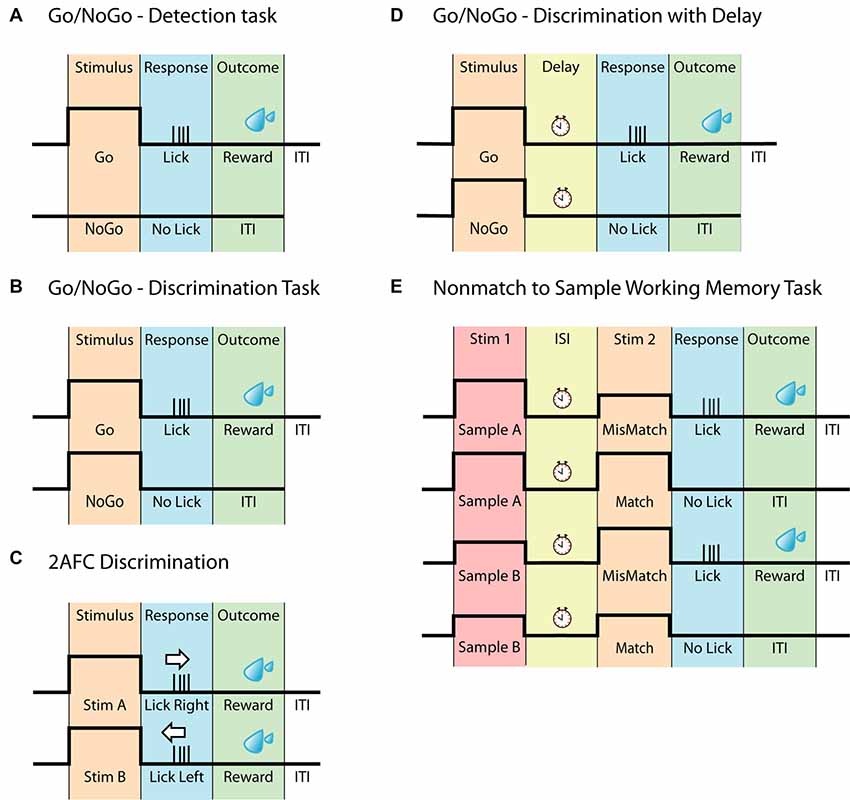
Figure 1. Head-fixed behavioral paradigms. (A) Schematic of the Go/NoGo task often referred to as a detection task. The animal should only respond upon detecting the stimulus. (B) Schematic of the Go/NoGo task involving discrimination of two stimuli. The animal should only respond to the Go-stimulus. This task is often referred to as a discrimination task. (C) Schematic of the two-alternative forced-choice (2AFC) task. Similar to (B), this task requires the discrimination between two different stimuli however, to eliminate pseudo responses, the animal must respond to both stimuli, for example by either licking left or licking right. (D) Schematic of the Go/NoGo discrimination task with delay. This task differs from (B) by having a delay separating the stimulus and the response. (E) Schematic of the Nonmatch to Sample Working memory task, based on the Go/NoGo discrimination paradigm. In this task, the animal is presented with two consecutive stimuli, separated by a delay. The animal must compare the two stimuli and respond accordingly.
Two Alternative Forced Choice
Similar to the Go/NoGo task, the two-alternative forced-choice (2AFC) task (Figure 1C) can be used to explore perceptual decision making. Here, an animal is presented with two stimuli however, instead of withholding a response to one of the stimuli (as in the Go/NoGo task), the animal now must report in both cases. This can be accomplished by either having the animal lick left or right (O’Connor et al., 2010a,b; Guo et al., 2014b; Peron et al., 2015; Zhong et al., 2019), turn a steering wheel (Burgess et al., 2017) or push a joystick (Estebanez et al., 2017; Morandell and Huber, 2017). The 2AFC task adds another level of clarity to behavioral outcomes, as the task design eliminates uncertainty behind NoGo responses, which may not be due to an inability to perform the task, but instead due to lack of motivation/engagement. The 2AFC task is however not completely immune to behavioral response misinterpretations, as animals can be biased toward one side of reporting (Guo et al., 2014a). As with all behaviors, the time it takes an animal to learn the 2AFC task depends on several factors such as sensory modality, type of stimulus, training methods and number of trials per session. However, due to its increased complexity compared to the simple discrimination tasks, learning the 2AFC task, in general, takes longer, ranging between 1 and 6 weeks (Mayrhofer et al., 2013; Guo et al., 2014a,b). Although it potentially can be considered a more robust behavior than the Go/NoGo tasks, the increased learning time can be a consideration for various chronic recording techniques. The 2AFC task is adaptable and can be changed according to particular experiments/questions, such as the two alternative unforced choices (2AUC) task which combines the 2AFC task with a “NoGo” signal, rewarding the animal for not responding (Burgess et al., 2017). The adaptability of the 2AFC task has led to many sensory systems being probed using this two-response behavior, including the vibrissal (Mayrhofer et al., 2013; Li et al., 2015; Peron et al., 2015), olfactory (Zariwala et al., 2013), visual (Busse et al., 2011; Burgess et al., 2017) and auditory (Znamenskiy and Zador, 2013; Wei et al., 2019) to name a few.
Working Memory Models
To probe the neural basis of higher cognitive functions such as working memory in the head-fixed preparation, behaviors have been developed that are based on the simpler behavioral tasks, such as the Go/NoGo and 2AFC. Working memory has been addressed using a delay-task in both monkeys (Quintana et al., 1988; Yajeya et al., 1988; Fuster, 2001) and rodents (Gilad et al., 2018; Inagaki et al., 2019). Here, animals are presented with a stimulus followed by a delay during which the animal needs to retain their decision until they are able to report their decision, often indicated by a response cue (Figure 1D). Of greater complexity are the comparison-based working memory tasks such as (but not limited to) Delayed Nonmatch to Sample (Figure 1E; Dudchenko, 2004), which engages working memory by having two sensory stimuli separated by an inter-stimulus delay, forcing the animal to retain a memory trace of the first stimulus to compare to the second stimulus. For the Nonmatch to Sample working memory task the animal has to compare the stimuli and report if the second stimulus is different (mismatch) to the sample or withhold its response if the two are identical (match). This working memory task builds on the principles of the Go/NoGo task and has for example been used for odor samples (Liu et al., 2014). The comparison-based working memory tasks can also build upon the principles of the 2AFC paradigm, as is the case for the pioneering Flutter Discrimination task developed for primates (Mountcastle et al., 1990; Hernández et al., 1997). This task has been successfully adapted to freely moving rats (Fassihi et al., 2014), but to our knowledge, it has not yet been successfully adapted to head-fixed rodents. For a review on other working memory tasks in rodents, see Dudchenko (2004).
Locomotion and Exploration
Many, if not all, behaviors require the integration of feedforward and feedback information from multiple senses. Take for example spatial exploration. Here, the visual system relies on the coordination of visual input with motor output to successfully explore and navigate through an environment (Randel et al., 2014; Heindorf et al., 2018). Probing sub-cellular neural activity during locomotion requires a behavioral platform that enables movement and associated stimuli in a head-fixed preparation. This can be achieved with a spherical (Dombeck et al., 2007; Harvey et al., 2012; Schmidt-Hieber and Häusser, 2013; Heindorf et al., 2018), circular (Hawrylycz et al., 2016; Schneider et al., 2018) or linear (Domnisoru et al., 2013; Lovett-Barron et al., 2014; Cichon and Gan, 2015; Bittner et al., 2017) track which allows precise location to be measured during locomotion in small laboratory animals. These platforms can be paired with a virtual reality-based environment. Combined with various sensory stimuli, platforms that enable locomotion during head-fixation are used to address various questions, including the neural activity underlying spatial navigation (Harvey et al., 2009; Dombeck et al., 2010; Domnisoru et al., 2013; Sheffield et al., 2017; Thurley and Ayaz, 2017), sensory processing (Niell and Stryker, 2010; Saleem et al., 2013; Makino and Komiyama, 2015; Sofroniew et al., 2015; Radvansky and Dombeck, 2018), arousal (Niell and Stryker, 2010; Polack et al., 2013; McGinley et al., 2015; Vinck et al., 2015; Shimaoka et al., 2018) and learning and memory (Lovett-Barron et al., 2014; Cichon and Gan, 2015). Behavioral platforms have also been developed that enable head-fixed mice to navigate through a physical environment (Kislin et al., 2014; Nashaat et al., 2016), as opposed to a virtual reality-based environment. Termed the air-track system (Nashaat et al., 2016), this is a lightweight maze floating on air, much like the spherical ball used in virtual reality set-ups, where the animal is head-fixed and uses its paws to navigate the maze. The air-track system offers both the stability required for most single-cell recording techniques as well as activation of different sensory modalities coupled to motor output in a “real-world” fashion.
Exploring Cortical Functions Using Head-Fixed Behavioral Models
The neocortex is enigmatic. It is a diverse structure with various regions dedicated to specific aspects of behaviors such as encoding sensory information and integrating this information with other sensory inputs. With the classical view of the role of the cortex during simple sensory-based behavior and encoding recently being challenged (Hong et al., 2018; Beltramo and Scanziani, 2019), deeper insight into the neural networks contributing to sensory perception and cognition is required. Below are summaries of the neural encoding of behavior in selected cortical regions that aim to disentangle their role in sensory-based behaviors (Figure 2).
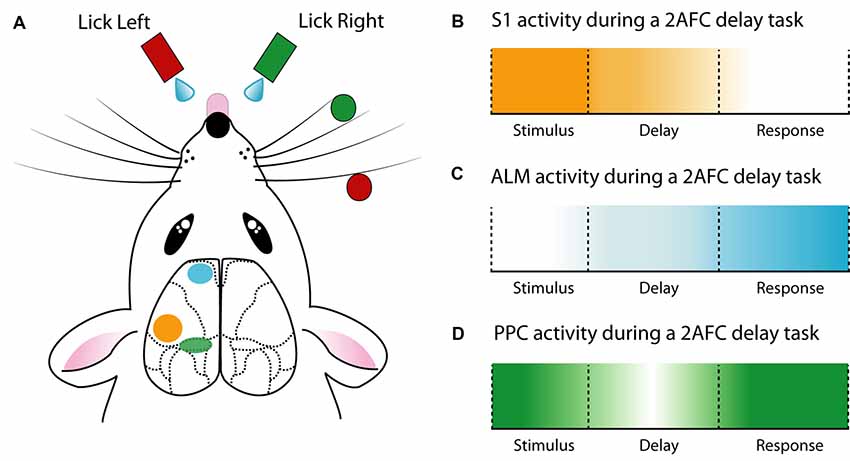
Figure 2. Cortical activity during a delay 2AFC task. (A) Schematic of a whisker-mediated object localization 2AFC task. Modified from O’Connor et al. (2010a,b), Guo et al. (2014b) and Chen et al. (2017). (B) The primary somatosensory cortex (S1) has maximal influence during stimulus presentation (Guo et al., 2014b). (C) Anterior lateral motor cortex (ALM) ramps activity during the delay epoch and reaches a maximum during the response epoch (Guo et al., 2014b; Li et al., 2015; Inagaki et al., 2018). (D) The posterior parietal cortex (PPC) is active throughout the 2AFC task, especially the stimulus and response epochs (Harvey et al., 2012; Goard et al., 2016).
Primary Sensory Cortices
The role of the primary sensory cortex is to encode sensory information. To investigate the neural basis of sensory encoding at the cellular or sub-cellular level, various head-fixed behavioral paradigms have been developed. Combining the clearly defined cortical-microstructure with the prominent and easily assessable location of whiskers, the barrel cortex provides an ideal primary sense to probe during sensory-based behavior in the head-fixed preparation. Therefore, numerous studies have probed the neural activity contributing to vibrissae–based behavior. For example, the neural activity underlying perception of whisker deflection, induced by magnetic stimulation of identified whiskers coated with metallic particles has been probed using two-photon calcium imaging (Takahashi et al., 2016) and whole-cell patch-clamp (Sachidhanandam et al., 2013). Using a simple Go/NoGo behavioral task, these studies reveal that the perception of highly controlled whisker deflection requires calcium spikes in apical dendrites of layer 5 pyramidal neurons (Takahashi et al., 2016) and generates a reliable excitatory response in the somatic membrane potential which depends on cortical state (Sachidhanandam et al., 2013). Similarly, several studies have contributed to the understanding of whisker-mediated object localization, using variations of the Go/NoGo paradigm. For instance, active touch, which is the combination of whisking and touch, generates a strong activation of the apical tuft dendrites of layer 5 pyramidal neurons in the barrel cortex, driven by projections from the primary motor cortex (Xu et al., 2012). Axonal projections from the primary motor cortex to S1 were found to relay signals related to many task-related features, which, combined with ongoing sensory input, allows S1 neurons to combine and compute object localization (Petreanu et al., 2012). Moreover, S1 projections are recruited differently depending on task conditions. Chen et al. (2013) reported higher activity in neurons projecting to the primary motor cortex during the object localization task, while more neurons which project to secondary somatosensory cortex were active during texture discrimination. Despite these differences, both primary motor cortex-projecting and secondary somatosensory cortex-projecting S1 neurons could discriminate between Go and NoGo trials. Neural encoding of trial type during whisker-based object localization has also been shown to be both cell-type (Yu et al., 2019) and layer-specific, with a greater proportion of discriminating neurons located within layer 4 and 5 of the barrel cortex (O’Connor et al., 2010b). Taken together, the simple Go/NoGo behavioral task adopted in various laboratories has helped unravel the complex microcircuitry underlying touch perception and object localization.
Another sensory cortical region that has been the focus of much research is the primary visual cortex (V1). Here, the head-fixed preparation is advantageous as the animal can be oriented towards the visual stimulus (although this does not ensure stereotyped pupil location, see Wallace et al., 2013). By combining the head-fixed preparation with a spherical treadmill (Niell and Stryker, 2010) illustrated that the V1 response is strongly modulated by behavioral state, i.e., moving, stationary, sleeping, etc. Surprisingly, even in the absence of visual input, V1 neurons typically respond to a combination of running speed and visual speed (Polack et al., 2013; Saleem et al., 2013). Other studies have focused on the involvement of V1 in perceptual decision-making tasks. Here, V1 neurons were found to respond mainly during stimulus presentation, and inhibition of V1 impaired performance of the task (Goard et al., 2016). The head-fixed preparation also enables precise control over the direction and intensity of sound delivery for investigating sensory encoding and functional connectivity in the primary auditory cortex (A1; Francis et al., 2018). Using a sound-guided detection task (Kato et al., 2015) found that task engagement results in an increase in the fraction of responsive excitatory neurons, whereas responsive somatostatin expressing interneurons were reduced. Similar results were obtained in another study using two behavioral tasks of different complexity. Here, A1 can be bypassed when discriminating between simple sounds but is necessary when the discrimination involves more complex sounds with frequency overlap (Ceballo et al., 2019).
Posterior Parietal Cortex
The posterior parietal cortex (PPC) is a classical association cortical area which is involved in various behaviors, such as decision making (Raposo et al., 2014; Goard et al., 2016; Runyan et al., 2017), evidence accumulation (Morcos and Harvey, 2016), navigation (Krumin et al., 2018) and sensory representation (Song et al., 2017; Akrami et al., 2018; Mohan et al., 2018). Often described as an integrative hub for multiple senses (Song et al., 2017; Mohan et al., 2018; Lyamzin and Benucci, 2019), the PPC receives input from various sensory cortices (Wilber et al., 2015; Zhuang et al., 2017), the thalamus (Reep et al., 1994) as well as neuromodulatory input from the basal forebrain (Broussard, 2012).
Using a memory-guided navigation task in a virtual reality setup (Harvey et al., 2012) explored the neural dynamics of L2/3 pyramidal neurons within PPC using two-photon calcium imaging. Here, neurons exhibited choice-specific sequences that could be categorized according to their activity pattern during different task epochs (epoch-specific activity). This encoding of memory-guided decisions was mostly stable on single days but was reorganized over weeks (Driscoll et al., 2017). Although overall PPC activity reaches a set point to perform the memory-guided navigation task, individual neurons shift their activity over days, some being more inconsistent than others. Learned task features may have less consistency over time, as the neurons with the least consistent relationship between activity and behavior received greater information about task features, such as trial type and maze position (Driscoll et al., 2017). Since the PPC encodes posture (Mimica et al., 2018), it is important to note that similar involvement of the PPC during memory-guided decisions was also recorded in head-fixed visual discrimination tasks that did not involve navigation (Goard et al., 2016).
Anterior Lateral Motor Cortex
Due to the known involvement in motor planning and movement, the role of the anterior lateral motor cortex (ALM) has been extensively investigated during behavior. Building on previous work (Guo et al., 2014b) demonstrated the importance of the ALM in a 2AFC whisker-mediated delay task. Here, the ALM showed choice-specific preparatory activity as well as movement-related activity, with unilateral inhibition of the ALM during the delay epoch biasing the choice to the ipsilateral direction. Confirming and expanding on these findings (Li et al., 2015) demonstrated that ALM neurons have lateralized preference for contralateral movements. This lateralization was found to be driven by pyramidal tract neurons and not intratelencephallic neurons, although both were activated during preparatory activity. Within the ALM, the preparatory activity in intratelencephallic neurons was converted to motor output in pyramidal tract neurons, affecting the upcoming motor output. Interestingly, despite encoding sensory information (Chen et al., 2017), the ALM is required for motor planning independent of sensory modality (Inagaki et al., 2018). Here, tactile- or auditory- based tasks both displayed diverse but consistent ALM activity during a delay epoch, with behavioral performance decreasing during ALM photoinhibition. Combined with recordings during free-behavior (Erlich et al., 2011), these studies highlight the important role of the ALM in the planning and execution of motor output.
Important Considerations When Exploring Cortical Activity Using Head-Fixed Paradigms
While head-fixation is crucial for exploring the cellular and sub-cellular basis of many behaviors, the head-fixed state can alter overall behavior. For example, head-restrained rats have far fewer whisking movements compared with freely moving behavior (Sellien et al., 2005). Likewise (Whishaw et al., 2017) illustrated that rats used alternative strategies during exploration and reach-and-grasp movement when in the head-fixed state locating objects using “touch-release-grasp” rather than sniffing. However, when directly compared, there was no difference in performance during odor discrimination in head-fixed and freely moving mice (Abraham et al., 2012). Although sensory perception may not be dramatically altered, the head-fixed preparation limits natural aspects of behavior and the ability to naturally explore and maneuver the body during a sensory-based task. Therefore, although the head-fixed preparation is advantageous in the delivery of precise and reproducible stimuli, it has the disadvantage of a non-physiological restraint of the animal. Since recordings in both the head-fixed and freely-moving preparations illustrate that the head-fixed preparation influences brain state and neural activity (Lovett-Barron et al., 2014; Chung et al., 2017; Whishaw et al., 2017), simply freeing the animal’s body to move during recordings would not mitigate the non-physiological aspect of the head-fixed preparation. However, it must be noted that when reviewing neural activity underlying sensory-based cognitive tasks, limiting feedback from other systems such as the motor (Vinck et al., 2015; Dadarlat and Stryker, 2017; Ayaz et al., 2019) and head direction (Peyrache et al., 2015) systems can be advantageous.
It is important to consider the caveats of behavioral paradigms when exploring the cortical function and it must be noted that not all behaviors can be investigated using the head-fixed preparation, such as complex social behavior that requires physical interactions. Avoiding the need for head-fixation, miniaturized head-mounted microscopes and probes (Flusberg et al., 2008; Ghosh et al., 2011; Cai et al., 2016) allow the investigation of neural activity during natural behaviors (Helmchen et al., 2001; Sawinski et al., 2009; Miyamoto and Murayama, 2016; Chung et al., 2017; Zong et al., 2017; Meyer et al., 2018; Valero and English, 2019). Further advances using wireless head-mounted microscopes (Fan et al., 2011; Pinnell et al., 2015) remove the mechanical disturbances caused by cables attached to the animal. The use of miniaturized recording techniques allows freedom of movement, which is advantageous from an overall behavioral aspect, however freely moving animals do not allow the same highly controllable settings the head-fixed preparation allows. Furthermore, not all recording techniques have been developed for the freely moving preparation, and some head-mounted probes are not suitable for mice (due to weight and size requirements). Therefore, the head-fixed preparation is still typically deemed necessary for the in-depth investigation of cortical and sub-cortical activity during many behaviors.
Conclusion
The cortex and its role in behavior is enigmatic. To understand the underlying cortical circuits required for the execution of behaviors, it is crucial to examine neural activity while an animal is behaving. This can be achieved using the head-fixed preparation which provides highly controllable settings, and thereby precise stimuli presentation while allowing simultaneous cellular and sub-cellular recordings. The head-fixed preparation can be combined with behavioral paradigms ranging from simple sensory detection tasks, to complex, cross-modal, memory-guided decision-making tasks, allowing in-depth investigation into the neural activity underlying behaviors. Experiments using this preparation have provided insight into neural activity in various brain regions during sensory processing and higher-order cognitive functions. Although the head-fixed preparation limits natural behavior due to the head restraint, it provides valuable information about cognitive behaviors and the cortices involved. We are only beginning to understand and unravel the complexity of the neural activity underlying behavior, and while there is a rapid improvement in tethered and wireless recording techniques, the head-fixed preparation seems unlikely to be replaced as improvements to the head-fixed preparation steadily provides new platforms and setups, as well as new behavioral tasks to explore cortical functions during simple and complex behaviors. As recording techniques continue to push the boundaries of possibilities, such as functional imaging of deep brain structures with three-photon imaging (Wang et al., 2018) and simultaneous extracellular voltage recordings from hundreds of channels over 10 mm using the neuropixel probes (Jun et al., 2017), the head-fixed preparation will also continue to advance. For example, self-initiated head-fixation in the home cage will allow for the neural activity underlying more physiological behaviors to be investigated (Murphy et al., 2016). While there is no one-fits-all behavioral model, the flexibility of the head-fixed behavioral paradigms, combined with functional cellular and subcellular recordings and manipulations, provides insight into the neural dynamics underlying behaviors. Not only does this offer valuable information about single-cell dynamics, but it also expands our understanding of the individual cortices, both their individual and combined contributions to the overall behavioral output.
Author Contributions
A-SB and LP conceptualized and wrote the manuscript.
Funding
This work was supported by the NHMRC (APP1086082, APP1063533), ARC (DP160103047) and the Sylvia and Charles Viertel Charitable Foundation.
Conflict of Interest
The authors declare that the research was conducted in the absence of any commercial or financial relationships that could be construed as a potential conflict of interest.
References
Abraham, N. M., Guerin, D., Bhaukaurally, K., and Carleton, A. (2012). Similar odor discrimination behavior in head-restrained and freely moving mice. PLoS One 7:e51789. doi: 10.1371/journal.pone.0051789
Akrami, A., Kopec, C. D., Diamond, M. E., and Brody, C. D. (2018). Posterior parietal cortex represents sensory history and mediates its effects on behaviour. Nature 554, 368–372. doi: 10.1038/nature25510
Ayaz, A., Stauble, A., Hamada, M., Wulf, M. A., Saleem, A. B., and Helmchen, F. (2019). Layer-specific integration of locomotion and sensory information in mouse barrel cortex. Nat. Commun. 10:2585. doi: 10.1038/s41467-019-10564-8
Beltramo, R., and Scanziani, M. (2019). A collicular visual cortex: neocortical space for an ancient midbrain visual structure. Science 363, 64–69. doi: 10.1126/science.aau7052
Bittner, K. C., Milstein, A. D., Grienberger, C., Romani, S., and Magee, J. C. (2017). Behavioral time scale synaptic plasticity underlies CA1 place fields. Science 357, 1033–1036. doi: 10.1126/science.aan3846
Broussard, J. I. (2012). Posterior parietal cortex dynamically ranks topographic signals via cholinergic influence. Front. Integr. Neurosci. 6:32. doi: 10.3389/fnint.2012.00032
Burgalossi, A., Herfst, L., Von Heimendahl, M., Forste, H., Haskic, K., Schmidt, M., et al. (2011). Microcircuits of functionally identified neurons in the rat medial entorhinal cortex. Neuron 70, 773–786. doi: 10.1016/j.neuron.2011.04.003
Burgess, C. P., Lak, A., Steinmetz, N. A., Zatka-Haas, P., Bai Reddy, C., Jacobs, E. A. K., et al. (2017). High-yield methods for accurate two-alternative visual psychophysics in head-fixed mice. Cell Rep. 20, 2513–2524. doi: 10.1016/j.celrep.2017.08.047
Busse, L., Ayaz, A., Dhruv, N. T., Katzner, S., Saleem, A. B., Scholvinck, M. L., et al. (2011). The detection of visual contrast in the behaving mouse. J. Neurosci. 31, 11351–11361. doi: 10.1523/JNEUROSCI.6689-10.2011
Buzsáki, G. (2004). Large-scale recording of neuronal ensembles. Nat. Neurosci. 7, 446–451. doi: 10.1038/nn1233
Buzsáki, G., Anastassiou, C. A., and Koch, C. (2012). The origin of extracellular fields and currents—EEG, ECoG, LFP and spikes. Nat. Rev. Neurosci. 13, 407–420. doi: 10.1038/nrn3241
Cai, D. J., Aharoni, D., Shuman, T., Shobe, J., Biane, J., Song, W., et al. (2016). A shared neural ensemble links distinct contextual memories encoded close in time. Nature 534, 115–118. doi: 10.1038/nature17955
Carandini, M., and Churchland, A. K. (2013). Probing perceptual decisions in rodents. Nat. Neurosci. 16, 824–831. doi: 10.1038/nn.3410
Ceballo, S., Piwkowska, Z., Bourg, J., Daret, A., and Bathellier, B. (2019). Targeted cortical manipulation of auditory perception. Neuron 104, 1168.e5–1179.e5. doi: 10.1016/j.neuron.2019.09.043
Chen, J. L., Carta, S., Soldado-Magraner, J., Schneider, B. L., and Helmchen, F. (2013). Behaviour-dependent recruitment of long-range projection neurons in somatosensory cortex. Nature 499, 336–340. doi: 10.1038/nature12236
Chen, T. W., Li, N., Daie, K., and Svoboda, K. (2017). A map of anticipatory activity in mouse motor cortex. Neuron 94, 866.e4–879.e4. doi: 10.1016/j.neuron.2017.05.005
Chung, J., Sharif, F., Jung, D., Kim, S., and Royer, S. (2017). Micro-drive and headgear for chronic implant and recovery of optoelectronic probes. Sci. Rep. 7:2773. doi: 10.1038/s41598-017-03340-5
Cichon, J., and Gan, W. B. (2015). Branch-specific dendritic Ca2+ spikes cause persistent synaptic plasticity. Nature 520, 180–185. doi: 10.1038/nature14251
Dadarlat, M. C., and Stryker, M. P. (2017). Locomotion enhances neural encoding of visual stimuli in mouse V1. J. Neurosci. 37, 3764–3775. doi: 10.1523/jneurosci.2728-16.2017
Dombeck, D. A., Harvey, C. D., Tian, L., Looger, L. L., and Tank, D. W. (2010). Functional imaging of hippocampal place cells at cellular resolution during virtual navigation. Nat. Neurosci. 13, 1433–1440. doi: 10.1038/nn.2648
Dombeck, D. A., Khabbaz, A. N., Collman, F., Adelman, T. L., and Tank, D. W. (2007). Imaging large-scale neural activity with cellular resolution in awake, mobile mice. Neuron 56, 43–57. doi: 10.1016/j.neuron.2007.08.003
Domnisoru, C., Kinkhabwala, A. A., and Tank, D. W. (2013). Membrane potential dynamics of grid cells. Nature 495, 199–204. doi: 10.1038/nature11973
Driscoll, L. N., Pettit, N. L., Minderer, M., Chettih, S. N., and Harvey, C. D. (2017). Dynamic reorganization of neuronal activity patterns in parietal cortex. Cell 170, 986.e16–999.e16. doi: 10.1016/j.cell.2017.07.021
Dudchenko, P. A. (2004). An overview of the tasks used to test working memory in rodents. Neurosci. Biobehav. Rev. 28, 699–709. doi: 10.1016/j.neubiorev.2004.09.002
Erlich, J. C., Bialek, M., and Brody, C. D. (2011). A cortical substrate for memory-guided orienting in the rat. Neuron 72, 330–343. doi: 10.1016/j.neuron.2011.07.010
Estebanez, L., Hoffmann, D., Voigt, B. C., and Poulet, J. F. A. (2017). Parvalbumin-expressing GABAergic neurons in primary motor cortex signal reaching. Cell Rep. 20, 308–318. doi: 10.1016/j.celrep.2017.06.044
Fan, D., Rich, D., Holtzman, T., Ruther, P., Dalley, J. W., Lopez, A., et al. (2011). A wireless multi-channel recording system for freely behaving mice and rats. PLoS One 6:e22033. doi: 10.1371/journal.pone.0022033
Fassihi, A., Akrami, A., Esmaeili, V., and Diamond, M. E. (2014). Tactile perception and working memory in rats and humans. Proc. Natl. Acad. Sci. U S A 111, 2331–2336. doi: 10.1073/pnas.1315171111
Flusberg, B. A., Nimmerjahn, A., Cocker, E. D., Mukamel, E. A., Barretto, R. P., Ko, T. H., et al. (2008). High-speed, miniaturized fluorescence microscopy in freely moving mice. Nat. Methods 5, 935–938. doi: 10.1038/nmeth.1256
Francis, N. A., Winkowski, D. E., Sheikhattar, A., Armengol, K., Babadi, B., and Kanold, P. O. (2018). Small networks encode decision-making in primary auditory cortex. Neuron 97, 885.e6–897.e6. doi: 10.1016/j.neuron.2018.01.019
Fuster, J. M. (2001). The prefrontal cortex—an update: time is of the essence. Neuron 30, 319–333. doi: 10.1016/s0896-6273(01)00285-9
Ghosh, K. K., Burns, L. D., Cocker, E. D., Nimmerjahn, A., Ziv, Y., Gamal, A. E., et al. (2011). Miniaturized integration of a fluorescence microscope. Nat. Methods 8, 871–878. doi: 10.1038/nmeth.1694
Gilad, A., Gallero-Salas, Y., Groos, D., and Helmchen, F. (2018). Behavioral strategy determines frontal or posterior location of short-term memory in neocortex. Neuron 99, 814.e7–828.e7. doi: 10.1016/j.neuron.2018.07.029
Goard, M. J., Pho, G. N., Woodson, J., and Sur, M. (2016). Distinct roles of visual, parietal and frontal motor cortices in memory-guided sensorimotor decisions. Elife 5:e13764. doi: 10.7554/elife.13764
Guo, Z. V., Hires, S. A., Li, N., O’Connor, D. H., Komiyama, T., Ophir, E., et al. (2014a). Procedures for behavioral experiments in head-fixed mice. PLoS One 9:e88678. doi: 10.1371/journal.pone.0088678
Guo, Z. V., Li, N., Huber, D., Ophir, E., Gutnisky, D., Ting, J. T., et al. (2014b). Flow of cortical activity underlying a tactile decision in mice. Neuron 81, 179–194. doi: 10.1016/j.neuron.2013.10.020
Harvey, C. D., Coen, P., and Tank, D. W. (2012). Choice-specific sequences in parietal cortex during a virtual-navigation decision task. Nature 484, 62–68. doi: 10.1038/nature10918
Harvey, C. D., Collman, F., Dombeck, D. A., and Tank, D. W. (2009). Intracellular dynamics of hippocampal place cells during virtual navigation. Nature 461, 941–946. doi: 10.1038/nature08499
Hawrylycz, M., Anastassiou, C., Arkhipov, A., Berg, J., Buice, M., Cain, N., et al. (2016). Inferring cortical function in the mouse visual system through large-scale systems neuroscience. Proc. Natl. Acad. Sci. U S A 113, 7337–7344. doi: 10.1073/pnas.1512901113
Heindorf, M., Arber, S., and Keller, G. B. (2018). Mouse motor cortex coordinates the behavioral response to unpredicted sensory feedback. Neuron 99, 1040.e5–1054.e5. doi: 10.1016/j.neuron.2018.07.046
Helmchen, F., Fee, M. S., Tank, D. W., and Denk, W. (2001). A miniature head-mounted two-photon microscope. high-resolution brain imaging in freely moving animals. Neuron 31, 903–912. doi: 10.1016/s0896-6273(01)00421-4
Helmchen, F., Gilad, A., and Chen, J. L. (2018). Neocortical dynamics during whisker-based sensory discrimination in head-restrained mice. Neuroscience 368, 57–69. doi: 10.1016/j.neuroscience.2017.09.003
Hernández, A., Salinas, E., Garcia, R., and Romo, R. (1997). Discrimination in the sense of flutter: new psychophysical measurements in monkeys. J. Neurosci. 17, 6391–6400. doi: 10.1523/jneurosci.17-16-06391.1997
Higley, M. J. (2012). “Determination of cortical circuit function using current source-density analysis in vivo,” in Neuronal Network Analysis, eds T. Fellin and M. Halassa (Totowa, NJ: Humana Press), 205–218.
Hong, Y. K., Lacefield, C. O., Rodgers, C. C., and Bruno, R. M. (2018). Sensation, movement and learning in the absence of barrel cortex. Nature 561, 542–546. doi: 10.1038/s41586-018-0527-y
Inagaki, H. K., Fontolan, L., Romani, S., and Svoboda, K. (2019). Discrete attractor dynamics underlies persistent activity in the frontal cortex. Nature 566, 212–217. doi: 10.1038/s41586-019-0919-7
Inagaki, H. K., Inagaki, M., Romani, S., and Svoboda, K. (2018). Low-dimensional and monotonic preparatory activity in mouse anterior lateral motor cortex. J. Neurosci. 38, 4163–4185. doi: 10.1523/jneurosci.3152-17.2018
Jun, J. J., Steinmetz, N. A., Siegle, J. H., Denman, D. J., Bauza, M., Barbarits, B., et al. (2017). Fully integrated silicon probes for high-density recording of neural activity. Nature 551, 232–236. doi: 10.1038/nature24636
Kato, H. K., Gillet, S. N., and Isaacson, J. S. (2015). Flexible sensory representations in auditory cortex driven by behavioral relevance. Neuron 88, 1027–1039. doi: 10.1016/j.neuron.2015.10.024
Kislin, M., Mugantseva, E., Molotkov, D., Kulesskaya, N., Khirug, S., Kirilkin, I., et al. (2014). Flat-floored air-lifted platform: a new method for combining behavior with microscopy or electrophysiology on awake freely moving rodents. J. Vis. Exp. 88:e51869. doi: 10.3791/51869
Krumin, M., Lee, J. J., Harris, K. D., and Carandini, M. (2018). Decision and navigation in mouse parietal cortex. Elife 7:e42583. doi: 10.7554/elife.42583
Lenschow, C., and Brecht, M. (2015). Barrel cortex membrane potential dynamics in social touch. Neuron 85, 718–725. doi: 10.1016/j.neuron.2014.12.059
Li, N., Chen, T. W., Guo, Z. V., Gerfen, C. R., and Svoboda, K. (2015). A motor cortex circuit for motor planning and movement. Nature 519, 51–56. doi: 10.1038/nature14178
Liu, D., Gu, X., Zhu, J., Zhang, X., Han, Z., Yan, W., et al. (2014). Medial prefrontal activity during delay period contributes to learning of a working memory task. Science 346, 458–463. doi: 10.1126/science.1256573
Lovett-Barron, M., Kaifosh, P., Kheirbek, M. A., Danielson, N., Zaremba, J. D., Reardon, T. R., et al. (2014). Dendritic inhibition in the hippocampus supports fear learning. Science 343, 857–863. doi: 10.1126/science.1247485
Lyamzin, D., and Benucci, A. (2019). The mouse posterior parietal cortex: anatomy and functions. Neurosci. Res. 140, 14–22. doi: 10.1016/j.neures.2018.10.008
Makino, H., and Komiyama, T. (2015). Learning enhances the relative impact of top-down processing in the visual cortex. Nat. Neurosci. 18, 1116–1122. doi: 10.1038/nn.4061
Mayrhofer, J. M., Skreb, V., Von Der Behrens, W., Musall, S., Weber, B., and Haiss, F. (2013). Novel two-alternative forced choice paradigm for bilateral vibrotactile whisker frequency discrimination in head-fixed mice and rats. J. Neurophysiol. 109, 273–284. doi: 10.1152/jn.00488.2012
McGinley, M. J., David, S. V., and Mccormick, D. A. (2015). Cortical membrane potential signature of optimal states for sensory signal detection. Neuron 87, 179–192. doi: 10.1016/j.neuron.2015.05.038
Meyer, A. F., Poort, J., O’Keefe, J., Sahani, M., and Linden, J. F. (2018). A head-mounted camera system integrates detailed behavioral monitoring with multichannel electrophysiology in freely moving mice. Neuron 100, 46.e7–60.e7. doi: 10.1016/j.neuron.2018.09.020
Micallef, A. H., Takahashi, N., Larkum, M. E., and Palmer, L. M. (2017). A reward-based behavioral platform to measure neural activity during head-fixed behavior. Front. Cell. Neurosci. 11:156. doi: 10.3389/fncel.2017.00156
Mimica, B., Dunn, B. A., Tombaz, T., Bojja, V., and Whitlock, J. R. (2018). Efficient cortical coding of 3D posture in freely behaving rats. Science 362, 584–589. doi: 10.1126/science.aau2013
Miyamoto, D., and Murayama, M. (2016). The fiber-optic imaging and manipulation of neural activity during animal behavior. Neurosci. Res. 103, 1–9. doi: 10.1016/j.neures.2015.09.004
Mohan, H., De Haan, R., Mansvelder, H. D., and De Kock, C. P. J. (2018). The posterior parietal cortex as integrative hub for whisker sensorimotor information. Neuroscience 368, 240–245. doi: 10.1016/j.neuroscience.2017.06.020
Morandell, K., and Huber, D. (2017). The role of forelimb motor cortex areas in goal directed action in mice. Sci. Rep. 7:15759. doi: 10.1038/s41598-017-15835-2
Morcos, A. S., and Harvey, C. D. (2016). History-dependent variability in population dynamics during evidence accumulation in cortex. Nat. Neurosci. 19, 1672–1681. doi: 10.1038/nn.4403
Mountcastle, V. B., Steinmetz, M. A., and Romo, R. (1990). Frequency discrimination in the sense of flutter: psychophysical measurements correlated with postcentral events in behaving monkeys. J. Neurosci. 10, 3032–3044. doi: 10.1523/jneurosci.10-09-03032.1990
Murphy, T. H., Boyd, J. D., Bolanos, F., Vanni, M. P., Silasi, G., Haupt, D., et al. (2016). High-throughput automated home-cage mesoscopic functional imaging of mouse cortex. Nat. Commun. 7:11611. doi: 10.1038/ncomms11611
Nashaat, M. A., Oraby, H., Sachdev, R. N., Winter, Y., and Larkum, M. E. (2016). Air-track: a real-world floating environment for active sensing in head-fixed mice. J. Neurophysiol. 116, 1542–1553. doi: 10.1152/jn.00088.2016
Niell, C. M., and Stryker, M. P. (2010). Modulation of visual responses by behavioral state in mouse visual cortex. Neuron 65, 472–479. doi: 10.1016/j.neuron.2010.01.033
O’Connor, D. H., Clack, N. G., Huber, D., Komiyama, T., Myers, E. W., and Svoboda, K. (2010a). Vibrissa-based object localization in head-fixed mice. J. Neurosci. 30, 1947–1967. doi: 10.1523/jneurosci.3762-09.2010
O’Connor, D. H., Peron, S. P., Huber, D., and Svoboda, K. (2010b). Neural activity in barrel cortex underlying vibrissa-based object localization in mice. Neuron 67, 1048–1061. doi: 10.1016/j.neuron.2010.08.026
Parker, A. J., and Newsome, W. T. (1998). Sense and the single neuron: probing the physiology of perception. Annu. Rev. Neurosci. 21, 227–277. doi: 10.1146/annurev.neuro.21.1.227
Peron, S. P., Freeman, J., Iyer, V., Guo, C., and Svoboda, K. (2015). A cellular resolution map of barrel cortex activity during tactile behavior. Neuron 86, 783–799. doi: 10.1016/j.neuron.2015.03.027
Petreanu, L., Gutnisky, D. A., Huber, D., Xu, N. L., O’Connor, D. H., Tian, L., et al. (2012). Activity in motor-sensory projections reveals distributed coding in somatosensation. Nature 489, 299–303. doi: 10.1038/nature11321
Peyrache, A., Lacroix, M. M., Petersen, P. C., and Buzsáki, G. (2015). Internally organized mechanisms of the head direction sense. Nat. Neurosci. 18, 569–575. doi: 10.1038/nn.3968
Pinnell, R. C., Dempster, J., and Pratt, J. (2015). Miniature wireless recording and stimulation system for rodent behavioural testing. J. Neural Eng. 12:066015. doi: 10.1088/1741-2560/12/6/066015
Polack, P. O., Friedman, J., and Golshani, P. (2013). Cellular mechanisms of brain state-dependent gain modulation in visual cortex. Nat. Neurosci. 16, 1331–1339. doi: 10.1038/nn.3464
Poulet, J. F., and Petersen, C. C. (2008). Internal brain state regulates membrane potential synchrony in barrel cortex of behaving mice. Nature 454, 881–885. doi: 10.1038/nature07150
Quintana, J., Yajeya, J., and Fuster, J. M. (1988). Prefrontal representation of stimulus attributes during delay tasks. I. Unit activity in cross-temporal integration of sensory and sensory-motor information. Brain Res. 474, 211–221. doi: 10.1016/0006-8993(88)90436-2
Radvansky, B. A., and Dombeck, D. A. (2018). An olfactory virtual reality system for mice. Nat. Commun. 9:839. doi: 10.1038/s41467-018-03262-4
Randel, N., Asadulina, A., Bezares-Calderon, L. A., Veraszto, C., Williams, E. A., Conzelmann, M., et al. (2014). Neuronal connectome of a sensory-motor circuit for visual navigation. Elife 3:e02730. doi: 10.7554/eLife.02730
Rao, R. P., Mielke, F., Bobrov, E., and Brecht, M. (2014). Vocalization-whisking coordination and multisensory integration of social signals in rat auditory cortex. Elife 3:e03185. doi: 10.7554/eLife.03185
Raposo, D., Kaufman, M. T., and Churchland, A. K. (2014). A category-free neural population supports evolving demands during decision-making. Nat. Neurosci. 17, 1784–1792. doi: 10.1038/nn.3865
Reep, R. L., Chandler, H. C., King, V., and Corwin, J. V. (1994). Rat posterior parietal cortex: topography of corticocortical and thalamic connections. Exp. Brain Res. 100, 67–84. doi: 10.1007/bf00227280
Runyan, C. A., Piasini, E., Panzeri, S., and Harvey, C. D. (2017). Distinct timescales of population coding across cortex. Nature 548, 92–96. doi: 10.1038/nature23020
Sachidhanandam, S., Sreenivasan, V., Kyriakatos, A., Kremer, Y., and Petersen, C. C. (2013). Membrane potential correlates of sensory perception in mouse barrel cortex. Nat. Neurosci. 16, 1671–1677. doi: 10.1038/nn.3532
Saleem, A. B., Ayaz, A., Jeffery, K. J., Harris, K. D., and Carandini, M. (2013). Integration of visual motion and locomotion in mouse visual cortex. Nat. Neurosci. 16, 1864–1869. doi: 10.1038/nn.3567
Sawinski, J., Wallace, D. J., Greenberg, D. S., Grossmann, S., Denk, W., and Kerr, J. N. (2009). Visually evoked activity in cortical cells imaged in freely moving animals. Proc. Natl. Acad. Sci. U S A 106, 19557–19562. doi: 10.1073/pnas.0903680106
Schmidt-Hieber, C., and Häusser, M. (2013). Cellular mechanisms of spatial navigation in the medial entorhinal cortex. Nat. Neurosci. 16, 325–331. doi: 10.1038/nn.3340
Schneider, D. M., Sundararajan, J., and Mooney, R. (2018). A cortical filter that learns to suppress the acoustic consequences of movement. Nature 561, 391–395. doi: 10.1038/s41586-018-0520-5
Sellien, H., Eshenroder, D. S., and Ebner, F. F. (2005). Comparison of bilateral whisker movement in freely exploring and head-fixed adult rats. Somatosens. Mot. Res. 22, 97–114. doi: 10.1080/08990220400015375
Sheffield, M. E. J., Adoff, M. D., and Dombeck, D. A. (2017). Increased prevalence of calcium transients across the dendritic arbor during place field formation. Neuron 96, 490.e5–504.e5. doi: 10.1016/j.neuron.2017.09.029
Shimaoka, D., Harris, K. D., and Carandini, M. (2018). Effects of arousal on mouse sensory cortex depend on modality. Cell Rep. 25:3230. doi: 10.1016/j.celrep.2018.11.105
Sofroniew, N. J., Vlasov, Y. A., Hires, S. A., Freeman, J., and Svoboda, K. (2015). Neural coding in barrel cortex during whisker-guided locomotion. Elife 4:e12559. doi: 10.7554/eLife.12559
Song, Y. H., Kim, J. H., Jeong, H. W., Choi, I., Jeong, D., Kim, K., et al. (2017). A neural circuit for auditory dominance over visual perception. Neuron 93, 1236–1237. doi: 10.1016/j.neuron.2017.02.026
Suzuki, M., and Larkum, M. E. (2017). Dendritic calcium spikes are clearly detectable at the cortical surface. Nat. Commun. 8:276. doi: 10.1038/s41467-017-00282-4
Takahashi, N., Oertner, T. G., Hegemann, P., and Larkum, M. E. (2016). Active cortical dendrites modulate perception. Science 354, 1587–1590. doi: 10.1126/science.aah6066
Tang, Q., Brecht, M., and Burgalossi, A. (2014). Juxtacellular recording and morphological identification of single neurons in freely moving rats. Nat. Protoc. 9, 2369–2381. doi: 10.1038/nprot.2014.161
Thurley, K., and Ayaz, A. (2017). Virtual reality systems for rodents. Curr. Zool. 63, 109–119. doi: 10.1093/cz/zow070
Valero, M., and English, D. F. (2019). Head-mounted approaches for targeting single-cells in freely moving animals. J. Neurosci. Methods 326:108397. doi: 10.1016/j.jneumeth.2019.108397
Vinck, M., Batista-Brito, R., Knoblich, U., and Cardin, J. A. (2015). Arousal and locomotion make distinct contributions to cortical activity patterns and visual encoding. Neuron 86, 740–754. doi: 10.1016/j.neuron.2015.03.028
Wallace, D. J., Greenberg, D. S., Sawinski, J., Rulla, S., Notaro, G., and Kerr, J. N. (2013). Rats maintain an overhead binocular field at the expense of constant fusion. Nature 498, 65–69. doi: 10.1038/nature12153
Wang, T., Ouzounov, D. G., Wu, C., Horton, N. G., Zhang, B., Wu, C. H., et al. (2018). Three-photon imaging of mouse brain structure and function through the intact skull. Nat. Methods 15, 789–792. doi: 10.1038/s41592-018-0115-y
Wei, Z., Inagaki, H., Li, N., Svoboda, K., and Druckmann, S. (2019). An orderly single-trial organization of population dynamics in premotor cortex predicts behavioral variability. Nat. Commun. 10:216. doi: 10.1038/s41467-018-08141-6
Whishaw, I. Q., Faraji, J., Kuntz, J., Mirza Agha, B., Patel, M., Metz, G. A. S., et al. (2017). Organization of the reach and grasp in head-fixed vs. freely-moving mice provides support for multiple motor channel theory of neocortical organization. Exp. Brain Res. 235, 1919–1932. doi: 10.1007/s00221-017-4925-4
Wilber, A. A., Clark, B. J., Demecha, A. J., Mesina, L., Vos, J. M., and Mcnaughton, B. L. (2015). Cortical connectivity maps reveal anatomically distinct areas in the parietal cortex of the rat. Front. Neural Circuits 8:146. doi: 10.3389/fncir.2014.00146
Xu, N. L., Harnett, M. T., Williams, S. R., Huber, D., O’Connor, D. H., Svoboda, K., et al. (2012). Nonlinear dendritic integration of sensory and motor input during an active sensing task. Nature 492, 247–251. doi: 10.1038/nature11601
Yajeya, J., Quintana, J., and Fuster, J. M. (1988). Prefrontal representation of stimulus attributes during delay tasks: II. The role of behavioral significance. Brain Res. 474, 222–230. doi: 10.1016/0006-8993(88)90437-4
Yu, J., Hu, H., Agmon, A., and Svoboda, K. (2019). Recruitment of GABAergic interneurons in the barrel cortex during active tactile behavior. Neuron 104, 412.e4–427.e4. doi: 10.1016/j.neuron.2019.07.027
Zariwala, H. A., Kepecs, A., Uchida, N., Hirokawa, J., and Mainen, Z. F. (2013). The limits of deliberation in a perceptual decision task. Neuron 78, 339–351. doi: 10.1016/j.neuron.2013.02.010
Zhong, L., Zhang, Y., Duan, C. A., Deng, J., Pan, J., and Xu, N. L. (2019). Causal contributions of parietal cortex to perceptual decision-making during stimulus categorization. Nat. Neurosci. 22, 963–973. doi: 10.1038/s41593-019-0383-6
Zhuang, J., Ng, L., Williams, D., Valley, M., Li, Y., Garrett, M., et al. (2017). An extended retinotopic map of mouse cortex. Elife 6:e18372. doi: 10.7554/eLife.18372
Znamenskiy, P., and Zador, A. M. (2013). Corticostriatal neurons in auditory cortex drive decisions during auditory discrimination. Nature 497, 482–485. doi: 10.1038/nature12077
Keywords: cortex, head-fixed, sensory-based behavior, primary sensory cortices, posterior parietal cortex, anterior lateral motor cortex, Go/NoGo, 2AFC
Citation: Bjerre A-S and Palmer LM (2020) Probing Cortical Activity During Head-Fixed Behavior. Front. Mol. Neurosci. 13:30. doi: 10.3389/fnmol.2020.00030
Received: 18 October 2019; Accepted: 10 February 2020;
Published: 28 February 2020.
Edited by:
Robert J. Harvey, University of the Sunshine Coast, AustraliaReviewed by:
Christina Cerkevich, University of Pittsburgh, United StatesThomas Bessaïh, Université Pierre et Marie Curie, France
Copyright © 2020 Bjerre and Palmer. This is an open-access article distributed under the terms of the Creative Commons Attribution License (CC BY). The use, distribution or reproduction in other forums is permitted, provided the original author(s) and the copyright owner(s) are credited and that the original publication in this journal is cited, in accordance with accepted academic practice. No use, distribution or reproduction is permitted which does not comply with these terms.
*Correspondence: Lucy M. Palmer, lucy.palmer@florey.edu.au