- 1Department of Radiology, The Affiliated Xi’an Central Hospital of Xi’an Jiaotong University, Xi’an, China
- 2Department of Radiology, The Affiliated Xi’an XD Group Hospital of Shanxi University of Traditional Chinese Medicine, Xi’an, China
Cervical discogenic pain (CDP) is a clinically common pain syndrome caused by cervical disk degeneration. A large number of studies have reported that CDP results in brain functional impairments. However, the detailed dynamic brain functional abnormalities in CDP are still unclear. In this study, using resting-state functional magnetic resonance imaging, we explored the neural basis of CDP with 40 CDP patients and 40 age-, gender-matched healthy controls to delineate the changes of the voxel-level static and dynamic amplitude of low frequency fluctuations (ALFF). We found increased static ALFF in left insula (INS) and posterior precuneus (PCu), and decreased static ALFF in left precentral/postcentral gyrus (PreCG/PoCG), thalamus (THA), and subgenual anterior cingulate cortex in CPD patients compared to healthy controls. We also found decreased dynamic ALFF in left PreCG/PoCG, right posterior middle temporal gyrus, and bilateral THA. Moreover, we found that static ALFF in left PreCG/PoCG and dynamic ALFF in THA were significantly negatively correlated with visual analog scale and disease duration, respectively. Our findings provide the neurophysiological basis for CDP and facilitate understanding the neuropathology of CDP.
Introduction
Cervical discogenic pain (CDP) is a clinically common pain syndrome caused by cervical disk degeneration which results in chronic pain in the head, neck, shoulder and upper limbs, as well as pain associated with numbness (Tracy and Bartleson, 2010; Thoomes et al., 2012). Long-term chronic pain seriously affects patients’ physical and mental health and quality of life. The clinical studies have demonstrated that patients with long-term duration chronic pain led to brain functional impairments of sensory, motor, cognition, memory, and affective processing (Montero-Homs, 2009; Linton, 2013; Denkinger et al., 2014). Although a few studies have reported functional connectivity and local regional homogeneity abnormalities in CDP patients using resting-state functional magnetic resonance imaging (fMRI) (Yu et al., 2017; Woodworth et al., 2018), the dynamic changes of the low-frequency oscillation in CDP is remains unknown.
Resting-state fMRI is commonly used to measure the spontaneous low-frequency fluctuations of brain activities with blood oxygen level-dependent (BOLD) signals (Biswal et al., 1995; Fox and Raichle, 2007). A large number of previous studies applied resting-state fMRI to reveal the intrinsic functional organization of brain in healthy subjects and to identify the changes of the intrinsic functional connectivities under diseases (Greicius et al., 2003; Fox et al., 2006; Wang et al., 2015, 2017d; Sun et al., 2018; Xu et al., 2019a; Gao et al., 2020). Moreover, many recent studies have applied resting-state fMRI to reveal the brain mechanism of neuromodulation treatment for clinical disorders (Peng et al., 2012; Liu et al., 2015; Wang et al., 2017b,c,d, 2020; Wang J. et al., 2018; Bai et al., 2018, 2019). The amplitude of low frequency fluctuations (ALFF) is a main measurement to characterize the functional activity during resting-sate (Zang et al., 2007). Combining the ALFF with sliding-window approach, the dynamic ALFF (dALFF) method was proposed to measure the variance of ALFF over time (Fu et al., 2018). The dALFF provides a new avenue to depict time-varying local brain activity. Generally, the static ALFF was calculated by taking all the time points’ series as a whole for power analyses, whereas the dALFF was calculated by dividing the whole time series into different small segmentations for power analysis. Therefore, using static and dynamic ALFF may provide a complementary evidence to uncover the brain functional changes in patients with CDP.
In this study, we aimed to identify the brain functional abnormalities caused by CDP using static and dynamic ALFF method in 40 CDP patients and 40 age- and gender-matched healthy controls. We hypothesized that the static and dynamic could reveal different but overlapped brain areas showing functional abnormalities. The voxel-wise static and dynamic ALFF maps were calculated and compared between CDP patients and healthy controls. The correlation analyses were performed to identify the relationships between ALFF measurements and clinical characteristics.
Materials and Methods
Participants
Forty right-handed CDP patients (22 females and 18 males; mean age: 53.6 ± 6.9 years) and 40 gender- and age-matched healthy controls (22 females and 18 males; mean age: 52.8 ± 7.6 years) were used in current study. All the CDP patients were diagnosed by one orthopedist with physical and imaging examination. A main characteristic for CDP is that the damaged disk showed reduced weighted T2 signals compared to the neighboring normal disk. The patients with cervical disk herniation, disk degeneration and stenosis, and cervical spondylosis were excluded. Moreover, only the pain caused by the internal disorder of the intervertebral disk not by radiation pain or segmental nerve disorder was considered to be CDP. The CDP patients with hypertension, diabetes, mental illness, intracranial infection, craniocerebral trauma, MRI contraindication, and a history of chronic pain were excluded. Forty right-handed healthy controls were recruited from the community and not have any hypertension, diabetes, mental illness, intracranial infection, craniocerebral trauma, and a history of chronic pain. The clinical measures were obtained to assess the pain intensity, cognitive functions and emotional states. The visual analog scale (VAS) test was used to assess the pain intensity. The Montreal cognitive assessment scale (MoCA) was used to evaluate the cognitive ability. The Hamilton depression scale (HAMD) and Hamilton anxiety scale (HAMA) were applied to determine their depression and anxiety levels, respectively. The written informed consents were provided and obtained from all subjects. The study was approved by the Ethics Committee of the Affiliated Xi’an Central Hospital of Xi’an Jiaotong University and was accordance with the Helsinki declaration and its later amendments or comparable ethical standards.
Resting-State fMRI Data Acquisition
The resting-state fMRI data was collected using a Philips 3.0T MRI scanner. All the subjects were asked not to move as much as possible and to keep their eyes closed without falling asleep. Foam pads and headphones were used to reduce head movement and scanner noise. The resting-state fMRI data was collected using echo plane imaging (EPI) with the following parameters: repetition time/echo time ratio = 2000/30 ms, flip angle = 90°, acquisition matrix size = 64 × 64, field of view = 230 × 230, the voxel size = 3.6 mm × 3.6 mm × 3.6 mm, 38 slices with 0.6 mm gap, 240 volumes.
Resting-State fMRI Data Preprocessing
The resting-state fMRI data was preprocessed using the toolkit of DPARSF version 2.3.1 The first 10 volumes were discarded to facilitate magnetization equilibrium effects and were realigned to the first volume to correct head motion. Then, the fMRI images were normalized to EPI template and resampled to 3 mm3 isotropic voxels. Friston 24-parameter of head motion, white matter, cerebrospinal fluid, and whole brain mean signals were regressed out and the fMRI images were filtered a frequency band of 0.01–0.08 Hz. Finally, the voxel-wise static ALFF and dynamic ALFF were calculated.
Static and Dynamic ALFF Calculation
The ALFF was mainly used to characterize the resting-state functional activity of each brain voxel (Zou et al., 2008). To calculate the static ALFF, the time series of each voxel was first transformed to frequency domain for power spectrum calculation. ALFF is computed at the power within the low-frequency range (0.01–0.1 Hz). The dynamic ALFF was calculated using a sliding window method. For dynamic ALFF calculation, the length of sliding window is absence of a standard criterion. To exclude the spurious fluctuations, many previous studies have demonstrated the minimum window length should be larger than 1/fmin, where fmin is the minimum frequency of time series (Leonardi and Van De Ville, 2015; Du et al., 2017; Li et al., 2019). Thus, a window length of 50 TR (100 s) with step size of 5 TR (10 s) as the optimal parameter was applied in this study to keep the balance between capturing a reliable dynamics and to obtain steady correlations between regions for dynamic ALFF calculation. In each window, the ALFF map was computed, and the variance of the ALFF maps across all the windows was used to measure the dynamic. Finally, the static and dynamic ALFF maps were normalized to z-scores and smoothed with 6 mm Gaussian kernel for statistical analyses.
Statistical and Correlation Analyses
Two-sample t-tests with MoCA, HAMD, and HAMA as covariates were performed to compare the static and dynamic ALFF maps between healthy controls and CDP patients. The significance was determined using a Gaussian random field (GRF) correction method with p < 0.05.
To explore the relationship between the static and dynamic ALFF values and clinical measurements, correlation analyses were performed between the regional mean static and dynamic ALFF values and VAS, MoCA, HAMD, and HAMA scores in CDP patients. The significance was set at p < 0.05 with Bonferroni correction.
Results
Clinical Characteristics
There were no significant differences in age (p = 0.15) between CDP patients and healthy controls (Table 1). There were significantly decreased MoCA and increased HAMD, HAMA scores in CDP patients compared to healthy controls (Table 1).
Changed Static ALFF
Static ALFF analyses identified increased ALFF in the left insula (INS) and the left precuneus (PCu), and decreased ALFF in left precentral/postcentral gyrus (PreCG/PoCG), thalamus (THA), and subgenual anterior cingulate cortex (sgACC) in CDP patients compared to healthy controls (Table 2 and Figure 1).
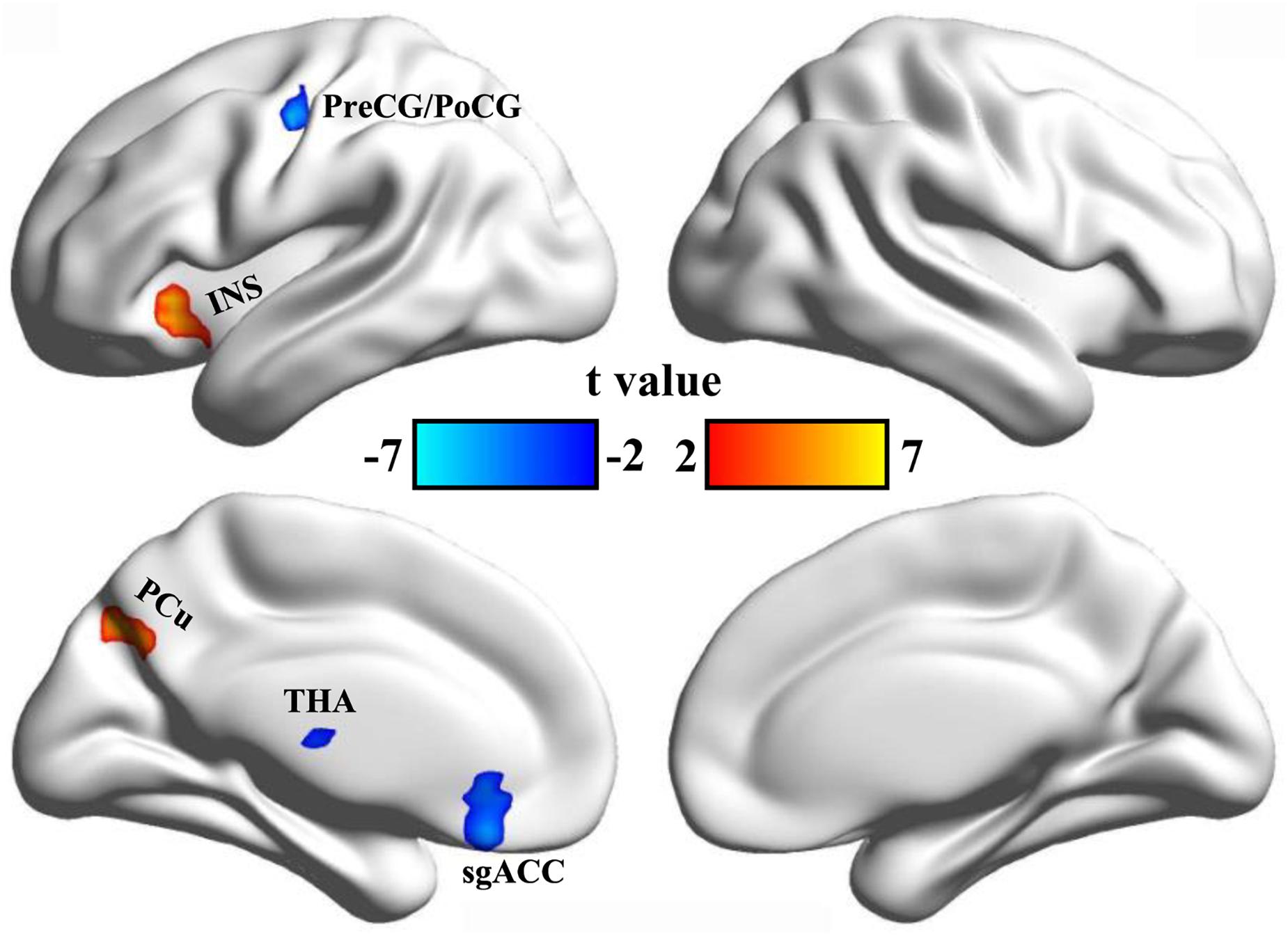
Figure 1. Changed static ALFF in CDP patients. A two-sample t-test was used to compare the static ALFF maps between CDP patients and healthy controls. Significantly increased static ALFF in left anterior insula and left posterior precuneus were found in CDP patients. Moreover, significant reeducations of static ALFF in left precentral/postcentral gyrus, subgenual anterior cingulate cortex, and thalamus were found in CDP patients.
Changed Dynamic ALFF
Using dynamic ALFF analysis, we found decreased dynamic ALFF in the left PreCG/PoCG, the right posterior middle temporal gyrus (MTG), and the bilateral THA in CDP patients compared to healthy controls (Table 2 and Figure 2).
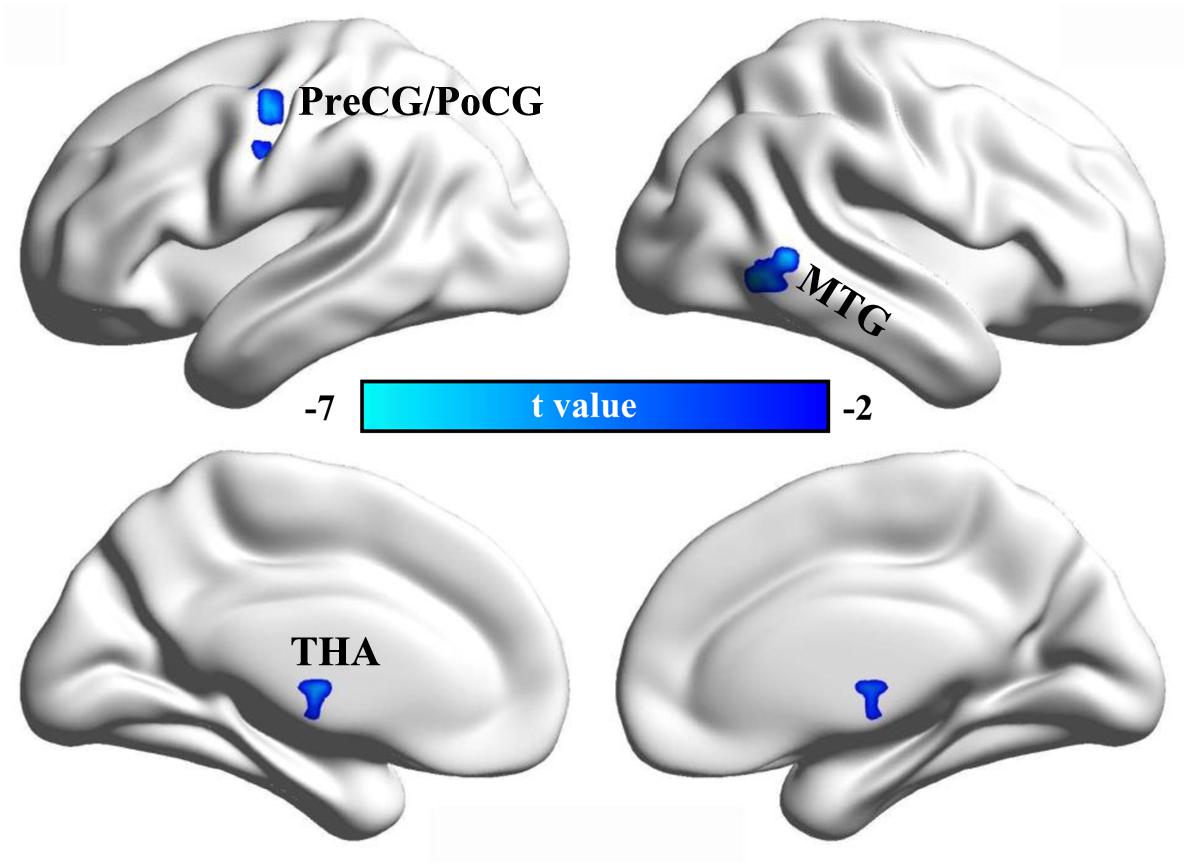
Figure 2. Changed dynamic ALFF in CDP patients. A two-sample t-test was used to compare the dynamic ALFF maps between CDP patients and healthy controls. Significantly decreased dynamic ALFF in left precentral/postcentral gyrus, right posterior middle temporal gyrus, and bilateral thalamus were found in CDP patients.
Correlation Analyses
Correlation analyses identified negative correlations between static ALFF in left PreCG/PoCG and VAS scores (r = −0.417, p = 0.0074), and between dynamic ALFF in left THA and disease duration (r = −0.494, p = 0.0012) in CDP patients (Figure 3).
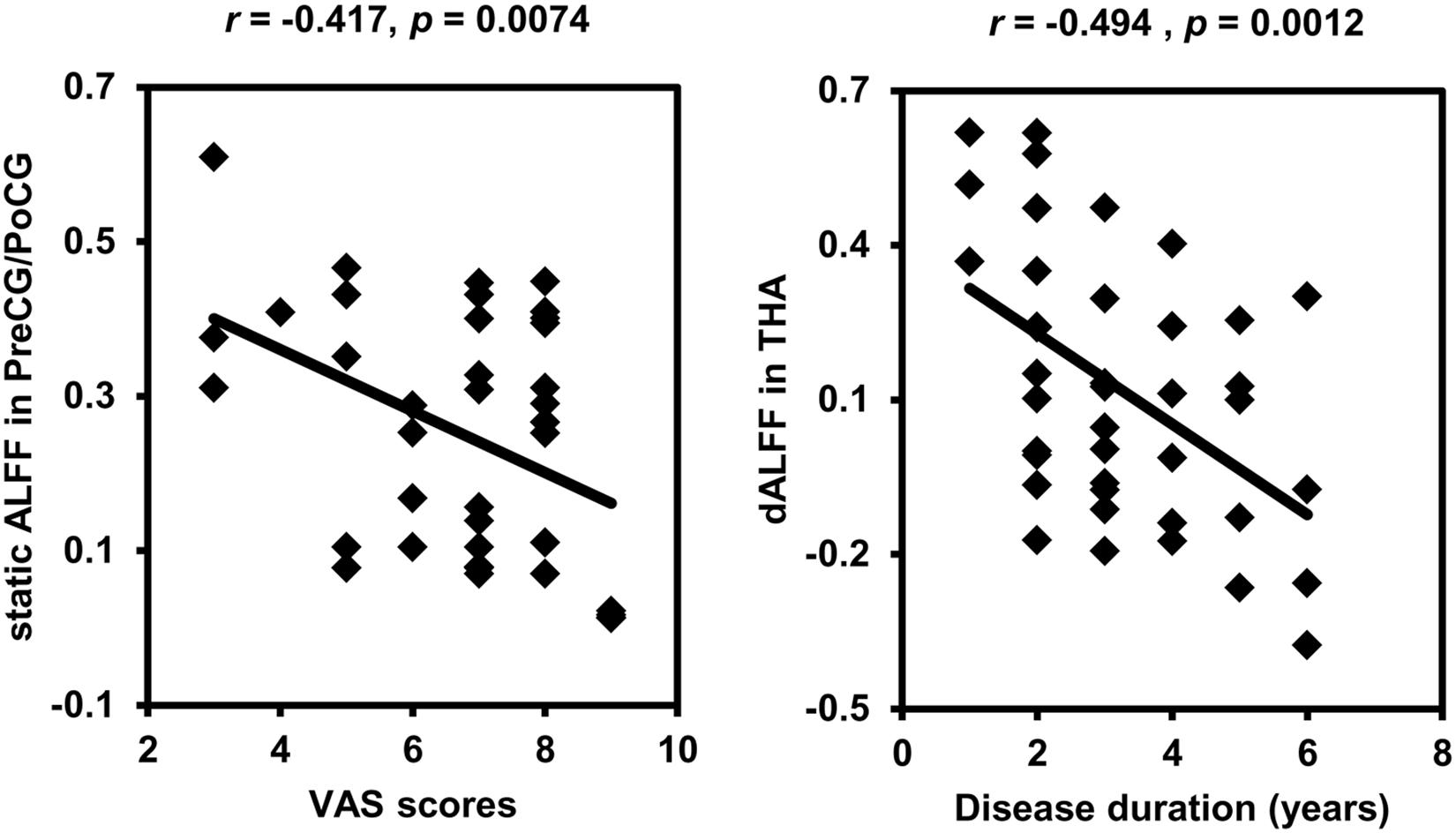
Figure 3. Correlation analyses between static, dynamic ALFF and clinical characteristics. Significant correlations were found between the static ALFF in left precentral/postcentral gyrus and VAS scores and between the dynamic ALFF in left thalamus and disease duration in CDP patients.
Discussion
In this study, we identified changed static and dynamic ALFF in insula, precentral/postcentral gyrus, precuneus, posterior middle temporal gyrus, and bilateral thalamus in CDP patients. Moreover, the static ALFF in precentral/postcentral gyrus and dynamic ALFF in thalamus were negatively correlated with VAS scores and duration of disease, respectively. Our findings revealed dynamic changes of brain functional activities in CDP patients which provide the neurophysiological basis for CDP.
We found increased static ALFF in left insula and precuneus in CDP patients. The insula is a heterogeneous area and is important for processing of emotion and pain perception (Dosenbach et al., 2007; Thielscher and Pessoa, 2007; Kelly et al., 2012). The insula is a key part of the pain matrix and trigger the pain matrix network for subjective pain experience (Taylor et al., 2009; Isnard et al., 2011; Favilla et al., 2014). The insula is also critical for emotional processing and pain memory (Gasquoine, 2014). A large number of studies have revealed the functional disruption of anterior insula in depression and pain-related diseases (Wang et al., 2017a; Wang J. et al., 2020; Wang Y. et al., 2018, 2020; Wang L. et al., 2020). The posterior precuneus mainly participates in visual and memory processing (Zhang et al., 2014; Wang et al., 2019). Thus, increased ALFF in anterior insula and posterior precuneus may be a compensatory mechanism and is related to elevated pain memory and abnormal emotion and visuospatial attention processing in CDP patients.
We also found decreased static and dynamic ALFF in sensorimotor network including precentral/postcentral gyrus and bilateral thalamus (Otti et al., 2013). This finding was supported by previous studies in cervical spondylosis and chronic knee osteoarthritis (Liao et al., 2018; Woodworth et al., 2019). The sensorimotor network is involved in processing changing pain intensity and participates in discrimination of the sensory components of pain perception (Kanda et al., 2000; Peyron et al., 2000). The pain intensity processing of SMN network was supported by our correlation analysis between the static ALFF in precentral/postcentral gyrus and VAS scores. The thalamus is a key gate for processing sensory and motor signals and is as a hub for information exchanges between different subcortical areas and the cerebral cortex (Hwang et al., 2017; Lipiec and Wisniewska, 2019; Tang et al., 2020). It is also plays an important role in regulation of consciousness (Steriade and Llinas, 1988; Redinbaugh et al., 2020). The important role of thalamus in pain processing was further supported by the correlation analysis of the dynamic ALFF and disease duration in our study. In a word, all these findings demonstrated the disrupted low frequency oscillation of SMN network may be a basic neuropathology of CDP.
Moreover, we found decreased static ALFF in left subgenual anterior cingulate cortex and decreased dynamic ALFF in right posterior MTG in CDP patients. The posterior MTG has been reported to be involved in social cognitive and language processing (Xu et al., 2015, 2019a). The disrupted functional connectivity pattern of the posterior MTG is closely associated the autism spectrum disorder (Xu et al., 2019b). The sgACC is a key region for emotion, reward and decision making processing (Johansen-Berg et al., 2008), and the structural abnormality has been identified in patients with chronic knee osteoarthritis pain (Liao et al., 2018). Functional impairments of sgACC has also been widely observed in depression (Drevets et al., 1998, 2008; Botteron et al., 2002; Wu et al., 2016). Therefore, the decreased ALFF in the two areas may suggest the abnormal reward, emotion, and social cognition processing in CDP patients.
This study has several limitations. First, the selection of the sliding window length remains a topic of debate, and optimal length for obtaining the dynamics of brain activity is unclear. We selected 50 TR as window length on the basis of the criteria that the minimum length should be larger than 1/f min, which was proposed by previous studies (Leonardi and Van De Ville, 2015; Li et al., 2019). The results of different sliding window lengths were similar to the main results of 50 TR, demonstrating that our findings of dALFF variability were relatively stable. Second, given the high comorbidity of anxiety and depression, excluding individuals with depressive disorder may decrease the generalizability of our findings. More comorbid samples are required to replicate and complement our findings.
There are several limitations. First, how to select the length of sliding window is still an open problem, which is a main drawback of dynamic method. Second, all the patients took medications, which may affect the current findings. Third, the samples is still small, the future studies with a larger samples are needed.
Conclusion
Our current study revealed the static and dynamic changes of low-frequency oscillation in CDP patients. We identified increased ALFF in insula and posterior precuneus which may reflect the elevated pain memory. Moreover, we found the decreased ALFF in sensorimotor, social cognition and emotional networks indicating that chronic pain will result functional impairments in emotion, sensorimotor and social cognition. Our findings further demonstrated that combing static and dynamic analyses can provide complementary evidence for understand the neuropathology of CDP.
Data Availability Statement
The raw data supporting the conclusions of this article will be made available by the authors, without undue reservation.
Ethics Statement
The studies involving human participants were reviewed and approved by the Affiliated Xi’an Central Hospital of Xi’an Jiaotong University. The patients/participants provided their written informed consent to participate in this study.
Author Contributions
All authors listed have made a substantial, direct and intellectual contribution to the work, and approved it for publication.
Funding
This work was supported by grants from the Shanxi Key Science and Technology project of Social Development (No. 2016SF-129).
Conflict of Interest
The authors declare that the research was conducted in the absence of any commercial or financial relationships that could be construed as a potential conflict of interest.
Footnotes
References
Bai, T., Wei, Q., Xie, W., Wang, A., Wang, J., Ji, G. J., et al. (2018). Hippocampal-subregion functional alterations associated with antidepressant effects and cognitive impairments of electroconvulsive therapy. Psychol. Med. 49, 1357–1364. doi: 10.1017/s0033291718002684
Bai, T., Wei, Q., Zu, M., Xie, W., Wang, J., Gong-Jun, J., et al. (2019). Functional plasticity of the dorsomedial prefrontal cortex in depression reorganized by electroconvulsive therapy: validation in two independent samples. Hum. Brain Mapp. 40, 465–473. doi: 10.1002/hbm.24387
Biswal, B., Yetkin, F. Z., Haughton, V. M., and Hyde, J. S. (1995). Functional connectivity in the motor cortex of resting human brain using echo-planar MRI. Magn. Reson. Med. 34, 537–541. doi: 10.1002/mrm.1910340409
Botteron, K. N., Raichle, M. E., Drevets, W. C., Heath, A. C., and Todd, R. D. (2002). Volumetric reduction in left subgenual prefrontal cortex in early onset depression. Biol. Psychiatry 51, 342–344. doi: 10.1016/s0006-3223(01)01280-x
Denkinger, M. D., Lukas, A., Nikolaus, T., Peter, R., and Franke, S. (2014). Multisite pain, pain frequency and pain severity are associated with depression in older adults: results from the ActiFE Ulm study. Age Ageing 43, 510–514. doi: 10.1093/ageing/afu013
Dosenbach, N. U., Fair, D. A., Miezin, F. M., Cohen, A. L., Wenger, K. K., Dosenbach, R. A., et al. (2007). Distinct brain networks for adaptive and stable task control in humans. Proc. Natl. Acad. Sci. U.S.A. 104, 11073–11078. doi: 10.1073/pnas.0704320104
Drevets, W. C., Ongur, D., and Price, J. L. (1998). Neuroimaging abnormalities in the subgenual prefrontal cortex: implications for the pathophysiology of familial mood disorders. Mol. Psychiatry 3, 190–221.
Drevets, W. C., Savitz, J., and Trimble, M. (2008). The subgenual anterior cingulate cortex in mood disorders. CNS Spectr. 13, 663–681. doi: 10.1017/s1092852900013754
Du, Y., Pearlson, G. D., Lin, D., Sui, J., Chen, J., Salman, M., et al. (2017). Identifying dynamic functional connectivity biomarkers using GIG-ICA: application to schizophrenia, schizoaffective disorder, and psychotic bipolar disorder. Hum. Brain Mapp. 38, 2683–2708. doi: 10.1002/hbm.23553
Favilla, S., Huber, A., Pagnoni, G., Lui, F., Facchin, P., Cocchi, M., et al. (2014). Ranking brain areas encoding the perceived level of pain from fMRI data. Neuroimage 90, 153–162. doi: 10.1016/j.neuroimage.2014.01.001
Fox, M. D., Corbetta, M., Snyder, A. Z., Vincent, J. L., and Raichle, M. E. (2006). Spontaneous neuronal activity distinguishes human dorsal and ventral attention systems. Proc. Natl. Acad. Sci. U.S.A. 103, 10046–10051. doi: 10.1073/pnas.0604187103
Fox, M. D., and Raichle, M. E. (2007). Spontaneous fluctuations in brain activity observed with functional magnetic resonance imaging. Nat. Rev. Neurosci. 8, 700–711. doi: 10.1038/nrn2201
Fu, Z., Tu, Y., Di, X., Du, Y., Pearlson, G. D., Turner, J. A., et al. (2018). Characterizing dynamic amplitude of low-frequency fluctuation and its relationship with dynamic functional connectivity: an application to schizophrenia. Neuroimage 180, 619–631. doi: 10.1016/j.neuroimage.2017.09.035
Gao, Z., Guo, X., Liu, C., Mo, Y., and Wang, J. (2020). Right inferior frontal gyrus: an integrative hub in tonal bilinguals. Hum. Brain Mapp. 41, 2152–2159. doi: 10.1002/hbm.24936
Gasquoine, P. G. (2014). Contributions of the insula to cognition and emotion. Neuropsychol. Rev. 24, 77–87. doi: 10.1007/s11065-014-9246-9
Greicius, M. D., Krasnow, B., Reiss, A. L., and Menon, V. (2003). Functional connectivity in the resting brain: a network analysis of the default mode hypothesis. Proc. Natl. Acad. Sci. U.S.A. 100, 253–258. doi: 10.1073/pnas.0135058100
Hwang, K., Bertolero, M. A., Liu, W. B., and D’Esposito, M. (2017). The human thalamus is an integrative hub for functional brain networks. J. Neurosci. 37, 5594–5607. doi: 10.1523/jneurosci.0067-17.2017
Isnard, J., Magnin, M., Jung, J., Mauguiere, F., and Garcia-Larrea, L. (2011). Does the insula tell our brain that we are in pain? Pain 152, 946–951. doi: 10.1016/j.pain.2010.12.025
Johansen-Berg, H., Gutman, D. A., Behrens, T. E., Matthews, P. M., Rushworth, M. F., Katz, E., et al. (2008). Anatomical connectivity of the subgenual cingulate region targeted with deep brain stimulation for treatment-resistant depression. Cereb. Cortex 18, 1374–1383. doi: 10.1093/cercor/bhm167
Kanda, M., Nagamine, T., Ikeda, A., Ohara, S., Kunieda, T., Fujiwara, N., et al. (2000). Primary somatosensory cortex is actively involved in pain processing in human. Brain Res. 853, 282–289. doi: 10.1016/s0006-8993(99)02274-x
Kelly, C., Toro, R., Di Martino, A., Cox, C. L., Bellec, P., Castellanos, F. X., et al. (2012). A convergent functional architecture of the insula emerges across imaging modalities. Neuroimage 61, 1129–1142. doi: 10.1016/j.neuroimage.2012.03.021
Leonardi, N., and Van De Ville, D. (2015). On spurious and real fluctuations of dynamic functional connectivity during rest. Neuroimage 104, 430–436. doi: 10.1016/j.neuroimage.2014.09.007
Li, C., Xia, L., Ma, J., Li, S., Liang, S., Ma, X., et al. (2019). Dynamic functional abnormalities in generalized anxiety disorders and their increased network segregation of a hyperarousal brain state modulated by insomnia. J. Affect. Disord. 246, 338–345. doi: 10.1016/j.jad.2018.12.079
Liao, X., Mao, C., Wang, Y., Zhang, Q., Cao, D., Seminowicz, D. A., et al. (2018). Brain gray matter alterations in Chinese patients with chronic knee osteoarthritis pain based on voxel-based morphometry. Medicine 97:e0145. doi: 10.1097/md.0000000000010145
Linton, S. J. (2013). A transdiagnostic approach to pain and emotion. J. Appl. Biobehav. Res. 18, 82–103. doi: 10.1111/jabr.12007
Lipiec, M. A., and Wisniewska, M. B. (2019). We have to find a way - growth and guidance of thalamocortical axons. Postepy Biochem. 65, 135–142.
Liu, C., Dai, Z., Zhang, R., Zhang, M., Hou, Y., Qi, Z., et al. (2015). Mapping intrinsic functional brain changes and repetitive transcranial magnetic stimulation neuromodulation in idiopathic restless legs syndrome: a resting-state functional magnetic resonance imaging study. Sleep Med. 16, 785–791. doi: 10.1016/j.sleep.2014.12.029
Montero-Homs, J. (2009). [Nocioceptive pain, neuropathic pain and pain memory]. Neurologia 24, 419–422.
Otti, A., Guendel, H., Henningsen, P., Zimmer, C., Wohlschlaeger, A. M., and Noll-Hussong, M. (2013). Functional network connectivity of pain-related resting state networks in somatoform pain disorder: an exploratory fMRI study. J. Psychiatry Neurosci. 38, 57–65. doi: 10.1503/jpn.110187
Peng, H., Zheng, H., Li, L., Liu, J., Zhang, Y., Shan, B., et al. (2012). High-frequency rTMS treatment increases white matter FA in the left middle frontal gyrus in young patients with treatment-resistant depression. J. Affect. Disord. 136, 249–257. doi: 10.1016/j.jad.2011.12.006
Peyron, R., Laurent, B., and Garcia-Larrea, L. (2000). Functional imaging of brain responses to pain. A review and meta-analysis. Neurophysiol. Clin. 30, 263–288. doi: 10.1016/s0987-7053(00)00227-6
Redinbaugh, M. J., Phillips, J. M., Kambi, N. A., Mohanta, S., Andryk, S., Dooley, G. L., et al. (2020). Thalamus modulates consciousness via layer-specific control of cortex. Neuron 106, 66–75.e12. doi: 10.1016/j.neuron.2020.01.005
Steriade, M., and Llinas, R. R. (1988). The functional states of the thalamus and the associated neuronal interplay. Physiol. Rev. 68, 649–742. doi: 10.1152/physrev.1988.68.3.649
Sun, H., Luo, L., Yuan, X., Zhang, L., He, Y., Yao, S., et al. (2018). Regional homogeneity and functional connectivity patterns in major depressive disorder, cognitive vulnerability to depression and healthy subjects. J. Affect. Disord. 235, 229–235. doi: 10.1016/j.jad.2018.04.061
Tang, Y., Wang, M., Zheng, T., Yuan, F., Yang, H., Han, F., et al. (2020). Grey matter volume alterations in trigeminal neuralgia: a systematic review and meta-analysis of voxel-based morphometry studies. Prog. Neuropsychopharmacol. Biol. Psychiatry 98:109821. doi: 10.1016/j.pnpbp.2019.109821
Taylor, K. S., Seminowicz, D. A., and Davis, K. D. (2009). Two systems of resting state connectivity between the insula and cingulate cortex. Hum. Brain Mapp. 30, 2731–2745. doi: 10.1002/hbm.20705
Thielscher, A., and Pessoa, L. (2007). Neural correlates of perceptual choice and decision making during fear-disgust discrimination. J. Neurosci. 27, 2908–2917. doi: 10.1523/jneurosci.3024-06.2007
Thoomes, E. J., Scholten-Peeters, G. G., de Boer, A. J., Olsthoorn, R. A., Verkerk, K., Lin, C., et al. (2012). Lack of uniform diagnostic criteria for cervical radiculopathy in conservative intervention studies: a systematic review. Eur. Spine J. 21, 1459–1470. doi: 10.1007/s00586-012-2297-9
Tracy, J. A., and Bartleson, J. D. (2010). Cervical spondylotic myelopathy. Neurologist 16, 176–187.
Wang, C., Wu, H., Chen, F., Xu, J., Li, H., Li, H., et al. (2017a). Disrupted functional connectivity patterns of the insula subregions in drug-free major depressive disorder. J. Affect. Disord. 234, 297–304. doi: 10.1016/j.jad.2017.12.033
Wang, J., Becker, B., Wang, L., Li, H., Zhao, X., and Jiang, T. (2019). Corresponding anatomical and coactivation architecture of the human precuneus showing similar connectivity patterns with macaques. Neuroimage 200, 562–574. doi: 10.1016/j.neuroimage.2019.07.001
Wang, J., Wei, Q., Bai, T., Zhou, X., Sun, H., Becker, B., et al. (2017b). Electroconvulsive therapy selectively enhanced feedforward connectivity from fusiform face area to amygdala in major depressive disorder. Soc. Cogn. Affect. Neurosci. 12, 1983–1992. doi: 10.1093/scan/nsx100
Wang, J., Wei, Q., Yuan, X., Jiang, X., Xu, J., Zhou, X., et al. (2017c). Local functional connectivity density is closely associated with the response of electroconvulsive therapy in major depressive disorder. J. Affect. Disord. 225, 658–664. doi: 10.1016/j.jad.2017.09.001
Wang, J., Xie, S., Guo, X., Becker, B., Fox, P. T., Eickhoff, S. B., et al. (2017d). Correspondent functional topography of the human left inferior parietal lobule at rest and under task revealed using resting-state fMRI and Coactivation Based Parcellation. Hum. Brain Mapp. 38, 1659–1675. doi: 10.1002/hbm.23488
Wang, L., Wei, Q., Wang, C., Xu, J., Wang, K., Tian, Y., et al. (2020). Altered functional connectivity patterns of insular subregions in major depressive disorder after electroconvulsive therapy. Brain Imaging Behav. 14, 753–761. doi: 10.1007/s11682-018-0013-z
Wang, L., Yu, L., Wu, F., Wu, H., and Wang, J. (2019). Altered whole brain functional connectivity pattern homogeneity in medication-free major depressive disorder. J. Affect. Disord. 253, 18–25. doi: 10.1016/j.jad.2019.04.040
Wang, J., Ji, Y., Li, X., He, Z., Wei, Q., Bai, T., et al. (2020). Improved and residual functional abnormalities in major depressive disorder after electroconvulsive therapy. Prog. Neuropsychopharmacol. Biol. Psychiatry 100:109888. doi: 10.1016/j.pnpbp.2020.109888
Wang, J., Wei, Q., Wang, L., Zhang, H., Bai, T., Cheng, L., et al. (2018). Functional reorganization of intra- and internetwork connectivity in major depressive disorder after electroconvulsive therapy. Hum. Brain Mapp. 39, 1403–1411. doi: 10.1002/hbm.23928
Wang, J., Yang, Y., Fan, L., Xu, J., Li, C., Liu, Y., et al. (2015). Convergent functional architecture of the superior parietal lobule unraveled with multimodal neuroimaging approaches. Hum. Brain Mapp. 36, 238–257. doi: 10.1002/hbm.22626
Wang, Y., Zhang, Y., Zhang, J., Wang, J., Xu, J., Li, J., et al. (2018). Structural and functional abnormalities of the insular cortex in trigeminal neuralgia: a multimodal magnetic resonance imaging analysis. Pain 159, 507–514. doi: 10.1097/j.pain.0000000000001120
Woodworth, D. C., Holly, L. T., Mayer, E. A., Salamon, N., and Ellingson, B. M. (2019). Alterations in cortical thickness and subcortical volume are associated with neurological symptoms and neck pain in patients with cervical spondylosis. Neurosurgery 84, 588–598. doi: 10.1093/neuros/nyy066
Woodworth, D. C., Holly, L. T., Salamon, N., and Ellingson, B. M. (2018). Resting-state functional magnetic resonance imaging connectivity of the brain is associated with altered sensorimotor function in patients with cervical spondylosis. World Neurosurg. 119, e740–e749. doi: 10.1016/j.wneu.2018.07.257
Wu, H., Sun, H., Xu, J., Wu, Y., Wang, C., Xiao, J., et al. (2016). Changed Hub and corresponding functional connectivity of subgenual anterior cingulate cortex in major depressive disorder. Front. Neuroanat. 10:120. doi: 10.3389/fnana.2016.00120
Xu, J., Lyu, H., Li, T., Xu, Z., Fu, X., Jia, F., et al. (2019a). Delineating functional segregations of the human middle temporal gyrus with resting-state functional connectivity and coactivation patterns. Hum. Brain Mapp. 40, 5159–5171. doi: 10.1002/hbm.24763
Xu, J., Wang, J., Fan, L., Li, H., Zhang, W., Hu, Q., et al. (2015). Tractography-based Parcellation of the Human Middle Temporal Gyrus. Sci. Rep. 5:18883.
Xu, J., Wang, C., Xu, Z., Li, T., Chen, F., Chen, K., et al. (2019b). Specific functional connectivity patterns of middle temporal gyrus subregions in children and adults with autism spectrum disorder. Autism Res. 13, 410–422. doi: 10.1002/aur.2239
Yu, C. X., Ji, T. T., Song, H., Li, B., Han, Q., Li, L., et al. (2017). Abnormality of spontaneous brain activities in patients with chronic neck and shoulder pain: a resting-state fMRI study. J. Int. Med. Res. 45, 182–192. doi: 10.1177/0300060516679345
Zang, Y. F., He, Y., Zhu, C. Z., Cao, Q. J., Sui, M. Q., Liang, M., et al. (2007). Altered baseline brain activity in children with ADHD revealed by resting-state functional MRI. Brain Dev. 29, 83–91. doi: 10.1016/j.braindev.2006.07.002
Zhang, Y., Fan, L., Zhang, Y., Wang, J., Zhu, M., Zhang, Y., et al. (2014). Connectivity-based parcellation of the human posteromedial cortex. Cereb. Cortex 24, 719–727. doi: 10.1093/cercor/bhs353
Keywords: cervical discogenic pain, ALFF, resting-state, static, dynamic
Citation: Ma M, Zhang H, Liu R, Liu H, Yang X, Yin X, Chen S and Wu X (2020) Static and Dynamic Changes of Amplitude of Low-Frequency Fluctuations in Cervical Discogenic Pain. Front. Neurosci. 14:733. doi: 10.3389/fnins.2020.00733
Received: 16 May 2020; Accepted: 22 June 2020;
Published: 14 July 2020.
Edited by:
Jiaojian Wang, University of Electronic Science and Technology of China, ChinaReviewed by:
Bochao Cheng, West China Second University Hospital, Sichuan University, ChinaJinping Xu, Shenzhen Institutes of Advanced Technology (CAS), China
Copyright © 2020 Ma, Zhang, Liu, Liu, Yang, Yin, Chen and Wu. This is an open-access article distributed under the terms of the Creative Commons Attribution License (CC BY). The use, distribution or reproduction in other forums is permitted, provided the original author(s) and the copyright owner(s) are credited and that the original publication in this journal is cited, in accordance with accepted academic practice. No use, distribution or reproduction is permitted which does not comply with these terms.
*Correspondence: Mingyue Ma, mmy_2005@163.com; Song Chen, cyc750818@163.com; Xiaoping Wu, szping518@163.com
†These authors have contributed equally to this work