- 1School of Psychological Sciences – Sagol School of Neuroscience, Tel Aviv University, Tel Aviv, Israel
- 2Department of Radiology and Biomedical Research Imaging Center, School of Medicine, University of North Carolina at Chapel Hill, Chapel Hill, NC, United States
- 3Center for Brain Functions, Institute of Pain Medicine, Tel Aviv Sourasky Medical Center, Tel Aviv, Israel
- 4Sackler Faculty of Medicine, Tel Aviv University, Tel Aviv, Israel
Motor performance varies substantially between individuals. This variance is rooted in individuals’ innate motor abilities, and should thus have a neural signature underlying these differences in behavior. Could these individual differences be detectable with neural measurements acquired at rest? Here, we tested the hypothesis that motor performance can be predicted by resting motor-system functional connectivity and motor-evoked-potentials (MEPs) induced by non-invasive brain stimulation. Twenty healthy right handed subjects performed structural and resting-state fMRI scans. On a separate day, MEPs were measured using transcranial magnetic stimulation (TMS) over the contrateral primary motor cortex (M1). At the end of the session, participants performed a finger-tapping task using their left non-dominant hand. Resting-state functional connectivity between the contralateral M1 and the supplementary motor area (SMA) predicted motor task performance, indicating that individuals with stronger resting M1-SMA functional connectivity exhibit better motor performance. This prediction was neither improved nor reduced by the addition of corticospinal excitability to the model. These results confirm that motor behavior can be predicted from neural measurements acquired prior to task performance, primarily relying on resting functional connectivity rather than corticospinal excitability. The ability to predict motor performance from resting neural markers, provides an opportunity to identify the extent of successful rehabilitation following neurological damage.
Introduction
Since most of our daily behavior requires efficient and accurate motor function, studying the motor system has been a central focus of neuroscience research. People vary greatly in their motor performance. Upon initial presentation of a motor task, some will exhibit high motor performance from the first attempt, while others might struggle and get better only through extensive practice. Newly acquired motor skills can be directly evaluated through behavioral performance (for example see Karni et al., 1995; Walker et al., 2003; Korman et al., 2007; Kantak et al., 2010; Censor et al., 2014b; de Beukelaar et al., 2014; Herszage and Censor, 2017; Lugassy et al., 2018), which remains a commonly used and robust measure in studies exploring the motor system. As previously argued (Krakauer et al., 2017), although technological improvements enable an extensive exploration of the nervous system, cognitive neuroscience research is highly dependent on behavioral level measurements. Indeed, behavior undoubtedly detects the quality of skill acquisition and improvements in performance. In motor skill tasks (Karni et al., 1995) for example, better skill acquisition is usually observed as a higher number of accurate sequences tapped in a fixed duration.
In parallel, the motor system is frequently evaluated through multiple modalities and scales, including neural measurements. While these measurements can enrich the collected data beyond behavior per se, it might result in even greater variability between measures. Current research lacks the knowledge of a possible integration of data sampled from different measurement levels, limiting the ability to explain individual differences in motor performance.
Could behavior be predicted from an integration of neural measurements acquired prior to task performance? Execution of a motor task requires multiple levels of precise neural processes involving motor planning, motor control and skill acquisition at the central nervous system (Allen et al., 1997; Braitenberg et al., 1997; Muellbacher et al., 2002; Hanakawa et al., 2008; Cohen et al., 2009; Narayana et al., 2014; Gabitov et al., 2016), transformation of the motor command along the corticospinal tract to the peripheral nervous system (Rossini et al., 1994; Di Lazzaro et al., 1998; Groppa et al., 2012), and a correct execution of the movement by the corresponding peripheral muscle.
Correspondingly, motor function can be measured from the central nervous system by assessing functional connectivity within the motor system. This can be achieved through functional MRI scans acquired during resting-state sessions, while measuring the correlation between motor regions of interests (Friston, 1994; Biswal et al., 1995). Such evaluation provides an opportunity to assess the underlying mechanism of motor performance. For example, measurements of functional connectivity before and after motor skill acquisition, showed increased connectivity within the motor system (Taubert et al., 2011). Neuroimaging studies repeatedly show that bilateral primary motor cortex (M1) and the supplementary motor area (SMA) (Perez et al., 2007; Kasess et al., 2008; Dayan and Cohen, 2011) constitute the core motor network not only during active tasks but also during rest, in health and in recovery from stroke (Grefkes et al., 2010; van den Heuvel and Hulshoff Pol, 2010; Kristo et al., 2014).
An additional prominent tool which provides a measurement of the motor system is non-invasive brain stimulation. Transcranial magnetic stimulation (TMS) administered over M1 can induce a movement in subjects’ contralateral hand, known as the motor-evoked-potential (MEP), measured with electromyography (EMG). As such, it reflects the passage of information from the central nervous system toward the peripheral muscle. Single pulse TMS over the M1 in posterior-anterior orientation is known to produce I-waves (Di Lazzaro et al., 1998) which are activated by trans-synaptic corticospinal neurons within M1 (Volz et al., 2014). These signals are thought to reflect the excitability of the underlying motor cortex (Calancie et al., 1999; Hamzei et al., 2006; Di Lazzaro et al., 2008; Hiraoka et al., 2010; Volz et al., 2014; Strigaro et al., 2016), and can be modulated directly via TMS (Volz et al., 2019), or due to motor learning (Tunovic et al., 2014; Ostadan et al., 2016). As such, corticospinal excitability may play an important role in the investigation of the motor system.
While each of the above measurements provides valuable information on the motor system, they are measured at different levels of the motor system, and could thus provide different data sets. A timely goal is to unravel a holistic framework integrating brain and behavior, which could provide the opportunity to unify between multiple levels of analysis. This effort was previously addressed across domains, spanning from molecular to systems neuroscience (Friston, 2010; Love, 2016; Kim et al., 2017). In correspondence with this view, this study aims to integrate three levels of motor system measurements in humans: brain functional connectivity, corticospinal excitability, and behavior. Namely, could subjects’ behavioral performance in a task be predicted from recordings of functional connectivity and corticospinal excitability? Such integration could shed light on individual differences in motor performance, and the neural markers enabling these differences in performance.
Materials and Methods
Subjects
A total of 20 healthy volunteers (8 males and 12 females; mean age = 26.1 ± 0.8 years) participated in the study. Subjects were all right handed. Five additional subjects were excluded from the experiment: three subjects were excluded before receiving TMS due to artifacts in the MRI scans, and 2 subjects stopped their participation during the TMS session due to discomfort. All subjects provided written informed consent and all procedures were in accordance with a protocol approved by the Tel-Aviv Sourasky Medical Center and Tel-Aviv University’s Ethics committees. Musicians and video-gamers (past or present) were excluded from the study, as well as subjects with psychiatric or neurological history. In addition, subjects were required to sleep at least 6 hr before each of the experimental sessions.
Procedure and Task
The study comprised of 2 sessions (see Figure 1). Subjects first underwent an imaging session where resting-state scans were acquired, in which they were instructed to keep their eyes closed and not fall asleep. Then, on a different session, subjects received single pulse TMS over the right M1 (see details below), while measuring their measuring their MEPs. At the end of this session, following the MEP recordings, subjects performed a sequential finger-tapping task, with their non-dominant left hand. During the task, participants were required to repeatedly tap a 5-element sequence of finger movements (4-1-3-2-4 or 1-4-2-3-1, constantly displayed on the screen), for 30 s, as quickly and accurately as possible. Tapping movements were performed using a 4-key response box (Cedrus Lumina LU440) which was placed in front of the subjects at a comfortable distance and height. Response data were collected for offline analysis using Psychtoolbox (Matlab 8.4). During the task, the sequence was displayed at the middle of the screen and remained in this position throughout the task. The number of correct sequences tapped served as the primary behavioral outcome measure, a common and highly replicable end-point measure for performance in motor sequence tasks (Karni et al., 1995; Walker et al., 2003; Korman et al., 2007; Censor et al., 2010, 2014a,2014b; de Beukelaar et al., 2014). The full procedure included two additional rTMS sessions, conducted after session 2, and thus assured no interfering outcomes. rTMS in those sessions was applied over the lateral prefrontal cortex and vertex, designed to probe the role of human prefrontal cortex in successful reinforced skill formation, reported elsewhere (Dayan et al., 2018).
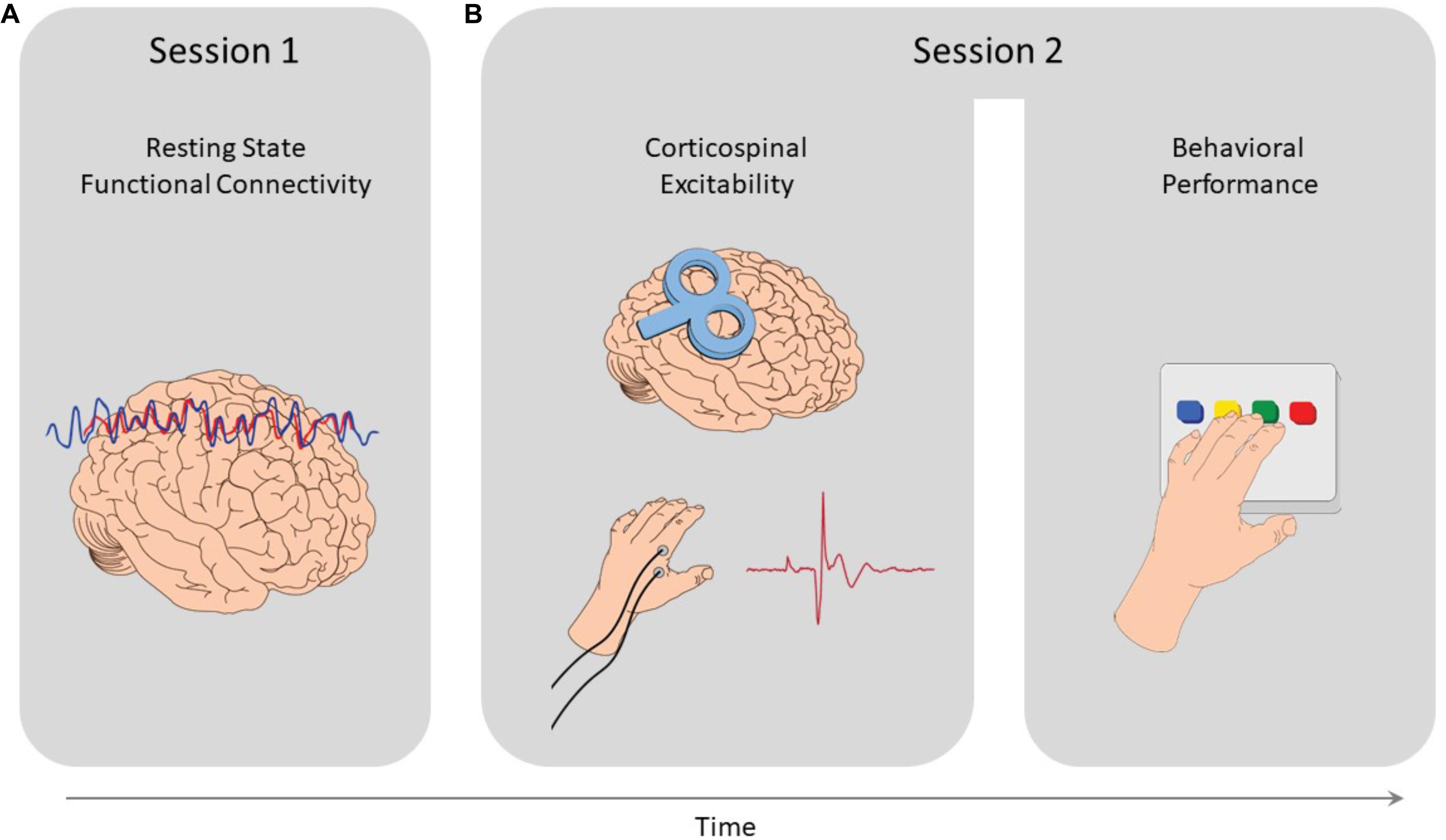
Figure 1. Study design. (A) In the first session resting-state functional MRI scans were acquired, from which functional connectivity measures where extracted. (B) Then, in the second session, MEPs were induced via single pulse TMS applied over the right M1, and measured from the left FDI muscle to quantify corticospinal excitability. At the end of that session, participants performed a sequential finger tapping task, to measure their motor performance.
Non-invasive Brain Stimulation
Transcranial magnetic stimulation was administered using a Magstim® 70 mm double coil, placed over the right hand-knob area of M1, oriented at 45° to the midsagittal line at a posterior-anterior (PA) direction, with interstimulus intervals jittered between 3–4 s. Individual resting motor thresholds (RMT) were defined as the minimal M1 stimulation intensity yielding five out of ten motor-evoked potentials (MEPs) greater than 0.05 mV in the left first dorsal interosseous (FDI) muscle (Rossini et al., 1994). Brainsight® 2 (Rogue Research, Montreal, QC, Canada)1 was used to coregister participants’ head and to mark stimulation sites prior to TMS administration. Four landmarks were used for coregistering the participants’ head to their MRI anatomic scan (nasion, tip of the nose, left and right crus of helix).
Electromyography (EMG)
Electromyography data were measured from the left first dorsal interosseous (FDI) muscle (Rossini et al., 1994), corresponding to the main behavioral task performed with subjects’ non-dominant left hand. MEP recordings of the FDI muscle are the most common measurement for motor corticospinal excitability, and have been reported in previous studies (for example see Di Lazzaro et al., 1998; Tunovic et al., 2014; Volz et al., 2014, along with most of the TMS studies using the finger tapping task, which measured MEP from the FDI to set the motor threshold such as Perez et al., 2007; Censor et al., 2014b; Narayana et al., 2014). Two 10 mm diameter Ag/AgCl surface electrodes were placed on the left FDI muscle, and one additional ground electrode was placed on subjects’ left ulnar tuberosity. MEP data were amplified using a Digitimer D360 amplifier (Digitimer, Welwyn Garden City, United Kingdom) at a gain of 1000×, band-pass filtered 25 Hz to 1 kHz, and notch filtered at 50 Hz. Data were sampled via a Cambridge Electronic Design (CED; Cambridge, United Kingdom) 1401 A/D converter at a rate of 2 kHz and stored on computer using a commercial data collection software (Signal 6.02, CED).
Corticospinal Excitability
Motor-evoked-potentials amplitudes were extracted from each response to single-pulse TMS. Individual recruitment-curves were measured based on MEP amplitudes with increasing stimulation intensities by 10% of the RMT. 12 stimulation pulses were given at 100% RMT, and 6 pulses per each condition of 110%, 120%, 130%, 140%, and 150% of RMT (van der Salm et al., 2009), in a non-randomized order (Wassermann et al., 1998). Recruitment curves were then individually extrapolated as the average amplitude at each intensity and excitability was quantified as the slope of the recruitment curve (Wassermann et al., 1998; Ward et al., 2006; Rosenkranz et al., 2007; Orth et al., 2008; Schippling et al., 2009).
Imaging Data Acquisition
Imaging data were acquired with a 3T SIEMENS MAGNETOM Prisma scanner equipped with a 20-channel head coil at the Wohl Institute for Advanced Imaging, Tel Aviv Sourasky Medical Center. Structural images were acquired with a MPRAGE sequence [repetition time/echo time (TR/TE) = 1860/2.74 ms; flip angle = 8°; field of view (FOV) = 256 mm × 256 mm; slice thickness = 1 mm; 208 axial slices]. Resting-state fMRI images were acquired with a gradient echo-planar imaging (EPI) sequence of functional T2∗-weighted images [TR/TE = 2000/35 ms; flip angle = 90°; field of view (FOV) = 384 mm × 384 mm; slice thickness = 4 mm; 34 interleaved axial slices per volume]. The functional scans comprised a total of 240 volumes which lasted 8 minutes. The first 3 volumes were discarded to account of T1-equilibrium effects. Two subjects (of the total 20) were scanned with different functional parameters [TR/TE = 3000/35 ms; flip angle = 90°; field of view (FOV) = 672 mm × 672 mm; slice thickness = 3 mm; 46 interleaved axial slices per volume].
Imaging Data Analysis
Imaging data analysis was performed with Brain Voyager software (R. Goebel, Brain Innovation, Maastricht, Netherlands). Preprocessing of functional images included realignment and slice-time correction, band-pass filtering (0.01 to 0.1 Hz), segmentation of gray-matter, white matter, and cerebrospinal fluid (CSF) and normalization to the MNI template. The data were additionally spatially smoothed with a Gaussian kernel set at 4 mm full width at half maximum. Signals from the segmented white matter and CSF, and the six motion realignment parameters were regressed out of the signal. Subsequently, reference time courses were extracted from core components of the cortical motor system: the right primary motor cortex (M1) handknob area (each subject’s specific stimulation location, contralateral to the left hand from which behavioral and MEP data was measured, as described above), left primary motor cortex and the SMA, set at MNI (−32, −30, and 51) and (1, −21, and 54) correspondingly (Censor et al., 2014a), each defined as a sphere with radial size of 5 voxels. Correlations between these reference time courses were then calculated for each subject. Only significant correlations were considered for further analysis (p < 0.05 resulting in r > 0.128 for a sample size of n = 237 time points). Accordingly, data from 17/20 subjects in whom there were significant resting-state correlation measurements were included for further behavioral and corticospinal analysis.
Behavioral Data Analysis
Behavioral data were analyzed with SPSS 25 and Matlab 2017a. Behavioral performance was measured by the number of correct sequences tapped, a highly common measure which accounts for both speed and accuracy (Walker et al., 2003; Korman et al., 2007; Censor et al., 2010, 2014b; de Beukelaar et al., 2014; Herszage and Censor, 2017). To test for the relation between functional connectivity and behavior, as well as other pairwise correlations, we computed Pearson’s coefficient. One subject was excluded from the analysis due to extremely high influence value (Cook’s distance = 7.48, see Cook, 1977), hence all of the analyses in this study were conducted with 16 subjects in total. To test for the relation between all three motor measurements (functional connectivity, corticospinal excitability and behavior), a hierarchical multiple regression was conducted with behavioral performance as the dependent variable (Gelman and Hill, 2006).
Results
To test whether behavior can be predicted from resting-state functional connectivity of the motor system, we first calculated Pearson’s correlation coefficients between the two core regions of the cortical motor system, the contralateral M1 and SMA (Perez et al., 2007; Kasess et al., 2008; van den Heuvel and Hulshoff Pol, 2010; Dayan and Cohen, 2011; Kristo et al., 2014). Indeed, functional connectivity between the contralateral M1 and the SMA (see Figure 2A) significantly correlated with task performance (Pearson’s r = 0.64, p < 0.01, see Figure 2B) and accounted for R2 = 40.7% of the variation in participants’ motor behavior. The correlation was not affected by outliers, with Cook’s distance values lower than 0.15, well below the critical threshold of 1.
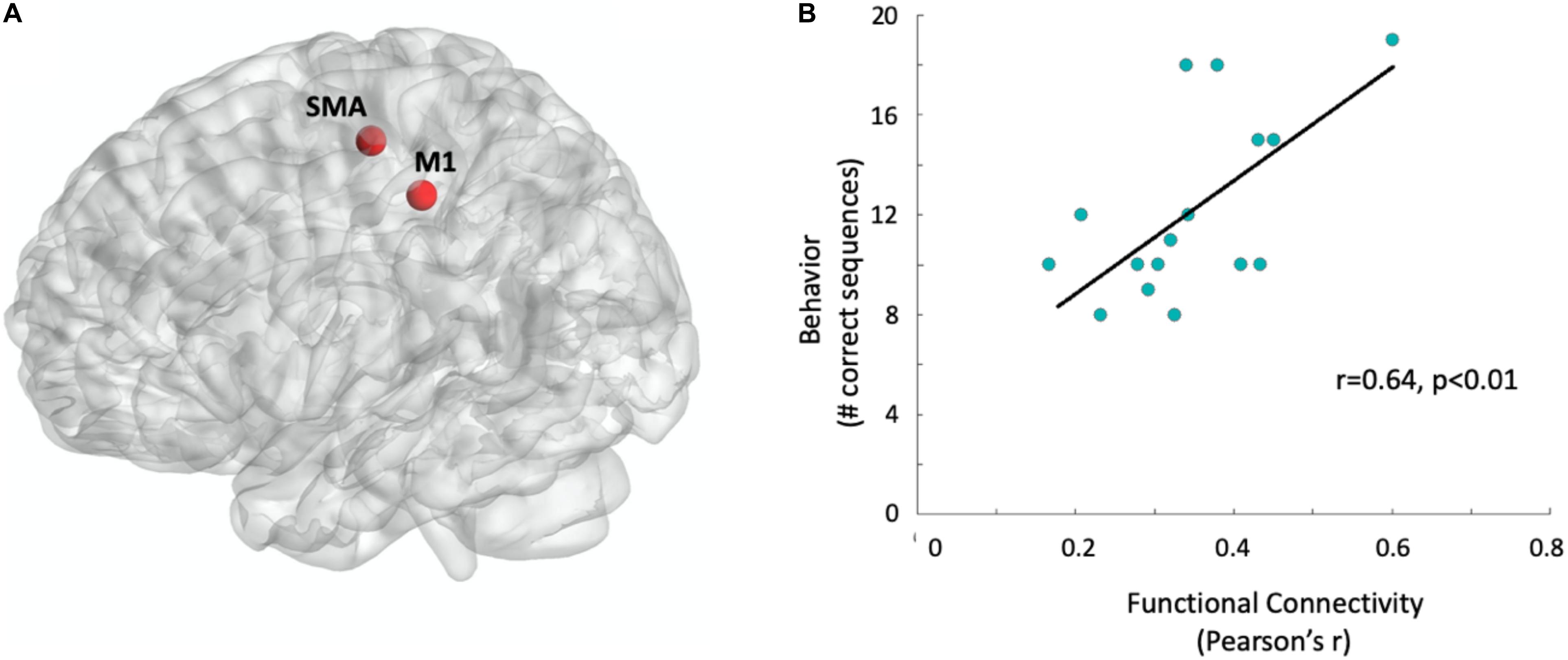
Figure 2. Functional connectivity predicts behavioral performance. (A) Sphere regions-of-interest used for the functional connectivity measurement: Supplementary motor area and right M1, contralateral to the left hand which later performed the motor task (see section “Materials and Methods” for MNI coordinates). Regions-of-interest are visualized with the BrainNetViewer (Xia et al., 2013, http://www.nitrc.org/projects/bnv/). (B) Correlation between behavior and functional connectivity. Data points were jittered at 2% to minimize overlap. The black line represents the single variant regression line.
To further examine the predictability of motor behavior, we conducted a hierarchical multiple regression. This enabled to test whether corticospinal excitability could further improve the prediction model. In accordance with the above result, the first step model included functional connectivity which indeed significantly predicted behavior (F1,14 = 9.63, p < 0.01). Then, at the second stage of the hierarchical regression, corticospinal excitability was added to the model, resulting in a non-significant contribution (R2 change = 2.7%, F1,13 = 0.63, p = 0.44). Nevertheless, the full model could still significantly predict behavior (ANOVA F2,13 = 5.0, p < 0.03, see Figure 3). The coefficients of the full regression model follow the equation:
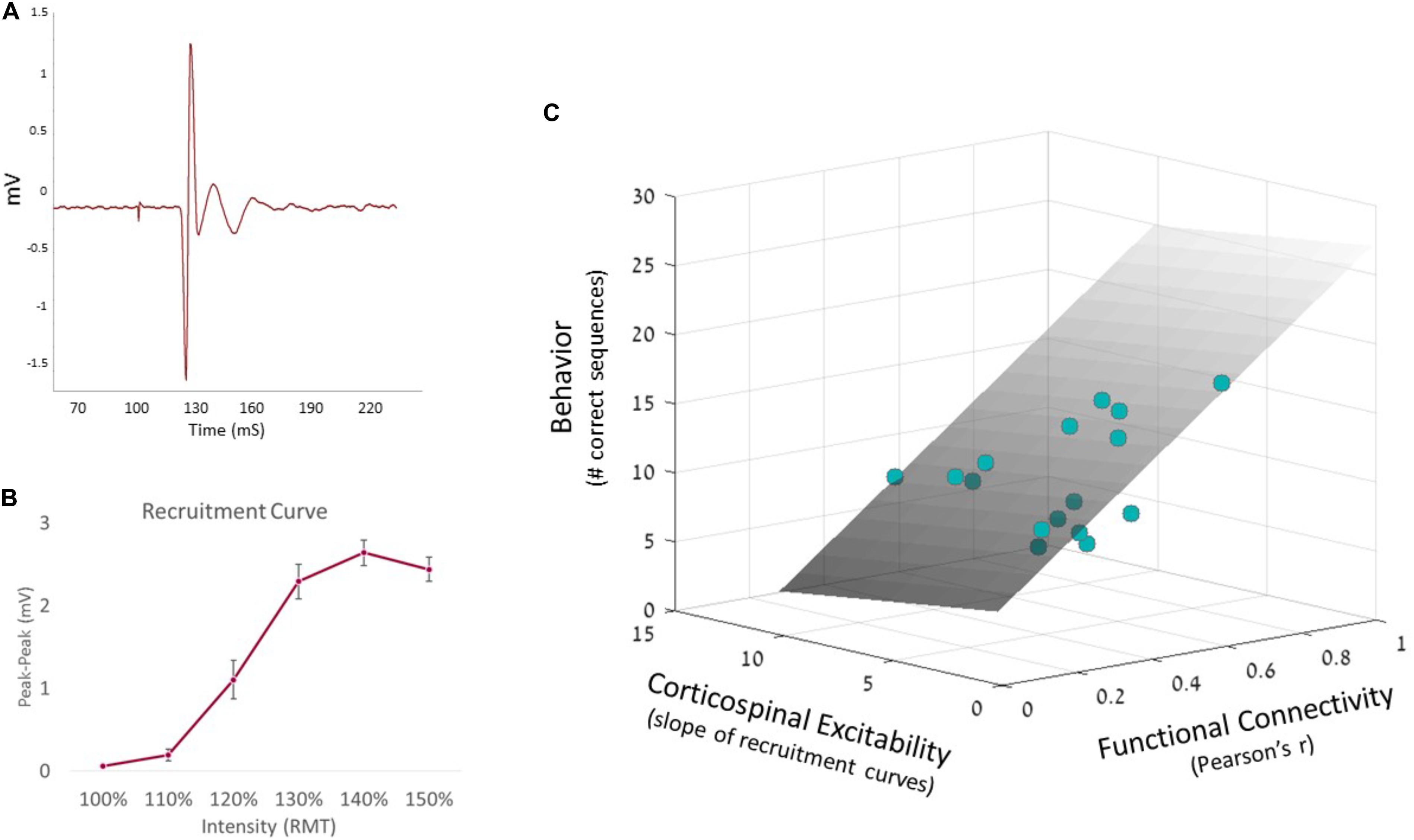
Figure 3. Hierarchical regression model. (A) MEP signal, measured from the FDI muscle via EMG system. (B) Corticospinal excitability recruitment curves were built based on all individual EMG recordings, averaging MEP amplitudes at each intensity. Error bars represent SEM. (C) Predicted behavior is portrayed by the regression plane (gray, see equation above), with the observed single-subject data behavior represented as colored dots. Since the plane is semi-transparent, darker dots represent data points which fall below the plane, while brighter dots represent data points above the plane.
Prior to conducting the hierarchical multiple regression, the relevant assumptions were tested. Hence, the assumption of singularity was met as the independent variables (corticospinal excitability and functional connectivity) were not significantly correlated (Pearson’s r = −0.15, p = 0.57). This was further supported by multicollinearity statistics (variance inflation factor = 1.02 for both predictors) which were all within accepted limits, asserting that the assumption of multicollinearity was met (Coakes, 2007). In addition, corticospinal excitability did not correlate directly with behavioral performance (Pearson’s r = −0.26, p = 0.33), and importantly, an independent two-sample t-test comparing behavior with sequence as between group factor showed no difference in performance between sequences [t(14) = −0.72, p = 0.48], confirming an equivalent level of difficulty for both sequences counter-balanced across participants.
Unimanual motor activity is known to activate connections between bilateral M1, known as inter hemispheric inhibition (IHI; for a review see Perez and Cohen, 2009), in addition to frontal areas (such as the SMA). To rule out effects of IHI per se (rather than innate differences in unimanual performance), we conducted a control analysis which showed that functional connectivity measured between bilateral primary motor cortices (right and left M1) did not significantly contribute to the model (R2 change = 1.2%, F1,12 = 0.27, p = 0.62), and was not correlated directly with performance (Pearson’s r = 0.17, p = 0.26).
Discussion
This study aimed to reveal origins of individual differences in motor skill task performance, by integrating three levels of motor system measurements in humans: brain functional connectivity, corticospinal excitability, and behavior. The results indicate that differences in behavior can be predicted from subjects’ resting-state functional connectivity between the SMA and the contralateral M1, corresponding to the hand performing the task. Specifically, the main finding predicts that individuals with stronger functional connectivity between the contralateral M1 and SMA would exhibit better motor performance. This prediction was not improved nor reduced by corticospinal excitability data, produced from brain stimulation over the same location in contralateral M1.
The main finding of this study, showing that functional connectivity can predict motor performance is in line with previous studies (Mueller et al., 2013; Hamann et al., 2014; Wu et al., 2014). For example, Wu et al. (2014) found that coherence with the region of the left M1 in resting EEG data was associated with motor skill acquisition. In addition, the relation between functional connectivity and behavior was reported in non-motor tasks as well, for example stronger hippocampal connectivity at rest was shown to predict lower episodic memory performance and declining longitudinal memory performance (Salami et al., 2014), while higher hippocampal and posteromedial connectivity at rest predicted better performance in an associative memory task (Wang et al., 2010).
In the current study, resting-state functional connectivity and corticospinal excitability were not significantly correlated. This result is consistent with previous studies investigating the relation between functional connectivity and TMS induced activity (Romei et al., 2007; Fox et al., 2012; Volz et al., 2014; Nettekoven et al., 2015). For example, Volz et al. (2014) reported that functional connectivity did not correlate with MEP latency, another common TMS induced measurement. However, when the TMS coil orientation was changed to an anterior-posterior orientation, different from the orientation used in the current study (see section “Materials and Methods”), Volz et al., found a significant correlation between latency and functional connectivity, indicating that the relation between functional connectivity and TMS induced activity might depend on stimulation parameters.
Corticospinal Excitability did not directly predict behavioral performance in the current study, and indeed, most of the studies linking excitability and behavior focused on time dependent changes in behavior, i.e., learning (for example see Tunovic et al., 2014; Ostadan et al., 2016). Adding to the existing literature, the current results suggest that while corticospinal excitability often changes due to learning processes, it might not be as critical in predicting behavioral performance at early stages of skill acquisition.
Interestingly, even though corticospinal excitability per se was not associated with behavior, a combination of functional connectivity and corticospinal excitability was found to predict behavior. Using this unified combination of all three measurements (functional connectivity, corticospinal excitability, and behavior) links data from different levels of measurement into a merged model that can explain the variability in motor performance.
Pairwise relations between these three measurements of the motor system were previously reported to associate with different clinical conditions. For example, the disruption of functional connectivity due to stroke, was found to predict performance impairment (Carter et al., 2010). Furthermore, Grefkes et al. (2010) demonstrated that in patients following subacute stroke, inhibitory TMS over the M1 of the unaffected hemisphere resulted in behavioral improvements and increased connectivity between ipsilesional SMA and M1. In line with these studies, corticospinal excitability was found to be increased in patients with Alzheimer disease (Alagona et al., 2001). Overall, the link of different diseases with functional connectivity and corticospinal excitability has been reported separately in many studies, pointing to potential clinical application using the combination of both measurements not only to predict motor performance in normal populations, but also for the prediction and detection of clinical conditions.
In sum, the current study shows that behavior can be predicted from individuals’ functional connectivity measures, while highlighting the need for additional research into the predictive combination of resting-state functional connectivity and brain stimulation to explain differences in motor performance. Importantly, the ability to predict motor performance from resting state scans in healthy populations supports the utilization of such measurements for clinical use such as assessment of successful rehabilitation likelihood following stroke.
Data Availability Statement
The datasets generated for this study are available on request to the corresponding author.
Ethics Statement
The studies involving human participants were reviewed and approved by the Tel-Aviv Sourasky Medical Center and the Tel-Aviv University’s Ethics committees. The patients/participants provided their written informed consent to participate in this study.
Author Contributions
JH, ED, HS, and NC designed the study and experimental protocol. JH performed the experiments, collected the data, and analyzed the data. JH, ED, and NC wrote and edited the manuscript.
Funding
This study was supported by the Israel Science Foundation (ISF, Grant 526/17), the United States – Israel Binational Science Foundation (BSF, Grant 2016058), and by a Colton Foundation scholarship to JH.
Conflict of Interest
The authors declare that the research was conducted in the absence of any commercial or financial relationships that could be construed as a potential conflict of interest.
Acknowledgments
We thank Rony Laor-Maayany for the graphic illustrations, and Nadav Stoppelman and Talma Hendler from Tel Aviv Sourasky Medical Center.
Footnotes
References
Alagona, G., Bella, R., Ferri, R., Carnemolla, A., Pappalardo, A., Costanzo, E., et al. (2001). Transcranial magnetic stimulation in Alzheimer disease: motor cortex excitability and cognitive severity. Neurosci. Lett. 314, 57–60.
Allen, G., Buxton, R. B., Wong, E. C., and Courchesne, E. (1997). Attentional activation of the cerebellum independent of motor involvement. Science 275, 1940–1943.
Biswal, B., Yetkin, F. Z., Haughton, V. M., and Hyde, J. S. (1995). Functional connectivity in the motor cortex of resting human brain using echo-planar MRI. Magn. Reson. Med. 34, 537–541.
Braitenberg, V., Heck, D., and Sultan, F. (1997). The detection and generation of sequences as a key to cerebellar function: experiments and theory. Behav. Brain Sci. 20, 229–245.
Calancie, B., Alexeeva, N., Broton, J. G., Suys, S., Hall, A., and Klose, K. J. (1999). Distribution and latency of muscle responses to transcranial magnetic stimulation of motor cortex after spinal cord injury in humans. J. Neurotrauma 16, 49–67. doi: 10.1089/neu.1999.16.49
Carter, A. R., Astafiev, S. V., Lang, C. E., Connor, L. T., Rengachary, J., Strube, M. J., et al. (2010). Resting interhemispheric functional magnetic resonance imaging connectivity predicts performance after stroke. Ann. Neurol. 67, 365–375. doi: 10.1002/ana.21905
Censor, N., Dayan, E., and Cohen, L. G. (2014a). Cortico-subcortical neuronal circuitry associated with reconsolidation of human procedural memories. Cortex 58, 281–288. doi: 10.1016/j.cortex.2013.05.013
Censor, N., Dimyan, M. A., and Cohen, L. G. (2010). Modification of existing human motor memories is enabled by primary cortical processing during memory reactivation. Curr. Biol. 20, 1545–1549. doi: 10.1016/j.cub.2010.07.047
Censor, N., Horovitz, S. G., and Cohen, L. G. (2014b). Interference with Existing Memories Alters Offline Intrinsic Functional Brain Connectivity. Neuron 81, 69–76. doi: 10.1016/j.neuron.2013.10.042
Coakes, S. J. (2007). Analysis Without Anguish: Version 12.0 for Windows. Hoboken, NJ: John Wiley & Sons, Inc.
Cohen, N. R., Cross, E. S., Wymbs, N. F., and Grafton, S. T. (2009). Transient disruption of M1 during response planning impairs subsequent offline consolidation. Exp. Brain Res. 196, 303–309. doi: 10.1007/s00221-009-1838-x
Cook, R. D. (1977). Detection of influential observation in linear regression. Technometrics 19, 15–18.
Dayan, E., and Cohen, L. G. (2011). Neuroplasticity subserving motor skill learning. Neuron 72, 443–454. doi: 10.1016/j.neuron.2011.10.008
Dayan, E., Herszage, J., Laor-Maayany, R., Sharon, H., and Censor, N. (2018). Neuromodulation of reinforced skill learning reveals the causal function of prefrontal cortex. Hum. Brain Mapp 39, 4724–4732. doi: 10.1002/hbm.24317
de Beukelaar, T. T., Woolley, D. G., and Wenderoth, N. (2014). Gone for 60 seconds: Reactivation length determines motor memory degradation during reconsolidation. Cortex 59, 138–145. doi: 10.1016/j.cortex.2014.07.008
Di Lazzaro, V., Oliviero, A., Profice, P., Saturno, E., Pilato, F., Insola, A., et al. (1998). Comparison of descending volleys evoked by transcranial magnetic and electric stimulation in conscious humans. Electroencephalogr. Clin. Neurophysiol. Mot. Control 109, 397–401.
Di Lazzaro, V., Pilato, F., Dileone, M., Profice, P., Capone, F., Ranieri, F., et al. (2008). Modulating cortical excitability in acute stroke: a repetitive TMS study. Clin. Neurophysiol. 119, 715–723. doi: 10.1016/j.clinph.2007.11.049
Fox, M. D., Halko, M. A., Eldaief, M. C., and Pascual-Leone, A. (2012). Measuring and manipulating brain connectivity with resting state functional connectivity magnetic resonance imaging (fcMRI) and transcranial magnetic stimulation (TMS). Neuroimage 62, 2232–2243. doi: 10.1016/j.neuroimage.2012.03.035
Friston, K. (2010). The free-energy principle: a unified brain theory? Nat. Rev. Neurosci. 11:127. doi: 10.1038/nrn2787
Friston, K. J. (1994). Functional and effective connectivity in neuroimaging: a synthesis. Hum. Brain Mapp. 2, 56–78. doi: 10.1002/hbm.460020107
Gabitov, E., Manor, D., and Karni, A. (2016). Learning from the other limb’s experience: sharing the “trained” M1 representation of the motor sequence knowledge. J. Physiol. 594, 169–188. doi: 10.1113/JP270184
Gelman, A., and Hill, J. (2006). Data Analysis Using Regression and Multilevel/Hierarchical Models. Cambridge: Cambridge university press.
Grefkes, C., Nowak, D. A., Wang, L. E., Dafotakis, M., Eickhoff, S. B., and Fink, G. R. (2010). Modulating cortical connectivity in stroke patients by rTMS assessed with fMRI and dynamic causal modeling. Neuroimage 50, 233–242. doi: 10.1016/j.neuroimage.2009.12.029
Groppa, S., Oliviero, A., Eisen, A., Quartarone, A., Cohen, L. G., Mall, V., et al. (2012). A practical guide to diagnostic transcranial magnetic stimulation: report of an IFCN committee. Clin. Neurophysiol. 123, 858–882. doi: 10.1016/j.clinph.2012.01.010
Hamann, J. M., Dayan, E., Hummel, F. C., and Cohen, L. G. (2014). Baseline frontostriatal-limbic connectivity predicts reward-based memory formation. Hum. Brain Mapp. 35, 5921–5931. doi: 10.1002/hbm.22594
Hamzei, F., Liepert, J., Dettmers, C., Weiller, C., and Rijntjes, M. (2006). Two different reorganization patterns after rehabilitative therapy: an exploratory study with fMRI and TMS. Neuroimage 31, 710–720. doi: 10.1016/j.neuroimage.2005.12.035
Hanakawa, T., Dimyan, M. A., and Hallett, M. (2008). Motor planning, imagery, and execution in the distributed motor network: a time-course study with functional MRI. Cereb. Cortex 18, 2775–2788. doi: 10.1093/cercor/bhn036
Herszage, J., and Censor, N. (2017). Memory reactivation enables long-term prevention of interference. Curr. Biol. 27, 1529.e2–1534.e2. doi: 10.1016/j.cub.2017.04.025
Hiraoka, K., Horino, K., Yagura, A., and Matsugi, A. (2010). Cerebellar TMS evokes a long latency motor response in the hand during a visually guided manual tracking task. The Cerebellum 9, 454–460. doi: 10.1007/s12311-010-0187-4
Kantak, S. S., Sullivan, K. J., Fisher, B. E., Knowlton, B. J., and Winstein, C. J. (2010). Neural substrates of motor memory consolidation depend on practice structure. Nat. Neurosci. 13:923. doi: 10.1038/nn.2596
Karni, A., Meyer, G., Jezzard, P., Adams, M. M., Turner, R., and Ungerleider, L. G. (1995). Functional MRI evidence for adult motor cortex plasticity during motor skill learning. Nature 377, 155–158. doi: 10.1038/377155a0
Kasess, C. H., Windischberger, C., Cunnington, R., Lanzenberger, R., Pezawas, L., and Moser, E. (2008). The suppressive influence of SMA on M1 in motor imagery revealed by fMRI and dynamic causal modeling. Neuroimage 40, 828–837. doi: 10.1016/j.neuroimage.2007.11.040
Kim, C. K., Adhikari, A., and Deisseroth, K. (2017). Integration of optogenetics with complementary methodologies in systems neuroscience. Nat. Rev. Neurosci. 18:222. doi: 10.1038/nrn.2017.15
Korman, M., Doyon, J., Doljansky, J., Carrier, J., Dagan, Y., and Karni, A. (2007). Daytime sleep condenses the time course of motor memory consolidation. Nat. Neurosci. 10, 1206–1213. doi: 10.1038/nn1959
Krakauer, J. W., Ghazanfar, A. A., Gomez-Marin, A., MacIver, M. A., and Poeppel, D. (2017). Neuroscience needs behavior: correcting a reductionist bias. Neuron 93, 480–490. doi: 10.1016/j.neuron.2016.12.041
Kristo, G., Rutten, G.-J., Raemaekers, M., de Gelder, B., Rombouts, S. A. R. B., and Ramsey, N. F. (2014). Task and task-free FMRI reproducibility comparison for motor network identification. Hum. Brain Mapp. 35, 340–352. doi: 10.1002/hbm.22180
Love, B. C. (2016). Cognitive models as bridge between brain and behavior. Trends Cogn. Sci. 20, 247–248. doi: 10.1016/j.tics.2016.02.006
Lugassy, D., Herszage, J., Pilo, R., Brosh, T., and Censor, N. (2018). Consolidation of complex motor skill learning: evidence for a delayed offline process. Sleep 41:zsy123. doi: 10.1093/sleep/zsy123
Muellbacher, W., Ziemann, U., Wissel, J., Dang, N., Kofler, M., Facchini, S., et al. (2002). Early consolidation in human primary motor cortex 3. Nature 415, 640–644. doi: 10.1038/nature712
Mueller, S., Wang, D., Fox, M. D., Yeo, B. T. T., Sepulcre, J., Sabuncu, M. R., et al. (2013). Individual variability in functional connectivity architecture of the human brain. Neuron 77, 586–595.
Narayana, S., Zhang, W., Rogers, W., Strickland, C., Franklin, C., Lancaster, J. L., et al. (2014). Concurrent TMS to the primary motor cortex augments slow motor learning. Neuroimage 85, 971–984. doi: 10.1016/j.neuroimage.2013.07.024
Nettekoven, C., Volz, L. J., Leimbach, M., Pool, E. M., Rehme, A. K., Eickhoff, S. B., et al. (2015). Inter-individual variability in cortical excitability and motor network connectivity following multiple blocks of rTMS. Neuroimage 118, 209–218. doi: 10.1016/j.neuroimage.2015.06.004
Orth, M., Münchau, A., and Rothwell, J. C. (2008). Corticospinal system excitability at rest is associated with tic severity in Tourette syndrome. Biol. Psychiatry 64, 248–251. doi: 10.1016/j.biopsych.2007.12.009
Ostadan, F., Centeno, C., Daloze, J.-F., Frenn, M., Lundbye-Jensen, J., and Roig, M. (2016). Changes in corticospinal excitability during consolidation predict acute exercise-induced off-line gains in procedural memory. Neurobiol. Learn. Mem. 136, 196–203. doi: 10.1016/j.nlm.2016.10.009
Perez, M. A., and Cohen, L. G. (2009). Interhemispheric inhibition between primary motor cortices: what have we learned? J. Physiol. 587, 725–726. doi: 10.1113/jphysiol.2008.166926
Perez, M. A., Tanaka, S., Wise, S. P., Sadato, N., Tanabe, H. C., Willingham, D. T., et al. (2007). Neural substrates of intermanual transfer of a newly acquired motor skill. Curr. Biol. 17, 1896–1902. doi: 10.1016/j.cub.2007.09.058
Romei, V., Brodbeck, V., Michel, C., Amedi, A., Pascual-Leone, A., and Thut, G. (2007). Spontaneous fluctuations in posterior α-band EEG activity reflect variability in excitability of human visual areas. Cereb. cortex 18, 2010–2018.
Rosenkranz, K., Williamon, A., and Rothwell, J. C. (2007). Motorcortical excitability and synaptic plasticity is enhanced in professional musicians. J. Neurosci. 27, 5200–5206.
Rossini, P. M., Barker, A. T., Berardelli, A., Caramia, M. D., Caruso, G., Cracco, R. Q., et al. (1994). Non-invasive electrical and magnetic stimulation of the brain, spinal cord and roots: basic principles and procedures for routine clinical application. Report of an IFCN committee. Electroencephalogr. Clin. Neurophysiol. 91, 79–92.
Salami, A., Pudas, S., and Nyberg, L. (2014). Elevated hippocampal resting-state connectivity underlies deficient neurocognitive function in aging. Proc. Natl. Acad. Sci. U.S.A. 111, 17654–17659. doi: 10.1073/pnas.1410233111
Schippling, S., Schneider, S. A., Bhatia, K. P., Münchau, A., Rothwell, J. C., Tabrizi, S. J., et al. (2009). Abnormal motor cortex excitability in preclinical and very early Huntington’s disease. Biol. Psychiatry 65, 959–965.
Strigaro, G., Hamada, M., Cantello, R., and Rothwell, J. C. (2016). Variability in response to 1Hz repetitive TMS. Clin. Neurophysiol. 127:e40. doi: 10.1016/j.clinph.2015.11.126
Taubert, M., Lohmann, G., Margulies, D. S., Villringer, A., and Ragert, P. (2011). Long-term effects of motor training on resting-state networks and underlying brain structure. Neuroimage 57, 1492–1498. doi: 10.1016/j.neuroimage.2011.05.078
Tunovic, S., Press, D. Z., and Robertson, E. M. (2014). A physiological signal that prevents motor skill improvements during consolidation. J. Neurosci. 34, 5302–5310. doi: 10.1523/JNEUROSCI.3497-13.2014
van den Heuvel, M. P., and Hulshoff Pol, H. E. (2010). Exploring the brain network: a review on resting-state fMRI functional connectivity. Eur. Neuropsychopharmacol. 20, 519–534. doi: 10.1016/j.euroneuro.2010.03.008
van der Salm, S. M. A., van Rootselaar, A. F., Foncke, E. M. J., Koelman, J. H. T. M., Bour, L. J., Bhatia, K. P., et al. (2009). Normal cortical excitability in myoclonus-Dystonia–a TMS study. Exp. Neurol. 216, 300–305. doi: 10.1016/j.expneurol.2008.12.001
Volz, L. J., Hamada, M., Michely, J., Pool, E., Nettekoven, C., Rothwell, J. C., et al. (2019). Modulation of I-wave generating pathways by theta-burst stimulation: a model of plasticity induction. J. Physiol 597, 5963–5971. doi: 10.1113/JP278636
Volz, L. J., Hamada, M., Rothwell, J. C., and Grefkes, C. (2014). What makes the muscle twitch: motor system connectivity and TMS-Induced activity. Cereb. Cortex 25, 2346–2353. doi: 10.1093/cercor/bhu032
Walker, M. P., Brakefield, T., and Hobson, J. A. (2003). Dissociable stages of human memory consolidation and reconsolidation. Nature 425, 616–620. doi: 10.1038/nature01951.1
Wang, L., LaViolette, P., O’Keefe, K., Putcha, D., Bakkour, A., Van Dijk, K. R. A., et al. (2010). Intrinsic connectivity between the hippocampus and posteromedial cortex predicts memory performance in cognitively intact older individuals. Neuroimage 51, 910–917. doi: 10.1016/j.neuroimage.2010.02.046
Ward, N. S., Newton, J. M., Swayne, O. B. C., Lee, L., Thompson, A. J., Greenwood, R. J., et al. (2006). Motor system activation after subcortical stroke depends on corticospinal system integrity. Brain 129, 809–819.
Wassermann, E. M., Wedegaertner, F. R., Ziemann, U., George, M. S., and Chen, R. (1998). Crossed reduction of human motor cortex excitability by 1-Hz transcranial magnetic stimulation. Neurosci. Lett. 250, 141–144.
Wu, J., Srinivasan, R., Kaur, A., and Cramer, S. C. (2014). Resting-state cortical connectivity predicts motor skill acquisition. Neuroimage 91, 84–90. doi: 10.1016/j.neuroimage.2014.01.026
Keywords: functional connectivity, motor skill, individual differences, transcranial magnetic stimulation, motor-evoked-potentials, excitability
Citation: Herszage J, Dayan E, Sharon H and Censor N (2020) Explaining Individual Differences in Motor Behavior by Intrinsic Functional Connectivity and Corticospinal Excitability. Front. Neurosci. 14:76. doi: 10.3389/fnins.2020.00076
Received: 31 August 2019; Accepted: 20 January 2020;
Published: 05 February 2020.
Edited by:
Valeria Della-Maggiore, University of Buenos Aires, ArgentinaReviewed by:
Laura Säisänen, Kuopio University Hospital, FinlandNobuaki Mizuguchi, National Center for Geriatrics and Gerontology (NCGG), Japan
Copyright © 2020 Herszage, Dayan, Sharon and Censor. This is an open-access article distributed under the terms of the Creative Commons Attribution License (CC BY). The use, distribution or reproduction in other forums is permitted, provided the original author(s) and the copyright owner(s) are credited and that the original publication in this journal is cited, in accordance with accepted academic practice. No use, distribution or reproduction is permitted which does not comply with these terms.
*Correspondence: Nitzan Censor, censornitzan@tauex.tau.ac.il