- 1Department of Medical Imaging, Jinling Hospital, Medical School of Nanjing University, Nanjing, China
- 2Division of Cardiovascular Imaging, Department of Radiology and Radiological Science, Medical University of South Carolina, Charleston, SC, United States
Objective: To explore the relationship among serum uric acid (SUA) levels in different states of disease, human cognition, and spontaneous brain activities by resting-state functional MRI (rs-fMRI).
Methods: We prospectively recruited 100 subjects (age 58 ± 11 years, 55 females) who underwent fasting blood sampling, cognitive tests and rs-fMRI scans. The subjects were divided into two groups by sex and each sex group was further stratified into three subgroups according to SUA level in different states of disease. The amplitude of low-frequency fluctuation (ALFF) method was applied to assess spontaneous brain activity among groups. Pearson’s correlation analysis was used to investigate the relationships between the mean ALFF values (mALFF) and cognitive tests.
Results: A total of 97 patients completed the study protocol successfully. Significant differences in age, education level, number connection test (NCT), and word fluency were observed among the three subgroups in males (all P < 0.05). Results of group-by-sex interaction were distributed in bilateral pallidum and putamen [voxel P-value < 0.001, cluster P-value < 0.05, Gaussian random field (GRF)-corrected]. The tendency of the SUA effect on mALFF was different in males and females, particularly in corresponding High SUA subgroups (that is pre-hyperuricemia, both P < 0.001). Among the male subjects, mALFF values of the bilateral pallidum and putamen negatively correlated with attention/executive function.
Conclusion: Our results suggest that elevated SUA levels have different effects on spontaneous brain activities and cognitive function in males and females. Males with pre-hyperuricemia and hyperuricemia are more susceptible to changes in spontaneous brain activities and lower neuropsychological assessment scores, particularly in word fluency tests and NCT, compared to females.
Introduction
Gout is a chronic and progressive disease caused by the deposition of monosodium urate crystals. The incidence of gout is growing from 0.30 per 1000 person-years to 2.68 per 1000 person-years from the 1970s to the 2000s (Dalbeth and Stamp, 2014). Comorbidities are common in patients with gout according to the 2007–2008 National Health and Nutrition Examination Survey data, such as hypertension and chronic kidney disease (Zhu et al., 2012; Dalbeth and Merriman, 2016). Gout is also associated with an increased risk of death, primarily due to cardiovascular disease (Choi and Curhan, 2007; Dalbeth and Merriman, 2016). Hyperuricemia, considered as stage A of gout in a new clinical staging system proposed in 2014, is the primary risk factor for gout (Dalbeth and Stamp, 2014). However, as serum uric acid (SUA) is an end product of purine metabolism and a natural antioxidant, it may also have neuroprotective effects (Khan et al., 2016). Consequently, more and more researchers have been committed to exploring the effect of SUA on cognitive function.
Previous studies found that patients with mild cognitive impairment and Alzheimer’s disease had lower SUA levels than healthy controls (Rinaldi et al., 2003; Kim et al., 2006). The Rotterdam study also found that individuals with higher SUA levels may have a lower risk of dementia and better cognitive function later in life (Euser et al., 2009). Recently, researchers studied the curative effect of SUA in acute stroke to confirm its neuroprotective effects. It has been suggested that SUA might reduce infarct growth and improve outcomes in patients with hyperglycemia during acute stroke (Amaro et al., 2015). However, other studies did not identify such neuroprotective effects related to SUA and even reported controversial results. One study found that there was no difference in outcome after stroke or safety concerns between the use of SUA in thrombolytic therapy or a placebo (Chamorro et al., 2014). Furthermore, another preclinical study found that SUA may induce hippocampal inflammation and may cause cognitive dysfunction in rodents and humans (Shao et al., 2016). In addition, elderly people with high SUA levels may have an increased risk of vascular or mixed dementia (Latourte et al., 2018), and mildly elevated SUA levels might increase the burden of cerebral ischemic pathology, particularly in older adults (Schretlen et al., 2007). In short, the neuroprotective effects of SUA are controversial and still uncertain.
Resting-state functional MRI (rs-fMRI), a sensitive and effective modality used to explore subtle brain function alterations, has been widely used in neuroimaging studies. The amplitude of low-frequency fluctuation (ALFF) is a reliable measure to detect the spontaneous neuronal activity in blood-oxygenation-level-dependent (BOLD)-related signals (Zhao et al., 2018). ALFF has been used in many neurodegenerative and neurometabolic disease studies, such as evaluating learning ability (Qian et al., 2016), consciousness level in acquired brain injury (Sharp et al., 2014; Zou et al., 2017), cognitive dysfunction in Alzheimer’s disease (Han et al., 2011; Wen et al., 2013), and schizophrenia (Meda et al., 2015; Hare et al., 2017). However, studies focusing on the effect of SUA levels on rs-fMRI-based cognitive function are warranted. Since hyperuricemia shows sex differences (Kuo et al., 2015; Dalbeth and Merriman, 2016), we hypothesize that SUA levels have different effects on cognitive function in males and females. Thus, this study aims to explore the effect of SUA levels and sex on spontaneous brain activity, as evaluated by ALFF, a rs-fMRI analytical algorithm.
Materials and Methods
Subjects
The study protocol was approved by the local Ethics Committee and all subjects provided written informed consent prior to the MRI and neuropsychological tests. This prospective study recruited 100 subjects from the local community. The inclusion criteria were as follows: right-handedness and aged 20–80 years without any history of psychiatric or neurological diseases. Participants with contraindications to MRI, history of gout, major adverse cardiovascular events, cerebrovascular disease, and severe chronic diseases with poor therapeutic control were excluded. Participants who had head motions with rotation of more than 1.0° or translation of more than 1.0 mm were excluded as well.
Serum Assessment
Each subject underwent serum biochemical tests, including SUA, fasting plasma glucose (FPG), cholesterol (CHO), triglyceride (TRI), high-density lipoprotein cholesterol (HDL-C), low-density lipoprotein cholesterol (LDL-C), serum urea and serum creatinine. We estimated glomerular filtration rate (eGFR) using the Modification of Diet in Renal Disease (MDRD) equation to assess renal function (Poggio et al., 2005). Since SUA levels are consistently lower in females than in males (Rho et al., 2011; Latourte et al., 2018), subjects were grouped by sex. Then, each sex group was further stratified into three subgroups based on SUA levels: hyperuricemia level, high level (that is pre-hyperuricemia) and healthy level. Subgroups in males were: hyperuricemia level (Dis; SUA > 7.0 mg/dl); high level (High; SUA: 5.83–7.0 mg/dl); and healthy level (HC; SUA: 2.5–5.82 mg/dl), while subgroups in females were: Dis (SUA > 6.0 mg/dl); High (SUA: 5.0–6.0 mg/dl); and HC (1.66–5.0 mg/dl) (Chizyński, 2005).
Neuropsychological Assessment
Neuropsychological assessment was conducted by a well-trained researcher (XX) with 5-year experience. Mini-Mental State Examination (MMSE) and Montreal Cognitive Assessment (MoCA) tests were used to assess general cognition status (Galea and Woodward, 2005; Nasreddine et al., 2005). Learning/memory and attention/executive function were assessed by vocabulary learning and word fluency tests, number connection test (NCT), and digit symbol test (DST). In addition, line tracing test (LTT), serial dotting test (SDT), and Stroop test have been applied to assess reaction capability (Su et al., 2016; Zheng et al., 2017).
MR Imaging Data Acquisition
A 3-T MRI scanner (TIM Trio, Siemens Healthineers, Erlangen, Germany) equipped with the standard 12-channel head coil was used to scan all subjects. Participants were required to close their eyes and keep their head still during the scan (Su et al., 2016; Zheng et al., 2017). T2 fluid-attenuated inversion-recovery acquisition was used for clinically silent lesions screening with the following pulse sequence parameters: number of axial slices 25, slice thickness 4 mm, slice gap 1.2 mm, image matrix 232 × 256, field of view [FOV] 220 × 220 mm2, repetition time [TR]/echo time [TE] 9000 ms/93 ms, flip angle 130°, and inversion time 2500 ms. rs-fMRI data were acquired using a single-shot, gradient-recalled echo planar imaging sequence aligned along the anterior–posterior commissure to cover the whole brain for a period of 500 s, with the following parameters: number of volumes 250, image matrix 64 × 64, FOV 240 × 240 mm2, voxel size 3.75 × 3.75 × 4 mm3, number of axial slices 30, TR/TE 2000 ms/40 ms, and flip angle 90°. Lastly, anatomical images were obtained in the sagittal orientation using a high-resolution, T1-weighted, 3D, magnetization-prepared rapid gradient-echo sequence (T1 W-3D-MPRAGE) with the following parameters: TR/TE 2300 ms/2.98 ms, flip angle 9°, number of slices 191, FOV 256 × 256 mm2, acquisition matrix 256 × 256, and slice thickness 1 mm. The overall scan time was 20 minutes for each subject, and we inquired them after scan. All the subjects reported that they were not falling asleep during scanning.
Data Preprocessing
The preprocessing was performed using Data Processing Assistant for Resting-State fMRI (DPARSF1 ; Yan and Zang, 2010; Yan et al., 2016). Initially, the first 10 volumes were deleted for both adjustment to the signal equilibrium and subjects’ adaptation to the environment. The images were then processed for slice timing and realignment. Subjects with translation or rotation parameters of more than 1.0 mm or 1.0° were excluded. Then, T1 W-3D-MPRAGE images were segmented into gray matter, white matter, and cerebrospinal fluid then normalized to the Montreal Neurological Institute space by using DARTEL (voxel size of 3 × 3 × 3 mm3). The T1 W-3D-MPRAGE images were thus co-registered to the fMRI data and smoothed with an isotropic Gaussian kernel of 6 mm. Finally, the processed data were detrended and nuisance covariates were regressed as well. Additionally, global signal regression was reported to reduce the relationships between motion and inter-individual differences in rs-fMRI metrics (Saad et al., 2012; Yan et al., 2012), we thus calculated the ALFF without global signal regression.
ALFF Analysis
Amplitude of low-frequency fluctuation measures the amplitude of time series fluctuations at each voxel (Zhang et al., 2007), based on the DPARSF software. First, the time series for each voxel was filtered (band-pass, 0.01–0.1 Hz) and subsequently converted to the frequency domain by fast Fourier transform (FFT). The square root was calculated at each frequency of the power spectrum. The averaged square root was taken as the ALFF, and the mean ALFF was finally computed by the normalization of ALFF by the mean within-brain ALFF value for each subject.
Statistical Analysis
The demographic and neuropsychiatric data analysis was performed with IBM SPSS Statistics 21.0. The Kolmogorov–Smirnov test was used to assess the normality of quantitative data. Normally distributed data were expressed as mean ± standard deviation. Then, the differences between the three subgroups, within the larger groups of males and females, were assessed by ANOVA test and the homogeneity of variance in these data was examined by the Levene test. The least significant difference (LSD) test was employed when the variance was homogeneous, while the Tamhane’s T2 was applied for the data with heterogeneous variance. Independent sample non-parametric test was used to analyze the data with non-normal distribution, which were reported as median and inter-quartile range [M(QU-QL)]. If the variance analysis revealed significant differences, post hoc analysis was conducted for inter-group comparisons. The differences between subgroups within each group were assessed by independent sample t test. P < 0.05 was regarded as a significant difference.
The rs-fMRI data were analyzed by Statistical Parametric Mapping 12 (SPM12)2. Primary analysis was assessed with a full factorial design (group-by-sex interactions, main effect of group, main effect of sex), with the covariance of age, education years, body mass index (BMI) and eGFR. The results were then corrected by Gaussian random field correction (GRF) (significance level was set at voxel P value < 0.001 and cluster P value < 0.05). Post hoc analysis was further performed with IBM SPSS Statistics 21.0 by extracting the values of brain regions with statistical differences using independent sample t test (between female and male with the corresponding group) and analysis of variance (among three subgroups respectively, in males and females). Significance level was set at P < 0.05. All the mALFF of the brain regions with significant differences were extracted for correlation analysis against the scores of the cognitive performance. Correlation analyses with IBM SPSS Statistics 21.0 were performed by using Pearson (normal distribution data) or Spearman correlation analysis (non-normal distribution data) with significant difference at P values < 0.05.
We calculated the power for two factor interactions (sex and uric acid levels) by using the G Power Software (version 3.1), which was described in detail in the Supplementary Material. In order to further study the effect of SUA concentration on the brain, we used SUA concentration as a continuous variable to study the interaction between sex and SUA concentration. Two sample t test design was used (SUA concentration-by-sex interactions, regression between SUA concentration and males/females), with the covariance of age, education years. The results were then corrected by the GRF (significance level was set at voxel P value < 0.001 and cluster P value < 0.05).
Results
Demographic and Neuropsychological Assessment Data
The demographics and neuropsychological assessment data are shown in Table 1. Subjects with head motions with translation of more than 1.0 mm or rotation of more than 1.0° (n = 2) and clinically silent lesions (posterior fossa cyst, n = 1) were excluded. Ninety-seven subjects including 55 females (mean age of 58 ± 11 years) and 42 males (mean age of 58 ± 14 years) were included in data analysis.
There were no significant differences in age, BMI, education level or neuropsychological assessment among the three subgroups of females (all P > 0.05). However, there were significant differences in age, education level, NCT, and word fluency among the three subgroups of males. Specifically, the differences of age and NCT were shown between Dis and HC subgroups (P = 0.037, P = 0.021, respectively), and education years were different between Dis and High subgroups (P = 0.021). The word fluency tests showed significant difference between Dis versus High subgroups and High versus HC subgroups (P = 0.048; P = 0.009, respectively).
For blood biochemical indices, there were no differences among subgroups of males and females (all P > 0.05), except for LDL-C, Scr and eGFR in females (P = 0.003).
Results of ALFF Analysis
Group-By-Sex Interaction
The results of group-by-sex interaction and its post hoc analysis is shown in Figure 1 and Table 2. Our study found that the mALFF in bilateral pallidum and putamen was modulated (GRF correction, P value < 0.001 at voxel level and P value < 0.05 at cluster level). The tendency of SUA effect on mALFF was different in males and females (Figures 1A,B). Specifically, as for the sex difference with the corresponding SUA level, the mALFF of bilateral pallidum and putamen were different in the High group (both P < 0.001), while only the mALFF of the right pallidum and putamen were different in the Dis group (P = 0.005) (Figures 1C,D).
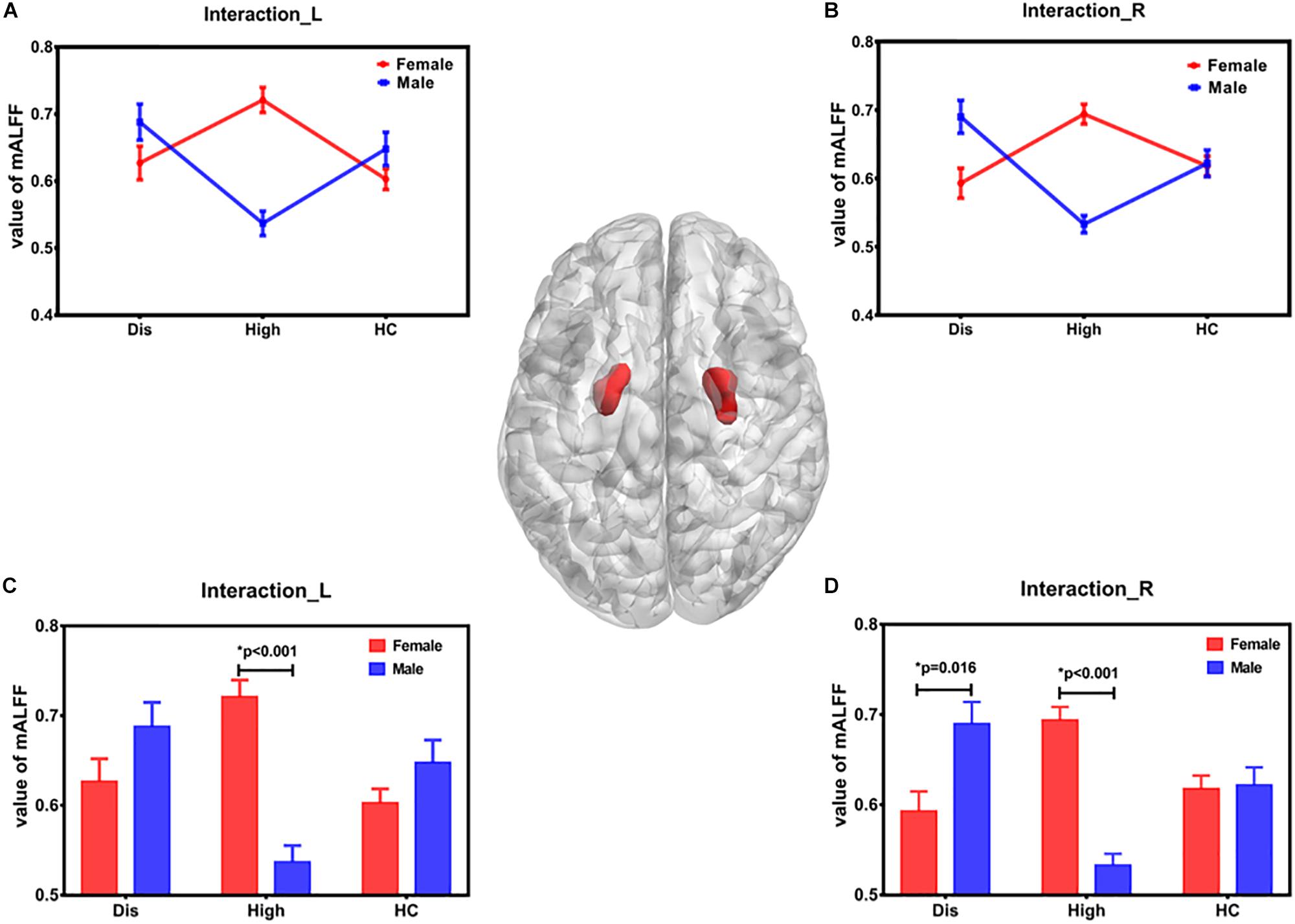
Figure 1. Brain regions with statistical differences in group-by-sex interaction. The brain regions influenced by group-by-sex interaction was bilateral pallidum and putamen, shown in the middle brain figure. Panels (A,B) show the tendency of SUA effect on ALFF in males and females. In females, the mean ALFF value of High subgroup was the highest, while the lowest in males. Panels (C,D) display the mALFF difference between females and males in each SUA level subgroup. L – left, R – right, F – female, M – male, SUA – serum uric acid, mALFF – mean value of ALFF, Dis – hyperuricemia level, High – high level, HC – healthy level.
The differences among the three subgroups of males and females are shown in Figure 2. In females, the differences were shown in the bilateral pallidum and putamen between Dis vs. High subgroups (Dis < High, right: P = 0.001; left: P = 0.018) and between High vs. HC subgroups (High > HC, right: P = 0.001; left: P < 0.001) (Figures 2A,C). In males, the differences of the bilateral pallidum and putamen were between Dis vs. High subgroups (Dis > High, right: P < 0.001; left: P = 0.001) and High vs. HC subgroups (High < HC, right: P = 0.004; left: P = 0.006), and differences in the right brain regions between Dis vs. HC subgroups (Dis > HC, P = 0.014) (Figures 2B,D).
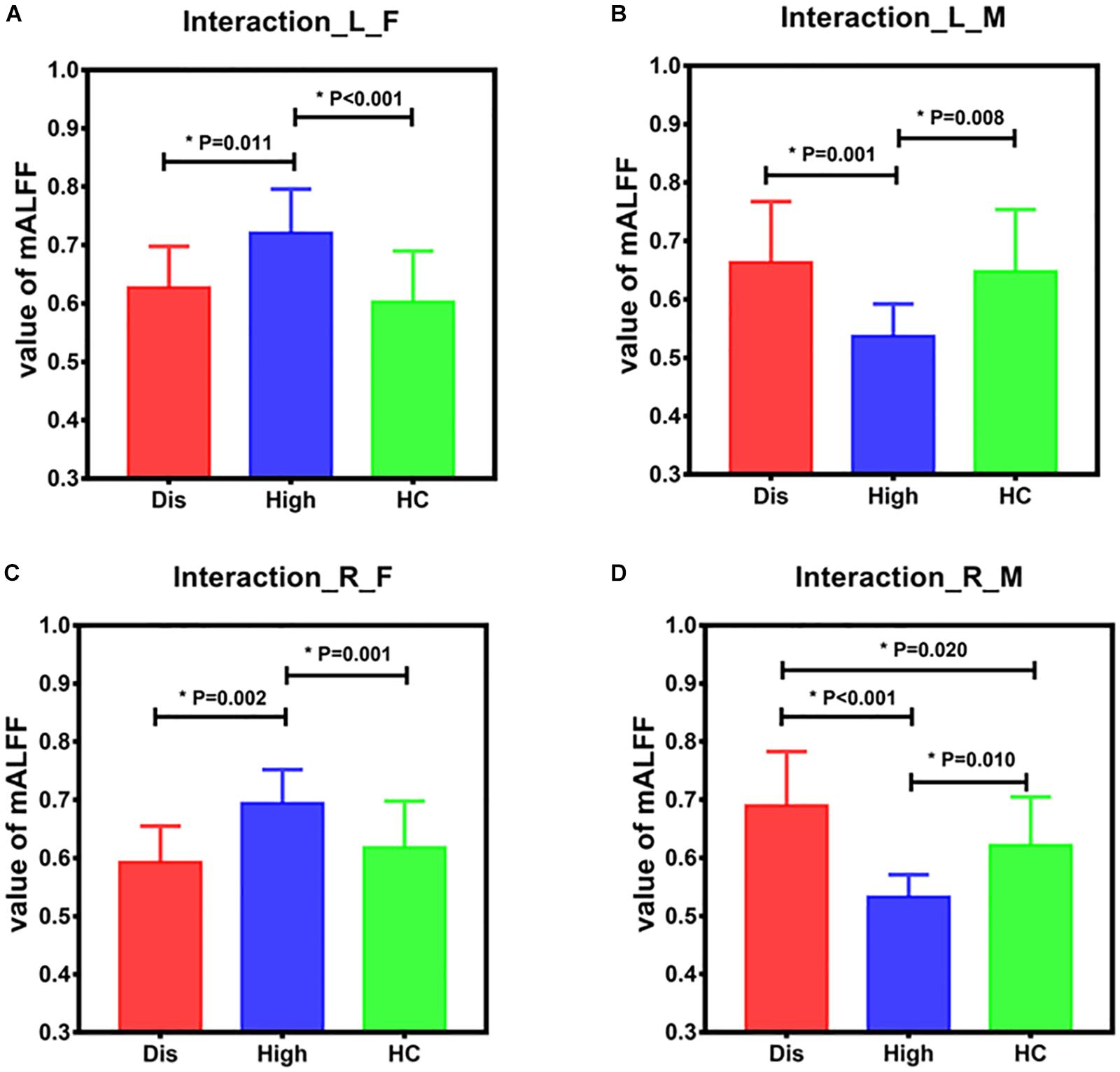
Figure 2. Differences of brain regions influenced by group-by-sex interaction among the three subgroups for male and female. Panels (A,B) show the mean ALFF value extracted from left pallidum and putamen among three subgroups for females and males. Panels (C,D) show the mean ALFF value extracted from right pallidum and putamen among three subgroups for females and males. In females, the differences are shown in bilateral pallidum and putamen between Dis and High subgroups and between High and HC subgroups. In males, there are differences in bilateral pallidum and putamen between Dis and High subgroups and between High vs. HC subgroups, and differences in right pallidum and putamen between Dis vs. HC subgroups. L – left, R – right, F – female, M – male, mALFF – mean vale of ALFF, Dis – hyperuricemia level, High – high level, HC – healthy level.
The correlation analysis between brain regions with statistical differences among groups and neuropsychological assessment is described in Table 3. For male subjects, the mALFF values of bilateral pallidum and putamen negatively correlated with the NCT scores (right, P = 0.027, R = –0.342; left, P = 0.039, R = –0.320), while the mean ALFF values of left pallidum and putamen positively correlated with the scores of word fluency (P = 0.004, R = 0.439). The mean ALFF values of bilateral pallidum and putamen were not correlated with scores of any neuropsychological tests across female subjects.
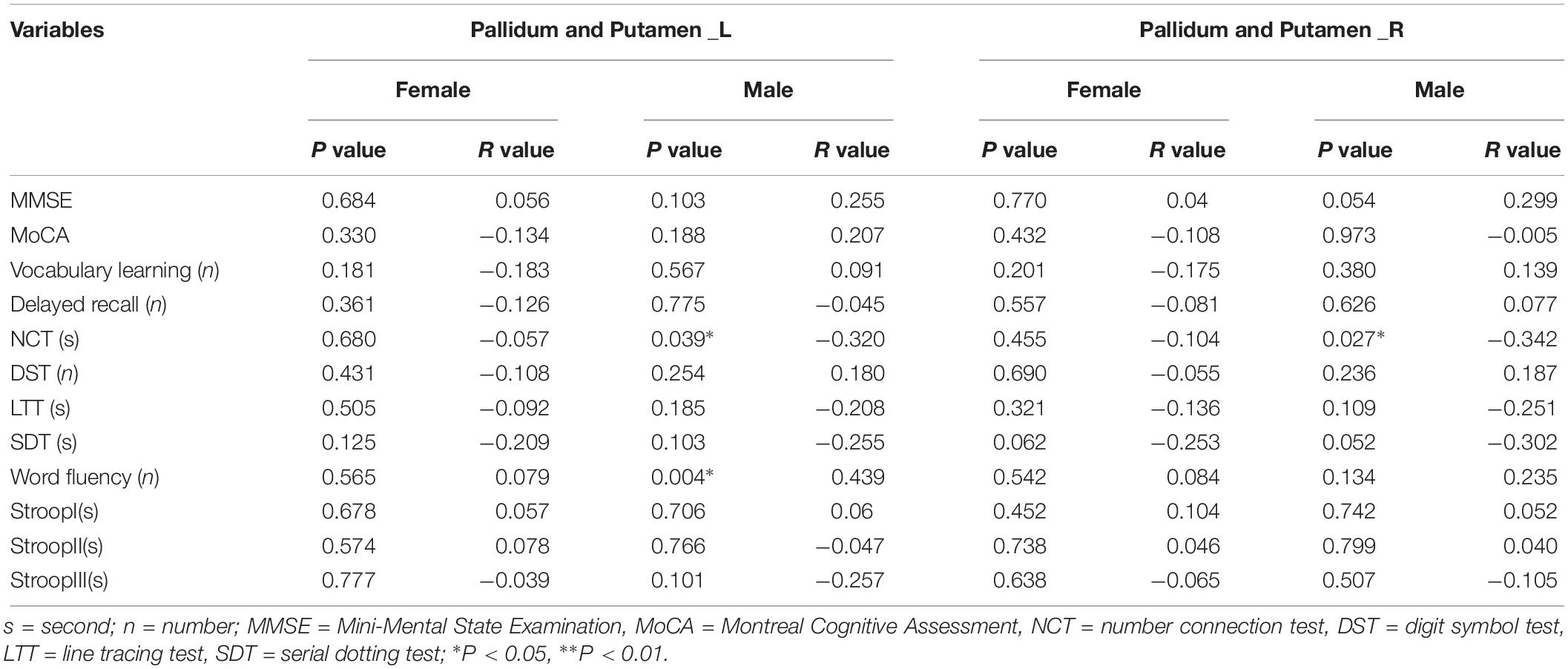
Table 3. Correlation Analysis between brain regions with statistical differences of interaction and neuropsychological assessment data.
Main Effect of Sex and Group
Brain regions influenced by the main effect of sex were distributed in superior frontal gyrus, anterior cingulate cortex, paracentral lobule in bilateral cerebrum, inferior frontal gyrus, middle temporal gyrus, precentral gyrus in right cerebrum, transverse temporal gyrus, and postcentral gyrus in left cerebrum (voxel P value < 0.001, cluster P value < 0.05, GRF-corrected) (Figure 3). However, we did not find any brain regions influenced by the main effect of group after GRF correction.
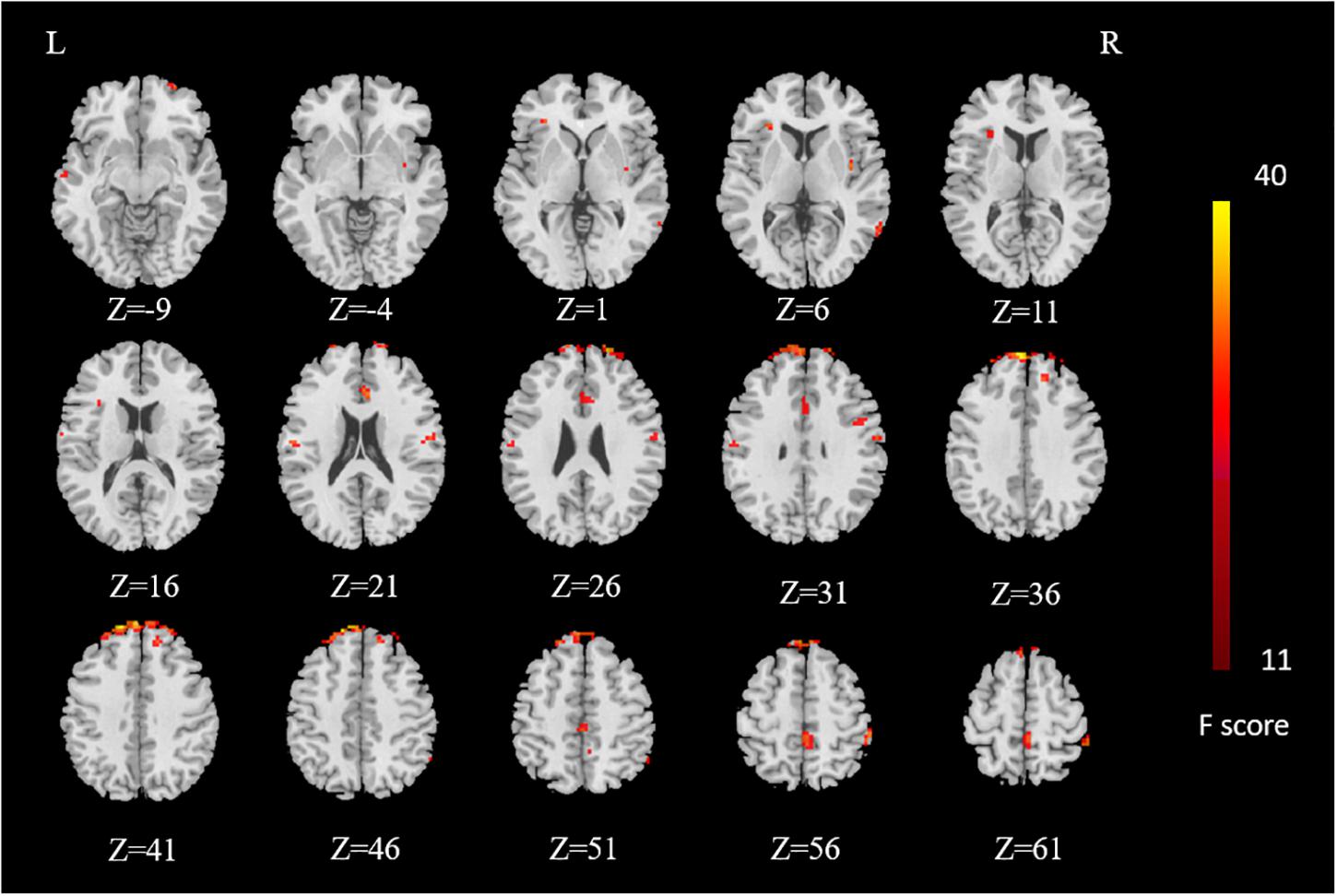
Figure 3. Results of main effect of sex. Brain regions influenced by main effect of sex are distributed in superior frontal gyrus, anterior cingulate, paracentral lobule in bilateral cerebrum, inferior frontal gyrus, middle temporal gyrus, precentral gyrus in right cerebrum, transverse temporal gyrus, and postcentral gyrus in left cerebrum. The F value color-coded scale is reported at the right side of the images. L – left, R – right.
Result of Secondary Analysis
We used SUA as a continuous variable to study SUA concentration-by-sex interactions. There was no cluster survived after GRF correction. Furthermore, there was no cluster survived after GRF correction neither in males or females, when we did regression between SUA concentration and males/females, respectively.
Discussion
This study analyzed the potential association between SUA in different states of disease and spontaneous brain activities evaluated by rs-fMRI. We found different patterns of spontaneous brain activities in males and females with different SUA levels in different states of disease. Our results suggest that elevated SUA levels are associated with lower neuropsychological assessment scores, particularly in word fluency tests and NCT. Furthermore, changes in spontaneous brain activities and cognition assessment scores appeared before hyperuricemia, and males were more susceptible than females. These findings shed a new light on cognitive function changes influenced by the different SUA levels.
Our study showed that the changes in spontaneous brain activities were mainly in the pallidum and putamen, which were correlated with word fluency and NCT scores. Pallidum and putamen are the structures that compose the basal ganglia. The putamen plays an important role in several basal ganglia–thalamocortical circuits (Alexander et al., 1986) involved in motor control and learning, as well as selecting and enabling cognitive, executive, and emotional programs (Ring and Serra-Mestres, 2002). Putamen has also been found to play a key role in the pathophysiology of patients with attention-deficit/hyperactivity disorder (Greven et al., 2015). Consistently, our study showed that the mALFF of the pallidum and putamen was positively correlated with word fluency and negatively correlated with NCT. These findings indicate that the results were closely related to attention/executive function. Moreover, since a lower score of NCT and a higher score of word fluency stand for better attention/executive function, we speculate that subjects with a higher mALFF might have better attention/executive function.
We also found that the High subgroup of males had the lowest mALFF and performed worst in word fluency tests and NCT. However, we did not observe this finding in females. This suggests a possible sex-related effect of SUA on attention/executive function. Our study thus implies that attention/executive function might be impaired in males with pre-hyperuricemia, in accordance with previous studies (Vannorsdall et al., 2008; Shao et al., 2016; Desideri et al., 2017). One previous study found that female hormones are associated with lower SUA levels through renal clearance (Hak and Choi, 2008), the possible reason might be a relative protective effect of estrogen (Mauvais-Jarvis, 2015; Dekkers et al., 2019).
Vannorsdall et al. (2008) found that high SUA levels were associated with greater white matter hyperintensities and poorer working memory, processing speed, fluency, and verbal memory. Another study suggested that an increased SUA level may result in cognitive dysfunction by causing hippocampal inflammation (Shao et al., 2016). Furthermore, an in vitro experimental model documented that high SUA levels could exert detrimental effects on brain structure and function by directly influencing the viability of neuronal cells and their ability to establish synaptic connections (Desideri et al., 2017). Importantly, our investigation, in agreement with the study by Baena et al. (2017), found a possible sex-related effect of SUA on executive function in a middle-aged population. However, Baena et al. (2017) found better cognitive performance in an executive function test in men but not in women, while our results showed the attention/executive function impaired in the High subgroup of males. Possible explanation for the difference is that the high SUA level of their subjects might be in compensatory state, and the results were just like what we found in Dis level. Furthermore, we did not find any brain regions with statistical difference by secondary analysis, which corresponds with the results of primary analysis as the trend showed in Figures 1A,B.
All the alterations indicated the sensitivity and vulnerability of the mALFF derived from the period before the hyperuricemia. However, the European League Against Rheumatism and American College of Rheumatology recommend reducing SUA level in the state of hyperuricemia (Richette et al., 2017). According to our current study findings, it is rational to pay more attention to the subjects with pre-hyperuricemia rather than one with hyperuricemia. However, more clinical studies are needed to validate our hypothesis.
Our study has some limitations. First, the sample size was small, thus it is necessary to further validate our findings in a larger cohort. Second, subjects in the different subgroups were not matched. Third, we only studied the difference of ALFF among SUA levels, and more brain function indices should be used to confirm our findings. Fourth, our study is a cross-sectional study, which could not show cognitive function changes during the process of disease progression. Fifth, subjects with their eyes closed in the scanner may not be awake all the time, although we collected rs-fMRI data at first, and told the subject not to fall asleep before scanning, in order to reduce the probability of the subject falling asleep. In future study, we will add some objective indicators to monitor the state of subjects during scanning, such as electroencephalogram. Last but not least, although we controlled for many cardiovascular disease risk factors, there are many other confounding factors as well. We could not exclude the possibility that SUA affected cognitive function by increasing the risk of cardiovascular disease. More work should be done to confirm our findings in the future.
In conclusion, our results suggest that elevated SUA levels were associated with lower neuropsychological assessment scores, particularly in word fluency tests and NCT. Furthermore, changes in spontaneous brain activities and cognition appeared before hyperuricemia, and males are more susceptible than females. This is very implicative for the clinical management for patients with pre-hyperuricemia and hyperuricemia although further clinical studies are urgently needed to demonstrate this finding.
Ethics Statement
The study protocol was approved by the local Ethics Committee and all subjects provided written informed consent prior to the MRI and neuropsychological tests.
Author Contributions
All authors listed have made a substantial, direct and intellectual contribution to the work, and approved it for publication.
Funding
This work was supported by grants from National Natural Science Foundation of China (Grants Nos. 81322020 and 81230032 to LJZ).
Conflict of Interest Statement
UJS is a consultant for and/or receives research support from Astellas, Bayer, Elucid BioImaging, General Electric, Guerbet, HeartFlow, and Siemens Healthineers. AV-S receives institutional research support from Siemens.
The remaining authors declare that the research was conducted in the absence of any commercial or financial relationships that could be construed as a potential conflict of interest.
Supplementary Material
The Supplementary Material for this article can be found online at: https://www.frontiersin.org/articles/10.3389/fnins.2019.00763/full#supplementary-material
Footnotes
References
Alexander, G. E., DeLong, M. R., and Strick, P. L. (1986). Parallel organization of functionally segregated circuits linking basal ganglia and cortex. Ann. Rev. Neurosci. 9, 357–381. doi: 10.1146/annurev.neuro.9.1.357
Amaro, S., Llull, L., Renú, A., Laredo, C., Perez, B., Vila, E., et al. (2015). Uric acid improves glucose-driven oxidative stress in human ischemic stroke. Ann. Neurol. 77, 775–783. doi: 10.1002/ana.24378
Baena, C. P., Suemoto, C. K., Barreto, S., Lotufo, P. A., and Benseñor, I. (2017). Serum uric acid is associated with better executive function in men but not in women: baseline assessment of the ELSA-Brasil study. Exp. Gerontol. 92, 82–86. doi: 10.1016/j.exger.2017.03.010
Chamorro, A., Amaro, S., Castellanos, M., Segura, T., Arenillas, J., Martí-Fábregas, J., et al. (2014). Safety and efficacy of uric acid in patients with acute stroke (URICO-ICTUS): a randomised, double-blind phase 2b/3 trial. Lancet Neurol. 13, 453–460. doi: 10.1016/S1474-4422(14)70054-7
Choi, H. K., and Curhan, G. (2007). Independent impact of gout on mortality and risk for coronary heart disease. Circulation 116, 894–900. doi: 10.1161/circulationaha.107.703389
Dalbeth, N., and Merriman, T. R. (2016). Gout. Lancet 388, 2039–2052. doi: 10.1016/S0140-6736(16)00346-9
Dalbeth, N., and Stamp, L. (2014). Hyperuricaemia and gout: time for a new staging system? Ann. Rheum. Dis. 73, 1598–1600. doi: 10.1136/annrheumdis-2014-205304
Dekkers, I. A., Jansen, P. R., and Lamb, H. J. (2019). Obesity, brain volume, and white matter microstructure at MRI: a cross-sectional UK biobank study. Radiology 291, 763–771. doi: 10.1148/radiol.2019181012
Desideri, G., Gentile, R., Antonosante, A., Benedetti, E., Grassi, D., Cristiano, L., et al. (2017). Uric acid amplifies Aβ amyloid effects involved in the cognitive dysfunction/dementia: evidences from an experimental model in vitro. J. Cell Physiol. 232, 1069–1078. doi: 10.1002/jcp.25509
Euser, S. M., Hofman, A., Westendorp, R. G., and Breteler, M. M. (2009). Serum uric acid and cognitive function and dementia. Brain 132, 377–382. doi: 10.1093/brain/awn316
Galea, M., and Woodward, M. (2005). Mini-mental state examination (MMSE). Aust. J. Physiother. 51, 198. doi: 10.1016/s0004-9514(05)70034-9
Greven, C. U., Bralten, J., Mennes, M., O’Dwyer, L., van Hulzen, K. J., Rommelse, N., et al. (2015). Developmentally stable whole-brain volume reductions and developmentally sensitive caudate and putamen volume alterations in those with attention-deficit/hyperactivity disorder and their unaffected siblings. JAMA Psychiatry 72, 490–499. doi: 10.1001/jamapsychiatry.2014.3162
Hak, A. E., and Choi, H. K. (2008). Menopause, postmenopausal hormone use and serum uric acid levels in US women–the third national health and nutrition examination survey. Arthritis Res. Ther. 10:R116. doi: 10.1186/ar2519
Han, Y., Wang, J., Zhao, Z., Min, B., Lu, J., Li, K., et al. (2011). Frequency-dependent changes in the amplitude of low-frequency fluctuations in amnestic mild cognitive impairment: a resting-state fMRI study. NeuroImage 55, 287–295. doi: 10.1016/j.neuroimage.2010.11.059
Hare, S. M., Ford, J. M., Ahmadi, A., Damaraju, E., Belger, A., Bustillo, J., et al. (2017). Functional imaging biomedical informatics research: modality-dependent impact of hallucinations on low-frequency fluctuations in schizophrenia. Schizophr. Bull. 43, 389–396. doi: 10.1093/schbul/sbw093
Khan, A. A., Quinn, T. J., Hewitt, J., Fan, Y., and Dawson, J. (2016). Serum uric acid level and association with cognitive impairment and dementia: systematic review and meta-analysis. Age 38:16. doi: 10.1007/s11357-016-9871-8
Kim, T. S., Pae, C. U., Yoon, S. J., Jang, W. Y., Lee, N. J., Kim, J. J., et al. (2006). Decreased plasma antioxidants in patients with alzheimer’s disease. Int. J. Geriatr. Psychiatry 21, 344–348.
Kuo, C.-F., Grainge, M. J., Zhang, W., and Doherty, M. (2015). Global epidemiology of gout: prevalence, incidence and risk factors. Nat. Rev. Rheumatol. 11, 649–662. doi: 10.1038/nrrheum.2015.91
Latourte, A., Soumaré, A., Bardin, T., Perez-Ruiz, F., Debette, S., Richette, P., et al. (2018). Uric acid and incident dementia over 12 years of follow-up: a population-based cohort study. Ann. Rheu. Dis. 77, 328–335. doi: 10.1136/annrheumdis-2016-210767
Mauvais-Jarvis, F. (2015). Sex differences in metabolic homeostasis, diabetes, and obesity. Biol. Sex Differ. 6:14. doi: 10.1186/s13293-015-0033-y
Meda, S. A., Wang, Z., Ivleva, E. I., Poudyal, G., Keshavan, M. S., Tamminga, C. A., et al. (2015). Frequency-specific neural signatures of spontaneous low-frequency resting state fluctuations in psychosis: evidence from bipolar-schizophrenia network on intermediate phenotypes (B-SNIP) Consortium. Schizophr. Bull. 41, 1336–1348. doi: 10.1093/schbul/sbv064
Nasreddine, Z. S., Phillips, N. A., Bédirian, V., Charbonneau, S., Whitehead, V., Collin, I., et al. (2005). The montreal cognitive assessment, MoCA: a brief screening tool for mild cognitive impairment. J. Am. Geriatr. Soc. 53, 695–699. doi: 10.1111/j.1532-5415.2005.53221.x
Poggio, E. D., Nef, P. C., Wang, X., Greene, T., Van Lente, F., Dennis, V. W., et al. (2005). Performance of the cockcroft-gault and modification of diet in renal disease equations in estimating GFR in ill hospitalized patients. Am. J. Kidney Dis. 46, 242–252. doi: 10.1053/j.ajkd.2005.04.023
Qian, Y., Bi, Y., Wang, X., Zhang, Y. W., and Bi, H. Y. (2016). Visual dorsal stream is associated with chinese reading skills: a resting-state fMRI study. Brain Lang. 160, 42–49. doi: 10.1016/j.bandl.2016.07.007
Rho, Y. H., Zhu, Y., and Choi, H. K. (2011). The epidemiology of uric acid and fructose. Semin. Nephrol. 31, 410–419. doi: 10.1016/j.semnephrol.2011.08.004
Richette, P., Doherty, M., Pascual, E., Barskova, V., Becce, F., Castañeda-Sanabria, J., et al. (2017). Updated EULAR evidence-based recommendations for the management of gout. Ann. Rheum Dis. 2017, 29–42. doi: 10.1136/annrheumdis-2016-209707
Rinaldi, P., Polidori, M. C., Metastasio, A., Mariani, E., Mattioli, P., Cherubini, A., et al. (2003). Plasma antioxidants are similarly depleted in mild cognitive impairment and in alzheimer’s disease. Neurobiol. Aging 24, 915–919. doi: 10.1016/s0197-4580(03)00031-9
Ring, H. A., and Serra-Mestres, J. (2002). Neuropsychiatry of the basal ganglia. J. Neurol. Neurosurg. Psychiatry 72, 12–21.
Saad, Z. S., Gotts, S. J., Murphy, K., Chen, G., Jo, H. J., Martin, A., et al. (2012). Trouble at rest: how correlation patterns and group differences become distorted after global signal regression. Brain Connect. 2, 25–32. doi: 10.1089/brain.2012.0080
Schretlen, D. J., Inscore, A. B., Vannorsdall, T. D., Kraut, M., Pearlson, G. D., Gordon, B., et al. (2007). Serum uric acid and brain ischemia in normal elderly adults. Neurology 69, 1418–1423. doi: 10.1212/01.wnl.0000277468.10236.f1
Shao, X., Lu, W., Gao, F., Li, D., Hu, J., Li, Y., et al. (2016). Uric acid induces cognitive dysfunction through hippocampal inflammation in rodents and humans. J. Neurosci. 36, 10990–11005. doi: 10.1523/jneurosci.1480-16.2016
Sharp, D. J., Scott, G., and Leech, R. (2014). Network dysfunction after traumatic brain injury. Nat. Rev. Neurol. 10, 156–166. doi: 10.1038/nrneurol.2014.15
Su, Y. Y., Zhang, X. D., Schoepf, U. J., Varga-Szemes, A., Stubenrauch, A., Liang, X., et al. (2016). Lower functional connectivity of default mode network in cognitively normal young adults with mutation of APP, presenilins and APOE ε4. Brain Imaging Behav. 11, 818–828. doi: 10.1007/s11682-016-9556-z
Vannorsdall, T. D., Jinnah, H. A., Gordon, B., Kraut, M., and Schretlen, D. J. (2008). Cerebral ischemia mediates the effect of serum uric acid on cognitive function. Stroke 39, 3418–3420. doi: 10.1161/STROKEAHA.108.521591
Wen, X., Wu, X., Li, R., Fleisher, A. S., Reiman, E. M., Wen, X., et al. (2013). Alzheimer’s disease-related changes in regional spontaneous brain activity levels and inter-region interactions in the default mode network. Brain Res. 1509, 58–65. doi: 10.1016/j.brainres.2013.03.007
Yan, C., Cheung, B., Kelly, L., Colcombe, S., Craddock, R. C., Martino, A. D., et al. (2012). The motion crisis in functional connectomics: damage assessment and control for resting-state fMRI[C]. Brain Connect. 2:A22.
Yan, C. G., Wang, X. D., Zuo, X. N., and Zang, Y. F. (2016). DPABI: data processing & analysis for (resting-state) brain imaging. Neuroinformatics 14, 339–351. doi: 10.1007/s12021-016-9299-4
Yan, C. G., and Zang, Y. F. (2010). DPARSF: a MATLAB toolbox for “Pipeline” data analysis of resting-state fMRI. Front. Syst. Neurosci. 4:13. doi: 10.3389/fnsys.2010.00013
Zhang, Y. F., He, Y., Zhu, C. Z., Cao, Q. J., Sui, M. Q., Liang, M., et al. (2007). Altered baseline brain activity in children with ADHD revealed by resting-state functional MRI. Brain Dev. 29, 83–91. doi: 10.1016/j.braindev.2006.07.002
Zhao, N., Yuan, L. X., Jia, X. Z., Zhou, X. F., Deng, X. P., He, H. J., et al. (2018). Intra- and inter-scanner reliability of voxel-wise whole-brain analytic metrics for resting state fMRI. Front. Neuroinform. 12:54. doi: 10.3389/fninf.2018.00054
Zheng, L. J., Su, Y. Y., Wang, Y. F., Schoepf, U. J., Varga-Szemes, A., Pannell, J., et al. (2017). Different hippocampus functional connectivity patterns in healthy young adults with mutations of APP/Presenilin-1/2 and APOEε4. Mol. Neurobiol. 55, 1–12. doi: 10.1007/s12035-017-0540-4
Zhu, Y., Pandya, B., and Choi, H. (2012). Comorbidities of gout and hyperuricemia in the US general population: NHANES 2007–2008. Am. J. Med. 125, 679–687. doi: 10.1016/j.amjmed.2011.09.033
Keywords: uric acid, cognitive function, resting-stage functional magnetic resonance imaging, blood oxygen level dependent, amplitude of low-frequency fluctuation
Citation: Lin L, Zheng LJ, Joseph Schoepf U, Varga-Szemes A, Savage RH, Wang YF, Zhang H, Zhang XY, Lu GM and Zhang LJ (2019) Uric Acid Has Different Effects on Spontaneous Brain Activities of Males and Females: A Cross-Sectional Resting-State Functional MR Imaging Study. Front. Neurosci. 13:763. doi: 10.3389/fnins.2019.00763
Received: 07 January 2019; Accepted: 09 July 2019;
Published: 26 July 2019.
Edited by:
Pierrette Gaudreau, Université de Montréal, CanadaReviewed by:
Arrigo Francesco Cicero, University of Bologna, ItalyZhaowei Meng, Tianjin Medical University General Hospital, China
Copyright © 2019 Lin, Zheng, Joseph Schoepf, Varga-Szemes, Savage, Wang, Zhang, Zhang, Lu and Zhang. This is an open-access article distributed under the terms of the Creative Commons Attribution License (CC BY). The use, distribution or reproduction in other forums is permitted, provided the original author(s) and the copyright owner(s) are credited and that the original publication in this journal is cited, in accordance with accepted academic practice. No use, distribution or reproduction is permitted which does not comply with these terms.
*Correspondence: Long Jiang Zhang, kevinzhlj@163.com
†These authors have contributed equally to this work