- 1NMR Research Unit, Queen Square MS Centre, Department of Neuroinflammation, UCL Institute of Neurology, Faculty of Brain Sciences, University College London, London, United Kingdom
- 2Department of Brain and Behavioural Sciences, University of Pavia, Pavia, Italy
- 3Brain MRI 3T Mondino Research Center, IRCCS Mondino Foundation, Pavia, Italy
- 4Department of Radiology, School of Clinical Medicine, University of Cambridge, Cambridge, United Kingdom
- 5Neuroradiology Unit, IRCCS Mondino Foundation, Pavia, Italy
- 6Center for Medical Image Computing, Department of Medical Physics and Biomedical Engineering, University College London, London, United Kingdom
- 7Department of Electrical, Computer and Biomedical Engineering, University of Pavia, Pavia, Italy
- 8NMR Research Unit, Queen Square MS Centre, Department of Brain Repair and Rehabilitation, UCL Institute of Neurology, Faculty of Brain Sciences, University College London, London, United Kingdom
- 9Universitat Oberta de Catalunya, Barcelona, Spain
- 10Brain Connectivity Center, IRCCS Mondino Foundation, Pavia, Italy
Brain function has been investigated via the blood oxygenation level dependent (BOLD) effect using magnetic resonance imaging (MRI) for the past decades. Advances in sodium imaging offer the unique chance to access signal changes directly linked to sodium ions (23Na) flux across the cell membrane, which generates action potentials, hence signal transmission in the brain. During this process 23Na transiently accumulates in the intracellular space. Here we show that quantitative functional sodium imaging (fNaI) at 3T is potentially sensitive to 23Na concentration changes during finger tapping, which can be quantified in gray and white matter regions key to motor function. For the first time, we measured a 23Na concentration change of 0.54 mmol/l in the ipsilateral cerebellum, 0.46 mmol/l in the contralateral primary motor cortex (M1), 0.27 mmol/l in the corpus callosum and -11 mmol/l in the ipsilateral M1, suggesting that fNaI is sensitive to distributed functional alterations. Open issues persist on the role of the glymphatic system in maintaining 23Na homeostasis, the role of excitation and inhibition as well as volume distributions during neuronal activity. Haemodynamic and physiological signal recordings coupled to realistic models of tissue function will be critical to understand the mechanisms of such changes and contribute to meeting the overarching challenge of measuring neuronal activity in vivo.
Introduction
Ever since the BOLD effect was described, fMRI has dominated neuroscience as a mean to evaluate brain activity (Ogawa et al., 1990a,b, 1992). It allows mapping of signal changes generated by the mismatch between oxygen delivery and consumption upon neuronal activation. While providing significant insight into brain function, a major limitation of BOLD is that it is an indirect measure and is affected by subject-specific haemodynamic factors. Thus, an approach that could directly measure neuronal activity in humans in vivo, non-invasively, would have a major advantage over BOLD-fMRI.
In this paper, we propose that, thanks to sodium MRI technology, we are within reach of measuring directly a local transient change of sodium ions (23Na) concentration in the intracellular space. During activity, neuronal action potentials cause a transient 23Na flux from the extracellular to intracellular space over a temporal scale of several milliseconds. If sodium imaging, dynamically repeated, was successful in detecting 23Na concentration changes evoked by specific tasks, it would open up a new way of investigating human brain function, complementing BOLD-fMRI.
Imaging aspects of the brain electrical activity, other than BOLD, related to transmembrane sodium-potassium ion exchange during depolarization could provide a direct access to primary brain function everywhere. Although sodium channels are predominantly located in the axonal initial segment (Häusser and Clark, 1997; Chadderton et al., 2004; Rancz et al., 2007; Masoli et al., 2015; Powell et al., 2015; Dover et al., 2016), they are also expressed in Ranvier nodes along the WM axons. While BOLD signals capture mainly the large energy demand supporting brain function related to the sodium-potassium pump to re-establish ionic gradients after action potentials in GM (Brockhaus et al., 1993; Koch and Barish, 1994), 23Na concentration changes could be sensitive to activity also in WM and therefore contribute to our understanding of brain circuits involved in specific tasks.
Despite its limitations, BOLD-fMRI has been very successful in neurological research applications to study mechanisms of disease. Pathologies where blood perfusion is impaired, such as multiple sclerosis (Paling et al., 2013) and stroke (Sakatani et al., 2007), reveal alterations during task BOLD fMRI. However, these may be mediated by a dysfunction in evoked blood oxygenation or by neuronal damage itself. Experimental neurophysiology also indicates that psychiatric conditions, such as autism, are characterized by altered patterns of neuronal firing, which are difficult to capture in vivo using BOLD-fMRI (Giza et al., 2010; Leblond et al., 2014). Considering its substantial research output, BOLD-fMRI is rarely used clinically, though, besides pre-surgical planning. Yet, while growing evidence supports that minimizing residual tumor mass improves survival, false functional localisation may render it less effective (Morrison et al., 2016; Suchorska et al., 2016). This means that there is a pressing need for tools able to directly map brain function, rather than through haemodynamic effects, and with greater reliability. Again, measuring 23Na concentration changes could meet this need, complementing BOLD-fMRI, with the potential of impacting clinical practice.
From a physiological point of view, neuronal cells’ function has recently been mapped in vitro with high specificity, describing distribution and functionality of 23Na channels with incredible details (Masoli et al., 2015; Dover et al., 2016). In parallel, physiological studies have also led to a better understanding of the neurovascular coupling (Howarth et al., 2009; Lippert et al., 2010; Mapelli et al., 2016) at the origin of the BOLD-fMRI signal (Ogawa et al., 1990a,b, 1992). These data are the bases for constructing emerging realistic models of neuronal activity, built on ever accurate physiological recordings of cellular function, and could provide an invaluable tool to interpret large scale measures of brain function from MRI (Blanchard et al., 2016; D’Angelo and Wheeler-Kingshott, 2017; Friston et al., 2017).
From a technological point of view, it is now feasible to measure 23Na concentrations in vivo, which are key in retaining physiologically balanced tissues. Indeed, it is now possible to non-invasively measure quantitatively total (i.e., intra + extra cellular) 23Na concentrations (TSC) of the human brain tissue in vivo using high field MRI scanners (Thulborn, 2018). Furthermore, 23Na in the intra and extracellular spaces have different MR properties due to their cellular environment, hence any alteration in volume fractions or in intra or extracellular 23Na concentrations could affect the measured TSC. Arguably one could say that TSC is sensitive to changes due to tissue composition as well as to pathological changes of cellularity, albeit with a lower sensitivity than proton (1H), or indeed due to transient TSC changes (ΔTSC) during functional activity.
With preliminary data and biophysical hypothesis of ΔTSC changes in tissue in vivo, we intend to establish a framework for developing fNaI, demonstrating an exciting opportunity for measuring brain function and potentially neuronal activity, addressing a pressing need for a multi-disciplinary integration.
Materials and Methods
Subjects
Eight right-handed healthy volunteers (mean age 33 years, range 27–45, 5 males) gave written consent to this study approved by the NRES Committee London – Harrow, in accordance with the Declaration of Helsinki.
fNaI Acquisition Protocol
Data was acquired on a 3T Philips Achieva system (Philips, Netherlands) with a single-tuned volume head-coil (Rapid, Germany) using a 4-times undersampled 3D-Cones ultra-short echo time sequence (Gurney et al., 2006; Riemer et al., 2014), 4 mm isotropic resolution, 240 mm field-of-view, 90° flip-angle, TR = 50 ms, TE = 0.22 ms, 6 NEX, total scan time per volume = 60 s. TE was defined as from the end of the pulse to the start of readout (0.22 ms). The RF pulse was 320 μs, so the time from the center of the RF pulse to the start of readout is 0.38 ms. The length of the readout was 30 ms.
fNaI Paradigm Design
Functional sodium imaging was performed back-to-back 6-times (3-rest conditions interleaved with 3-tasks). Subjects were asked (verbally) to perform a right-hand finger-tapping task (self-pacing at a frequency of 1 Hz), opposing the thumb to each one of the fingers, repeatedly from the index to the little finger and back, with ample extension of the movements.
fNaI Data Analysis
Images were reconstructed to 2 mm isotropic resolution using SNR-enhancing sub-Nyquist k-space sample weighting (Pipe, 2000). All analyses were performed with SPM8. Images were rigidly registered, smoothed with a 8 mm × 8 mm × 8 mm Gaussian-kernel and normalized to the proton density (PD) MNI152 template. Statistical analysis was performed using the SPM8-PET group analysis toolbox. Statistical maps were calculated with p = 0.001, cluster extent of k = 20 voxels and family-wise error (FWE) correction.
fNaI Clusters Identification
Maps of t-statistics were saved from the fNaI data analysis and imported in the xjview toolbox1 of SPM for a detailed cluster report in terms of peak, number of voxels, location, and anatomical areas involved in Tailarach atlas space (Yoon et al., 2012).
Sodium Ions (23Na) Flux and ΔTSC
Voxel-wise TSC was calculated according to (Christensen et al., 1996), using two reference phantoms (33 and 66 mmol/l sodium agar) placed either side of the brain for 4 out of 8 volunteers. ΔTSC was calculated from TSC on/off maps for cerebellar, ipsi, and contralateral M1 and corpus callosum (CC) clusters and reported as (mean ΔTSC ± standard deviation) across the four subjects.
Results
Functional sodium imaging was successfully performed in eight subjects during a right-hand finger-tapping task at 3T. Figure 1a) shows transverse slices from one fNaI volume of a randomly chosen subject, while Figure 1b) shows fNaI statistical activation maps from the group analysis. A total of 16 main clusters were identified and are reported in Table 1. These include the contralateral M1 (Precentral), somatosensory (Postcentral) and supplementary motor (Superior Frontal) gyri, and the ipsilateral anterior cerebellum (lobule I-IV) as well as lobule VI, Crus I-II and the dentate nucleus (Figure 1c). Noticeable are the large number of ipsilateral areas that were also activated in the ipsilateral cerebrum (e.g., frontal and temporal lobes, postcentral gyrus, deep GM including the right thalamus, the insular cortex, the limbic lobe, and parahippocampal gyrus. The lingual gyrus, Brodmann areas (BA) 2, 19, and 37 were also activated ipsilaterally to the movement). Interestingly, WM areas were identified in the CC, contralateral paracentral lobule and medial frontal gyrus, corticospinal tract (CST), posterior cingulum, and ipsilateral supramarginal gyrus.
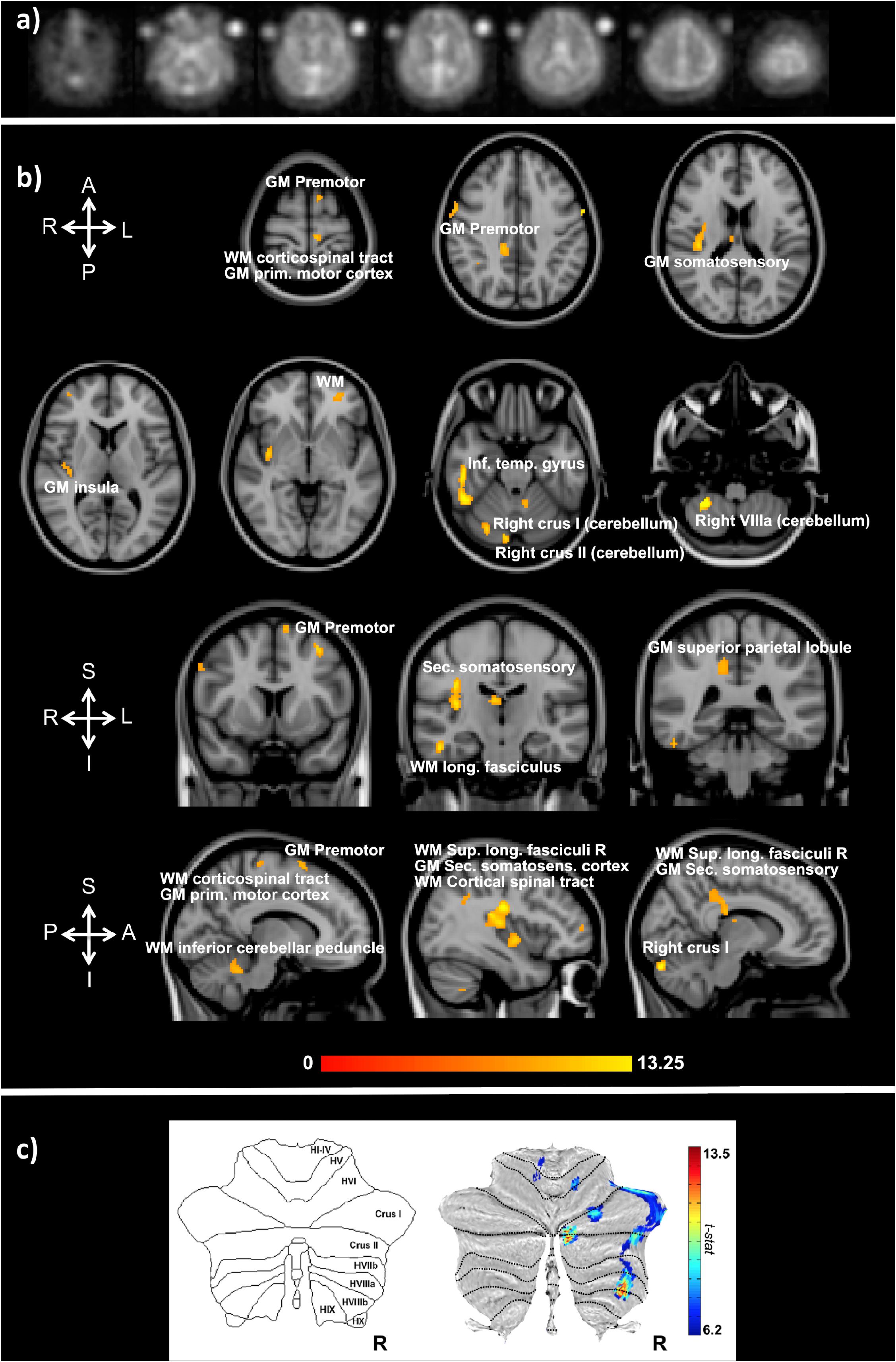
FIGURE 1. (a) Example of transverse slices from a single functional sodium imaging (fNaI) volume after smoothing. The circles either side of the brain are known concentration phantoms. Signal to noise ratio in WM was measured as (17.5 ± 1.4) a.u. in all eight subjects. (b) Activation clusters from proof of concept fNaI experiment where the subject performed a 1 Hz finger-tapping paradigm. Results are from group analysis of eight volunteers (FWE corrected, p < 0.001, 20 voxels), overlaid on 3D T1-weighted structural images with anatomical annotations. Maps were poorly localized at p < 0.05, hence the higher than usual threshold. Worth noticing that signal changes for fNaI were of the order of 10%, which is twice what is normally detected using BOLD-fMRI. Activations are seen in motor-function related areas. (c) The cerebellum shows enhanced activations in Crus I/II and lobule VI related to finger tapping and motor planning. GM, gray matter; WM, white matter.
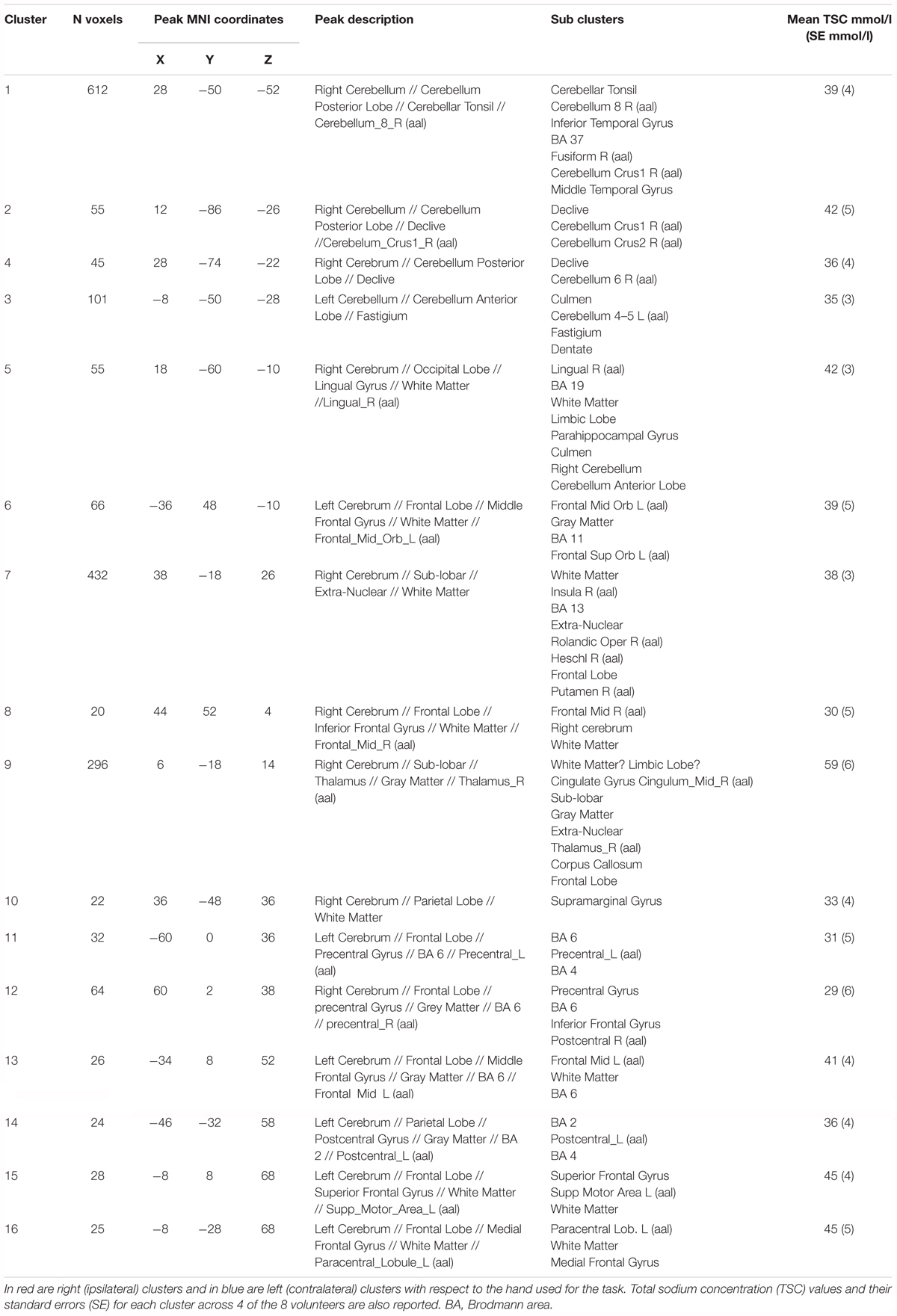
TABLE 1. Clusters of activations and identification of areas involved according to the Tailarach atlas in XJVIEW. Total sodium concentration (TSC) and standard error (SE) for each area of activation is also reported.
ΔTSC in the anterior cerebellar cluster was (0.54 ± 0.17) mmol/l, while it was (0.27 ± 0.08) mmol/l in the CC (0.46 ± 0.10) mmol/l in the contralateral M1; a negative ΔTSC change was measured in the ipsilateral M1 (-0.11 ± 0.06) mmol/l.
Discussion
We have shown preliminary evidence that brain function can be assessed non-invasively by sodium imaging in vivo using a 3T MRI scanner, a major step forward in the overarching aim to directly assess neuronal activity. Indeed, fNaI successfully detected changes in activation between finger tapping and rest across the entire brain. Activations in motor and executive control areas indicate that fNaI has the potential to be an effective biomarker of functional activity.
The localisation of activated regions is conveying interesting results. Several clusters are at the border between GM and WM, where a high concentration of sodium channels in the axon initial segment could lead to a large intracellular 23Na accumulation (Dover et al., 2016). The task employed in this initial experiment was indeed demanding: 1 min of self-paced finger taping requires motor planning and concentration. Interestingly, as well as M1 (BA4), the study shows activations in the primary somatosensory cortex (BA2), i.e., the main cortical area for processing the sense of touch, and in the premotor cortex (BA6), which is involved (with the cerebellum) in self-pacing finger tapping (Witt et al., 2008; Mak et al., 2016). In the cerebellum, activations occurred in posterior ipsilateral areas, namely Crus I/II, lobule VI, and lobule VIIIa, which are heavily involved in integrative aspects of motor control and cognitive processing (Witt et al., 2008; Mak et al., 2016). A further finding is the presence of patches of activity in WM, including: the CST, which is the major pathway for the motor system; the superior longitudinal fasciculus that connects parietal to prefrontal cortices with associative fibers integrating body awareness and perception; the CC, which connects both hemispheres; the cingulum, which receives afferent fibers from the thalamus, as part of the spino-thalamic tract. These tracts are myelinated and enriched in sodium channels at the nodes of Ranvier, forming the axonal pathways wiring-up the sensorimotor network. Given that ionic fluxes (including those involving astrocytes) are generally smaller in WM than GM, it will be important to verify these activations in future studies, to exclude possible partial volume effects and to assess whether neurotransmitter signaling could cause enough accumulation of 23Na at synapsis junctions to be detectable with fNaI. If these results were going to be confirmed, reconstruction of axonal circuits supporting functions would find invaluable information in WM matter fNaI results.
From the present data it is impossible to determine the mechanisms underlying fNaI changes, i.e., does ΔTSC reflect sensitivity mainly to the shift of 23Na between the intracellular and extracellular compartments (Gilles et al., 2017), or does it reflect also changes in vascular and perivascular spaces through neurovascular coupling between glucose metabolism and increase blood delivery? In other words, we cannot exclude that in the present measurements, a significant contribution comes from the blood. Moreover, given the experimental TR, T1-weighting could play a role in the contrast: any excess of blood-related signal flowing through the vessels in activated regions could lead to artefactual increases in sodium signal. Future fNaI studies should consider minimizing this possible effect.
Whilst a precise estimate would require sophisticated models and combinations of experimental measurements in vivo and in vitro, here we can probe likely/expected scenarios and propose some ab initio calculations. Can the molar flux of 23Na displaced during activity be sufficient to generate a meaningful fNaI signal? For example, in the cerebellum, ΔTSC was 0.54 ± 0.17 mmol/l. The measured ATP consumption during activity in the cerebellar cortex is 20.5 mmol of ATP/(g.min) (Sokoloff et al., 1977; Howarth et al., 2009, 2012). Of this ATP, ∼50% is used for computation while the other ∼50% for maintenance (Brockhaus et al., 1993; Koch and Barish, 1994; Howarth et al., 2009, 2012), so about 10 mmol of ATP/(g.min) are used for function. One ATP corresponds to shifting three 23Na (previously accumulated inside the cell) through the cell membrane to re-establish ionic balance; this means that during activity there is a shift of the order of 30 mmol/(g.min) of 23Na, i.e., 30∗103mmol/(l.min) of 23Na, which is orders of magnitude larger than our measured value. Therefore, there is sufficient 23Na displacement to possibly explain the fNaI signal. The larger 23Na displacement expected from calculations compared to ΔTSC is likely to reflect the fact that, while 23Na enters through sodium channels during the action potentials, soon thereafter it leaves the cell through sodium-potassium pumps and sodium exchangers. What is established during a given time-frame is a dynamic equilibrium between 23Na influx and efflux with a residual unbalance, that is potentially captured by the measured ΔTSC. But there are yet other sources of 23Na flux that should be considered. Other fluxes that contribute to reaching equilibrium are due to 23Na co-transport with other ions, metabolites and neurotransmitters in neurons and glial cells, in support of the energy budget during activation (Dienel and Cruz, 2008; Hertz et al., 2015; DiNuzzo et al., 2017). How all of these contribute to ΔTSC sign and magnitude remains to be discovered. Furthermore, with the current 4 mm × 4 mm × 4 mm resolution of fNaI, it is also possible that this displacement – which happens on a microstructure scale – is actually diluted or even averaged out. It is also important to assess the contribution of changes in cerebral blood volume (CBV) during brain function and changes in Virchow-Robin space volume (VRSV) and the glymphatic system. During functional activity CBV changes because of the arteriole (CBVa), where CBVa at rest is of the order of 0.8 ml/100 g, with a change of ΔCBVa = 0.34 ml/100 g (Lee et al., 2001; Kim et al., 2007; Hua et al., 2011). This is transferred to the capillaries, where the venous side passively follows the arterioles dilation and resistance changes, which induce blood flow and volume changes (Buxton, 2012). If ΔCBVa is added to the extracellular space compartment, reducing the cellular volume fraction even by as little as 0.5%, this would be enough to cause ΔTSC of the order of the measured one, given the 10 times higher extracellular molar concentration. This ΔCBVa, though, would not affect the ΔTSC measured if its fractional volume was balanced by a corresponding reduction in VRSV, i.e., if the extracellular space (CBV + extracellular matrix + VRSV) and the cellular space (e.g., intracellular space + myelin) proportions remained constant. In this hypothesis, the perivascular space and therefore the glymphatic system, would work as a compensatory chamber, with “rigid” neuronal structures within the extracellular matrix (Thulborn, 2018). The cerebrospinal fluid role in buffering the changes in CBV during functional activity has been investigated and reported to be reduced during activation, using relaxometry in the visual cortex, which would support this hypothesis (Piechnik et al., 2009).
On the other hand, we must not forget that the ΔTSC measured experimentally in this study in vivo is an “apparent” TSC change, as the current acquisition protocol cannot distinguish between the intra and extracellular sodium, but can only record the overall TSC, sensitive to both altered volume fractions or altered intra and extra cellular concentrations. Moreover, the spatial resolution of sodium imaging at 3T is poor (with a nominal resolution of 4 mm × 4 mm × 4 mm), which implies that our measurements are currently affected by partial volume. In particular, head motion could affect voxels adjacent to CSF, and be responsible for increase or decrease ΔTSC in such areas. However, acquisition of a sufficient number of (control – rest) images should provide statistical power to account for any motion not sufficiently corrected by registration. Furthermore, the functional paradigm here is non-standard and would benefit from faster image acquisition methods to increase the temporal resolution of the experiment. Nevertheless, the results presented here are all statistically significant and FWE corrected (p < 0.001, 20 voxels).
Ultimately, one could speculate on the tissue composition of an imaging voxel further and try to assess the potential contribution to ΔTSC coming from many compartments, defining, e.g., fractions of the axonal volume, soma, myelin, extra cellular matrix (including astrocytes), VRSV (or CSF) space, and CBV. A comprehensive model should consider exchange of 23Na at synapsis and in other cells such as glial cells, be adapted for different brain regions and different functional tasks (Alahmadi et al., 2017).
This is therefore a proposal for a novel framework, essential for advancing our understanding of the human brain function, where knowledge must bridge gaps between cellular and large-scale systems (D’Angelo and Wheeler-Kingshott, 2017). Dedicated major efforts should be employed to speed up acquisition and at the same time improve spatial resolution of sodium imaging. Potentially, this emerging and exciting field of research could greatly benefit from higher field strength systems (e.g., 7T) (Riemer et al., 2015; Ranjeva et al., 2018). In order to disentangle the sources of the quantitative ΔTSC from fNaI, it would be important to design multi-modal studies that assess a number of variables, such as CBV, CBF, oxygen consumption rates and metabolism for a better estimate of brain energy dynamics (Germuska et al., 2018). Models of fNaI changes could be validated using a range of hemodynamic and physiological signal recordings, including, e.g., magnetoencephalography (MEG), near infrared spectroscopy (NIRS) and positron emission tomography (PET), (Shibasaki, 2008).
Conclusion
In conclusion, sodium changes during activity are sufficiently large to be detected and quantified using fNaI in vivo. Preliminary quantitative data show encouraging results in terms of coherence of the ΔTSC values between cerebellum and M1 (0.54 vs. 0.46 mmol/l). Interpretations of the reduced value of ΔTSC in the CC (0.27 mmol/l) and of the negative ΔTSC in the ipsilateral M1 (-11 mmol/l) (Hamzei et al., 2002) must be cautious and deferred to future studies. Improvements in data acquisition and computational modeling of neurovascular coupling in relation to 23Na flux during action potential generation and maintenance could open a new way forward to assess neuronal activation in humans in vivo non-invasively.
Author Contributions
CGW-K developed the idea of fNaI and contributed to all aspects of its realisation. FR contributed to the implementation and data acquisition and analysis. FPa contributed to discussion on feasibility. AR helped with data acquisition. GC contributed to image analysis. XG participated to the development of sodium imaging and useful discussion. FPr contributed to data reconstruction and analysis. BS contributed to image acquisition and development. ED’A supported the idea development and contributed with physiological interpretation.
Funding
The NMR unit where this work was performed was supported by grants from the UK Multiple Sclerosis Society and was supported by the UCL/UCLH NIHR (National Institute for Health Research) BRC (Biomedical Research Centre). CGW-K also received funding from the Horizon 2020 EU programme [H2020-EU.3.1 (634541)], ISRT, Wings for Life, CHNF. GC has an ECTRIMS fellowship. FR was funded for the duration of this study by the Medical Research Council (MRC). FPr has a non-Clinical Postdoctoral Guarantors of Brain fellowship. FPa was supported by the Italian Ministry of Health (NET2013-02355313). ED’A received grants from the Human Brain Project, Centro Fermi, and the Italian Ministry of Health.
Conflict of Interest Statement
The authors declare that the research was conducted in the absence of any commercial or financial relationships that could be construed as a potential conflict of interest.
Abbreviations
23Na, sodium ions; ATP, adenosine triphosphate; BOLD, blood oxygenation level dependent; fMRI, functional magnetic resonance imaging; fNaI, functional sodium imaging; GM, gray matter; M1, primary motor cortex; MRI, magnetic resonance imaging; TSC, total sodium concentration; WM, white matter.
Footnotes
References
Alahmadi, A. A. S., Samson, R. S., Pardini, M., D’Angelo, E., Friston, K. J., Toosy, A. T., et al. (2017). Investigating the relationship between multiple grip forces and BOLD signal in the cerebellum and dentate nuclei of MS subjects. Front. Abstract Book 62–66. doi: 10.3389/978-2-88945-240-8
Blanchard, S., Saillet, S., Ivanov, A., Benquet, P., Bénar, C. G., Pélégrini-Issac, M., et al. (2016). A new computational model for neuro-glio-vascular coupling: astrocyte activation can explain cerebral blood flow nonlinear response to interictal events. PLoS One 11:e0147292. doi: 10.1371/journal.pone.0147292
Brockhaus, J., Ballanyi, K., Smith, J. C., and Richter, D. W. (1993). Microenvironment of respiratory neurons in the in vitro brainstem-spinal cord of neonatal rats. J. Physiol. 462, 421–445. doi: 10.1113/jphysiol.1993.sp019562
Buxton, R. B. (2012). Dynamic models of BOLD contrast. Neuroimage 62, 953–961. doi: 10.1016/j.neuroimage.2012.01.012
Chadderton, P., Margie, T. W., and Häusser, M. (2004). Integration of quanta in cerebellar granule cells during sensory processing. Nature 428, 856–860. doi: 10.1038/nature02442
Christensen, J. D., Barrere, B. J., Boada, F. E., Vevea, J. M., and Thulborn, K. R. (1996). Quantitative tissue sodium concentration mapping of normal rat brain. Magn. Reson. Med. 36, 83–89.
D’Angelo, E., and Wheeler-Kingshott, C. A. M. (2017). Modelling the brain: elementary components to explain ensemble functions. Riv. Del Nuovo Cim. 40, 297–333.
Dienel, G. A., and Cruz, N. F. (2008). Imaging brain activation: simple pictures of complex biology. Ann. N. Y. Acad. Sci. 1147, 139–170. doi: 10.1196/annals.1427.011
DiNuzzo, M., Giove, F., Maraviglia, B., and Mangia, S. (2017). Computational flux balance analysis predicts that stimulation of energy metabolism in astrocytes and their metabolic interactions with neurons depend on uptake of k+ rather than glutamate. Neurochem. Res. 42, 202–216. doi: 10.1007/s11064-016-2048-0
Dover, K., Marra, C., Solinas, S., Popovic, M., Subramaniyam, S., Zecevic, D., et al. (2016). FHF-independent conduction of action potentials along the leak-resistant cerebellar granule cell axon. Nat. Commun. 7:12895. doi: 10.1038/ncomms12895
Friston, K. J., Preller, K. H., Mathys, C., Cagnan, H., Heinzle, J., Razi, A., et al. (2017). Dynamic causal modelling revisited. Neuroimage doi: 10.1016/j.neuroimage.2017.02.045 [Epub ahead of print].
Germuska, M., Chandler, H. L., Stickland, R. C., Foster, C., Fasano, F., Okell, T. W., et al. (2018). Dual-calibrated fMRI measurement of absolute cerebral metabolic rate of oxygen consumption and effective oxygen diffusivity. Neuroimage 184, 717–728. doi: 10.1016/j.neuroimage.2018.09.035
Gilles, A., Nagel, A. M., and Madelin, G. (2017). Multipulse sodium magnetic resonance imaging for multicompartment quantification: proof-of-concept. Sci. Rep. 7:17435. doi: 10.1038/s41598-017-17582-w
Giza, J., Urbanski, M. J., Prestori, F., Bandyopadhyay, B., Yam, A., Friedrich, V., et al. (2010). Behavioral and cerebellar transmission deficits in mice lacking the autism-linked gene islet brain-2. J. Neurosci. 30, 14805–14816. doi: 10.1523/jneurosci.1161-10.2010
Gurney, P. T., Hargreaves, B. A., and Nishimura, D. G. (2006). Design and analysis of a practical 3D cones trajectory. Magn. Reson. Med. 5, 575–582. doi: 10.1002/mrm.20796
Hamzei, F., Dettmers, C., Rzanny, R., Liepert, J., Büchel, C., and Weiller, C. (2002). Reduction of excitability (“inhibition”) in the ipsilateral primary motor cortex is mirrored by fMRI signal decreases. Neuroimage 17, 490–496. doi: 10.1006/nimg.2002.1077
Häusser, M., and Clark, B. A. (1997). Tonic synaptic inhibition modulates neuronal output pattern and spatiotemporal synaptic integration. Neuron 19, 665–678. doi: 10.1016/S0896-6273(00)80379-7
Hertz, L., Xu, J., Song, D., Du, T., Li, B., Yan, E., et al. (2015). Astrocytic glycogenolysis: mechanisms and functions. Metab. Brain Dis. 30, 317–333. doi: 10.1007/s11011-014-9536-1
Howarth, C., Gleeson, P., and Attwell, D. (2012). Updated energy budgets for neural computation in the neocortex and cerebellum. J. Cereb. Blood Flow Metab. 32, 1222–1232. doi: 10.1038/jcbfm.2012.35
Howarth, C., Peppiatt-Wildman, C. M., and Attwell, D. (2009). The energy use associated with neural computation in the cerebellum. J. Cereb. Blood Flow Metab. 30, 403–414. doi: 10.1038/jcbfm.2009.231
Hua, J., Qin, Q., Pekar, J. J., and van Zijl, P. C. M. (2011). Measurement of absolute arterial cerebral blood volume in human brain without using a contrast agent. NMR Biomed. 24, 1313–1325. doi: 10.1002/nbm.1693
Kim, T., Hendrich, K. S., Masamoto, K., and Kim, S. G. (2007). Arterial versus total blood volume changes during neural activity-induced cerebral blood flow change: implication for BOLD fMRI. J. Cereb. Blood Flow Metab. 27, 1235–1247. doi: 10.1038/sj.jcbfm.9600429
Koch, R. A., and Barish, M. E. (1994). Perturbation of intracellular calcium and hydrogen ion regulation in cultured mouse hippocampal neurons by reduction of the sodium ion concentration gradient. J. Neurosci. 14(5 Pt 1), 2585–2593.
Leblond, C. S., Nava, C., Polge, A., Gauthier, J., Huguet, G., Lumbroso, S., et al. (2014). Meta-analysis of SHANK mutations in autism spectrum disorders: a gradient of severity in cognitive impairments. PLoS Genet. 10:e1004580. doi: 10.1371/journal.pgen.1004580
Lee, S. P., Duong, T. Q., Yang, G., Iadecola, C., and Kim, S. G. (2001). Relative changes of cerebral arterial and venous blood volumes during increased cerebral blood flow: implications for bold fMRI. Magn. Reson. Med. 45, 791–800. doi: 10.1002/mrm.1107
Lippert, M. T., Steudel, T., Ohl, F., Logothetis, N. K., and Kayser, C. (2010). Coupling of neural activity and fMRI-BOLD in the motion area MT. Magn. Reson. Imaging 28, 1087–1094. doi: 10.1016/j.mri.2009.12.028
Mak, M. K., Cheung, V., Ma, S., Lu, Z. L., Wang, D., Lou, W., et al. (2016). Increased cognitive control during execution of finger tap movement in people with Parkinson’s disease. J. Parkinsons Dis. 6, 639–650. doi: 10.3233/JPD-160849
Mapelli, L., Gagliano, G., Soda, T., Laforenza, U., Moccia, F., and D’Angelo, E. (2016). Granular layer neurons control cerebellar neurovascular coupling through an NMDA receptor/NO-dependent system. J. Neurosci. 37, 1340–1351. doi: 10.1523/jneurosci.2025-16.2016
Masoli, S., Solinas, S., and D’Angelo, E. (2015). Action potential processing in a detailed Purkinje cell model reveals a critical role for axonal compartmentalization. Front. Cell. Neurosci. 9:47. doi: 10.3389/fncel.2015.00047
Morrison, M. A., Tam, F., Garavaglia, M. M., Hare, G. M. T., Cusimano, M. D., Schweizer, T. A., et al. (2016). Sources of variation influencing concordance between functional MRI and direct cortical stimulation in brain tumor surgery. Front. Neurosci. 10:461. doi: 10.3389/fnins.2016.00461
Ogawa, S., Lee, T. M., Nayak, A. S., and Glynn, P. (1990b). Oxygenation-sensitive contrast in magnetic resonance image of rodent brain at high magnetic fields. Magn. Reson. Med. 14, 68–78. doi: 10.1002/mrm.1910140108
Ogawa, S., Lee, T. M., Kay, A. R., and Tank, D. W. (1990a). Brain magnetic resonance imaging with contrast dependent on blood oxygenation. Proc. Natl. Acad. Sci. U.S.A. 87, 9868–9872.
Ogawa, S., Tank, D. W., Menon, R., Ellermann, J. M., Kim, S. G., Merkle, H., et al. (1992). Intrinsic signal changes accompanying sensory stimulation: functional brain mapping with magnetic resonance imaging. Proc. Natl. Acad. Sci. U.S.A. 89, 5951–5955.
Paling, D., Thade Petersen, E., Tozer, D. J., Altmann, D. R., Wheeler-Kingshott, C. A. M., Kapoor, R., et al. (2013). Cerebral arterial bolus arrival time is prolonged in multiple sclerosis and associated with disability. J. Cereb. Blood Flow Metab. 34, 34–42. doi: 10.1038/jcbfm.2013.161
Piechnik, S. K., Evans, J., Bary, L. H., Wise, R. G., and Jezzard, P. (2009). Functional changes in CSF volume estimated using measurement of water T2 relaxation. Magn. Reson. Med. 61, 579–586. doi: 10.1002/mrm.21897
Pipe, J. G. (2000). Reconstructing MR images from undersampled data: data-weighting considerations. Magn. Reson. Med. 43, 867–875.
Powell, K., Mathy, A., Duguid, I., and Häusser, M. (2015). Synaptic representation of locomotion in single cerebellar granule cells. eLife 4:e07290. doi: 10.7554/eLife.07290
Rancz, E. A., Ishikawa, T., Duguid, I., Chadderton, P., Mahon, S., and Häusser, M. (2007). High-fidelity transmission of sensory information by single cerebellar mossy fibre boutons. Nature 450, 1245–1248. doi: 10.1038/nature05995
Ranjeva, J. P., Bydder, M., Ridley, B., Soubrier, M., Bertinetti, M., Guye, M., et al. (2018). Functional sodium (23Na) MRI at 7T - Extracellular sodium decreases during cortical activation in the human brain. Proc. Int. Soc. Magn. Reson. Med. 26:707.
Riemer, F., Solanky, B. S., Golay, X., D’Angelo, E., and Wheeler-Kingshott, C. A. M. (2015). Sodium fMRI detects grey and white matter activations: neuronal firing or blood volume change? Proc. Int. Soc. Magn. Reson. Med. 23:3924.
Riemer, F., Solanky, B. S., Stehning, C., Clemence, M., Wheeler-Kingshott, C. A. M., and Golay, X. (2014). Sodium ((23)Na) ultra-short echo time imaging in the human brain using a 3D-Cones trajectory. MAGMA 27, 35–46. doi: 10.1007/s10334-013-0395-2
Sakatani, K., Murata, Y., Fujiwara, N., Hoshino, T., Nakamura, S., Kano, T., et al. (2007). Comparison of blood-oxygen-level-dependent functional magnetic resonance imaging and near-infrared spectroscopy recording during functional brain activation in patients with stroke and brain tumors. J. Biomed. Opt. 12:062110. doi: 10.1117/1.2823036
Shibasaki, H. (2008). Human brain mapping: hemodynamic response and electrophysiology. Clin. Neurophysiol. 119, 731–743. doi: 10.1016/j.clinph.2007.10.026
Sokoloff, L., Reivich, M., Kennedy, C., Rosiers, M. H., Des Patlak, C. S., Pettigrew, K. D., et al. (1977). The [14 C]deoxyglucose method for the measurement of local cerebral glucose utilization: theory, procedure, and normal values in the conscious and anesthetized albino rat. J. Neurochem. 28, 897–916. doi: 10.1111/j.1471-4159.1977.tb10649.x
Suchorska, B., Weller, M., Tabatabai, G., Senft, C., Hau, P., Sabel, M. C., et al. (2016). Complete resection of contrast-enhancing tumor volume is associated with improved survival in recurrent glioblastoma-results from the DIRECTOR trial. Neuro Oncol. 18, 549–556. doi: 10.1093/neuonc/nov326
Thulborn, K. R. (2018). Quantitative sodium MR imaging: a review of its evolving role in medicine. Neuroimage 168, 250–268. doi: 10.1016/j.neuroimage.2016.11.056
Witt, S. T., Laird, A. R., and Meyerand, M. E. (2008). Functional neuroimaging correlates of finger-tapping task variations: an ALE meta-analysis. Neuroimage 42, 343–356. doi: 10.1016/j.neuroimage.2008.04.025
Keywords: sodium imaging, functional imaging, neuronal activity, BOLD, MRI
Citation: Gandini Wheeler-Kingshott CAM, Riemer F, Palesi F, Ricciardi A, Castellazzi G, Golay X, Prados F, Solanky B and D’Angelo EU (2018) Challenges and Perspectives of Quantitative Functional Sodium Imaging (fNaI). Front. Neurosci. 12:810. doi: 10.3389/fnins.2018.00810
Received: 19 July 2018; Accepted: 17 October 2018;
Published: 09 November 2018.
Edited by:
Pedro Antonio Valdes-Sosa, Clinical Hospital of Chengdu Brain Science Institute, ChinaReviewed by:
Rob Stobbe, University of Alberta, CanadaFederico Giove, Centro Fermi – Museo Storico della Fisica e Centro Studi e Ricerche Enrico Fermi, Italy
Copyright © 2018 Gandini Wheeler-Kingshott, Riemer, Palesi, Ricciardi, Castellazzi, Golay, Prados, Solanky and D’Angelo. This is an open-access article distributed under the terms of the Creative Commons Attribution License (CC BY). The use, distribution or reproduction in other forums is permitted, provided the original author(s) and the copyright owner(s) are credited and that the original publication in this journal is cited, in accordance with accepted academic practice. No use, distribution or reproduction is permitted which does not comply with these terms.
*Correspondence: Claudia A. M. Gandini Wheeler-Kingshott, c.wheeler-kingshott@ucl.ac.uk
†These authors have contributed equally to this work