- 1Lab of Learning Sciences, Graduate School of Education, Peking University, Beijing, China
- 2The Affiliated Brain Hospital of Guangzhou Medical University (Guangzhou Hui’ai Hospital), Guangzhou, China
- 3Institute of Biomedical and Health Engineering, Shenzhen Institutes of Advanced Technology, Chinese Academy of Sciences, Shenzhen, China
Mounting studies have applied resting-state functional magnetic resonance imaging (rs-fMRI) to study major depressive disorder (MDD) and have identified abnormal functional activities. However, how the global functional connectivity patterns change in MDD is still unknown. Using rs-fMRI, we investigated the alterations of global resting-state functional connectivity (RSFC) patterns in MDD using weighted global brain connectivity (wGBC) method. First, a whole brain voxel-wise wGBC map was calculated for 23 MDD patients and 34 healthy controls. Two-sample t-tests were applied to compare the wGBC and RSFC maps and the significant level was set at p < 0.05, cluster-level correction with voxel-level p < 0.001. MDD patients showed significantly decreased wGBC in left temporal pole (TP) and increased wGBC in right parahippocampus (PHC). Subsequent RSFC analyses showed decreased functional interaction between TP and right posterior superior temporal cortex and increased functional interaction between PHC and right inferior frontal gyrus in MDD patients. These results revealed the abnormal global FC patterns and its corresponding disrupted functional connectivity in MDD. Our findings present new evidence for the functional interruption in MDD.
Introduction
Major depressive disorder (MDD) is a highly prevalent and worldwide psychiatric disorder causing severe societal and familial burdens (Mathers and Loncar, 2006). Brain structural changes, including gray matter volume of insula, amygdala, hippocampus, frontal and temporal cortex (Bora et al., 2012; Wang et al., 2017b), and surface morphological properties of hippocampus and amygdala have been widely reported in MDD patients (Chen et al., 2016). In addition, altered structural covariance between angular gyrus and amygdala, posterior cingulate cortex in MDD is also observed (Chen et al., 2017; Wu et al., 2017). Using resting-state functional magnetic resonance imaging (rs-fMRI), abnormal local brain activities in precuneus, cerebellum, lingual gyrus and inter-regional functional connectivity between subgenal anterior cingulate cortex and temporal cortex, between insula and thalamus, inferior parietal cortex, and between intraparietal sulcus and superior temporal gyrus (STG) were also identified (Wu et al., 2016a; Wang et al., 2017a,c; Sun et al., 2018; Wang J. et al., 2018). Moreover, using graph-theory method, disrupted whole brain functional topological organization of network has also been found (Gong and He, 2015). All these studies suggested that structure and function have changed in MDD. However, how and where the global functional connectivity patterns changed in MDD remains unclear.
A large number of literatures have revealed that brain function was constrained by its connectivity patterns (Passingham et al., 2002; Fan et al., 2014, 2016; Zhang et al., 2014; Wu et al., 2016b; Yang et al., 2016). A recently developed weighted global brain connectivity (wGCB) method can search for the global functional connectivity patterns based on resting-state functional connectivity (RSFC) MRI (Cole et al., 2010). Resting-state fMRI is a non-invasive way to study the functional interactions between different brain areas (Biswal et al., 1995; Wang et al., 2012, 2015a; Xu et al., 2015; Zhang et al., 2016; Wang L. et al., 2018). Resting-state fMRI has been widely adopted to characterize functional connectivity patterns to identify intrinsic functional modules (Fox et al., 2006; Buckner et al., 2009; Power et al., 2011; Yeo et al., 2011; Cole et al., 2014; Wang et al., 2015b, 2016b, 2017d; Mears and Pollard, 2016). It has also been applied to explore the abnormal functional couplings between brain areas to delineate brain intrinsic functional changes in disorders (Wang et al., 2016a; Wu et al., 2016c; Liu et al., 2018). wGCB can reveal global changes in the connectivity of a brain region by searching for globally connected or disconnected brain regions using a data-driven manner (Cole et al., 2010). Unlike the traditional seed-based or independent component analysis methods which can merely identify same spatial patterns of connectivity across subjects, the wGBC is less likely to be affected by within-region and between-subject spatial variations in connectivity patterns (Cole et al., 2011). Moreover, compared to unweighted GBC, wGBC does not need to threshold the connectivity strengths and can reveal globally connected regions with many low-strength connections removed by unweighted GBC thresholding. Therefore, wGCB provides a new way to study altered global functional connectivities to identify pathophysiology of MDD.
In this study, using resting-state fMRI, we examined the potentially abnormal global brain connectivity patterns and corresponding functional connectivity changes in 23 MDD patients and 34 gender-, age-, and education level-matched healthy controls (HC). First, we computed a voxel-wise wGCB maps for both MDD and HC to identify the abnormal global functional connectivities in MDD. Subsequently, we calculated the RSFC of the brain regions with changed wGCB to further reveal altered functional interactions in MDD.
Materials and Methods
Subjects
Twenty-three right-handed medication-free MDD patients and 34 right-handed HC subjects were recruited at the Department of Psychiatry at the Affiliated Brain Hospital of Guangzhou Medical University. The detailed information for MDD and HC subjects can be found in Table 1. MDD diagnosis was performed based on the Structured Clinical Interview of DSM-IV (SCID) criteria with 24-item Hamilton Depression Rating Scale. The HC subjects were recruited with SCID Non-Patient Edition. All the included MDD and HC subjects were out of serious medical, surgical illness, history of seizures, substance abuse, head trauma, and contraindications for MRI. All the subjects signed the written informed consent. All the experiments were approved by the ethics committees of the Affiliated Brain Hospital of Guangzhou Medical University.
Resting-State fMRI Data Acquisition
Resting-state fMRI data were acquired using an eight-channel 3.0-Tesla Philips MR scanner (Achieva X-series, the Netherlands) in the Department of Radiology, the Affiliated Brain Hospital of Guangzhou Medical University, China. The foam padding and earplugs were used to reduce head motion and to muffle scanner noise, respectively. During scanning, all the subjects were asked to stay awake, close their eyes, and think nothing. Resting-state fMRI data were scanned using an echo planar imaging sequence with the following parameters: repetition time = 2000 ms, echo time = 30 ms, flip angle = 900, field of view = 220 × 220 mm2, matrix = 64 × 64, 33 slices, slice thickness = 4 mm with 0.6 mm gap, and 240 volumes. The resting-state fMRI data have been used in our previous studies (Wu et al., 2016a; Wang et al., 2017a).
Resting-State fMRI Data Pre-processing
The resting-state data were pre-processed using SPM8 toolkit1. The pre-processing includes the following steps. discarding the first 10 volumes; slice timing; head motion correction; normalizing to MNI space; regressing motion parameters, white matter, cerebrospinal fluid, and global signals; and filtering with a temporal band-path of 0.01–0.1 Hz. To exclude the head motion effects, resting-state fMRI images with head-movement exceeded 1.5 mm of translation or 1.5 degrees of rotation in any direction were discarded if. In addition, “Scrubbing” method was also used to further reduce the effects of head motion if the frame displacement (FD) > 0.5 (Power et al., 2012). In our study, no frame was deleted because all subjects’ FD values were smaller than 0.3. For RSFC analyses, the resting-state data were first smoothed (6 mm FWHM) after normalization and then for the following pre-processing. We did not regress out the global signal to obtain reliable results because global signal regression will exaggerate anti-correlation.
wGCB Analysis
The calculation of voxel-wise wGCB map was constrained by a gray matter mask with gray matter probability value > 0.2 (Wu et al., 2016a). The wGBC was calculated as the following steps. First, each voxel of the gray mask was selected as the seed voxel. Next, Pearson’s correlations coefficient was calculated between each seed voxel and each of the whole brain voxel and transformed to z value using Fisher’s z transformation. Then, all the correlations were averaged and transformed back to r value for this voxel presenting the average connectivity (Cole et al., 2010). Using the same procedure, a whole brain wGCB map was obtained for each subject and smoothed using a 6 mm FWHM Gaussian kernel before statistical analyses. The distributions of wGCB in HC and MDD subjects were identified using one-sample t-tests and the significant level was set at p < 0.05, cluster-level correction with voxel-level p < 0.001.
To determine the group differences in wGCB, the two-sample t-test was used to compare the wGCB maps between HC and MDD patients with age, gender, and education as covariates. The significant level was set at p < 0.05, cluster-level correction with voxel-level p < 0.001 after using a cluster-level Monte Carlo simulation with 5000 times.
Functional Connectivity Analyses
Whole brain RSFC analyses were used to determine the changed functional connectivity of the brain regions with changed wGCB between MDD and HC. Functional connectivity was computed and transformed to z value using Fisher’s z transformation for each subject. A two-sample t-test was used to compare the functional connectivity maps between HC and MDD patients with age, gender, and education as covariates. The significant level was set at p < 0.05, cluster-level correction with voxel-level p < 0.001.
Results
Demographics and Clinical Characteristics
A chi-squared test and two-sample t-tests found that there were no significant differences in gender (p = 0.71), age (p = 0.69), and education level (p = 0.31) between HC and MDD groups.
wGCB Distribution in MDD and HC
Spatial distribution patterns of wGCB in MDD and HC found that the high wGCB were primarily located in the STG, lateral occipital gyrus, fusiform gyrus, intraparietal sulcus, medial temporal lobe, middle cingulate cortex, caudate, medial frontal cortex, lateral prefrontal cortex, inferior frontal cortex, and insula (Figure 1).
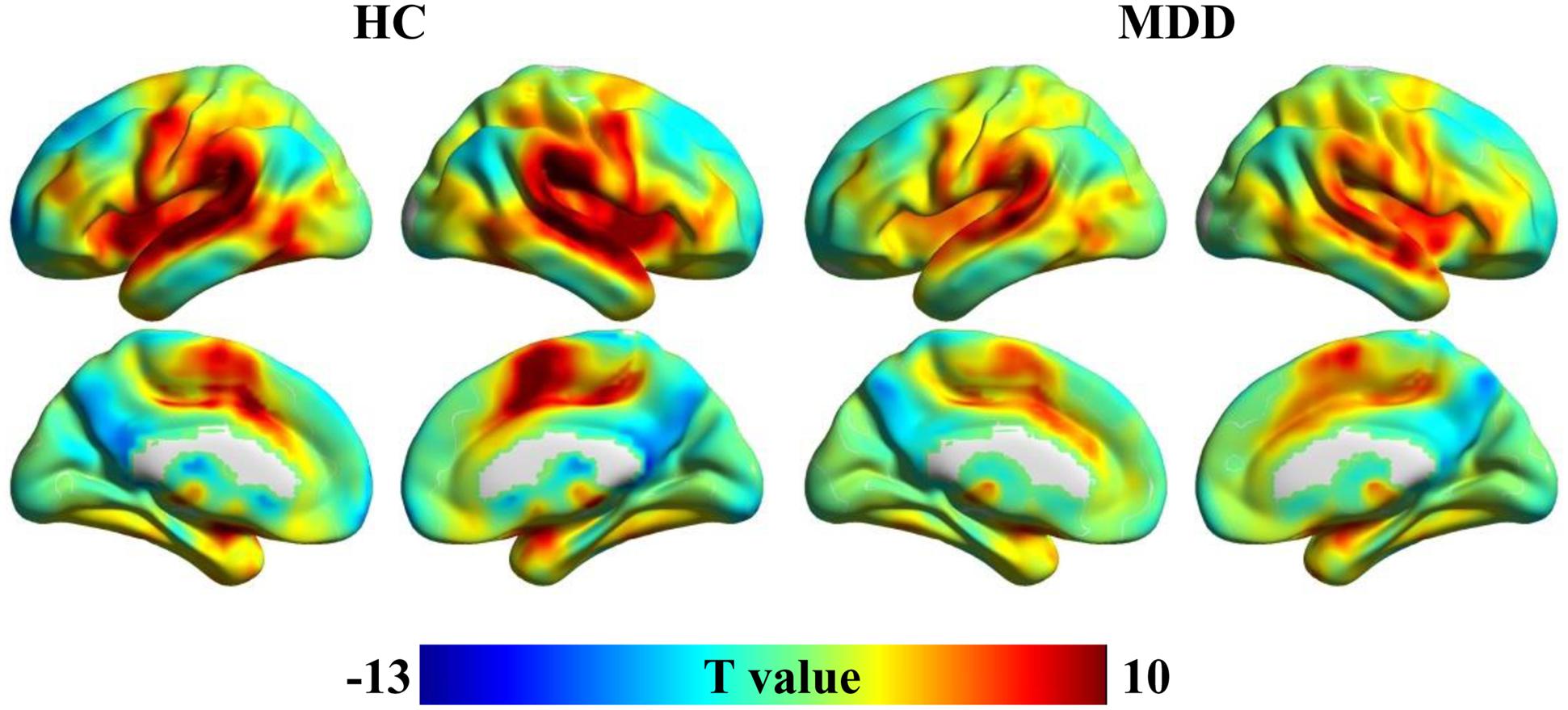
FIGURE 1. One-sample t-tests were used to identify the distribution of weighted global brain connectivity (wGCB) in major depressive disorder (MDD) and healthy controls (HC). The high wGCB were primarily detected in the superior temporal gyrus, lateral occipital gyrus, fusiform gyrus, intraparietal sulcus, medial temporal lobe, middle cingulate cortex, caudate, medial frontal cortex, lateral prefrontal cortex, inferior frontal cortex, and insula.
Changed wGCB in MDD
A two-sample t-test (the significant level was set at p < 0.05, cluster-level correction with voxel-level p < 0.001) was applied to compare the wGBC and found significantly decreased wGCB in left temporal pole (TP) (peak MNI coordinate: [−48 18 −3], 91 voxels) and significantly increased wGBC in right parahippocampus (PHC) (peak MNI coordinate: [21 −12 −21], 55 voxels) in MDD patients (Figure 2).
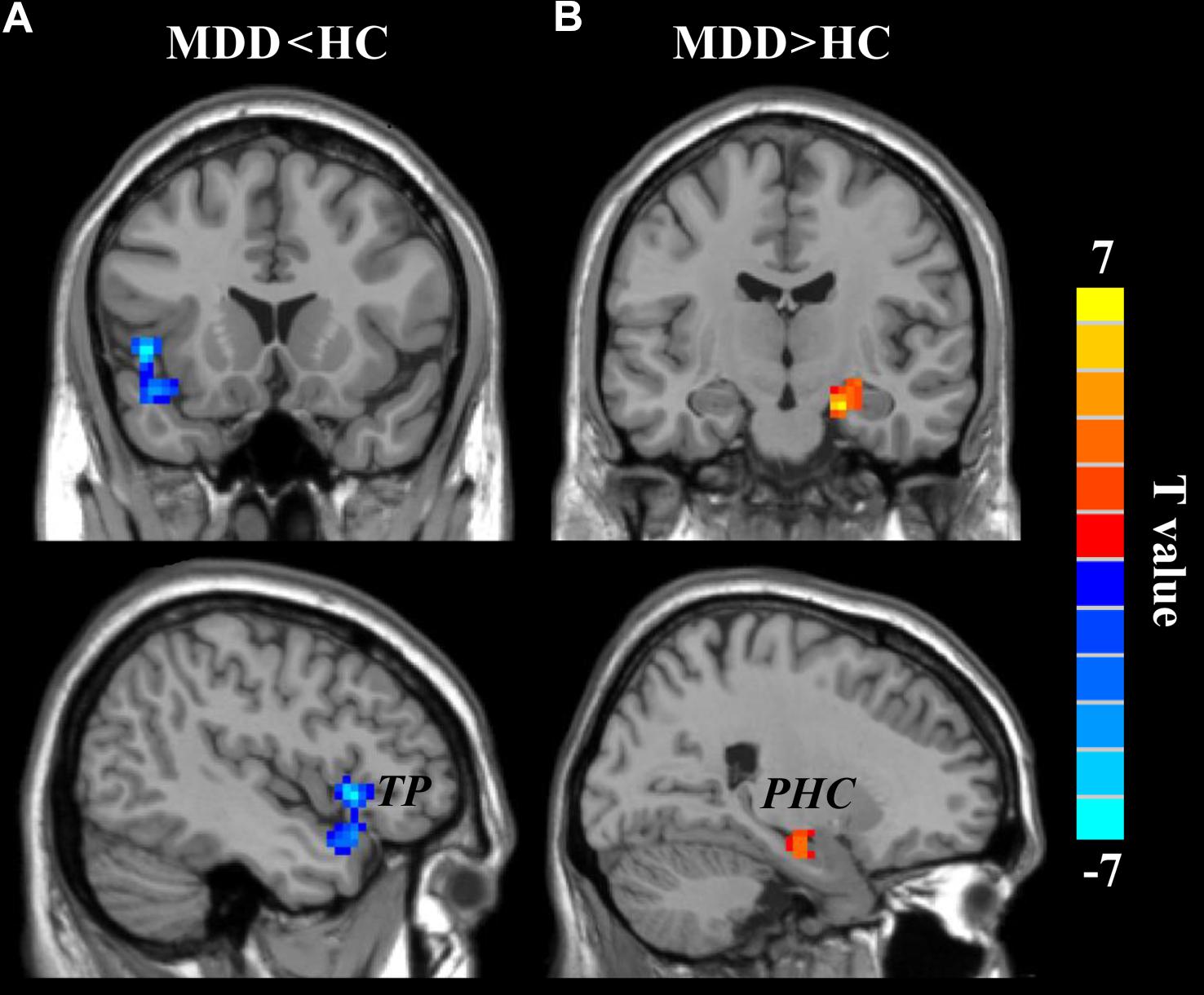
FIGURE 2. The changed weighted global brain connectivity (wGCB) in major depressive disorder (MDD) patients. Two-sample t-test was used to compare the wGCB maps between healthy controls (HC) and MDD patients and identified (A) decreased wGCB in left temporal pole (TP) and (B) increased wGCB in right parahippocampus (PHC). The significance was determined using a cluster-level Monte Carlo simulation (5000 times) corrected threshold of p < 0.05 (cluster-forming threshold at voxel-level p < 0.001).
Altered Functional Connectivities
A two-sample t-test (the significant level was set at p < 0.05, cluster-level correction with voxel-level p < 0.001) was applied to compare the whole brain RSFC maps and identified significantly decreased functional connection between left TP and right posterior superior temporal gyrus (STG: peak MNI coordinate: [63 −15 3], 139 voxels) and significantly increased functional connection between right PHC and right inferior frontal gyrus (IFG: peak MNI coordinate: [42 3 24], 100 voxels) in MDD compared to HC (Figure 3).
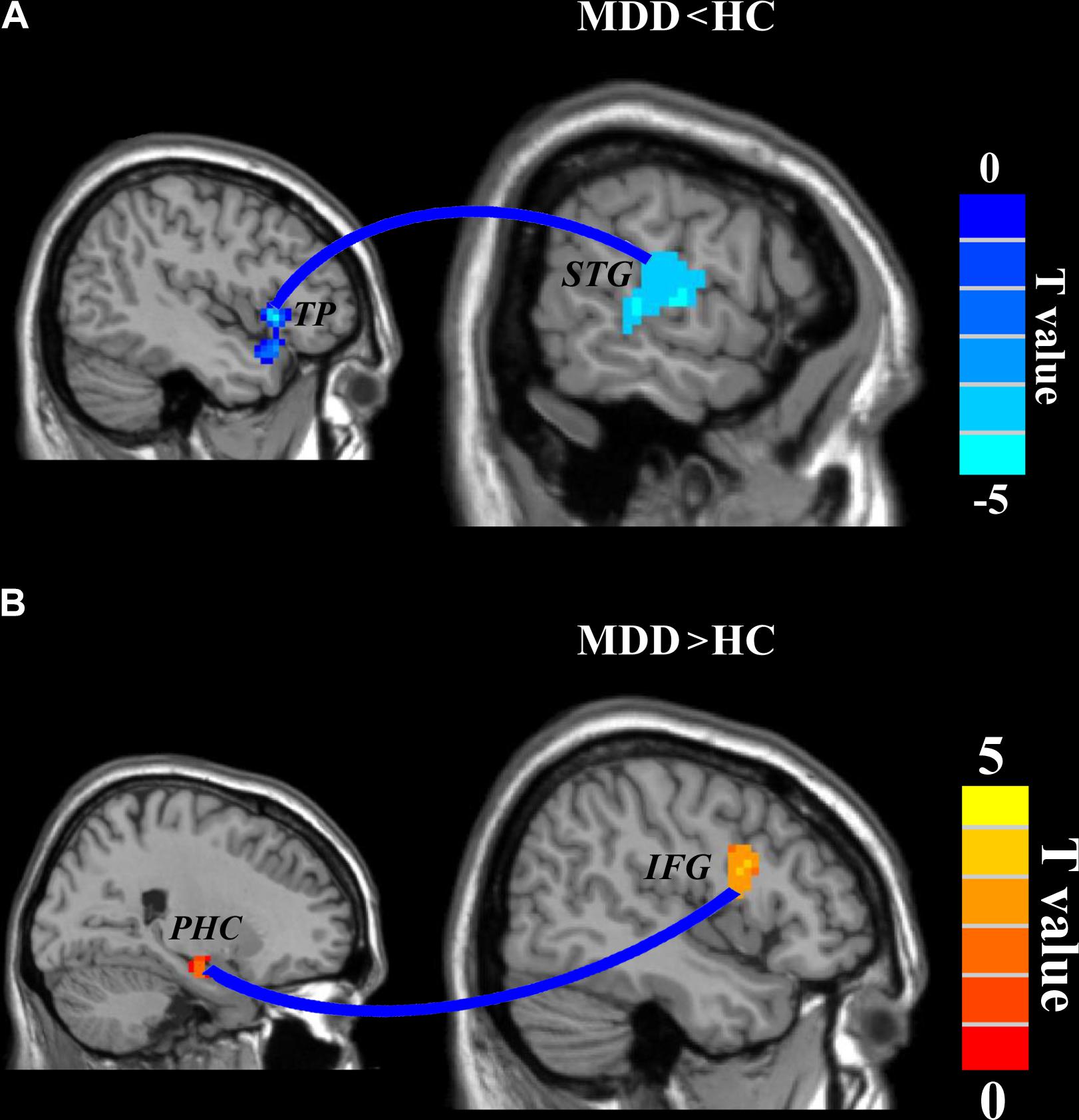
FIGURE 3. Disrupted functional connectivities in major depressive disorder (MDD) patients. Two-sample t-tests were used to identify the significant differences in functional connectivity between MDD and healthy control groups. (A) Significantly decreased functional connectivity between left temporal pole and right superior temporal gyrus and (B) significantly increased functional connectivity between right parahippocampus and right inferior frontal gyrus were found. The significance was determined using a cluster-level Monte Carlo simulation (5000 times) corrected threshold of p < 0.05 (cluster-forming threshold at voxel-level p < 0.001).
Discussion
In this study, we studied the changed global functional connectivity in MDD using wGCB method. wGCB analysis revealed decreased global functional connectivities in left TP and increased global functional connectivities in right PHC in MDD. The following functional connectivity analyses found decreased functional connectivity between left TP and right STG and increased functional connectivity between right PHC and right IFG in MDD. These findings suggested that abnormal emotion regulation and memory circuits play an important role in neuropathology of MDD.
Temporal pole and STG have been widely reported to be implicated in emotional processing and social cognition (Olson et al., 2007; Olson et al., 2013). TP is traditionally considered to participate in multimodal sensory integration (Skipper et al., 2011; Visser et al., 2012), but more and more studies have demonstrated that TP is also implicated in various high order cognitive functions, including face recognition (Olson et al., 2007), memory (Munoz-Lopez et al., 2010), and language processing (Hickok and Poeppel, 2007). The lateral TP which mainly connected with amygdala and orbital frontal cortex plays an important role in emotion regulation and theory of mind and is taken as a structure of emotional and social brain (Frith and Frith, 2010). The STG has also been reported to take part in emotional processing and social perception, especially the representation of emotional information during the initial stages of emotional regulation (Allison et al., 2000; Olsson and Ochsner, 2008). Structural and functional abnormalities of TP and STG in MDD were observed. Increased cortical thickness and decreased gray matter density of TP were identified in MDD (Fallucca et al., 2011; Peng et al., 2011; Igata et al., 2017). Abnormal functional activation of TP in MDD during sad emotion processing is also found (Beauregard et al., 2006; Keedwell et al., 2009). In STG, decreased gray matter volume and abnormal activity during sad response in MDD are also found (Fitzgerald et al., 2008; Takahashi et al., 2010). These studies indicated that TP and STG are two important nodes of affective network in emotion regulation. The decreased functional connectivity between TP and STG found in our study suggested that disconnectivity results in dysfunction of initial regulation of negative emotion in MDD patients.
The PHC which is an interface area between the hippocampus and the neocortex mainly takes part in memory function (Squire et al., 2004). PHC is also involved in recognition of emotional faces or scenes (Fitzgerald et al., 2008; Nummenmaa et al., 2008; Sabatinelli et al., 2011). The IFG plays a role in mood regulation (Baker et al., 1997; Northoff et al., 2000), associative emotional memory (Bookheimer, 2002; Price, 2003), and integrating emotional information and regulating the intensity of emotional responses (Cabeza and Nyberg, 2000; Fuster, 2001). PHC has been widely reported with decreased gray matter volume (Bora et al., 2012; Zhou et al., 2016), abnormal involvement during emotion and memory processing (Surguladze et al., 2005; Garrett et al., 2011; Palmer et al., 2014; Zamoscik et al., 2014), and damaged functional connectivity (Zeng et al., 2012). In our study, we found increased wGCB of PHC which is contrast to the gray matter volume changes. The inconsistency mainly results from gray matter volume and wGCB characterizing different properties of PHC. Structural and functional measurements of PHC may provide complementary evidence to better elucidate the role of PHC in MDD. During emotion processing in bipolar disorder during mania, hypoactivation of the IFG was observed during processing of negative faces (Altshuler et al., 2005), fear perception (Killgore et al., 2008), and negatively captioned pictures (Malhi et al., 2004). Functional disconnections of IFG have also been found in many previous studies (Murray et al., 2011; Tao et al., 2013). Moreover, reduced right IFG gray matter volume was found in MDD patients (Sabatinelli et al., 2011). All these studies suggested important roles of PHC and IFG in the pathology of MDD. In our study, we found increased functional connectivity between IFG and PHC in MDD patients compared to healthy controls. The hyperconnectivity between IFG and PHC indicated MDD patients need more efforts to inhibit negative emotion.
There are some limitations in this study. First, correlation analyses did not find significant associations between changed neuroimaging indices and HRSD scores. Thus, the conclusion needs to be further validated. Second, our samples are very small and these findings also need to be validated in a larger sample.
In conclusion, we used wGCB and functional connectivity analyses revealed abnormal global connectivity patterns in TP and PHC, and abnormal functional interactions between TP and STG, and between PHC and IFG. All these brain areas are parts of affective network and emotion regulation network. Our findings suggested that abnormal functional connectivity patterns of the two networks contribute to the pathology of MDD. The current findings will provide an important reference for future MDD therapy, including deep brain stimulation and transcranial magnetic stimulation.
Author Contributions
JX and JS designed this study and revised the manuscript. HW collected the data. LZ analyzed the data and wrote the manuscript. All the authors discussed the results.
Funding
This study was supported by the project “Studying Game-based Learning from the Perspective of Learning Sciences”, which belongs to the 2017 General Projects of Educational Research for the 13th Five-year Plan and was financed by China National Social Science Foundation No. BCA170072. This study was also supported by Basic Research Project of Peking University.
Conflict of Interest Statement
The authors declare that the research was conducted in the absence of any commercial or financial relationships that could be construed as a potential conflict of interest.
The handling Editor declared a past co-authorship with several of the authors HW and JX.
Footnotes
References
Allison, T., Puce, A., and McCarthy, G. (2000). Social perception from visual cues: role of the STS region. Trends Cogn. Sci. 4, 267–278. doi: 10.1016/S1364-6613(00)01501-1
Altshuler, L., Bookheimer, S., Proenza, M. A., Townsend, J., Sabb, F., Firestine, A., et al. (2005). Increased amygdala activation during mania: a functional magnetic resonance imaging study. Am. J. Psychiatry 162, 1211–1213. doi: 10.1176/appi.ajp.162.6.1211
Baker, S. C., Frith, C. D., and Dolan, R. J. (1997). The interaction between mood and cognitive function studied with PET. Psychol. Med. 27, 565–578. doi: 10.1017/S0033291797004856
Beauregard, M., Paquette, V., and Levesque, J. (2006). Dysfunction in the neural circuitry of emotional self-regulation in major depressive disorder. Neuroreport 17, 843–846. doi: 10.1097/01.wnr.0000220132.32091.9f
Biswal, B., Yetkin, F. Z., Haughton, V. M., and Hyde, J. S. (1995). Functional connectivity in the motor cortex of resting human brain using echo-planar MRI. Magn. Reson. Med. 34, 537–541. doi: 10.1002/mrm.1910340409
Bookheimer, S. (2002). Functional MRI of language: new approaches to understanding the cortical organization of semantic processing. Annu. Rev. Neurosci. 25, 151–188. doi: 10.1146/annurev.neuro.25.112701.142946
Bora, E., Fornito, A., Pantelis, C., and Yucel, M. (2012). Gray matter abnormalities in major depressive disorder: a meta-analysis of voxel based morphometry studies. J. Affect. Disord. 138, 9–18. doi: 10.1016/j.jad.2011.03.049
Buckner, R. L., Sepulcre, J., Talukdar, T., Krienen, F. M., Liu, H., Hedden, T., et al. (2009). Cortical hubs revealed by intrinsic functional connectivity: mapping, assessment of stability, and relation to Alzheimer’s disease. J. Neurosci. 29, 1860–1873. doi: 10.1523/JNEUROSCI.5062-08.2009
Cabeza, R., and Nyberg, L. (2000). Imaging cognition II: an empirical review of 275 PET and fMRI studies. J. Cogn. Neurosci. 12, 1–47. doi: 10.1162/08989290051137585
Chen, T., Kendrick, K. M., Wang, J., Wu, M., Li, K., Huang, X., et al. (2017). Anomalous single-subject based morphological cortical networks in drug-naive, first-episode major depressive disorder. Hum. Brain Mapp. 38, 2482–2494. doi: 10.1002/hbm.23534
Chen, V. C., Shen, C. Y., Liang, S. H., Li, Z. H., Tyan, Y. S., Liao, Y. T., et al. (2016). Assessment of abnormal brain structures and networks in major depressive disorder using morphometric and connectome analyses. J. Affect. Disord. 205, 103–111. doi: 10.1016/j.jad.2016.06.066
Cole, M. W., Anticevic, A., Repovs, G., and Barch, D. (2011). Variable global dysconnectivity and individual differences in schizophrenia. Biol. Psychiatry 70, 43–50. doi: 10.1016/j.biopsych.2011.02.010
Cole, M. W., Bassett, D. S., Power, J. D., Braver, T. S., and Petersen, S. E. (2014). Intrinsic and task-evoked network architectures of the human brain. Neuron 83, 238–251. doi: 10.1016/j.neuron.2014.05.014
Cole, M. W., Pathak, S., and Schneider, W. (2010). Identifying the brain’s most globally connected regions. Neuroimage 49, 3132–3148. doi: 10.1016/j.neuroimage.2009.11.001
Fallucca, E., MacMaster, F. P., Haddad, J., Easter, P., Dick, R., May, G., et al. (2011). Distinguishing between major depressive disorder and obsessive-compulsive disorder in children by measuring regional cortical thickness. Arch. Gen. Psychiatry 68, 527–533. doi: 10.1001/archgenpsychiatry.2011.36
Fan, L., Li, H., Zhuo, J., Zhang, Y., Wang, J., Chen, L., et al. (2016). The human brainnetome atlas: a new brain atlas based on connectional architecture. Cereb. Cortex 26, 3508–3526. doi: 10.1093/cercor/bhw157
Fan, L., Wang, J., Zhang, Y., Han, W., Yu, C., and Jiang, T. (2014). Connectivity-based parcellation of the human temporal pole using diffusion tensor imaging. Cereb. Cortex 24, 3365–3378. doi: 10.1093/cercor/bht196
Fitzgerald, P. B., Laird, A. R., Maller, J., and Daskalakis, Z. J. (2008). A meta-analytic study of changes in brain activation in depression. Hum. Brain Mapp. 29, 683–695. doi: 10.1002/hbm.20426
Fox, M. D., Corbetta, M., Snyder, A. Z., Vincent, J. L., and Raichle, M. E. (2006). Spontaneous neuronal activity distinguishes human dorsal and ventral attention systems. Proc. Natl. Acad. Sci. U.S.A. 103, 10046–10051. doi: 10.1073/pnas.0604187103
Frith, U., and Frith, C. (2010). The social brain: allowing humans to boldly go where no other species has been. Philos. Trans. R. Soc. London B Biol. Sci. 365, 165–176. doi: 10.1098/rstb.2009.0160
Fuster, J. M. (2001). The prefrontal cortex—an update: time is of the essence. Neuron 30, 319–333. doi: 10.1016/S0896-6273(01)00285-9
Garrett, A., Kelly, R., Gomez, R., Keller, J., Schatzberg, A. F., and Reiss, A. L. (2011). Aberrant brain activation during a working memory task in psychotic major depression. Am. J. Psychiatry 168, 173–182. doi: 10.1176/appi.ajp.2010.09121718
Gong, Q., and He, Y. (2015). Depression, neuroimaging and connectomics: a selective overview. Biol. Psychiatry 77, 223–235. doi: 10.1016/j.biopsych.2014.08.009
Hickok, G., and Poeppel, D. (2007). The cortical organization of speech processing. Nat. Rev. Neurosci. 8, 393–402. doi: 10.1038/nrn2113
Igata, R., Katsuki, A., Kakeda, S., Watanabe, K., Igata, N., Hori, H., et al. (2017). PCLO rs2522833-mediated gray matter volume reduction in patients with drug-naive, first-episode major depressive disorder. Transl. Psychiatry 7, e1140. doi: 10.1038/tp.2017.100
Keedwell, P., Drapier, D., Surguladze, S., Giampietro, V., Brammer, M., and Phillips, M. (2009). Neural markers of symptomatic improvement during antidepressant therapy in severe depression: subgenual cingulate and visual cortical responses to sad, but not happy, facial stimuli are correlated with changes in symptom score. J. Psychopharmacol. 23, 775–788. doi: 10.1177/0269881108093589
Killgore, W. D., Gruber, S. A., and Yurgelun-Todd, D. A. (2008). Abnormal corticostriatal activity during fear perception in bipolar disorder. Neuroreport 19, 1523–1527. doi: 10.1097/WNR.0b013e328310af58
Liu, C., Wang, J., Hou, Y., Qi, Z., Wang, L., Zhan, S., et al. (2018). Mapping the changed hubs and corresponding functional connectivity in idiopathic restless legs syndrome. Sleep Med. 45, 132–139. doi: 10.1016/j.sleep.2017.12.016
Malhi, G. S., Lagopoulos, J., Ward, P. B., Kumari, V., Mitchell, P. B., Parker, G. B., et al. (2004). Cognitive generation of affect in bipolar depression: an fMRI study. Eur. J. Neurosci. 19, 741–754. doi: 10.1111/j.0953-816X.2003.03159.x
Mathers, C. D., and Loncar, D. (2006). Projections of global mortality and burden of disease from 2002 to 2030. PLoS Med. 3:e442. doi: 10.1371/journal.pmed.0030442
Mears, D., and Pollard, H. B. (2016). Network science and the human brain: using graph theory to understand the brain and one of its hubs, the amygdala, in health and disease. J. Neurosci. Res. 94, 590–605. doi: 10.1002/jnr.23705
Munoz-Lopez, M. M., Mohedano-Moriano, A., and Insausti, R. (2010). Anatomical pathways for auditory memory in primates. Front. Neuroanat. 4:129. doi: 10.3389/fnana.2010.00129
Murray, E. A., Wise, S. P., and Drevets, W. C. (2011). Localization of dysfunction in major depressive disorder: prefrontal cortex and amygdala. Biol. Psychiatry 69, e43–e54. doi: 10.1016/j.biopsych.2010.09.041
Northoff, G., Richter, A., Gessner, M., Schlagenhauf, F., Fell, J., Baumgart, F., et al. (2000). Functional dissociation between medial and lateral prefrontal cortical spatiotemporal activation in negative and positive emotions: a combined fMRI/MEG study. Cereb. Cortex 10, 93–107. doi: 10.1093/cercor/10.1.93
Nummenmaa, L., Hirvonen, J., Parkkola, R., and Hietanen, J. K. (2008). Is emotional contagion special? An fMRI study on neural systems for affective and cognitive empathy. Neuroimage 43, 571–580. doi: 10.1016/j.neuroimage.2008.08.014
Olson, I. R., McCoy, D., Klobusicky, E., and Ross, L. A. (2013). Social cognition and the anterior temporal lobes: a review and theoretical framework. Soc. Cogn. Affect. Neurosci. 8, 123–133. doi: 10.1093/scan/nss119
Olson, I. R., Plotzker, A., and Ezzyat, Y. (2007). The enigmatic temporal pole: a review of findings on social and emotional processing. Brain 130, 1718–1731. doi: 10.1093/brain/awm052
Olsson, A., and Ochsner, K. N. (2008). The role of social cognition in emotion. Trends Cogn. Sci. 12, 65–71. doi: 10.1016/j.tics.2007.11.010
Palmer, S. M., Crewther, S. G., Carey, L. M., and Team, S. P. (2014). A meta-analysis of changes in brain activity in clinical depression. Front. Hum. Neurosci. 8:1045. doi: 10.3389/fnhum.2014.01045
Passingham, R. E., Stephan, K. E., and Kotter, R. (2002). The anatomical basis of functional localization in the cortex. Nat. Rev. Neurosci. 3, 606–616. doi: 10.1038/nrn893
Peng, J., Liu, J., Nie, B., Li, Y., Shan, B., Wang, G., et al. (2011). Cerebral and cerebellar gray matter reduction in first-episode patients with major depressive disorder: a voxel-based morphometry study. Eur. J. Radiol. 80, 395–399. doi: 10.1016/j.ejrad.2010.04.006
Power, J. D., Barnes, K. A., Snyder, A. Z., Schlaggar, B. L., and Petersen, S. E. (2012). Spurious but systematic correlations in functional connectivity MRI networks arise from subject motion. Neuroimage 59, 2142–2154. doi: 10.1016/j.neuroimage.2011.10.018
Power, J. D., Cohen, A. L., Nelson, S. M., Wig, G. S., Barnes, K. A., Church, J. A., et al. (2011). Functional network organization of the human brain. Neuron 72, 665–678. doi: 10.1016/j.neuron.2011.09.006
Price, J. L. (2003). Comparative aspects of amygdala connectivity. Ann. N. Y. Acad. Sci. 985, 50–58. doi: 10.1111/j.1749-6632.2003.tb07070.x
Sabatinelli, D., Fortune, E. E., Li, Q., Siddiqui, A., Krafft, C., Oliver, W. T., et al. (2011). Emotional perception: meta-analyses of face and natural scene processing. Neuroimage 54, 2524–2533. doi: 10.1016/j.neuroimage.2010.10.011
Skipper, L. M., Ross, L. A., and Olson, I. R. (2011). Sensory and semantic category subdivisions within the anterior temporal lobes. Neuropsychologia 49, 3419–3429. doi: 10.1016/j.neuropsychologia.2011.07.033
Squire, L. R., Stark, C. E., and Clark, R. E. (2004). The medial temporal lobe. Annu. Rev. Neurosci. 27, 279–306. doi: 10.1146/annurev.neuro.27.070203.144130
Sun, H., Luo, L., Yuan, X., Zhang, L., He, Y., Yao, S., et al. (2018). Regional homogeneity and functional connectivity patterns in major depressive disorder, cognitive vulnerability to depression and healthy subjects. J. Affect. Disord. 235, 229–235. doi: 10.1016/j.jad.2018.04.061
Surguladze, S., Brammer, M. J., Keedwell, P., Giampietro, V., Young, A. W., Travis, M. J., et al. (2005). A differential pattern of neural response toward sad versus happy facial expressions in major depressive disorder. Biol. Psychiatry 57, 201–209. doi: 10.1016/j.biopsych.2004.10.028
Takahashi, T., Yucel, M., Lorenzetti, V., Walterfang, M., Kawasaki, Y., Whittle, S., et al. (2010). An MRI study of the superior temporal subregions in patients with current and past major depression. Prog. Neuropsychopharmacol. Biol. Psychiatry 34, 98–103. doi: 10.1016/j.pnpbp.2009.10.005
Tao, H., Guo, S., Ge, T., Kendrick, K. M., Xue, Z., Liu, Z., et al. (2013). Depression uncouples brain hate circuit. Mol. Psychiatry 18, 101–111. doi: 10.1038/mp.2011.127
Visser, M., Jefferies, E., Embleton, K. V., and Lambon Ralph, M. A. (2012). Both the middle temporal gyrus and the ventral anterior temporal area are crucial for multimodal semantic processing: distortion-corrected fMRI evidence for a double gradient of information convergence in the temporal lobes. J. Cogn. Neurosci. 24, 1766–1778. doi: 10.1162/jocn_a_00244
Wang, C., Wu, H., Chen, F., Xu, J., Li, H., Li, H., et al. (2017a). Disrupted functional connectivity patterns of the insula subregions in drug-free major depressive disorder. J. Affect. Disord. 234, 297–304. doi: 10.1016/j.jad.2017.12.033
Wang, J., Wei, Q., Bai, T., Zhou, X., Sun, H., Becker, B., et al. (2017b). Electroconvulsive therapy selectively enhanced feedforward connectivity from fusiform face area to amygdala in major depressive disorder. Soc. Cogn. Affect. Neurosci. 12, 1983–1992. doi: 10.1093/scan/nsx100
Wang, J., Wei, Q., Yuan, X., Jiang, X., Xu, J., Zhou, X., et al. (2017c). Local functional connectivity density is closely associated with the response of electroconvulsive therapy in major depressive disorder. J. Affect. Disord. 225, 658–664. doi: 10.1016/j.jad.2017.09.001
Wang, J., Xie, S., Guo, X., Becker, B., Fox, P. T., Eickhoff, S. B., et al. (2017d). Correspondent functional topography of the human left inferior parietal lobule at rest and under task revealed using resting-state fMRI and coactivation based parcellation. Hum. Brain Mapp. 38, 1659–1675. doi: 10.1002/hbm.23488
Wang, J., Fan, L., Wang, Y., Xu, W., Jiang, T., Fox, P. T., et al. (2015a). Determination of the posterior boundary of Wernicke’s area based on multimodal connectivity profiles. Hum. Brain Mapp. 36, 1908–1924. doi: 10.1002/hbm.22745
Wang, J., Yang, Y., Fan, L., Xu, J., Li, C., Liu, Y., et al. (2015b). Convergent functional architecture of the superior parietal lobule unraveled with multimodal neuroimaging approaches. Hum. Brain Mapp. 36, 238–257. doi: 10.1002/hbm.22626
Wang, J., Fan, L., Zhang, Y., Liu, Y., Jiang, D., Zhang, Y., et al. (2012). Tractography-based parcellation of the human left inferior parietal lobule. Neuroimage 63, 641–652. doi: 10.1016/j.neuroimage.2012.07.045
Wang, J., Tian, Y., Wang, M., Cao, L., Wu, H., Zhang, Y., et al. (2016a). A lateralized top-down network for visuospatial attention and neglect. Brain Imaging Behav. 10, 1029–1037.
Wang, J., Zhang, J., Rong, M., Wei, X., Zheng, D., Fox, P. T., et al. (2016b). Functional topography of the right inferior parietal lobule structured by anatomical connectivity profiles. Hum. Brain Mapp. 37, 4316–4332. doi: 10.1002/hbm.23311
Wang, J., Wei, Q., Wang, L., Zhang, H., Bai, T., Cheng, L., et al. (2018). Functional reorganization of intra- and internetwork connectivity in major depressive disorder after electroconvulsive therapy. Hum. Brain Mapp. 39, 1403–1411. doi: 10.1002/hbm.23928
Wang, L., Xu, J., Wang, C., and Wang, J. (2018). Whole brain functional connectivity pattern homogeneity mapping. Front. Hum. Neurosci. 12:164. doi: 10.3389/fnhum.2018.00164
Wu, H., Sun, H., Wang, C., Yu, L., Li, Y., Peng, H., et al. (2017). Abnormalities in the structural covariance of emotion regulation networks in major depressive disorder. J. Psychiatr. Res. 84, 237–242. doi: 10.1016/j.jpsychires.2016.10.001
Wu, H., Sun, H., Xu, J., Wu, Y., Wang, C., Xiao, J., et al. (2016a). Changed hub and corresponding functional connectivity of subgenual anterior cingulate cortex in major depressive disorder. Front. Neuroanat. 10:120. doi: 10.3389/fnana.2016.00120
Wu, Y., Wang, J., Zhang, Y., Zheng, D., Zhang, J., Rong, M., et al. (2016b). The neuroanatomical basis for posterior superior parietal lobule control lateralization of visuospatial attention. Front. Neuroanat. 10:32. doi: 10.3389/fnana.2016.00032
Wu, Y., Zhang, Y., Liu, Y., Liu, J., Duan, Y., Wei, X., et al. (2016c). Distinct changes in functional connectivity in posteromedial cortex subregions during the progress of Alzheimer’s disease. Front. Neuroanat. 10:41. doi: 10.3389/fnana.2016.00041
Xu, J., Wang, J., Fan, L., Li, H., Zhang, W., Hu, Q., et al. (2015). Tractography-based parcellation of the human middle temporal gyrus. Sci. Rep. 5:18883. doi: 10.1038/srep18883
Yang, Y., Fan, L., Chu, C., Zhuo, J., Wang, J., Fox, P. T., et al. (2016). Identifying functional subdivisions in the human brain using meta-analytic activation modeling-based parcellation. Neuroimage 124, 300–309. doi: 10.1016/j.neuroimage.2015.08.027
Yeo, B. T., Krienen, F. M., Sepulcre, J., Sabuncu, M. R., Lashkari, D., Hollinshead, M., et al. (2011). The organization of the human cerebral cortex estimated by intrinsic functional connectivity. J. Neurophysiol. 106, 1125–1165. doi: 10.1152/jn.00338.2011
Zamoscik, V., Huffziger, S., Ebner-Priemer, U., Kuehner, C., and Kirsch, P. (2014). Increased involvement of the parahippocampal gyri in a sad mood predicts future depressive symptoms. Soc. Cogn. Affect. Neurosci. 9, 2034–2040. doi: 10.1093/scan/nsu006
Zeng, L. L., Shen, H., Liu, L., Wang, L., Li, B., Fang, P., et al. (2012). Identifying major depression using whole-brain functional connectivity: a multivariate pattern analysis. Brain 135, 1498–1507. doi: 10.1093/brain/aws059
Zhang, W., Wang, J., Fan, L., Zhang, Y., Fox, P. T., Eickhoff, S. B., et al. (2016). Functional organization of the fusiform gyrus revealed with connectivity profiles. Hum. Brain Mapp. 37, 3003–3016. doi: 10.1002/hbm.23222
Zhang, Y., Fan, L., Zhang, Y., Wang, J., Zhu, M., Zhang, Y., et al. (2014). Connectivity-based parcellation of the human posteromedial cortex. Cereb. Cortex 24, 719–727. doi: 10.1093/cercor/bhs353
Keywords: major depressive disorder, fMRI, resting-state, global brain connectivity, functional connectivity
Citation: Zhang L, Wu H, Xu J and Shang J (2018) Abnormal Global Functional Connectivity Patterns in Medication-Free Major Depressive Disorder. Front. Neurosci. 12:692. doi: 10.3389/fnins.2018.00692
Received: 15 July 2018; Accepted: 18 September 2018;
Published: 09 October 2018.
Edited by:
Jiaojian Wang, University of Pennsylvania, United StatesReviewed by:
Qinmu Peng, Huazhong University of Science and Technology, ChinaSangma Xie, Hangzhou Dianzi University, China
Copyright © 2018 Zhang, Wu, Xu and Shang. This is an open-access article distributed under the terms of the Creative Commons Attribution License (CC BY). The use, distribution or reproduction in other forums is permitted, provided the original author(s) and the copyright owner(s) are credited and that the original publication in this journal is cited, in accordance with accepted academic practice. No use, distribution or reproduction is permitted which does not comply with these terms.
*Correspondence: Jinping Xu, anAueHVAc2lhdC5hYy5jbg== Junjie Shang, ampzaGFuZ0Bwa3UuZWR1LmNu
†These authors have contributed equally to this work