A Commentary on
The Evolution of Musicality: What Can Be Learned from Language Evolution Research?
by Ravignani, A., Thompson, B., and Filippi, P. (2018). Front. Neurosci. 12:20. doi: 10.3389/fnins.2018.00020
Music evolution research requires an integrated approach combining experimental research and formal-mathematical modeling, especially computer simulation of cognitive and neural processes (Anderson, 2014). In their valuable methodological contribution, Ravignani et al. (2018) reminded us of the importance of an integrated approach. We agree that musicological research is indeed in need of an integrated methodology. However, such a methodology is not limited to a purely inductive-probabilistic approach of the empiricist, externalist tradition examining behavioral changes. Thus, we suggest to complement Ravignani's and colleagues' data-driven bottom-up approach from the perspective of cognitive musicology and comparative biomusicology by a theory-driven top-down approach investigating the biological foundations of the human music capacity as the study of a computational (neuro)cognitive system. Especially, by introducing computational neurocognitive modeling as a complementary approach which explores the evolving mind/brain within a biological framework, we highlight those aspects which Ravignani and colleagues almost completely neglect such as (1) internal mechanisms, i.e., cognitive and neural processes, and representations, (2) the evolving brain, and (3) theory-driven modeling or hypothesis building.
The most appropriate biological framework to investigate language and music evolution, as already suggested by several authors (e.g., Asano and Boeckx, 2015; Fitch, 2018), is provided by Tinbergen's four questions (Tinbergen, 1963): Mechanisms/causality: How does the steady state of the mind/brain, i.e., its functional architecture and its processing mechanisms (Shallice and Cooper, 2011), look like? Ontogeny: How does a system in interaction with its environment develop from its initial state to its steady state? Phylogeny: How did that species-specific initial state evolve? Function: Why did it evolve? Importantly, Tinbergen's term “mechanism” refers to internal causal processes or causes underlying behaviors. This indicates that music evolution research must take internal components of the functional architecture of the neurocognitive system seriously instead of observing or experimenting with external musics in terms of animal signals and behaviors (e.g., Honing, 2018). These internal mechanisms are investigated at an intermediate level of research which deals with cognitive and neural processes as computations on internal representation underlying animal signals and behaviors (Figure 1).
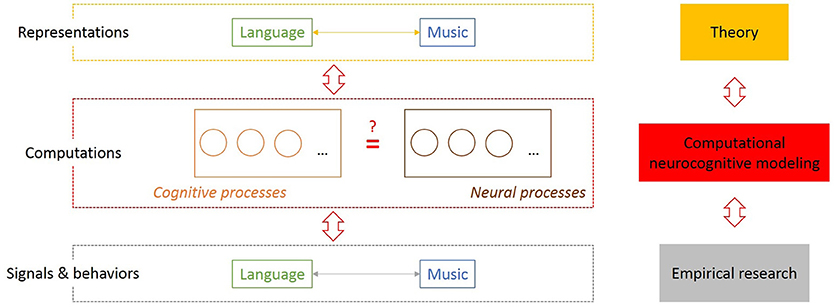
Figure 1. Computational neurocognitive modeling as a bridge between theory and empirical research as well as mind and brain in evolutionary research on the music capacity. This figure illustrates three levels of investigating neurocognitive systems and the mapping problem between mind and brain (Poeppel and Embick, 2005) or the explanatory gap (Levine, 1999) indicated with a question mark between cognitive and neural processes. The levels on the left and right side correspond to each other. Theories and hypotheses about (internal) representations based on intuition (Top) and quantified observable data on (animal) signals and behaviors (Bottom) are traditionally often opposed approaches in language evolution research and linguistics (Culicover and Jackendoff, 2010; Gibson and Fedorenko, 2010). Alongside the central hypothesis of cognitive science (Gallistel and King, 2009), a set of neurocognitive processes which execute computations are proposed as an intermediate level.
Although we admit that interaction and external factors need to be taken into account in research on language and music evolution (e.g., the dual-inheritance model by Richerson and Boyd, 2005; niche construction by Laland et al., 2013), we find it critical that cognitive and neural processes of the interacting computational agents and their relation to phenomenal experience, which Ravignani and colleagues avoid so deliberately, stay in focus of the approach of cognitive science and computational neuroscience to the mind/brain (Gallistel and King, 2009; Anderson, 2014). We use “computation” and “computational” as in automata theory and computability theory in the abstract sense of Turing-machine computability (Nelson, 1968; Cooper, 2004). Turing-machine computability provides a conceptual foundation with methodological implications for the research paradigms within cognitive science (cognitivism, connectionism, and dynamicism). In computer simulations of neurocognitive processes, this leads to different modeling strategies using logic, linear algebra, or differential equations.
In addition, evolutionary neuroscience is neglected although language evolution research already profited from and keeps pushing this approach (e.g., Deacon, 1997; Arbib, 2012 and many others). Its relation to biocultural coevolution research was also extensively discussed in neuroarchaeology (Stout and Hecht, 2017). Therefore, we suggest to put the evolving and developing mind/brain and computational neurocognitive modeling in the focus of an integrated approach (Poggio, 2012). This allows music evolution research to move toward a computational neuroethology or biology (Fitch, 2014; Arbib, 2016).
Dominey and colleagues' connectionist modeling approach (starting with Dominey and Inui, 2009) might serve as an example of potentially fruitful computational neurocognitive models for language and music evolution research. Their models carry out computations, i.e., transforming strings to meaning representations, on the basis of functional hypotheses. These hypotheses concern the neural implementation of specific cognitive processes required for thematic role assignment in the cortico-basal ganglia-thalamocortical (CBGT) circuits. The simulations replicated the findings of neuroimaging and neuropsychological studies. Their models provide rich comparative options by implementing hypotheses about the processing of neural circuits which are also involved in and necessary for musical rhythm processing (Kotz et al., 2009; Leow and Grahn, 2014) and are extensively studied in other animals (Jarvis, 2004; Fee and Goldberg, 2011; Mendoza and Merchant, 2014).
Several agent-based signaling game approaches such as iterated learning models as mentioned by Ravignani and colleagues and simulations of embodied agents such as Beuls and Steel (2013) made valuable contributions to language evolution research (but see Hauser et al., 2014). However, a computational Bayesian approach is not, as the article suggests, the only game in town to investigate evolutionary relevant learning mechanisms (Sun, 2008). In addition, there are serious theoretical problems with such an approach (e.g., Bowers and Davis, 2012; Glymour, 2015). We stress again that for an integrated approach computational modeling should focus on internal mechanisms and mechanisms for (socially) interacting brains as, for example, suggested by Arbib (2016, 2017) with a dyadic-brain modeling approach to imitative learning in computational comparative neuroprimatology.
Moreover, epistemologically and methodologically, scientific hypothesis formation cannot be equated with finding patterns in data, with prediction, and with law-like generalizations. Rather, it is a theory-driven hypothesis forming process with computational-representational theories as working hypotheses of the human music capacity that is required at the first place to restrict the “search space” to find mechanistic explanations for capacities underlying effects and patterns (see also McPherson, 2001; Bechtel, 2008). Figure 1 indicates the methodological levels between empirical research and theoretical reasoning with computational neurocognitive modeling at the center as necessary to bridge the gap between theory and empirical research in music evolution research and to deal with the explanatory gap (see Figure 1). The take-home message is: scientific hypothesis formation is more than statistical hypothesis formation, computational modeling is more than data fitting, explanation is more than prediction, and mechanistic explanations are more than finding covering-law explanations for regularities in data (Cummins, 2000). If we take off empiricist's blinders, then there is not only much more to learn for music evolution research from language evolution research, but also from (formal) epistemology and musicology.
Author Contributions
All authors listed have made a substantial, direct and intellectual contribution to the work, and approved it for publication.
Conflict of Interest Statement
The authors declare that the research was conducted in the absence of any commercial or financial relationships that could be construed as a potential conflict of interest.
Acknowledgments
We would like to thank the reviewer for helpful comments. Special thanks also to the Evolinguistics project (http://evolinguistics.net/en/) for providing RA with opportunities to discuss some of the materials elaborated in the current paper.
References
Anderson, B. (2014). Computational Neuroscience and Cognitive Modelling. A Student's Introduction to Methods and Procedures. Los Angeles, CA: Sage.
Arbib, M. A. (2012). How the Brain Got Language. The Mirror System Hypothesis. Oxford: Oxford University Press. doi: 10.1093/acprof:osobl/9780199896684.001.0001
Arbib, M. A. (2016). Towards a computational comparative neuroprimatology: framing the language-ready brain. Phys Life Rev. 16, 1–54. doi: 10.1016/j.plrev.2015.09.003
Arbib, M. A. (2017). Toward the language-ready brain: biological evolution and primate comparisons. Psychon. Bull. Rev. 24, 142–150. doi: 10.3758/s13423-016-1098-2
Asano, R., and Boeckx, C. (2015). Syntax in language and music: what is the right level of comparison? Front. Psychol. 6:942. doi: 10.3389/fpsyg.2015.00942
Bechtel, W. (2008). Mental Mechanisms: Philosophical Perspectives on Cognitive Neuroscience. New York, NY: Routledge.
Beuls, K., and Steel, L. (2013). Agent-based models of strategies for the emergence and evolution of grammatical agreement. Edited by Ricard V. Solé. PLoS ONE 8:e58960. doi: 10.1371/journal.pone.0058960
Bowers, J. S., and Davis, C. J. (2012). Bayesian just-so stories in psychology and neuroscience. Psychol. Bull. 138, 389–414. doi: 10.1037/a0026450
Culicover, P. W., and Jackendoff, R. (2010). Quantitative methods alone are not enough: response to Gibson and Fedorenko. Trends Cogn. Sci. 14, 234–235. doi: 10.1016/j.tics.2010.03.012
Cummins, R. (2000). ‘How does it work?' versus ‘what are the laws?': two conceptions of psychological analysis,” in Explanation and Cognition, eds F. C. Keil and R. A. Wilson (Cambridge, MA: The MIT Press), 117–144.
Deacon, T. W. (1997). The Symbolic Species. The Co-Evolution of Language and the Brain. New York, NY: Norton.
Dominey, P. F., and Inui, T. (2009). Cortico-striatal function in sentence comprehension: insights from neurophysiology and modeling. Cortex 45, 1012–1018. doi: 10.1016/j.cortex.2009.03.007
Fee, M. S., and Goldberg, J. H. (2011). A hypothesis for basal ganglia-dependent reinforcement learning in the songbird. Neuroscience 198, 152–170. doi: 10.1016/j.neuroscience.2011.09.069
Fitch, W. T. (2014). Toward a computational framework for cognitive biology: unifying approaches from cognitive neuroscience and comparative cognition. Phys. Life Rev. 11, 329–364. doi: 10.1016/j.plrev.2014.04.005
Fitch, W. T. (2018). “Four principles of biomusicology,” in The Origins of Musicality, eds H. Honing (Cambridge, MA: The MIT Press), 23–48.
Gallistel, C. R. (2009). Memory and the Computational Brain. Why Cognitive Science Will Transform Neuroscience. Chichester: Wiley-Blackwell. doi: 10.1002/9781444310498
Gibson, E., and Fedorenko, E. (2010). Weak quantitative standards in linguistics research. Trends Cogn. Sci. 14, 233–234; discussion 234–235. doi: 10.1016/j.tics.2010.03.005
Glymour, C. (2015). Thinkings Things Through: An Introduction to Philosophical Issues and Achievements. 2nd Edn. Cambridge, MA: The MIT Press.
Hauser, M. D., Yang, C., Berwick, R. C., Tattersall, I., Ryan, M. J., Watumull, J., et al. (2014). The mystery of language evolution. Front. Psychol. 5:401. doi: 10.3389/fpsyg.2014.00401
Jarvis, E. D. (2004). Learned Birdsong and the Neurobiology of Human Language. Ann. N.Y. Acad. Sci. 1016, 749–777. doi: 10.1196/annals.1298.038
Kotz, S. A., Schwartze, M., and Schmidt-Kassow, M. (2009). Non-motor basal ganglia functions: a review and proposal for a model of sensory predictability in auditory language perception. Cortex 45, 982–990. doi: 10.1016/j.cortex.2009.02.010
Laland, K. N., Odling-Smee, J., Hoppitt, W., and Uller, T. (2013). More on how and why: cause and effect in biology revisited. Biol. Philos. 28, 719–745. doi: 10.1007/s10539-012-9335-1
Leow, L. A., and Grahn, J. A. (2014). “Neural mechanisms of rhythm perception: present findings and future directions,” in Neurobiology of Interval Timing, Advances in Experimental Medicine and Biology, eds H. Merchant and V. de Lafuente (New York, NY: Springer), 325–338.
Levine, J. (1999). “Explanatory gap,” in The MIT Encyclopedia of the Cognitive Sciences, eds R. A. Wilson and F. C. Keil (Cambridge, MA: The MIT Press), 304–305.
McPherson, G. R. (2001). Teaching & learning the scientific method. Am. Biol. Teach. 63, 242–245. doi: 10.2307/4451093
Mendoza, G., and Merchant, H. (2014). Motor system evolution and the emergence of high cognitive functions. Progr. Neurobiol. 122, 73–93. doi: 10.1016/j.pneurobio.2014.09.001
Poeppel, D., and Embick, D. (2005). “Defining the relation between linguistics and neuroscience,” in Twenty-First Century Psycholinguistics: Four Cornerstones, eds A. Cutler (Hillsdale, MI: Erlbaum), 173–189.
Poggio, T. (2012). The levels of understanding framework, revised. Perception 41, 1017–1023. doi: 10.1068/p7299
Ravignani, A., Thompson, B., and Filippi, P. (2018). The evolution of musicality: what can be learned from language evolution research? Front. Neurosci. 12:20. doi: 10.3389/fnins.2018.00020
Richerson, P. J., and Boyd, R. (2005). Not by Genes Alone: How Culture Transformed Human Evolution. Chicago, IL: The University of Chicago Press.
Stout, D., and Hecht, E. E. (2017). Evolutionary neuroscience of cumulative culture. Proc. Natl. Acad. Sci. U.S.A. 114, 7861–7868. doi: 10.1073/pnas.1620738114
Sun, R. (2008). The Cambridge Handbook of Computational Psychology. Cambridge: Cambridge University Press. doi: 10.1017/CBO9780511816772
Keywords: computational neurocognitive modeling, music evolution, internal mechanisms, mechanistic explanation, integrated approach, evolving brain, theory-driven modeling, top-down approach
Citation: Asano R and Seifert U (2018) Commentary: The Evolution of Musicality: What Can Be Learned from Language Evolution Research? Front. Neurosci. 12:640. doi: 10.3389/fnins.2018.00640
Received: 29 June 2018; Accepted: 28 August 2018;
Published: 19 September 2018.
Edited by:
Daniela Sammler, Max-Planck-Institut für Kognitions- und Neurowissenschaften, GermanyReviewed by:
Bruno Gingras, Universität Wien, AustriaCopyright © 2018 Asano and Seifert. This is an open-access article distributed under the terms of the Creative Commons Attribution License (CC BY). The use, distribution or reproduction in other forums is permitted, provided the original author(s) and the copyright owner(s) are credited and that the original publication in this journal is cited, in accordance with accepted academic practice. No use, distribution or reproduction is permitted which does not comply with these terms.
*Correspondence: Rie Asano, cmllLmFzYW5vQHVuaS1rb2Vsbi5kZQ==