- 1Department of Psychology, Korea University, Seoul, South Korea
- 2Institute of Human Behavioral Medicine, Medical Research Center, Seoul National University, Seoul, South Korea
- 3Brain and Cognitive Sciences, College of Natural Sciences, Seoul National University, Seoul, South Korea
- 4Department of Psychiatry, Washington University in St. Louis, St. Louis, MO, United States
- 5Department of Radiology, Chungbuk National University Hospital, Cheongju, South Korea
- 6Department of Psychiatry, College of Medicine, Seoul National University, Seoul, South Korea
Recent advances of neuroimaging methodology and artificial intelligence have resulted in renewed interest in board games like chess and Baduk (called Go game in the West) and have provided clues as to the mechanisms behind the games. However, an interesting question that remains to be answered is whether the board game expertise as one of cognitive skills goes beyond just being good at the trained game and how it maps on networks associated with cognitive abilities that are not directly trained. To address this issue, we examined functional activity and connectivity in Baduk experts, compared to novices, while performing a visual n-back working memory (WM) task. We found that experts, compared to novices, had greater activation in superior parietal cortex during face WM, though there were no group differences in behavioral performances. Using a data-driven, whole-brain multivariate approach, we also found significant group differences in the multivariate pattern of connectivity in frontal pole and inferior parietal cortex, further showing greater connectivity between frontal and parietal regions and between frontal and temporal regions in experts. Our findings suggest that long-term trained Baduk experts have the reorganization of functional interactions between brain regions even for untrained cognitive ability.
Introduction
People have very different levels of cognitive ability, from profound impairments to superior skills. However, while our understanding of neural mechanisms of cognitive impairments has been greatly enhanced via neuroimaging studies for general and cognitive-impaired individuals, those of superior skills still remain poorly understood.
Baduk, as it is called in Korea (known as the game of Go in the West1), is an abstract strategy board game like chess; it is played on a square board with a 19 by 19 grid of lines. Its rule is simple; two players, one playing with white stones and the other playing with black ones, take turns placing a stone to capture more territory on the board than the opponent by surrounding the opponent’s stones (Figure 1A). Despite such simple rule, Baduk is considered more complex than chess owing to its enormous branching factors (i.e., the enormous number of move choices available) (Keene and Levy, 1991).
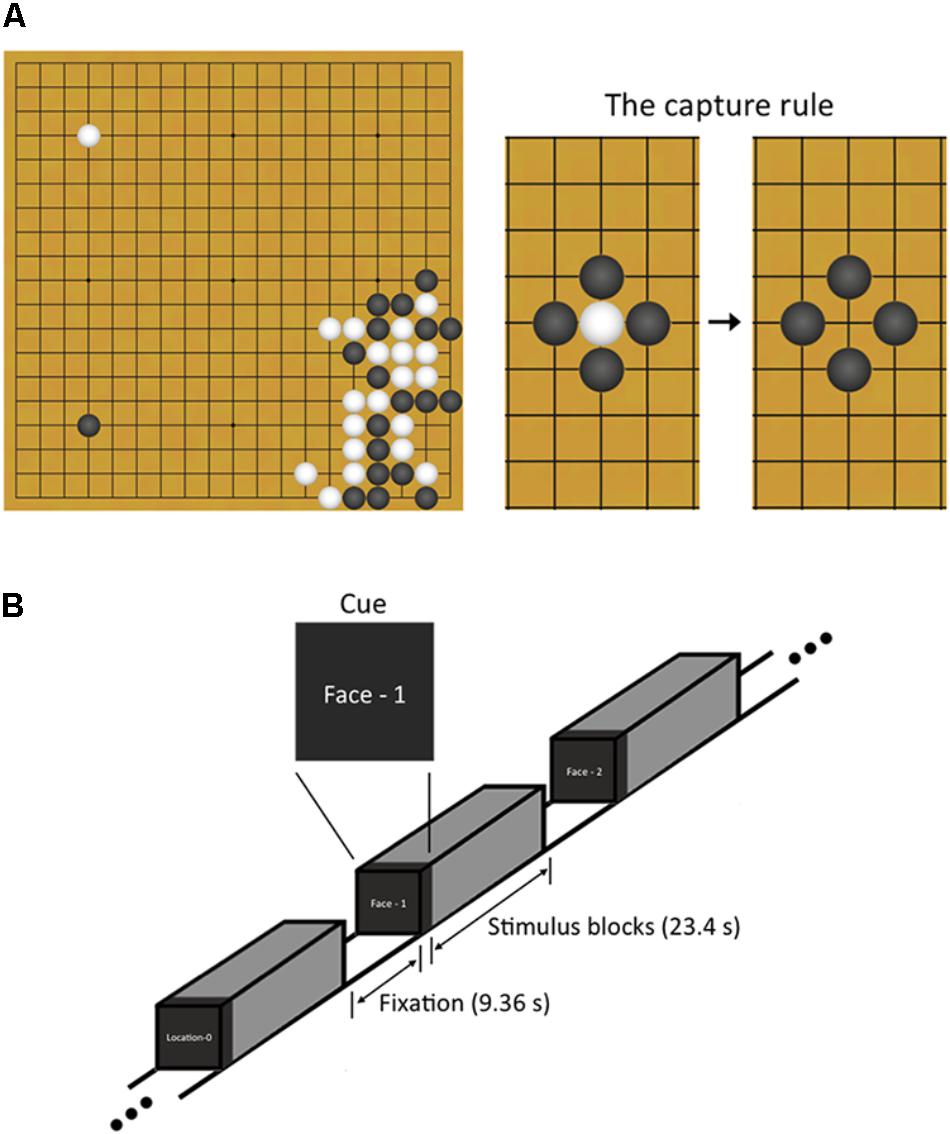
FIGURE 1. Explanation about Baduk and n-back working memory task used in this study. (A) Baduk is played using black and white stones on a square board with a 19 by 19 grid of lines. The object of Baduk is to surround and capture more empty territory on the board than the opponent but not to surround and capture the opponent’s stones. However, one strategy to achieve this object is to surround the opponent’s stones because all captured stones are removed from the board and the areas where the stones were removed from become the territory of the capturing player. (B) During brain scanning, participants performed a block-designed n-back task including both face and spatial working memory conditions. Each block included 10 trials (23.4 s) and was split up with the resting blocks (9.36 s). Visual instructions (2.34 s) preceded each block to indicate the upcoming condition.
Board games like Baduk and chess have been commonly used to investigate the mechanism underlying cognitive expertise, as playing involves diverse high-level cognitive functions such as visuospatial processing, decision making, and attention (Chase and Simon, 1973b; Gobet and Charness, 2006). For example, decades of neuroimaging studies have identified multiple brain regions engaged during board game play, including dorsolateral prefrontal cortex, premotor cortex, and occipitotemporal and parietal cortices (Chen et al., 2003; Atherton et al., 2003; Itoh et al., 2008). Additionally, researchers have explicitly investigated neural correlates of specific cognitive components closely related to board game by using a variety of domain-specific tasks employing the board game-related stimuli; these studies have revealed brain regions associated with object and pattern recognition on the board, such as the occipitotemporal junction, fusiform gyrus (FFA), and collateral sulcus (Bilalić et al., 2010, 2011), with intuitive best next-move generation on the board, such as the caudate nucleus (Wan et al., 2011, 2012), and with intuitive strategy decision making on the board, such as different parts of the cingulate cortex (Wan et al., 2015). Some of these regions, particularly in the occipitotemporal junction, FFA, and caudate nucleus, also showed significant group differences in structural morphology as well as structural and functional connectivity (FC) at rest between board game experts (BEs) and novices (Lee et al., 2010; Duan et al., 2012a,b, 2014; Jung et al., 2013; Hänggi et al., 2014). Interestingly, a recent meta-analysis on functional neuroimaging studies of long-term expertise has suggested the common mechanisms across different cognitive domains, showing enhanced or additional activity in the brain of experts compared to novices (Neumann et al., 2016). Especially, the meta-analysis on the studies with visual stimulation showed that experts had enhanced activation in inferior parietal cortex and lingual gyrus.
Despite the efforts mentioned above, an interesting question that remains to be answered is whether the board game expertise goes beyond just being good at the trained game. In other words, do behavioral and neural differences between BEs and novices exist in untrained cognitive abilities? Although there are some studies investigating the effects of chess instructions on untrained tasks (Sala and Gobet, 2016), it is still unclear whether board game expertise transfers to other cognitive skills. Moreover, most of functional neuroimaging studies comparing BEs and novices so far have focused on the regional areas associated with visual expertise using both trained and untrained stimuli (e.g., chess-related objects and positions, faces, rooms, and tools), such as FFA and occipitotemporal and occipitoparietal areas (Bilalić et al., 2010, 2011; Bilaliæ, 2016), rather than functional networks associated with other specific cognitive abilities.
Working memory (WM) is involved in the frontoparietal network (Owen et al., 2005). Particularly, object and spatial WM may be one of cognitive abilities that can be potentially enhanced through board game training based on its important role in the game; in the case of Baduk, to win the game, players are needed to remember the positions of stones on the board and to hold several future offensive moves and the opponent’s expected responses to each of the future moves in their WM. There have been existed several studies to test brain activity during 1-back WM task in chess experts compared to novices using chessboards and faces or scenes stimuli (Bilalić et al., 2011; Krawczyk et al., 2011; Bartlett et al., 2013). These studies reported consistent behavioral results, showing no group differences in behavioral performances except board-specific stimuli, but inconsistent neural results, showing an increase in BEs (Bilalić et al., 2011) or no group difference in FFA activation in response to chessboards (Krawczyk et al., 2011). These previous studies using 1-back task have investigated the neural activity in response to the recognition of trained and untrained stimuli rather than WM in BEs. Additionally, there is no a study to test functional coupling between brain regions during WM in BEs.
Here we examined the functional activity and connectivity of Baduk experts, compared to novices, while performing a visual n-back WM task with both object and spatial WM conditions. Especially, we chose to use neutral face stimuli for the task because like the board games, face discrimination and recognition rely on at least partly on holistic processing and reliably activate the FFA (Kanwisher et al., 1997), where the region is a general visual expertise module rather than face-specific (Gauthier et al., 1999, 2000). That is, using face stimuli allows us to test whether there are group differences in FFA function (Bilalić et al., 2011; Krawczyk et al., 2011; Bartlett et al., 2013). We also used a recently introduced FC technique, called multivariate distance-based matrix regression (MDMR) as part of a connectome-wise association study (CWAS) to identify brain regions showing group differences in the connectome, whole-brain FC patterns (Shehzad et al., 2014). It is a fully data-driven, whole-brain multivariate analytic approach, which provides a more comprehensive characterization of brain-behavior relationships than massive univariate approach. In the present study, the MDMR-based CWAS allowed us to evaluate the overall multivariate patterns of FC associated with a phenotype (BEs vs. novices as group) at each voxel while controlling confounding variables (age, sex, and in-scanner motion). Based on previous findings, we hypothesized that BEs, compared to novices, showed increased functional activity and connectivity particularly in the frontoparietal network during the n-back WM task.
Materials and Methods
Participants
Seventeen BEs participating in Baduk training from their childhood were recruited from the Korea Baduk Association2. Seventeen novices who were age, sex, and IQ-matched with those in the BEs, also recruited through online advertisements for purpose of comparison. Based on the simple screening questionnaire, all participants were not experts in any games, except Baduk for BEs. All participants were also right-handed and had no history of psychiatric or neurological disease. This dataset included resting-state fMRI, n-back WM task-based fMRI, T1-weighted anatomical MRI, and diffusion tensor imaging. Previous reports from this dataset have concerned differences in anatomical connectivity (Lee et al., 2010) and in gray matter volume (GMV) and resting-state FC (Jung et al., 2013) between BEs and novices. The procedures of this study were approved by the Institutional Review Board of Seoul National University Hospital and written informed consent was obtained from all participants, including parental consent for those younger than 18 years of age. All methods were performed in accordance with the approved guidelines and regulations.
Here we analyzed fMRI data obtained during the n-back WM task. Four participants (2 BEs and 2 novices) out of 34 were excluded from analyses due to excessive missing trials during the task. The final sample consisted of 15 BEs (mean age 17.3 years, range 16–20 years) and 15 novices (mean age 17.0 years, range 15–19 years; mean training duration 12.6 years). Our sample size corresponded to samples used in previous behavioral (Bilalić et al., 2008, 2009) and neuroimaging studies with BEs (Bilalić et al., 2011; Bilaliæ, 2016). Despite even the relatively small sample sizes, the direct comparison between experts and novices can provide power to capture the effects of interests.
Task
Participants performed a block-designed n-back WM task including both face matching and spatial location matching conditions (Figure 1B). The task had four WM loading conditions: 0-back, 1-back, 2-back, and 3-back. The 0-back served as a control condition, in which participants responded with a button press to a predetermined target stimulus. For the n-back face matching WM conditions, participants responded if the current face was identical to the previous one, two, and three faces ago, respectively. For the n-back spatial location matching WM conditions, participants responded if the current face was in the same place n faces ago regardless of the face identity. During the task, participants responded by pressing a button with their right index finger. The face stimuli consisted of 20 gray-scale pictures of neutral faces (10 Korean men and 10 Korean women), selected from stimuli used in our previous studies (Shin et al., 2008, 2015). The stimuli appeared in 35 different spatial positions on the screen during both the face identity and spatial tasks.
The task consisted of four runs with 48 blocks (12 blocks per run), resulting in six blocks for each of four loading conditions on face and spatial WM. Blocks were presented pseudo-randomly in order of increasing (or decreasing) memory load. Each block included 10 trials (23.4 s) and was split up with the resting blocks of 4 TRs (9.36 s). Visual instructions (2.34 s) preceded each block to indicate the upcoming condition. A face stimulus for each trial was presented for 1500 ms followed by 840 ms of fixation. Before scanning, the participant was given practice to learn the task rules.
Image Acquisition
All image data were acquired on a 1.5T Siemens Avanto MRI scanner. While performing the task, fMRI data were obtained via a gradient echo-planar image pulse sequence (repetition time (TR)/echo time (TE) = 2340/52 ms, flip angle (FA) = 90°, field of view (FOV) = 220 mm, voxel size = 3.44 mm × 3.44 mm × 5 mm). High-resolution anatomical images were acquired with T1-weighted 3-D MPRAGE sequence (TR/TE = 1160/4.76 ms, FA = 15°, FOV = 230, matrix size = 256 × 256). Other image parameters that are not related to the present study are not described herein.
Conventional fMRI Analysis
Image analysis was performed with SPM123. For each participant, after discarding the first three images in each run, images were then corrected for slice timing, realigned to the first volume, and co-registered with each participant’s structural image. There were no significant group differences in the head motion parameters. All images were then normalized to the MNI space using the normalization parameters estimated from the structural MRI. The normalized images were smoothed with 6 mm FWHM Gaussian kernel. First level-analyses were performed using the general linear model (GLM) with regressors for the cue, 0-back, l-back, 2-back, and 3-back conditions for each type of face and spatial WM in addition to six head motion parameters and a constant term. The regressors were modeled as boxcar functions convolved with a canonical hemodynamic response function for the length of each condition. To delineate brain regions activated during WM for each group and determine regions showing group differences, one-sample t-tests and two-sample t-tests were, respectively, performed for the contrast images of the 1-, 2-, 3-back versus 0-back conditions for each type of face and spatial WM. To further explore a group by WM load interaction for each type of face and spatial WM, we also performed a two (group) by three (WM load) analysis of variance (ANOVA) using the following contrast images: 1-back > 0-back, 2-back > 0-back, and 3-back > 0-back. The results were corrected for multiple comparisons to a significance level of p < 0.05 (height threshold of p < 0.001, uncorrected, combined with extent threshold of p < 0.05, family-wise error [FWE]-corrected).
MDMR-Based CWAS Analysis
The MDMR-based CWAS method has been described in detail elsewhere (Shehzad et al., 2014; Satterthwaite et al., 2015). For MDMR-based CWAS analysis, the preprocessed image data were down-sampled to 4-mm isotropic voxels for the purpose of computational feasibility. Next, the first two data-points (4.68 s) from every task block were excluded to account for the hemodynamic delay and next all volumes of the same task type (i.e., face or spatial n-back WM) were concatenated across load levels, except for 0-back, for each participant. We then performed the MDMR-based CWAS analysis according to the following three steps using Connector, an R package for CWAS4. First, we computed FC (Pearson correlation coefficient) between time series of a given voxel and those of every other voxel within the gray matter mask including cortical and subcortical areas. Second, we evaluated the overall multivariate pattern of FC for a given voxel by calculating a distance metric between every pair of FC calculated above for a given voxel (e.g., between two participants’ FC for a given voxel). To calculate the distance metric, we used , where γ is the Pearson correlation, resulting in a non-negative value that indicates how similar/different each pair is (0 = perfectly correlated, 2 = perfectly negatively correlated). Third, MDMR was used to test how well a phenotypic variable explains the distances between participants calculated in the second step. To examine group differences in FC between BEs and novices while controlling for confounding variables, modeled variables included group (BEs versus novices), age, sex, and head motion indexed by mean framewise displacement (Power et al., 2012). For each voxel’s FC pattern, MDMR yielded a pseudo-F statistic from a standard ANOVA model, by comparing the sum of squared distances between BEs and novices to the residual sum of squared distances (the error term), whose significance (i.e., p-value) was assessed using 5,000 iterations of a permutation test. All these steps were repeated for every gray matter voxel to produce a whole-brain p-value map. The p-value map was converted to z-value for multiple comparisons corrections. As in Satterthwaite et al. (2016), the z-map was thresholded at a voxel height of z > 2.326 and a corrected probability of p < 0.05 using 10,000 Monte Carlo simulations.
Follow-Up Seed-Based Connectivity Analysis
Although MDMR-based CWAS identifies clusters where group differences are present based on multivariate patterns of FC, it does not provide specific connections and direction of observed clusters (Shehzad et al., 2014). Accordingly, we performed post hoc seed-based FC analyses for each cluster identified by MDMR-based CWAS. Seed-based FC maps were generated by Pearson correlation between time series of each seed cluster and those of every other voxel and then Fisher r-to-z transformed. Two-sample t-tests were conducted to examine group differences in z-transformed seed-based FC maps. Statistical significance was set at a voxel-level FWE-corrected p < 0.05.
Region-of-Interest (ROI) Analysis
Once regions showing significant effects from the aforementioned analyses were detected, we further conducted partial correlation analyses (controlling for age and sex) between brain measures (i.e., neural activity measured as the percent signal change extracted by MarsBar toolbox5 or the strength of FC) in the identified regions as functional ROI and behavioral performances during the task and training duration in BEs.
Results
Demographic and Behavioral Data
Table 1 summarizes demographic information and behavioral performances during the n-back WM task in both BEs and novices. There were no significant group differences in age, sex, education, and IQ (all ps > 0.05). The two groups did not differ significantly in the accuracy and the reaction time (RT) during the face and spatial n-back WM (all ps > 0.05).
Group Differences in Neural Activity
Both BEs and novices showed similar activation patterns in the frontal and parietal regions during both face and spatial n-back WM (Figure 2A and Table 2). However, between-group comparisons revealed greater activation in the left superior parietal cortex (SPC) in BEs than novices during face WM at cluster-level family-wise error (FWE)-corrected p < 0.05 (Figure 2B and Table 2). The post hoc ROI analysis to further characterize the group difference in the region showed less SPC activation in BEs for the face 0-back control condition (t-/p-value = -1.904/0.067; Cohen’s d = -0.694), albeit only marginally significant, than novices but not for the face WM loading condition (t-/p-value = 0.292/0.773; Cohen’s d = 0.107). No significant correlations were found between neural activities in the SPC and behavioral performances (accuracy and RT) during the task and training durations in BEs (all ps > 0.05). There were no significant group differences in any other regions and other task conditions and no group by WM load interactions (all ps > 0.05).
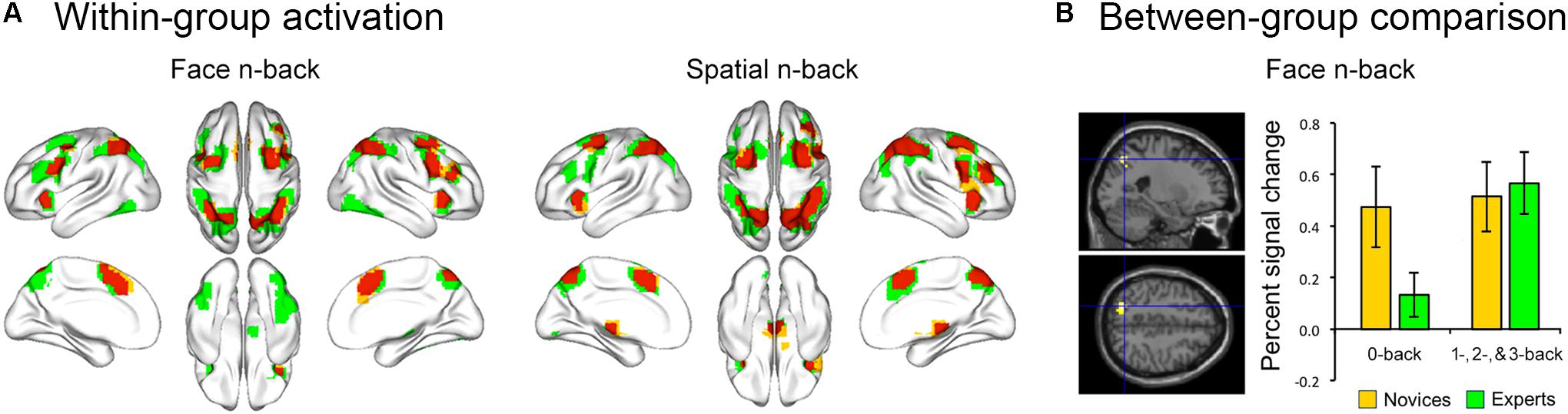
FIGURE 2. Brain activation during the n-back task. (A) The overlap (red) of activation maps for experts (green) and novices (yellow) during each WM task. The frontal and parietal areas were activated during both face and spatial n-back across working memory load (the 1-, 2-, and 3-back versus 0-back conditions). These activation maps were visualized with the BrainNet Viewer toolbox (Xia et al., 2013). (B) When comparing activation maps between experts and novices, experts showed greater activation in left superior parietal cortex (SPC) for the contrast of the face working memory load (1-, 2-, and 3-back) conditions versus the 0-back control condition. Percent signal change (PSC) was extracted for each participant and condition using MarsBar toolbox (http://marsbar.sourceforge.net/). The effect size was calculated by Cohen’s d to provide the standardized mean difference between the two groups, independent of sample size. The plot of mean PSC shows that group difference in the SPC region is caused by less activation in experts compared to novices for the 0-back condition (Cohen’s d = –0.694), whereas the PSCs of the working memory load condition for two groups are similar (Cohen’s d = 0.107).
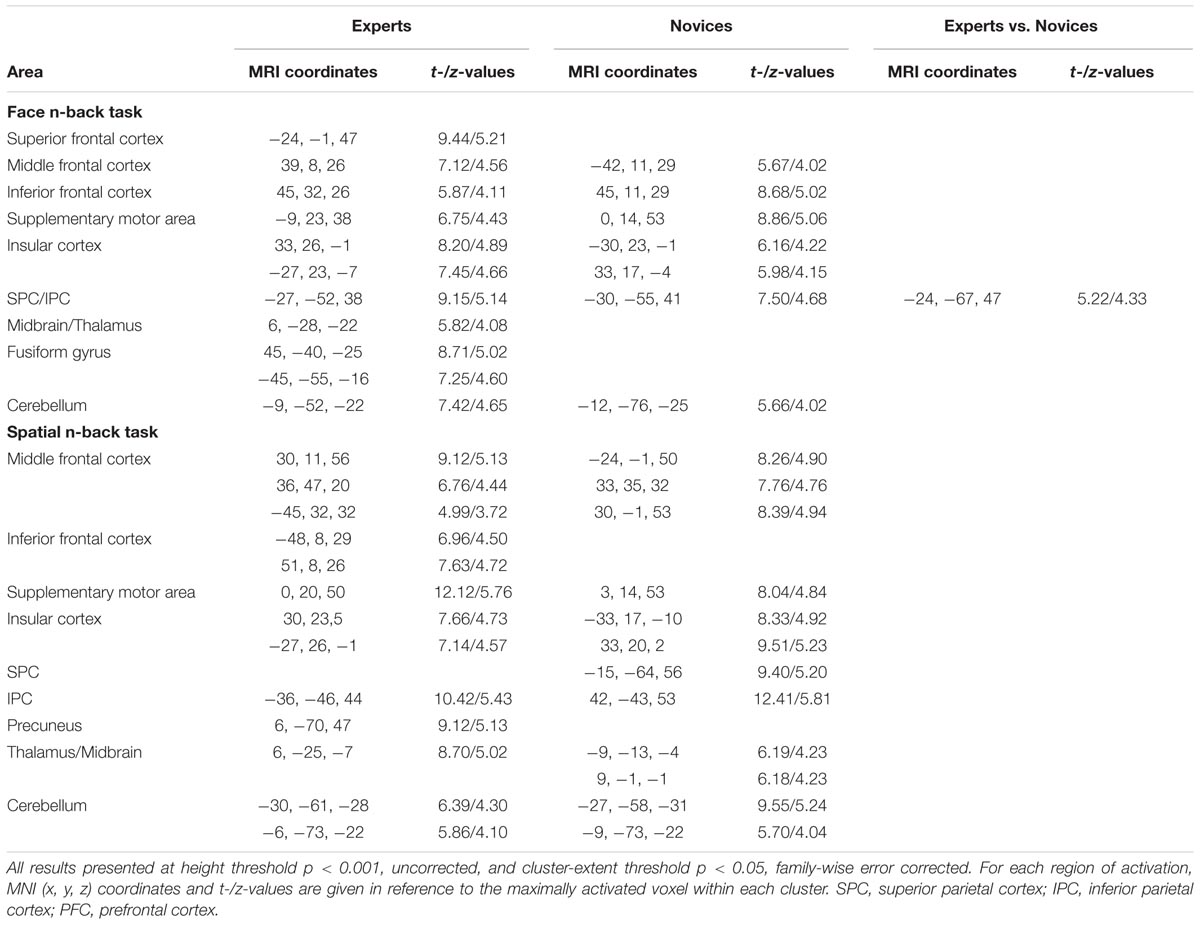
TABLE 2. Brain regions significantly activated in experts and novices during working memory tasks and the cluster with a significant difference between groups.
Brain Regions Identified by CWAS
Multivariate distance-based matrix regression-based CWAS analyses revealed two regions where the multivariate patterns of FC differ between BEs and novices at cluster-level corrected p < 0.05; one is the left frontal pole (FP; peak MNI x, y, z coordinates = -16, 60, 0) for face WM condition and the other is the left inferior parietal cortex (IPC; x, y, z = -52, -52, 44) for spatial WM condition (Figure 3A).
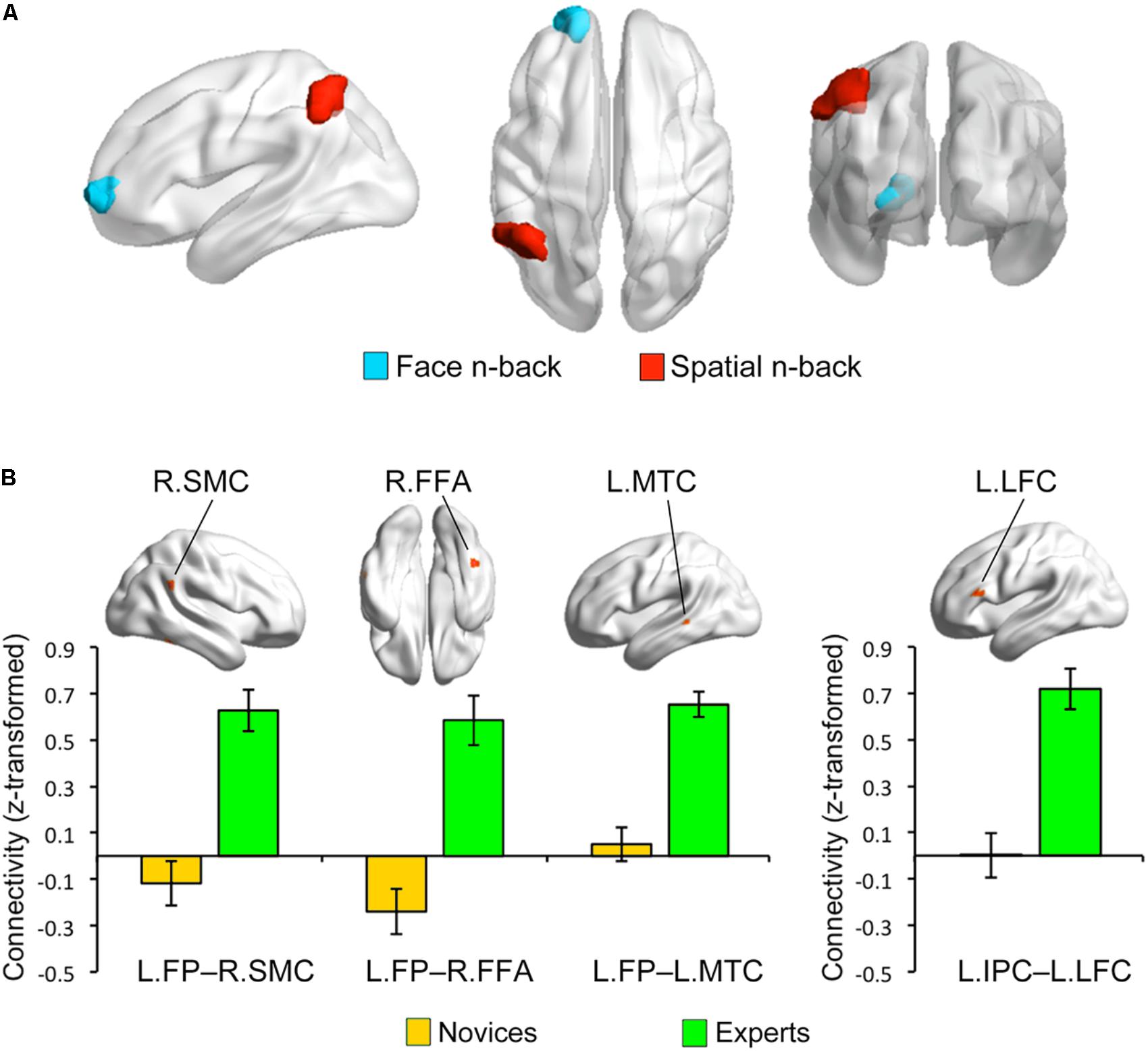
FIGURE 3. Group differences in functional connectivity. (A) Based on the multivariate patterns of functional connectivity, the left frontal pole (LFP) and inferior parietal cortex (LIPC) showed significant group differences during face and spatial n-back, respectively. (B) The seed-based connectivity analyses revealed that experts compared to novices had greater connectivity between the left frontal pole seed and right supramarginal cortex (RSMC) (Cohen’s d = 1.444), right fusiform cortex (RFFA) (Cohen’s d = 2.084), and left middle temporal cortex (LMTC) (Cohen’s d = 2.052) during the face n-back and greater connectivity between the left inferior parietal cortex seed and left lateral frontal cortex (LLFC) (Cohen’s d = 2.887) during the spatial n-back. Note that these bar graphs are presented for visualization purposes only.
Group Differences in Seed-to-Voxel Connectivity
To further characterize the FC of the regions identified by MDMR-based CWAS, we performed post hoc seed-based FC analysis to each of these identified clusters (Figure 3B). For face WM condition, BEs compared to novices had greater FC between the left FP seed and right FFA (x, y, z = 44, -48, -20; t-/z-values = 5.68/4.59), right supramarginal cortex (SMC; x, y, z = 60, -48, 28; t-/z-values = 5.68/4.60), left middle temporal cortex (MTC; x, y, z = -60, -36, 0; t-/z-values = 6.69/5.13) adjacent to superior temporal sulcus (STS). For spatial WM condition, BEs compared to novices had greater FC between the left IPC seed and left lateral frontal cortex (LFC; x, y, z = -56, 28, 16; t-/z-values = 7.10/5.33). However, the strengths of FC between these regions had no significant correlations with behavioral performances during the task and training durations in BEs.
Discussion
To address the question as to whether BEs, individuals having cognitive expertise including the highest level of domain-specific pattern recognition, differ from novices in untrained cognitive functions in terms of behavioral performance and brain function, here we explored the brain function of the Baduk (the game of Go) experts while performing n-back WM tasks. Despite no behavioral differences on task performance, BEs compared to novices showed greater SPC activation during face n-back task. Significant differences between BEs and novices were also found in the multifocal patterns of FC in the left FP and IPC for the face and spatial WM conditions, respectively, further showing greater functional couplings between frontal and parietal and temporal regions in BEs compared to novices.
The present study demonstrates that BEs with long-term training do not show an increase in WM ability but have disparate functional neural patterns. Consistent with our results, the same pattern of the absence of far transfer occurs in different types of training, including chess, music, and video game training (for a brief review, see Sala and Gobet, 2017a). For example, previous behavioral investigations have examined the correlates of expert performance (Chase and Simon, 1973a,b; Sala and Gobet, 2017b) and the effects of chess instruction on untrained tasks (Sala and Gobet, 2016). Some recent studies have reported the skill effect in the recall of meaningless domain-specific material (e.g., shuffled chess positions) (Gobet and Simon, 1996a,b; Sala and Gobet, 2017b) that contradicts the earlier claim for the lack of that skill effect (Chase and Simon, 1973b). However, the skill effect with meaningless material observed in experts is accounted for by meaningful chunks that occur in the position by chance, rather than superior cognitive function (Sala and Gobet, 2017b). A large number of the studies showing the effects of chess instruction on academic achievement (e.g., mathematics and literacy) suffered from the problem of confounding due to the overall poor design (e.g., the lack of control groups and no random assignment to groups; Sala and Gobet, 2016). It is thus suggested that engaging in intellectually demanding activities modifies the brain but the benefits are domain-specific rather than untrained cognitive abilities. Our findings from previous and present studies fit well with this pattern, showing differences in brain structure and function between BEs and novices, including structural connectivity (Lee et al., 2010), structural morphology and resting-state FC (Jung et al., 2013).
All BEs included in the present study have trained for 12.60 ± 1.55 years since their childhood. Achieving superior ability in one domain requires long periods of deliberate practice, which is known as a “10-year (or 10,000-h)-rule” (Ericsson et al., 1993). However, the deliberate practice is necessary but not sufficient to account for individual differences in experts and novices in music, sports, education, and board games (Campitelli and Gobet, 2011; Macnamara et al., 2014; Hambrick et al., 2018). Genetic predisposition and general intelligence may have more impact on ability than practice (Mosing et al., 2014, 2016; Sala et al., 2017). For example, more intelligent people tend to engage and excel in intellectually demanding activities such as chess (Burgoyne et al., 2016; Sala et al., 2017). In this regard, if general intelligence is controlled for (in our case, the IQ-matching of the two groups), differences between experts and novices in terms of untrained cognitive ability, WM, disappear. Our behavioral results that show no group differences support that idea. According to the theories that explain the domain-specificity of the effects of training, BEs use their knowledge structures of Baduk positions in long-term memory, called chunks and templates, as encoding and retrieval strategies, with less WM resources (Chase and Simon, 1973a; Gobet et al., 2016). However, given that the chucks (domain-specific information) are the building blocks of one’s expertise, such information is not transferable across domains. Therefore, our behavioral results may reflect that the chucks of experts are invalid for untrained tasks.
In the present study, both groups showed similar activation patterns in the frontoparietal areas and matched task performances for both face and spatial WM conditions. However, when comparing neural activity between groups, BEs compared to novice had greater SPC activation during face WM, particularly in response of the contrast of the face 1-, 2-, and 3-back versus 0-back control conditions. The post hoc analysis revealed that this activation difference was caused by less SPC activation in BEs, compared to novices, for the 0-back condition. The SPC is known to be involved in the top-down allocation of visual spatial attention (Giesbrecht et al., 2003; Shomstein, 2012). Given that the 0-back condition requires sustained attention/vigilance or recognition to a pre-specified target rather than WM (Owen et al., 2005) and in this condition BEs had less SPC activation, it is conceivable that visual or attention processing, rather than WM, might be different between the two groups. For example, BEs may use less attentional resource for perception and recognition of visual stimuli including face that requires at least partly holistic processing like board games, through long-term training (Guida et al., 2012, 2013). One speculated mechanism underlying the decreases of neural activity and GMV in experts is the usage-dependent possible selective elimination of synapses (Huttenlocher and Dabholkar, 1997; Takeuchi et al., 2011). Another possible explanation for our neural findings is that neural activation patterns observed do not necessarily represent training-induced changes in untrained tasks because their effects are very likely to be domain-specific. A recent longitudinal study has found no effects of commercial web-based cognitive training on brain activity and behavioral performance during decision-making as untrained tasks (Kable et al., 2017). As mentioned above, the IQ-matching of the two groups eliminates differences in untrained cognitive abilities. That is why various studies have found training-related neural patterns even in the absence of transfer effects on cognitive ability.
Expertise in board game playing may be associated with the change of FC between brain regions, rather than regional neural activity, to efficiently solve domain-specific problems (Duan et al., 2012b; Jung et al., 2013) and this may further affect the functional brain network associated with specific cognitive functions (e.g., the fronto-parietal network associated with WM). Consistent with this expectation, we found significant group differences in the multivariate patterns of FC in the left FP and IPC during the face and spatial WM, respectively, using a new data-driven multivariate approach, called MDMR-based CWAS (Shehzad et al., 2014). The post hoc seed-based FC analyses for these identified clusters further revealed group differences in FC between specific brain regions; BE compared to novices had greater FC between the left FP seed and several temporal and parietal areas, including the left MTC and right SMC and FFA, during face WM and greater FC between the left IPC seed and left LFC during spatial WM. Neuroimaging studies have consistently reported the co-activation of multiple frontal and parietal regions during spatial attention (LaBar et al., 1999; Ptak, 2012), WM (LaBar et al., 1999; Owen et al., 2005), and fluid reasoning tasks (Lee et al., 2006; Hampshire et al., 2011), suggesting the involvement of the frontoparietal network in such cognitive functions. A meta-analysis of functional neuroimaging studies using n-back tasks has been reported that the FP is one of regions consistently activated across all n-back studies and that has suggested that the FP plays an important role in the coordination of information processing and information transfer between multiple operations when solving the problem that requires two or more separate cognitive operations than one discrete cognitive process (Owen et al., 2005). The IPC, FFA, and MTG are higher-order visual areas. The IPC is involved in spatial perception as part of dorsal visual stream, whereas the FFA and MTG are involved in object and motion perception as part of ventral visual stream (Ungerleider and Haxby, 1994). Especially, the FFA is considered as a general visual expertise module that mediates automatic holistic processing of any higher familiar visual stimuli rather than face (Gauthier et al., 1999, 2000), while the posterior part of MTC adjacent to STS mediates object and face recognition (Hein and Knight, 2008; Bilalić et al., 2010). Recent studies using chess-related tasks for chess experts have demonstrated that both the MTC and FFA are related to object and pattern recognition for chess pieces and positions, respectively (Bilalić et al., 2010, 2011; Bilaliæ, 2016) and that the IPC is involved in an active search for patterns or chunks when processing distorted structure in their trained domain, such as random chessboards (Bartlett et al., 2013). Based on aforementioned findings and roles of these regions mentioned above in cognitive components involved in playing board games, altered FC between these regions in BEs may be associated with visual expertise acquired through long-term training. Our results may reflect the functional reorganization of BEs’ brain in a way that increases the strength of FC between frontal and parietal regions for spatial WM or adds new functional interactions between regions in the network and other regions, including FFA and MTC, for face WM that requires holistic processing.
The present study had some limitations to be addressed in future research. First, a relatively small sample size and scanning on a 1.5 T magnet may lead to resultant low statistical power and to limit the spatial resolution, respectively. However, our sample size corresponded to samples used in previous studies examining the differences in brain function between BEs and novices (Bilalić et al., 2011; Bilaliæ, 2016). Given that the neural data are not always consistent and there is currently an increasing interest in replication in psychology, future research with larger samples is needed to confirm the reliability of the present findings. Second, as a result of the cross-section nature of this study, it is unclear that brain function differences we found are directly caused by Baduk training or they are pre-existing group differences that predict whether or not a person takes up Baduk rather than a result of that training. Third, considering previous studies showing an interaction between resting-state activity and stimulus-induced activity (Northoff et al., 2010; Fransson et al., 2018) and significant differences in resting-state activity between experts and novices (Jung et al., 2013; Dong et al., 2014, 2015), it is speculated that different resting-state activity patterns between the two groups may be the foundation for the activity difference during task-state. Further research with both resting-state and task-stat fMRI will help to clarify this issue. Finally, we used the WM tasks and found neural differences between experts and novices. Thus, our findings raise some questions to be explored by future research. Do the aspects of brain function where we have identified differences are associated only with WM tasks, or are they also associated with domain-specific cognitive skills (recall of Baduk positions)? Future longitudinal studies with measure of both trained and untrained tasks are needed to address such issue.
To our knowledge, this is the first study to examine whether there were differences in the functional activity and connectivity between BEs and novices while performing standard n-back WM task with both the face and spatial WM conditions, associated with the frontoparietal network, unlike previous studies to test domain-specific pattern recognition. Despite no behavioral differences, greater SPC activation in BEs compared to novices was observed during face WM. We also found altered connectivity in the FP and IPC in BEs in terms of multivariate patterns of FC using a new data-driven multivariate FC approach and further observed greater FC between frontal and parietal and temporal regions in BEs during WM. Our results provide novel insights into the mechanism behind Baduk expertise beyond domain-specific cognitive ability and provide evidence for differences in brain circuits associated with WM ability between experts and novices.
Author Contributions
WJ and JK designed and supervised the research. WJ, YY, and C-HC performed the experiments and analyzed data. WJ, YY, and TL wrote the manuscript. All authors reviewed the manuscript.
Funding
This research was supported by Basic Science Research Program through the National Research Foundation of Korea (NRF) funded by the Ministry of Science, ICT and Future Planning (Grant No. 2016R1E1A1A02921618). This work was also supported by Korea University Grant to WJ.
Conflict of Interest Statement
The authors declare that the research was conducted in the absence of any commercial or financial relationships that could be construed as a potential conflict of interest.
The reviewer VT and handling Editor declared their shared affiliation.
Acknowledgments
We thank Baduk experts for participating, the Korea Baduk Association for supporting this study, Dr. Zarrar Shehzad for development of the Connector toolbox, and researchers for helpful comments on the earlier draft of the manuscript.
Footnotes
- ^ http://english.Baduk.or.kr
- ^ http://english.Baduk.or.kr/
- ^ www.fil.ion.ucl.ac.uk/spm
- ^ http://czarrar.github.io/connectir/
- ^ http://marsbar.sourceforge.net/
References
Atherton, M., Zhuang, J., Bart, W. M., Hu, X., and He, S. (2003). A functional MRI study of high-level cognition. I. The game of chess. Brain Res. Cogn. Brain Res. 16, 26–31.
Bartlett, J. C., Boggan, A. L., and Krawczyk, D. C. (2013). Expertise and processing distorted structure in chess. Front. Hum. Neurosci. 7:825. doi: 10.3389/fnhum.2013.00825
Bilaliæ, M. (2016). Revisiting the role of the fusiform face area in expertise. J. Cogn. Neurosci. 28, 1345–1357. doi: 10.1162/jocn_a_00974
Bilalić, M., Langner, R., Erb, M., and Grodd, W. (2010). Mechanisms and neural basis of object and pattern recognition: a study with chess experts. J. Exp. Psychol. Gen. 139, 728–742. doi: 10.1037/a0020756
Bilalić, M., Langner, R., Ulrich, R., and Grodd, W. (2011). Many faces of expertise: fusiform face area in chess experts and novices. J. Neurosci. 31, 10206–10214. doi: 10.1523/JNEUROSCI.5727-10.2011
Bilalić, M., McLeod, P., and Gobet, F. (2008). Inflexibility of experts–reality or myth? Quantifying the einstellung effect in chess masters. Cogn. Psychol. 56, 73–102. doi: 10.1016/j.cogpsych.2007.02.001
Bilalić, M., McLeod, P., and Gobet, F. (2009). Specialization effect and its influence on memory and problem solving in expert chess players. Cogn. Sci. 33, 1117–1143. doi: 10.1111/j.1551-6709.2009.01030.x
Burgoyne, A. P., Sala, G., Gobet, F., Macnamara, B. N., Campitelli, G., and Hambrick, D. Z. (2016). The relationship between cognitive ability and chess skill: a comprehensive meta-analysis. Intelligence 59, 72–83. doi: 10.1037/bul0000139
Campitelli, G., and Gobet, F. (2011). Deliberate practice: necessary but not sufficient. Curr. Dir. Psychol. Sci. 20, 280–285. doi: 10.1177/0963721411421922
Chase, W. G., and Simon, H. A. (1973a). Perception in chess. Cogn. Psychol. 4, 55–81. doi: 10.1016/0010-0285(73)90004-2
Chase, W. G., and Simon, H. A. (1973b). “The mind’s eye in chess,” in Visual Information Processing, ed. W. G. Chase (New York, NY: Academic Press).
Chen, X., Zhang, D., Zhang, X., Li, Z., Meng, X., He, S., et al. (2003). A functional MRI study of high-level cognition. II. The game of go. Brain Res. Cogn. Brain Res. 16, 32–37. doi: 10.1016/S0926-6410(02)00206-9
Dong, M., Li, J., Shi, X., Gao, S., Fu, S., Liu, Z., et al. (2015). Altered baseline brain activity in experts measured by amplitude of low frequency fluctuations (ALFF): a resting state fMRI study using expertise model of acupuncturists. Front. Hum. Neurosci. 9:99. doi: 10.3389/fnhum.2015.00099
Dong, M., Qin, W., Zhao, L., Yang, X., Yuan, K., Zeng, F., et al. (2014). Expertise modulates local regional homogeneity of spontaneous brain activity in the resting brain: an fMRI study using the model of skilled acupuncturists. Hum. Brain Mapp. 3, 1074–1084. doi: 10.1002/hbm.22235
Duan, X., He, S., Liao, W., Liang, D., Qiu, L., Wei, L., et al. (2012a). Reduced caudate volume and enhanced striatal-DMN integration in chess experts. Neuroimage 60, 1280–1286. doi: 10.1016/j.neuroimage.2012.01.047
Duan, X., Liao, W., Liang, D., Qiu, L., Gao, Q., Liu, C., et al. (2012b). Large-scale brain networks in board game experts: insights from a domain-related task and task-free resting state. PLoS One 7:e32532. doi: 10.1371/journal.pone.0032532
Duan, X., Long, Z., Chen, H., Liang, D., Qiu, L., Huang, X., et al. (2014). Functional organization of intrinsic connectivity networks in Chinese-chess experts. Brain Res. 1558, 33–43. doi: 10.1016/j.brainres.2014.02.033
Ericsson, K. A., Krampe, R. T., and Tesch-Römer, C. (1993). The role of deliberate practice in the acquisition of expert performance. Psychol. Rev. 100, 363–406. doi: 10.1037/0033-295X.100.3.363
Fransson, P., Schiffler, B. C., and Thompson, W. H. (2018). Brain network segregation and integration during an epoch-related working memory fMRI experiment. Neuroimage 178, 147–161. doi: 10.1016/j.neuroimage.2018.05.040
Gauthier, I., Skudlarski, P., Gore, J. C., and Anderson, A. W. (2000). Expertise for cars and birds recruits brain areas involved in face recognition. Nat. Neurosci. 3, 191–197. doi: 10.1038/72140
Gauthier, I., Tarr, M. J., Anderson, A. W., Skudlarski, P., and Gore, J. C. (1999). Activation of the middle fusiform ‘face area’ increases with expertise in recognizing novel objects. Nat. Neurosci. 2, 568–573. doi: 10.1038/9224
Giesbrecht, B., Woldorff, M. G., Song, A. W., and Mangun, G. R. (2003). Neural mechanisms of top-down control during spatial and feature attention. Neuroimage 19, 496–512. doi: 10.1016/S1053-8119(03)00162-9
Gobet, F., and Charness, N. (2006). Expertise in Chess. The Cambridge Handbook of Expertise and Expert Performance. Cambridge, MA: Cambridge University Press.
Gobet, F., Lloyd-Kelly, M., and Lane, P. C. (2016). What’s in a name? The multiple meanings of “chunk” and “chunking”. Front. Psychol. 7:102. doi: 10.3389/fpsyg.2016.00102
Gobet, F., and Simon, H. A. (1996a). Recall of rapidly presented random chess positions is a function of skill. Psychon. Bull. Rev. 3, 159–163. doi: 10.3758/BF03212414
Gobet, F., and Simon, H. A. (1996b). Recall of random and distorted chess positions: implications for the theory of expertise. Mem. Cognit. 24, 493–503.
Guida, A., Gobet, F., and Nicolas, S. (2013). Functional cerebral reorganization: a signature of expertise? Reexamining Guida, Gobet, Tardieu, and Nicolas’ (2012) two-stage framework. Front. Hum. Neurosci. 7:590. doi: 10.3389/fnhum.2013.00590
Guida, A., Gobet, F., Tardieu, H., and Nicolas, S. (2012). How chunks, long-term working memory and templates offer a cognitive explanation for neuroimaging data on expertise acquisition: a two-stage framework. Brain Cogn. 79, 221–244. doi: 10.1016/j.bandc.2012.01.010
Hambrick, D. Z., Burgoyne, A. P., Macnamara, B. N., and Ullén, F. (2018). Toward a multifactorial model of expertise: beyond born versus made. Ann. N. Y. Acad. Sci. doi: 10.1111/nyas.13586 [Epub ahead of print].
Hampshire, A., Thompson, R., Duncan, J., and Owen, A. M. (2011). Lateral prefrontal cortex subregions make dissociable contributions during fluid reasoning. Cereb. Cortex 21, 1–10. doi: 10.1093/cercor/bhq085
Hänggi, J., Brütsch, K., Siegel, A. M., and Jäncke, L. (2014). The architecture of the chess player’s brain. Neuropsychologia 62, 152–162. doi: 10.1016/j.neuropsychologia.2014.07.019
Hein, G., and Knight, R. T. (2008). Superior temporal sulcus–It’s my area: or is it? J. Cogn. Neurosci. 20, 2125–2136. doi: 10.1162/jocn.2008.20148
Huttenlocher, P. R., and Dabholkar, A. S. (1997). Regional differences in synaptogenesis in human cerebral cortex. J. Comp. Neurol. 387, 167–178. doi: 10.1002/(SICI)1096-9861(19971020)387:2<167::AID-CNE1>3.0.CO;2-Z
Itoh, K., Kitamura, H., Fujii, Y., and Nakada, T. (2008). Neural substrates for visual pattern recognition learning in Igo. Brain Res. 1227, 162–173. doi: 10.1016/j.brainres.2008.06.080
Jung, W. H., Kim, S. N., Lee, T. Y., Jang, J. H., Choi, C. H., Kang, D. H., et al. (2013). Exploring the brains of Baduk (Go) experts: gray matter morphometry, resting-state functional connectivity, and graph theoretical analysis. Front. Hum. Neurosci. 7:633. doi: 10.3389/fnhum.2013.00633
Kable, J. W., Caulfield, M. K., Falcone, M., McConnell, M., Bernardo, L., Parthasarathi, T., et al. (2017). No effect of commercial cognitive training on brain activity, choice behavior, or cognitive performance. J. Neurosci. 37, 7390–7402. doi: 10.1523/JNEUROSCI.2832-16.2017
Kanwisher, N., McDermott, J., and Chun, M. M. (1997). The fusiform face area: a module in human extrastriate cortex specialized for face perception. J. Neurosci. 17, 4302–4311. doi: 10.1523/JNEUROSCI.17-11-04302.1997
Keene, R., and Levy, D. (1991). How to Beat your Chess Computer (Batsford Chess Library). London: Henry Holt and Company Publisher.
Krawczyk, D. C., Boggan, A. L., McClelland, M. M., and Bartlett, J. C. (2011). The neural organization of perception in chess experts. Neurosci. Lett. 499, 64–69. doi: 10.1016/j.neulet.2011.05.033
LaBar, K. S., Gitelman, D. R., Parrish, T. B., and Mesulam, M. (1999). Neuroanatomic overlap of working memory and spatial attention networks: a functional MRI comparison within subjects. Neuroimage 10, 695–704. doi: 10.1006/nimg.1999.0503
Lee, B., Park, J. Y., Jung, W. H., Kim, H. S., Oh, J. S., Choi, C. H., et al. (2010). White matter neuroplastic changes in long-term trained players of the game of “Baduk” (GO): a voxel-based diffusion-tensor imaging study. Neuroimage 52, 9–19. doi: 10.1016/j.neuroimage.2010.04.014
Lee, K. H., Choi, Y. Y., Gray, J. R., Cho, S. H., Chae, J. H., Lee, S., et al. (2006). Neural correlates of superior intelligence: stronger recruitment of posterior parietal cortex. Neuroimage 29, 578–586. doi: 10.1016/j.neuroimage.2005.07.036
Macnamara, B. N., Hambrick, D. Z., and Oswald, F. L. (2014). Deliberate practice and performance in music, games, sports, education, and professions: a meta-analysis. Psychol. Sci. 25, 1608–1618. doi: 10.1177/0956797614535810
Mosing, M. A., Madison, G., Pedersen, N. L., and Ullén, F. (2016). Investigating cognitive transfer within the framework of music practice: genetic pleiotropy rather than causality. Dev. Sci. 19, 504–512. doi: 10.1111/desc.12306
Mosing, M. A., Pedersen, N. L., Madison, G., and Ullén, F. (2014). Genetic pleiotropy explains associations between musical auditory discrimination and intelligence. PLoS One 9:e113874. doi: 10.1371/journal.pone.0113874
Neumann, N., Lotze, M., and Eickhoff, S. B. (2016). Cognitive expertise: an ale meta-analysis. Hum. Brain Mapp. 37, 262–272. doi: 10.1002/hbm.23028
Northoff, G., Qin, P., and Nakao, T. (2010). Rest-stimulus interaction in the brain: a review. Trends Neurosci. 33, 277–284. doi: 10.1016/j.tins.2010.02.006
Owen, A. M., McMillan, K. M., Laird, A. R., and Bullmore, E. (2005). N-back working memory paradigm: a meta-analysis of normative functional neuroimaging studies. Hum. Brain Mapp. 25, 46–59. doi: 10.1002/hbm.20131
Power, J. D., Barnes, K. A., Snyder, A. Z., Schlaggar, B. L., and Petersen, S. E. (2012). Spurious but systematic correlations in functional connectivity MRI networks arise from subject motion. Neuroimage 59, 2142–2154. doi: 10.1016/j.neuroimage.2011.10.018
Ptak, R. (2012). The frontoparietal attention network of the human brain: action, saliency, and a priority map of the environment. Neuroscientist 18, 502–515. doi: 10.1177/1073858411409051
Sala, G., Burgoyne, A. P., Macnamara, B. N., Hambrick, D. Z., Campitelli, G., and Gobet, F. (2017). Checking the “academic selection” argument. chess players outperform non-chess players in cognitive skills related to intelligence: a meta-analysis. Intelligence 61, 130–139. doi: 10.1016/j.intell.2017.01.013
Sala, G., and Gobet, F. (2016). Do the benefits of chess instruction transfer to academic and cognitive skills? a meta-analysis. Educ. Res. Rev. 18, 46–57. doi: 10.1016/j.edurev.2016.02.002
Sala, G., and Gobet, F. (2017a). Does far Transfer exist? Negative evidence from chess, music, and working memory training. Curr. Dir. Psychol. Sci. 26, 515–520. doi: 10.1177/0963721417712760
Sala, G., and Gobet, F. (2017b). Experts’ memory superiority for domain-specific random material generalizes across fields of expertise: a meta-analysis. Mem. Cognit. 45, 183–193. doi: 10.3758/s13421-016-0663-2
Satterthwaite, T. D., Cook, P. A., Bruce, S. E., Conway, C., Mikkelsen, E., Satchell, E., et al. (2016). Dimensional depression severity in women with major depression and post-traumatic stress disorder correlates with fronto-amygdalar hypoconnectivty. Mol. Psychiatry 21, 894–902. doi: 10.1038/mp.2015.149
Satterthwaite, T. D., Vandekar, S. N., Wolf, D. H., Bassett, D. S., Ruparel, K., Shehzad, Z., et al. (2015). Connectome-wide network analysis of youth with psychosis-spectrum symptoms. Mol. Psychiatry 20, 1508–1515. doi: 10.1038/mp.2015.66
Shehzad, Z., Kelly, C., Reiss, P. T., Cameron Craddock, R., Emerson, J. W., McMahon, K., et al. (2014). A multivariate distance-based analytic framework for connectome-wide association studies. Neuroimage 93(Pt 1), 74–94. doi: 10.1016/j.neuroimage.2014.02.024
Shin, N. Y., Park, H. Y., Jung, W. H., Park, J. W., Yun, J. Y., Jang, J. H., et al. (2015). Effects of oxytocin on neural response to facial expressions in patients with schizophrenia. Neuropsychopharmacology 40, 1919–1927. doi: 10.1038/npp.2015.41
Shin, Y. W., Na, M. H., Ha, T. H., Kang, D. H., Yoo, S. Y., and Kwon, J. S. (2008). Dysfunction in configural face processing in patients with schizophrenia. Schizophr. Bull. 34, 538–543. doi: 10.1093/schbul/sbm118
Shomstein, S. (2012). Cognitive functions of the posterior parietal cortex: top-down and bottom-up attentional control. Front. Integr. Neurosci. 6:38. doi: 10.3389/fnint.2012.00038
Takeuchi, H., Taki, Y., Hashizume, H., Sassa, Y., Nagase, T., Nouchi, R., et al. (2011). Effects of training of processing speed on neural systems. J. Neurosci. 31, 12139–12148. doi: 10.1523/JNEUROSCI.2948-11.2011
Ungerleider, L. G., and Haxby, J. V. (1994). ‘What’ and ‘where’ in the human brain. Curr. Opin. Neurobiol. 4, 157–165. doi: 10.1016/0959-4388(94)90066-3
Wan, X., Cheng, K., and Tanaka, K. (2015). Neural encoding of opposing strategy values in anterior and posterior cingulate cortex. Nat. Neurosci. 18, 752–759. doi: 10.1038/nn.3999
Wan, X., Nakatani, H., Ueno, K., Asamizuya, T., Cheng, K., and Tanaka, K. (2011). The neural basis of intuitive best next-move generation in board game experts. Science 331, 341–346. doi: 10.1126/science.1194732
Wan, X., Takano, D., Asamizuya, T., Suzuki, C., Ueno, K., Cheng, K., et al. (2012). Developing intuition: neural correlates of cognitive-skill learning in caudate nucleus. J. Neurosci. 32, 17492–17501. doi: 10.1523/JNEUROSCI.2312-12.2012
Keywords: board game, connectome, frontoparietal network, functional connectivity, holistic processing
Citation: Jung WH, Lee TY, Yoon YB, Choi C-H and Kwon JS (2018) Beyond Domain-Specific Expertise: Neural Signatures of Face and Spatial Working Memory in Baduk (Go Game) Experts. Front. Hum. Neurosci. 12:319. doi: 10.3389/fnhum.2018.00319
Received: 27 February 2018; Accepted: 23 July 2018;
Published: 07 August 2018.
Edited by:
Antonino Vallesi, Università degli Studi di Padova, ItalyReviewed by:
Quanying Liu, California Institute of Technology, United StatesMinghao Dong, Xidian University, China
Vincenza Tarantino, Università degli Studi di Padova, Italy
Copyright © 2018 Jung, Lee, Yoon, Choi and Kwon. This is an open-access article distributed under the terms of the Creative Commons Attribution License (CC BY). The use, distribution or reproduction in other forums is permitted, provided the original author(s) and the copyright owner(s) are credited and that the original publication in this journal is cited, in accordance with accepted academic practice. No use, distribution or reproduction is permitted which does not comply with these terms.
*Correspondence: Jun Soo Kwon, a3dvbmpzQHNudS5hYy5rcg==