- 1Department of Psychiatry, Psychotherapy and Psychosomatics, Medical Faculty, RWTH Aachen University, Aachen, Germany
- 2Natural Media Lab, Human Technology Centre (HumTec), RWTH Aachen University, Aachen, Germany
- 3Center for Sign Language and Gesture (SignGes), RWTH Aachen University, Aachen, Germany
- 4Brain Imaging Facility, Interdisciplinary Centre for Clinical Studies (IZKF), Medical Faculty, RWTH Aachen University, Aachen, Germany
- 5Department of Biomedical Engineering, Faculty of Electronic Information and Electrical Engineering, Dalian University of Technology, Dalian, China
- 6JARA-Translational Brain Medicine, Aachen, Germany
Social interactions arise from patterns of communicative signs, whose perception and interpretation require a multitude of cognitive functions. The semiotic framework of Peirce’s Universal Categories (UCs) laid ground for a novel cognitive-semiotic typology of social interactions. During functional magnetic resonance imaging (fMRI), 16 volunteers watched a movie narrative encompassing verbal and non-verbal social interactions. Three types of non-verbal interactions were coded (“unresolved,” “non-habitual,” and “habitual”) based on a typology reflecting Peirce’s UCs. As expected, the auditory cortex responded to verbal interactions, but non-verbal interactions modulated temporal areas as well. Conceivably, when speech was lacking, ambiguous visual information (unresolved interactions) primed auditory processing in contrast to learned behavioral patterns (habitual interactions). The latter recruited a parahippocampal-occipital network supporting conceptual processing and associative memory retrieval. Requesting semiotic contextualization, non-habitual interactions activated visuo-spatial and contextual rule-learning areas such as the temporo-parietal junction and right lateral prefrontal cortex. In summary, the cognitive-semiotic typology reflected distinct sensory and association networks underlying the interpretation of observed non-verbal social interactions.
Introduction
1During social interactions, a multitude of auditory and visual cues interact to convey meaning. These cues range from spoken words and manual gestures to facial expressions, eye gaze, body orientation, and body movements (Lotze et al., 2006; Schilbach et al., 2006; Becchio et al., 2012; Saggar et al., 2014). Interpreting social interactions requires the interaction of various cognitive functions; among these are social attention mechanisms, mentalizing, language comprehension, and the recognition of faces, communicative gestures, goal-directed movements, and emotions (Ochsner and Lieberman, 2001; Adolphs, 2009; Hari and Kujala, 2009). In a laboratory setting, naturalistic stimuli such as movies or film sequences capture this complexity because they provide a means to represent the interacting information dynamically and within context (Spiers and Maguire, 2007; Hasson and Honey, 2012; Willems, 2015). With regard to social interactions in complex scenes, validated comprehensive typologies are lacking and their neural processing is unclear.
During watching movies or film sequences, different neural networks were found involved depending on the study protocol and stimulus material. The most consistently reported neural correlates of social-cognitive functions encompass superior temporal gyrus (STG), temporo-parietal junction (TPJ), medial prefrontal cortex (PFC), fusiform gyrus, and precuneus (Iacoboni et al., 2004; Wolf et al., 2010; Lahnakoski et al., 2012; Wagner et al., 2016). In particular, the posterior STG and TPJ of the right hemisphere serve as key regions during the processing of real-life social interactions and joint attention (Redcay et al., 2010; for a meta-analysis see Krall et al., 2015). Both regions may contribute to the analysis of social relations in movie clips (Iacoboni et al., 2004). Specific social signals in movies (e.g., faces, movement, social interactions, or speech) may even partially segregate four networks (Lahnakoski et al., 2012): Scenes depicting social interactions engaged two of those networks, namely a temporal-amygdala network and a prefrontal-insula network. The introduction of an explicit mentalizing task modulated neuronal recruitment patterns (Wolf et al., 2010).; rating an agent’s intention activated three independent neural networks, i.e., for face processing and recognition, language comprehension, as well as self-referential mental activity. Taken together, the significance of several cortical and subcortical structures for the processing of social interaction has become evident, but their specific contribution to the interpretation of interaction events, especially during naturalistic stimulation, remains to be determined.
Although social interactions comprise several interwoven, multimodal cues, a behavioral pattern, as a whole, acts as a communicative sign and can thus be described using sign theory (semiotics). Semiotic categories describe cognitive categories, among other related phenomena (Holenstein, 2008). Thus, semiotic models are amenable to cognitive-behavioral and neuroscientific testing (Paolucci, 2011; Galantucci et al., 2012; Zlatev, 2012). Among one of the most influential contributions to the field of semiotics are the UCs introduced by Charles Sanders Peirce (Peirce, 1955, 1960). Peirce’s theory has been considered an appropriate semiotic framework for the study of cognitive processes (Daddesio, 1994; Stjernfelt, 2007; Fusaroli and Paolucci, 2011; Sonesson, 2014) and has been applied to theoretical and empirical investigations in the fields of cognitive semiotics, linguistics, media science, and neurosciences. This semiotic theory is well suited for neuroimaging studies of multimodal communication because it emphasizes the perspective of the interpreting mind (Peirce, 1955), represented by the observers brain activity. This framework may describe the relation between perceived signs (e.g., the interactions shown in movie clips) and the interpretant (Peirce, 1955), i.e., the resulting cognitive representation in the participant’s mind and subsequent brain activity during a functional magnetic resonance imaging (fMRI) experiment.
With regard to the representation, transmission, and interpretation of signs, Peirce’s UCs distinguish between three levels: “Firstness” pertains to potential, not-yet-resolved meaning; “Secondness” encapsulates the specific, contextualized meaning of a sign, particularly of non-habitual and non-conventional signs; and “Thirdness” involves entrenched habits, patterns, and rules (Peirce, 1960). While semiotic categories typically interact to various degrees in a given sign process, one of them can be expected to predominate and, thus, determine the sign’s main function, as well as the way it is perceived and interpreted (Peirce, 1955). We categorized non-verbal social interactions occurring within a film sequence into three types (Figure 1): (1) “unresolved” interactions are ambiguous in the respective situation and their outcomes are not yet determined (emphasizing Firstness); (2) “non-habitual” interactions counteract learned behavioral patterns and are disambiguated by the local context (emphasizing Secondness); and (3) “habitual interactions” include implicitly or explicitly learned behavioral patterns, which conform to social conventions (emphasizing Thirdness). (4) Verbal interactions largely rely on the conventional codes of a given language and culture and are subsumed in a forth category (high degree of Thirdness; see Figure 1). This operationalization aims at the description of the sign-interpretant relation with respect to the UCs. We yielded three processing modes for the interpretation of non-verbal interactions and one for all verbal interactions, because spoken language is highly conventionalized and dominated by the Thirdness category.
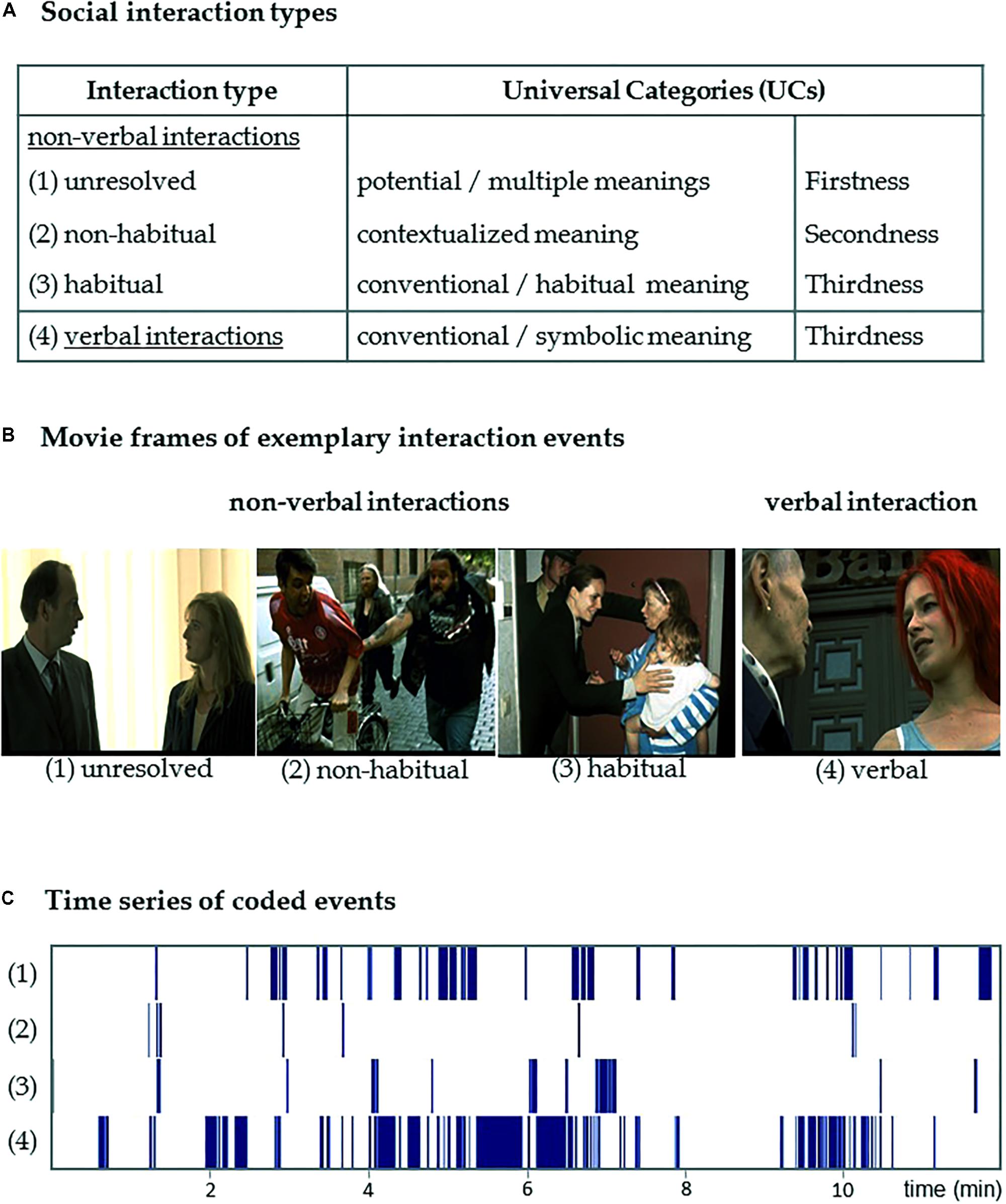
FIGURE 1. Coded interaction types. Social interaction events occurring in the movie excerpt were annotated. (A) Peirce’s semiotic framework of the Universal Categories inspired the differentiation of three cognitive processes during the interpretation of non-verbal and one during verbal social interactions. (1) Unresolved non-verbal interactions are ambiguous and the social situation is not yet resolved (reflecting the Firstness category of potential, not-yet-resolved meaning); (2) “non-habitual interactions” counteract learned behavioral patterns and are disambiguated by the local context (reflecting the Secondness category of contextualized meaning); (3) “habitual interactions” rely on learned behavioral patterns and social conventions (reflecting the Thirdness category of conventional and habitual meaning); and, finally (4) words rely on the conventional codes of the language and thus verbal interaction reflect always the Thirdness category. (B) The movie frames depict exemplary events for each of the coded types of social interactions: (1) unresolved: two people wordlessly stare at each other; (2) non-habitual: a cyclist is grabbed by a passerby; (3) habitual: a carried child is touched in greeting; and (4) verbal: two persons engage in a conversation. (C) Visualization of the time series of coded events in the 12-min movie excerpt. Copyrights of screen shots: Tykwer (1998).
Peirce’s UCs were chosen as the theoretical background to study social interactions because they have inspired various theoretical models that have been widely used to describe and interpret communicative actions and signs. However, experimental evidence is still scarce for Peirce’s basic constructs. Peirce aimed at a general semiotic theory that accounts for all kinds of sign processes in all kinds of modalities, including those occurring in nature and scientific inquiry; hence, his model of sign processes goes beyond communication per se (Jensen, 1995; Nöth, 2001). The UCs have inspired theoretical models developed to characterize and interpret manual gestures (e.g., McNeill, 1992, 2005; Fricke, 2007; Mittelberg, 2008, 2013, 2018; Mittelberg and Waugh, 2014), onomatopoeia in language (Jakobson, 1966; Guynes, 2013), and images (Sonesson, 2005; Jappy, 2011), and further, describe narrative comprehension in both spoken and written stories (Lee, 2012), film sequences (Deleuze, 1986, 1989; Sykes, 2009) and comics (Magnussen, 2000; Cohn, 2007; see also Bateman et al., 2017 on multimodality). Qualitative analyses utilized aspects of Peirce’s UCs for the investigation of mental imagery, human gestures, language evolution, and developmental aspects of communication and culture (for a review, see Zlatev, 2012). However, despite many theoretical and qualitative approaches, empirical investigations are still rare. Best known, the sign-object relation is one aspect of the UCs, and has founded a prominent theoretical framework for empirical analyses of manual gestures (as “iconic” (UC1), “deictic” (UC2), and “emblematic” (UC3) gestures; McNeill, 1992) in behavioral and neuroimaging studies on gesture perception and comprehension (for reviews, see Özyürek, 2014; Wagner et al., 2014; Yang et al., 2015). The sign-interpretant relation of the UCs has scarcely been used as a construct for neuroimaging studies. We investigated conventionality in co-speech gestures with the sign-interpretant relation of thirdness (UC3, Wolf et al., 2018). Perceiving a gesture as conventional increased intersubject covariance (ISC) in left inferior frontal gyrus (IFG) and posterior STG. The present study employed aspects of the UCs to create stimulus categories in a neurocognitive experiment. Social interactions were labeled with semiotic categories derived from the UCs and their neural response patterns were analyzed.
Assuming that semiotic categories influence the cognitive and neural processing of the interpretant, the semiotic characterization of social interactions differentiates neural processes during the observation of social interactions. Functional MRI recorded neural responses to social interactions portrayed during a 20-min movie narrative. The three types of non-verbal interactions (unresolved, non-habitual, and habitual) and any verbal interactions were coded. First, we aimed to confirm that this method yields neural correlates of social interactions encompassing the relevant sensory, language, visual, and social cognitive networks. In specific, we hypothesized that brain areas supporting the interpretation of verbal interactions lean more toward auditory processes whereas non-verbal interactions elicit stronger visuo-spatial processing. Second, the recruitment of these brain areas during the observation of non-verbal interactions was expected to depend on the predominant semiotic category (visible by the extracted beta values). Third, we explored neural patterns contributing to the encoding of Peirce’s semiotic categories in a whole-brain analysis.
Materials and Methods
Study Participants
Sixteen right-handed native German speakers (seven women, age 26.1 ± 3.8, range 22–34 years) participated in the present study. Participants had normal or corrected-to-normal vision, normal hearing, and no history of psychiatric, neurological, or mental disorders. The study protocol was approved by the local Ethics Committee and the experiment was designed and conducted in accordance with the Declaration of Helsinki. All participants gave written informed consent and received financial reimbursement.
Stimulation
Participants were presented with a film sequence showing a 20-min excerpt from the German movie “Lola rennt” (Engl.: “Run Lola Run”; 10:20–30:20 min; X-Filme Creative Pool, Germany, 1998). The movie excerpt was chosen because it comprises a self-contained narrative with a fast-paced story line within a reasonable time frame (20-min). Video was delivered by a projector system with reflecting mirrors (Psychology Software Tools, Sharpsburg, PA, United States), and audio was delivered by earplugs (Nordic Neurolab Bergen, Norway). The sound was individually adjusted to a comfortable hearing level. Stimulus delivery and timing was controlled using the stimulation software Presentation (Neurobehavioral Systems Inc., United States). Before the film sequence started, a fixation cross was presented for 25 s. The participants were asked to watch attentively.
Coding of Social Interactions in the Movie
A film sequence is a naturalistic stimulus in which a multitude of features interact. In order to model the specific appearance of social interactions we used an established content-coding approach for model-based analysis (Mathiak and Weber, 2006; Weber et al., 2009; Mathiak et al., 2011, 2013; Klasen et al., 2012b). We annotated the onsets and duration of social interaction events in the movie excerpt on a frame-by-frame basis with 67 ms accuracy, corresponding to a 15 Hz frame rate. The content-coding system distinguished three types of non-verbal social interactions: (1) unresolved, (2) non-habitual, and (3) habitual; as well as four verbal interactions (for an overview, see Figure 1). For each event, exactly one of the interaction types was annotated. Verbal interactions were coded whenever speech was involved in the interaction event. The duration of a verbal interaction event corresponded to the duration of the utterance. Pauses up to two seconds between words or utterances were coded as continuous verbal interaction. Pauses lasting longer than two seconds were coded as a non-verbal interaction. Non-verbal interactions could immediately precede or follow a verbal interaction (e.g., an “unresolved interaction” is followed by a “verbal interaction”). Furthermore, non-verbal interaction types may immediately follow each other (e.g., an “unresolved interaction” is followed by a “habitual interaction”). The complete movie excerpt was annotated twice by two independent coders. Inter-coder reliability for the differentiation between interaction types was determined with Krippendorff’s alpha (.62). In cases when the coders disagreed, a supervisory decision was taken by a third coder (D.W.). In total, 170 interaction events were annotated yielding a total duration of 476.5 s (average duration: 2.8 ± 5.8 s, mean ± SD). Of those interaction events, 65 were coded as “unresolved” (total duration: 132.6 s; average: 1.7 ± 2.5 s), 19 events were coded as “non-habitual” (total duration: 10.0 s; average: 0.5 ± 0.6 s), 22 events were coded as “habitual” (total duration: 42.2 s; average: 1.9 ± 2.5 s), and 64 events were coded as “verbal” (total duration: 291.7 s; average: 4.6 ± 8.8 s).
MR Data Acquisition
Functional MRI was conducted using a 3 Tesla Siemens Scanner (Magnetom Trio, Siemens Medical Systems, Erlangen, Germany) and a 32-channel phased-array receive-only head coil. Echo planar imaging (EPI) collected functional images sensitive to the blood-oxygenation-level-dependent (BOLD) contrast. The applied EPI sequence acquired multiple echoes after a single excitation pulse. Subsequently, the obtained images were weighted and combined. This procedure increases signal-to-noise ratio (Bhavsar et al., 2014), which may be particularly beneficial for investigations that utilize naturalistic stimuli. With the following parameters, 487 volumes were acquired: 24 slices; echo time (TE) = 17.0, 45.9, and 74.9 ms; repetition time (TR) = 2540 ms; flip angle (FA) = 90°; slice thickness = 3.5 mm; slice gap = 0.5 mm; matrix size = 64 × 64, field of view (FOV) = 224 mm2 × 224 mm2; voxel size = 3.5 mm3 × 3.5 mm3 × 3.5 mm3, and bandwidth = 2232 Hz/pixel.
Data Analysis
Functional MRI data analysis was conducted using the software Statistical Parametric Mapping (SPM 8, Wellcome Department of Imaging Neuroscience UCL, London, United Kingdom). The first five volumes of each session were discarded to reduce T1 saturation effects. Images were spatially realigned, normalized to the stereotactic MNI space (Montreal Neurological Institute; Evans et al., 1993) and resampled to 2 mm × 2 mm×2 mm voxels. Spatial smoothing with a full-width-at-half-maximum (FWHM) Gaussian kernel of 12 mm was applied to the normalized data. The single-echo images were combined after normalization based on the optimized-CNR approach, i.e., each image voxel was weighted with a function of the estimated signal-to-noise ratio and the expected BOLD sensitivity (Mathiak et al., 2004; Poser et al., 2006; see Bhavsar et al., 2014 for detailed methodological descriptions).
The onset and duration of each coded event entered a general linear model (GLM) with one predictor of interest for each interaction type (unresolved, non-habitual, habitual, and verbal). Using the canonical response function, a model for the BOLD responses to the events was constructed. In the first-level statistical analysis, the contrast of the four stimuli types against the baseline (the ongoing movie) was determined. The four contrast maps of each subject entered the repeated-measures ANOVA for second-level analysis. An F-test determined effects of the four interaction types against baseline. Post hoc t-tests compared the verbal with the non-verbal interactions. A second F-test explored the activation patterns differentiating the semiotic categories of observed non-verbal interactions. Post hoc t-tests compared non-verbal interaction types in a pairwise fashion. Each map in the second-level group analyses was corrected with a voxelwise family-wise error (FWE; Friston et al., 1994) of p < 0.05. All remaining clusters survived a clusterwise pFWE < 0.05 thresholding.
Region-of-interest (ROI) analyses investigated differences between the three non-verbal interaction types. Therefore, the selected ROIs differentiated the verbal from the non-verbal interactions and vice versa. For the verbal condition, peak locations were obtained from the contrast “verbal > non-verbal.” For the non-verbal conditions, the inversed contrast “non-verbal > verbal” was considered; extended clusters were segregated into meaningful anatomical structures according to the AAL atlas (Tzourio-Mazoyer et al., 2002): fusiform gyrus (bilateral), calcarine gyrus (bilateral), right IFG pars triangularis (IFG PTr), and right middle frontal gyrus (MFG). Due to the orthogonality of the SPM second-level design matrix, we assumed orthogonality of the estimate for the “verbal versus non-verbal” contrast with the one for the contrasts between the non-verbal subtypes; therefore the data were explored with standard univariate ANOVAs. Significant differences across the non-verbal interaction types were determined with one-factor ANOVAs and corrected for multiple comparisons according to Bonferroni-Holm. Statistics were performed with IBM SPSS (Statistics for Windows, Version 20.0, Armonk, NY, United States).
In a supplementary analysis the validity of the obtained neural functioning for the processing of verbal information and social interaction was confirmed with anatomical masks from Neurosynth2; this toolbox provides neuroanatomical information based on meta-analysis of fMRI studies. The images for the terms “verbal” (615 studies) and “social interaction” (94 studies) were merged and served for small-volume correction of the contrasts “verbal > non-verbal” and “non-verbal > verbal” as well as the individual t-contrasts comparing the non-verbal interaction types. Segregation of anatomical structures and the extraction of ROI data were performed as described above. Significant differences in peak-voxel activation were explored with univariate ANOVAs.
Results
Neural Responses to the Observation of Social Interactions
During social interactions, several brain regions exhibited activity increases, including primary auditory and visual areas as well as higher order visual pathways (Figure 2 and Table 1). Previous studies reported medial PFC activations (e.g., Iacoboni et al., 2004), which emerged in our data only after lowering the threshold to p < 0.001 uncorrected. Differences between verbal and non-verbal interactions were investigated with t-tests contrasting responses to verbal interactions against the average response to the three non-verbal interaction types. The contrast “verbal > non-verbal” yielded strong bilateral activation in auditory processing areas (STG extending into middle temporal gyrus (MTG); Figure 3A and Table 2). The reversed contrast “non-verbal > verbal” yielded widespread brain regions, in particular, the visual pathway emerged encompassing occipital, inferior temporal, and superior parietal cortices as well as right prefrontal areas (Figure 3B and Table 2).
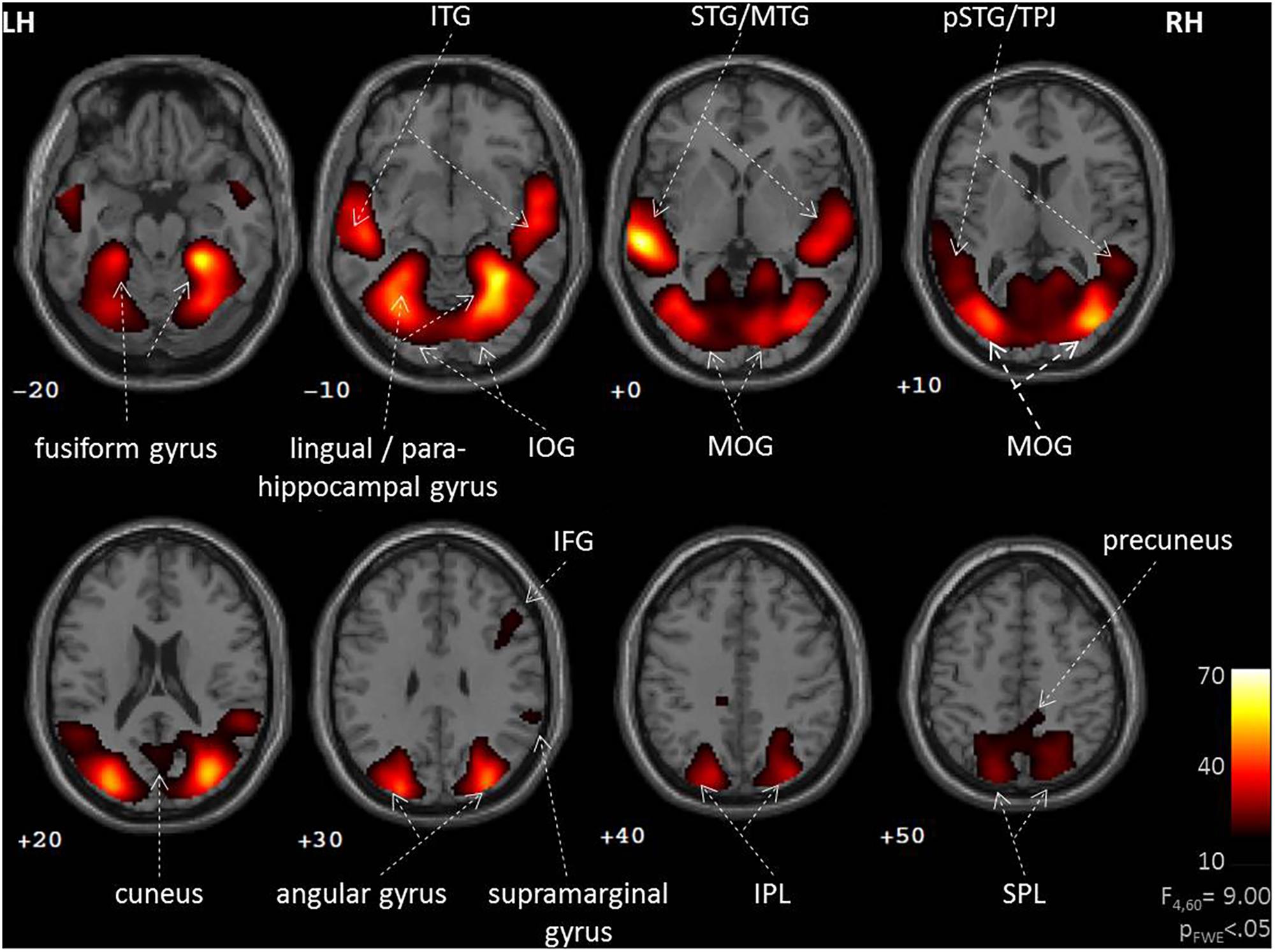
FIGURE 2. Neural correlates of social interactions. Social interaction events in a movie narrative were coded as four types (unresolved, non-habitual, habitual, and verbal). An F-test across the four predictors revealed widespread brain activation of auditory and visual networks. Such pattern is commonly observed during the perception of naturalistic social stimuli, suggesting an important role for the observation of social interaction events. LH, left hemisphere; RH, right hemisphere; IFG, inferior frontal gyrus; IOG, inferior occipital gyrus; IPL, inferior parietal lobe; ITG, inferior temporal gyrus; MOG, middle occipital gyrus; MTG, middle temporal gyrus; (p)STG, (posterior) superior temporal gyrus; SPL, superior parietal lobe; TPJ, temporo-parietal junction; z-coordinates are indicated beneath each slice.
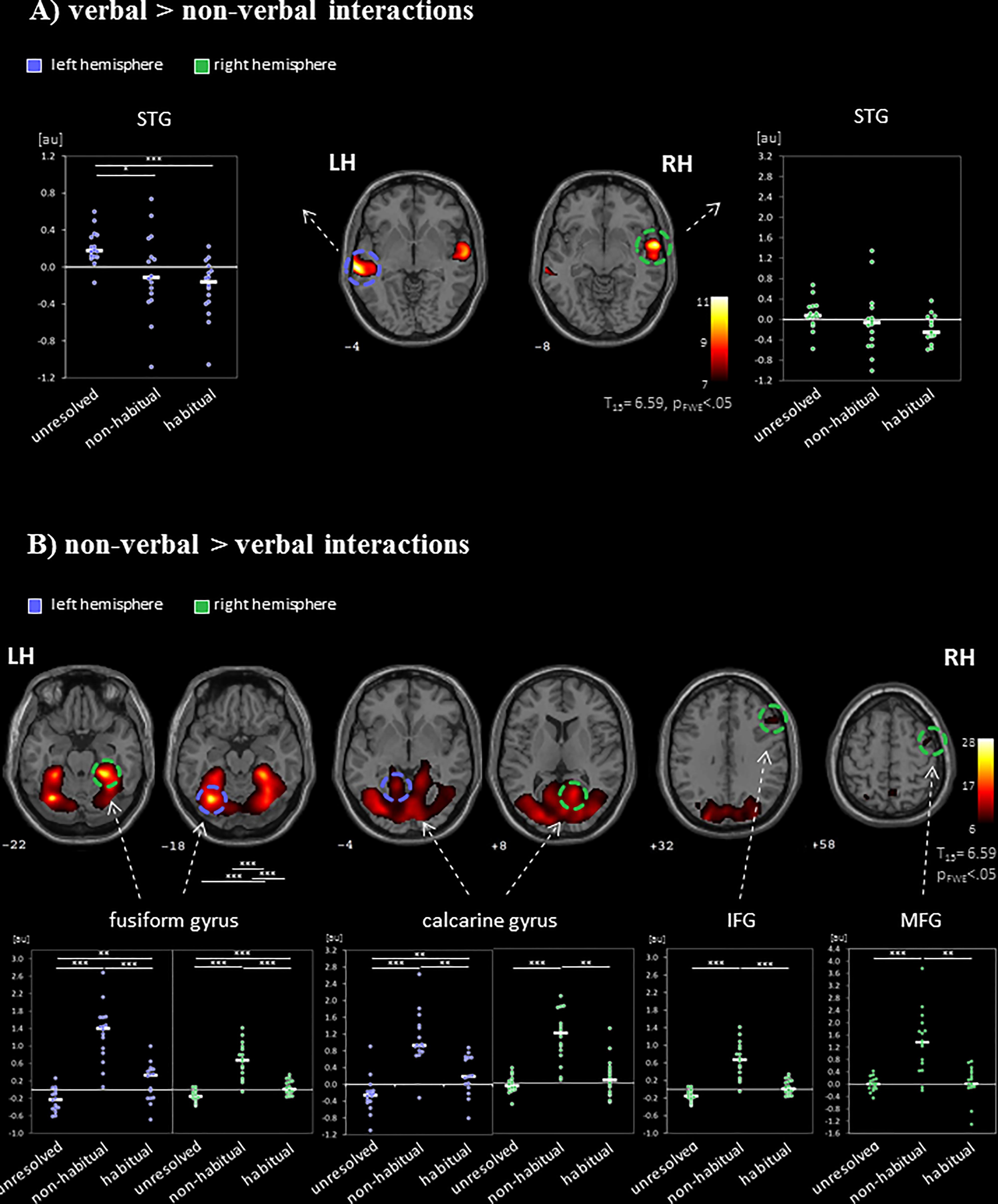
FIGURE 3. Neural networks for the processing of verbal and non-verbal interactions. The contrasts between (A) verbal (“verbal > non-verbal”) and (B) non-verbal (“non-verbal > verbal”) interactions separated the networks for visual-based and sound-based information processing. ROI analyses of regional peak voxels revealed specific contributions for the non-verbal interaction types (“unresolved,” “non-habitual,” and “habitual”). Auditory regions were recruited during the observations of unresolved interactions but suppressed during habitual interactions. Non-habitual interactions yielded the involvement of visual and prefrontal areas. LH, left hemisphere; RH, right hemisphere; IFG, inferior frontal gyrus; MFG, middle frontal gyrus; STG, superior temporal gyrus; ∗p < 0.05; ∗∗p < 0.01; ∗∗∗p < 0.001; z-coordinates are indicated beneath each slice.
To differentiate the effect of the three non-verbal interaction types, a ROI analysis was performed in the peak-voxels of the contrasts between verbal and non-verbal interactions. Verbal interactions yielded higher responses in bilateral auditory cortices than non-verbal ones [left: MNI (x,y,z) = −62, −26, −2 (STG); right: 62, 0, −8 (STG); Figure 3A]. In the left hemispheric ROI only, a modulation with respect to the three non-verbal interaction types emerged (left: F2,45 = 7.00, p = 0.002; right: F2,45 = 1.78, p = 0.18, n.s.). The activation differences were characterized by a gradient from unresolved to non-habitual to habitual interactions (see inserts in Figure 3A): In post hoc t-tests, unresolved interactions yielded higher activity as compared to habitual interactions (t15 = 5.28, p < 0.001) and to non-habitual interactions (t15 = 2.60, p = 0.02; habitual versus non-habitual: t15 = 0.98, p = 0.344, n.s.). For the non-verbal contrast, six ROIs emerged: left fusiform gyrus (−32, −64, and −18), right fusiform gyrus (28, −38, and −22), left calcarine gyrus (2, −80, and −4), right calcarine gyrus (16, −56, and 8), right IFG PTr (54, 24, and 32), and right MFG (44, 0, and 58). In each ROI a significant difference between the non-verbal conditions emerged (all p < 0.001; Figure 3B). In the post hoc t-test, non-habitual interactions yielded larger activation than the two other types (all t15 > 3.80, p < 0.003; see inserts in Figure 3B). After applying the Neurosynth-based mask, two ROIs emerged for verbal interactions over nonverbal interactions (peak voxels: left STG: −62, −26, and −2; right STG: 62, −2, and −6) and five ROIs for non-verbal interactions (peak voxels: left fusiform gyrus: −32, −62, and −18; right fusiform gyrus: 30, −62, and −16; left calcarine gyrus: −10, −90, and 4; right calcarine gyrus: 14, −68, and 8; right IFG PTr: 54, 24, and 32). The Neurosynth-mask did not cover the cluster detected in the right MFG. In each of the remaining ROIs, the significant differences between the non-verbal conditions as well as the larger activation for non-habitual interactions were confirmed (all p < 0.001; Supplementary Figure S1). Thus, the functional responses obtained during watching naturalistic stimulation were in accordance with meta-analytical maps for verbal stimulation and social interactions. The widespread activation of cortical regions during the processing of non-verbal interactions was grounded in the processing of non-habitual interactions.
Brain Regions Underlying UCs in Non-verbal Interactions
We further explored brain regions that engaged differentially in the encoding of the semiotic categories of the observed non-verbal social interactions. Thereto, we conducted mappings of the differences between the three conditions with an F-test and post hoc t-tests. The F-test yielded an extended pattern of brain regions with the highest activations in the fusiform gyrus and middle occipital gyrus (MOG; see Figure 4A and Table 1), similar to those obtained for the contrast between non-verbal and verbal interactions (compare with Figure 3B). Of the six directed comparisons in the post hoc t-tests, four yielded significant clusters at a threshold according to a pFWE < 0.05 (Figure 4B and Table 3). First, specific contributions of both other types – anticipation and non-habituality – emerged when contrasted to habitual interactions: Neural responses were increased in the left STG and TPJ during unresolved interactions (right upper panel in Figure 4B) and in the right fusiform gyrus and bilateral TPJ during non-habitual interactions (left upper panel in Figure 4B). Using the unresolved condition as baseline, habitual interactions yielded higher activation in the left parahippocampal gyrus and bilateral MOG (right lower panel in Figure 4B), and non-habitual interactions elicited higher activation in the right IFG as well as bilateral fusiform gyrus, TPJ, and MOG (left lower panel in Figure 4B). The contrasts “unresolved > non-habitual” and “habitual > non-habitual” did not yield differences at the same threshold. After correction of the contrasts with the Neurosynth-based mask, each of the detected clusters fell – at least partly – into the brain mask (Supplementary Figure S2 and Supplementary Table S1). Involvement of the reported brain regions in processing of verbal information and social interaction was thereby validated based on this meta-analytical approach.
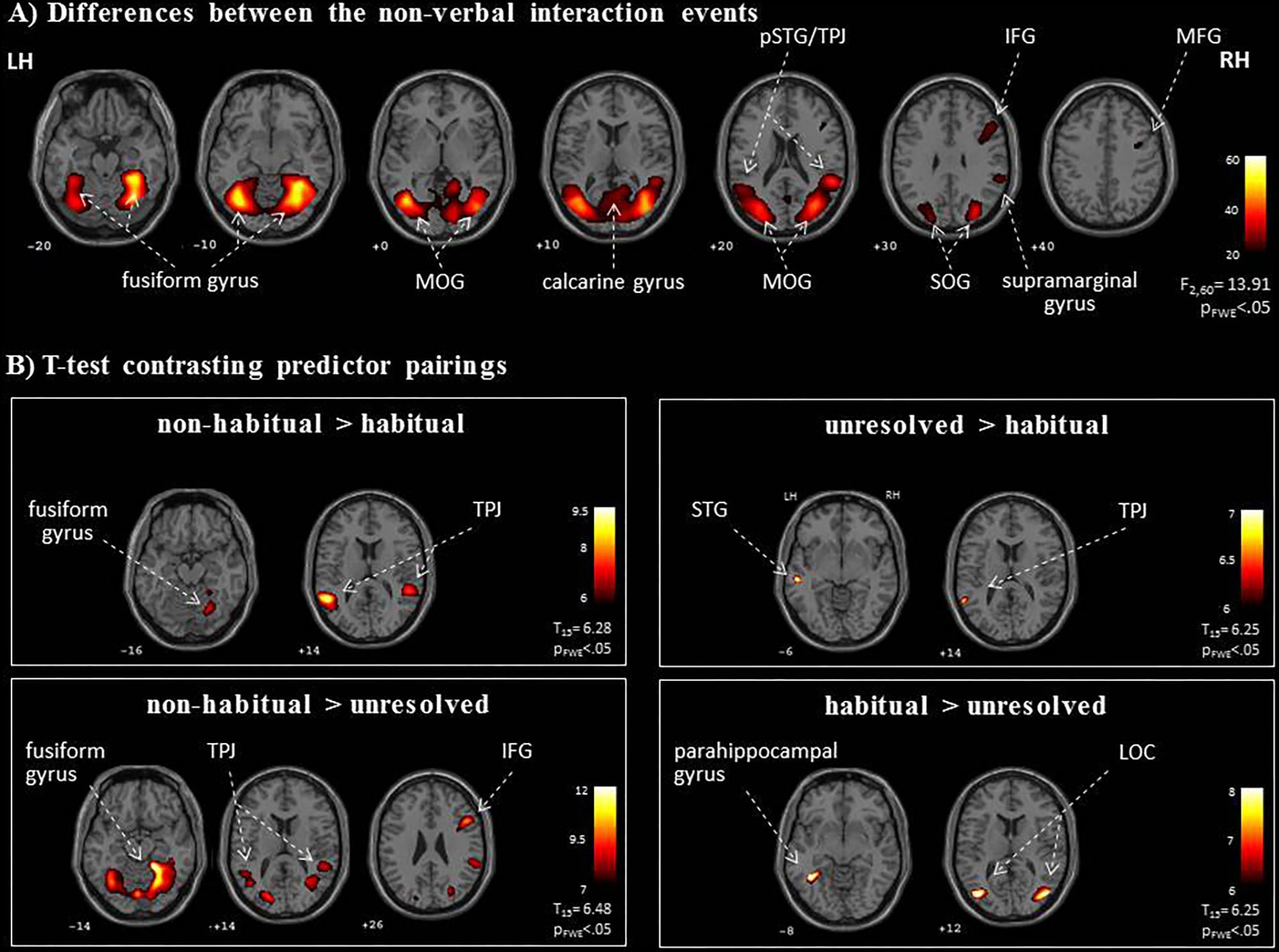
FIGURE 4. Neural networks for the processing of non-verbal interactions. (A) The specific F-test differentiated processing networks for the semiotic categories (“unresolved,” “non-habitual,” and “habitual”). (B) Post hoc t-maps determined specific effects in four directed contrasts. As an extension to the ROI analysis, the TPJ may be relevant for the interpretation of unresolved or non-habitual interactions, whereas the hippocampus and the LOC responded most strongly to habitual interactions. LH, left hemisphere; RH, right hemisphere; IFG, inferior frontal gyrus; LOC, lateral occipital complex; pSTG, posterior superior temporal gyrus; MFG, middle frontal gyrus; MOG, middle occipital gyrus; SOG, superior occipital gyrus; TPJ, temporo-parietal junction; z-coordinates are indicated beneath each slice.
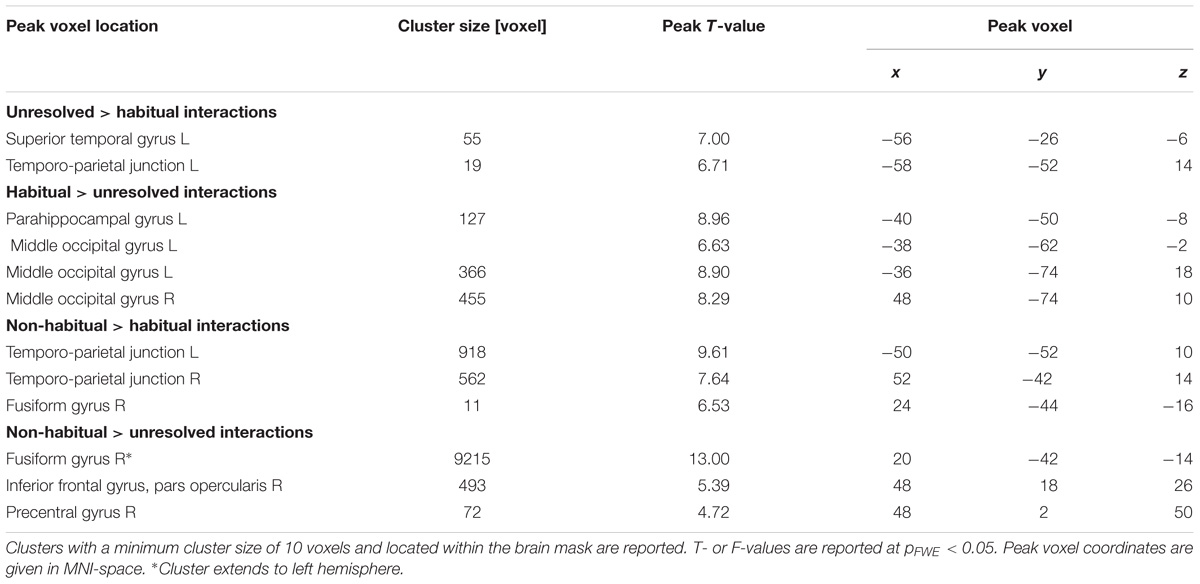
TABLE 3. Cluster table for comparison between non-verbal interaction types (Figure 4).
Discussion
We identified types of non-verbal social interactions in a movie narrative based on a coding scheme derived from the semiotic framework of Peirce’s UCs and segregated neural processes during the observation of such interactions. Three non-verbal interaction types were determined: unresolved (predominant Firstness Category), non-habitual (Secondness), and habitual (Thirdness). These non-verbal interactions together with the verbal ones yielded activations in brain structures involved during naturalistic viewing conditions. The non-verbal interactions yielded lower auditory activation than verbal ones, but significant effects of type emerged: Unresolved events primed auditory cortex, whereas habitual ones suppressed it. Increased activity after non-verbal compared to verbal interactions in the visual cortex and right lateral PFC was due to non-habitual interactions. Further exploration across interaction types revealed that non-habitual interactions yielded higher TPJ activations than unresolved and, most markedly, habitual interactions. The latter recruited the parahippocampal gyrus and lateral occipital complex (LOC) more than unresolved interactions. In summary, Peirce’s cognitive-semiotic categories distinguished three modes for interpreting social interactions. Their underlying cognitive mechanisms led to neural representations of perception and interpretation during the observation of an ongoing film sequence.
Neural Responses to Social Interactions
Widespread brain regions encompassing visual, auditory, and object-processing areas responded to social interactions shown in a movie. Similar patterns of activation have been observed when contrasting social versus non-social contents depicted in photographs (Deuse et al., 2016) and movie clips (Iacoboni et al., 2004; Wolf et al., 2010; Lahnakoski et al., 2012). In particular, the reported brain structures comprised bilateral fusiform cortex, superior temporal cortex extending into the TPJ, right inferior frontal cortex, and dorsomedial PFC. Such pattern is readily expected from sensory and perceptual processing, but lacks involvement of the language networks (i.e., left IFG “Broca” and pSTG “Wernicke”). Similarly, under naturalistic stimulation conditions in previous studies not explicitly investigating language processing, language network responded weakly only (Malinen et al., 2007; Jääskeläinen et al., 2008; Wilson et al., 2008). The weak recruitment of prefrontal structures in our study (not surpassing the corrected threshold) may be explained by the lack of an explicit task aiming at social cognition (Nummenmaa and Calder, 2009; Nardo et al., 2016). Indeed, frontal-cortical activation was found to be variable during film clips (Hasson, 2004; Kauppi et al., 2017). Taken together, the content-coded social interactions yielded activation patterns in established networks for perceptual processes and social cognition, yielding validity to our event related coding of social interactions during naturalistic stimulation.
Neuronal Correlates of Interactions Types
The contrast between non-verbal and verbal interactions separated networks for auditory and visual processing. As expected, the bilateral STG responded most strongly to verbal interactions, whereas non-verbal interactions activated visual pathways. The latter finding suggests that during verbal stimulation visual cues are less processed (Wolf et al., 2014). In addition to this clear pattern, the non-verbal, non-auditory interactions yielded a cross-modal modulation in the bilateral STG extending into the MTG. Both the ROI analysis and the comparative mapping across interaction types revealed that STG recruitment was increased for unresolved interactions and was decreased for habitual interactions. Attempts to disambiguate unresolved, socially relevant interactions may prime temporal auditory areas to seek additional auditory cues. In a similar vein, during an audio-visual emotion judgment task, the influence of one modality was greater when the other modality provided ambiguous information (Massaro and Egan, 1996). This effect is thought to be based on cross-modal modulation of basic perception. For instance, magnetoencephalographic studies reported anticipatory pre-activation of auditory cortex by visual motion cues (Hertrich et al., 2007) and modulatory effects of predictability in an audio-visual apparent motion task (Zvyagintsev et al., 2008). The cross-modal priming effect also enhances processing of communication and interaction. A modulation of superior temporal regions supporting auditory processing has been reported for changes in perceived communicative intent (Biau et al., 2016), contextual embedding of speech (Skipper, 2014), stimulus familiarity (Hein et al., 2007), and perceived intentionality of actions (Pelphrey et al., 2004). Taken together, the STG and MTG were sensitive to interpretation processes for communicative actions and interactions. Thus, in the absence of speech, ambiguous visual information (unresolved social interactions, Firstness category) increased neural involvement in processing anticipated speech and behavior.
Non-verbal interactions recruited visual areas and the right lateral PFC more than verbal interactions. This activation was entirely due to responses to non-habitual interactions (Secondness category) and not to the unresolved or habitual ones. It seems that observing non-habitual interactions required enhanced visual processing. Implication of the right lateral PFC regions indicates that the processing focus may be particularly on the contextualization of action and movement patterns. There is evidence that both the right IFG and MFG are involved in the representation of goal-directed aspects of actions (Koski et al., 2002) and in the interpretation of actions in social contexts (Decety and Sommerville, 2003). Indeed, the lateral PFC supports learning and employing rule knowledge relevant to actions (for a review, see Bunge, 2004). More specifically, the right PFC contributes to the creation and testing of rules (Bunge et al., 2003; Donohue et al., 2005), particularly when these need to be integrated into contextual information (Lenartowicz et al., 2010; Waskom et al., 2014). In previous research, the involvement of the lateral PFC during the learning and application of rules has been implicated in both concrete experiential tasks and the formation of abstract behavior-independent rules (Badre et al., 2010; Waskom et al., 2014), and, further, to patterns of appropriate behavior within a given social context (Burgess et al., 2000; Goel et al., 2004; Bolling et al., 2011). In the same vein, the right IFG was related to contextual integration involved in the comprehension of unconventional communicative object configurations (Tylén et al., 2009). Our finding that observing non-habitual interactions recruits right IFG/MFG contributes to this line of research. When encountering a behavior which discords with context-based expectations, new hypotheses of social rules and intentions need to be formulated; this process may recruit right IFG/MFG. This view is in agreement with the conceptualizing of Secondness by Peirce, where the interpreter contextualizes signs and has not yet formed rules (Peirce, 1960).
In non-habitual interactions the observed agent did not act in accordance with socially accepted behavior and, therefore, displayed unexpected behaviors that contrasted with the observer’s predictions (Lee and McCarthy, 2015). This may have led, first, to an allocation of attention toward the agent’s actions (Vossel et al., 2014) as well as toward the reaction of the interacting partner, and, second, to a re-evaluation and remodeling of the agent’s intentions and motivations (Pelphrey et al., 2004; Saxe et al., 2004). When directly contrasting non-habitual with habitual interactions and with unresolved interactions, we detected particularly strong bilateral TPJ activation. A susceptibility of TPJ to other people’s intentions was demonstrated for the right TPJ in response to observing incorrect goal-oriented hand/arm movements (Pelphrey et al., 2004), observing motoric and social errors (Jääskeläinen et al., 2016), and intentional whole body action (Saxe et al., 2004). These findings indicate enhanced recruitment of TPJ during the evaluation and modeling of an observed agent’s intentions.
An alternative explanation for increased activation in visual processing areas, TPJ, and right frontal cortex may reside in the increased saliency of unexpected behaviors. Although expectation (stimulus is expected versus unexpected) and attention (stimulus is relevant versus irrelevant) are dissociable mechanisms in the visual system (Summerfield and Egner, 2009), both may have interacted during the observation of non-habitual interactions. Increased activation in the right TPJ and the IFG has been found for experimentally induced competition between several salient visual events during free viewing of movie clips (Nardo et al., 2016). However, the scenes depicted single or interacting people and thus were social in nature as well. Furthermore, a sharp differentiation between salience-induced attentional processes and mentalizing processes (as part of Theory of Mind) may be inconsequential during social cognition. Both processes can be understood in terms of contextual updating, which is a suggested key function of TPJ (Geng and Vossel, 2013). Therefore, during observation of socially relevant scenes, attentional processes may help evaluate the appropriateness of the observed actions and model possible motives and reactions of the interacting partner.
Habitual Non-verbal Interactions
The contrasts between verbal and non-verbal interactions have elucidated the distinct contributions of unresolved and non-habitual interactions. Further, specific effects for habitual interactions (Thirdness category) emerged in the direct contrasts between non-verbal interaction types. The bilateral LOC and the left parahippocampal gyrus were activated more in response to habitual than to unresolved interactions. Habitual – and thus rule-conforming – actions are well-learned and regularly encountered in everyday life. Therefore, visual scene analysis and matching input to memory representations may dominate the interpretation process. The LOC is traditionally associated with processing and recognizing faces, body parts, and goal-directed movements as well as general motion patterns (for a review, see Lingnau and Downing, 2015). The parahippocampal gyrus contributes to associative memory and visuo-spatial processing (for a review, see Aminoff et al., 2013). Therefore, the LOC-parahippocampal network may represent real-world scenes complementing the perception of photographs (Park et al., 2011) and movie clips (Lahnakoski et al., 2014). Interpreting interactions as habitual and conforming to social rules seems to be associated with processing in object-recognition and memory structures.
Habitual interactions are not only well-learned but also conform to schematic behavioral patterns and social rules. The LOC-parahippocampal network may additionally contribute to the extraction, recognition, and contextualization of behavioral patterns. Involvement of the LOC has been reported for contextual guidance during visual search in complex scenes (Lin and Scott, 2011), the creation of category-level templates for recognizing humans and objects (van Koningsbruggen et al., 2013), and the abstraction of actions from agents (Kable and Chatterjee, 2006). Similarly, increased activation in the parahippocampal gyrus has been reported both for goal-oriented actions (Lahnakoski et al., 2012) and for more abstract functions of scene analysis such as scene categories (Peelen and Kastner, 2014), locational concepts (Huth et al., 2016), and perceptual schema representations (Bar, 2009). These processes may contribute to the understanding of action schemas, which are, on a mechanistic level, the basis of habitual social interactions. Thus, our results indicate that during the observation of habitual interactions, the parahippocampal gyrus and the LOC interact to evaluate the observed action patterns.
Universal Categories and Neural Activation Patterns
The interpretation of social interactions requires synergy of various cognitive functions raging from multimodal perception and social attention to language comprehension and mentalizing (Ochsner and Lieberman, 2001; Adolphs, 2009; Hari and Kujala, 2009). As novel means to a comprehensive typology, Peirce’s semiotic framework of UCs was utilized to inform three types of social interactions. These interaction types were presented in a movie context and yielded distinct neural patterns. The Firstness category is predominant in unresolved social interactions, which recruited bilateral STG. Conceivably, in ambiguous social situations, attention was directed toward the auditory modality (e.g., Massaro and Egan, 1996), as is predicted by the “principle of inverse effectiveness” (Stein and Meredith, 1993). The perception of a stimulus can be altered by a cue from another modality (Klasen et al., 2012a); in particular, audiovisual stimuli of lower intensity yield larger recognition benefit that those with higher intensity (Diederich and Colonius, 2004; Rach et al., 2011). During unresolved interactions, when information density of the visual modality was reduced, auditory processing increased. Spoken language is highly codified and thus effectively resolves a situation.
Social interactions of the Secondness category (non-habitual) required the highest processing demand in visuo-spatial, mentalizing, and contextual rule-learning areas (IFG). This pattern suggests increased attention toward the unexpected behavior and a re-evaluation of the observed agent’s intentions within the situational context (Lee and McCarthy, 2015). This mismatch between prior expectations and reality triggers a cognitive prediction error, which has been associated particularly with dorsolateral PFC (Fletcher et al., 2001; Turner, 2004). Prediction error processing elicits attentional orienting and underlies mentalizing and contextual learning (den Ouden et al., 2012).
The observation of habitual interactions (Thirdness category) recruited brain regions supporting conceptual processing and associative memory retrieval. Habitual behaviors may be encoded as learned action patterns and social schemas (Bolling et al., 2011), Social schemas are memory representations of typical contexts (Spalding et al., 2015), which guide and facilitate the processing of social information (Augoustinos and Innes, 1990) and their reconstruction from memory (Bartlett, 1932); the latter involves the ventromedial PFC regions (Spalding et al., 2015) rather than lateral PFC.
Taken together, characterizing non-verbal social interactions with Peirce’s cognitive-semiotic categories enabled the holistic neurosemiotic investigation of complex social cognition. Such a semiotic approach offers a novel means to investigate the neural representation of communication in naturalistic stimuli.
Building on Peirce’s semiotic theory, various theoretical frameworks highlight specific aspects of communicative signs. For instance, the UCs have been instrumental to empirical analyses of manual gestures (McNeill, 1992, 2005; Fricke, 2007; Mittelberg, 2008, 2013, 2018; Mittelberg and Waugh, 2014). McNeill’s Peirce-inspired differentiation of gestures into iconic gestures (icon, Firstness category), deictic gestures (index, Secondness category), and emblems (symbol, Thirdness category; McNeill, 1992, 2005) has become a prominent strand within gesture research. Neuroimaging studies on gesture perception and comprehension revealed that manual gestures in general recruited the language systems (IFG and posterior superior temporal sulcus) and the action-movement systems (inferior parietal and premotor cortex; Andric and Small, 2012) whereas iconic gestures, representing salient visual features of an object referred to in speech, activated a fronto- posterior temporal network (Özyürek, 2014). Furthermore, emblems, being symbolic and highly conventionalized signs, recruited the language networks (Bernardis and Gentilucci, 2006; Xu et al., 2009).
Symbolic and conventionalized meaning corresponds to Thirdness in Peirce’s UCs (Peirce, 1960; Potter, 1967; Bateman et al., 2017) and is considered in neurocognitive investigations not only for gesture comprehension (Villarreal et al., 2012; Andric et al., 2013) but also for the perception of communicative signs in a broader sense (Donohue et al., 2005; Sato et al., 2009; Pulvermüller, 2013). For instance, pictures of objects activated the language network (like words) if they were perceived as symbolic (Tylén et al., 2009) or when they conveyed abstract social meaning (Tylén et al., 2016). Furthermore, Peirce’s UCs have inspired theories regarding the emergence of social conventions and symbolic communication during language evolution (Deacon, 1997) and during child development (Daddesio, 1994). These examples emphasize the applicability of Peirce’s semiotics for the investigation of communicative signs and behaviors with respect to various signal properties. However, since empirical studies are still scarce and the operationalizations vary across studies, the picture is yet incoherent and impedes generalizations (Fusaroli and Paolucci, 2011; Zlatev, 2012). The application of UCs has a high potential with regards to investigating communication processes and should be further explored in the context of social learning or social impairments such as seen in patients with schizophrenia or autism spectrum disorders.
Methodological Considerations and Limitations
In order to relate functional responses to the social interaction types we conducted a GLM analysis and corrected the resulting brain maps with voxelwise FWE correction (pFWE < 0.05). To minimize spurious activation voxels, only cluster with size according to a cluster-wise FWE correction are reported. Furthermore, localizations were confirmed with a Neurosynth-based a priori mask, with one exception. The cluster in right MFG for non-verbal interactions over verbal interaction failed to overlap with the mask and, thus, may constitute a false positive result and needs to be confirmed in follow up studies.
The unequal distribution of events and event-durations introduces differences in the amount of observations. This heterogeneity may cause a violation of the heteroscedasticity assumption for analyses of variance. Therefore, the model estimation was done with assuming unequal-variance as provided by SPM. Thus we minimized the statistical bias due to the violation of the heteroscedasticity assumption of the regressors. The imbalance of event numbers and durations constitutes a limitation of the analysis, but also reflects the nature of naturalistic stimulation. Naturalistic stimuli such as movie clips are inherently complex and contain a multitude of diverse, dynamic, and interacting information. Although natural viewing conditions offer superior ecological validity compared to more traditional experimental paradigms, the stimulus conditions are less well controlled (Hasson, 2004). The number of events and the total duration vary across the coded interaction types. With our content-coding based GLM approach, we aimed to model the neural responses to social interaction types by generalizing across movie scenes and, therefore, by and large independently of other movie contents. However, since the presentation of events is not controlled or randomized, the stimuli may differ in complexity and may coincide with other influencing factors or physical characteristics. Nevertheless, the congruent neural patterns to other studies investigating social interactions lend credibility to this pseudo-experimental design, as shown in previous studies employing similar content-coding methodology (e.g., Mathiak and Weber, 2006).
The movie “Lola rennt” comprised a large variety of social situations and scenes as well as different cinematographic elements and thus may be considered a comprehensive naturalistic stimulus; nevertheless, the activation patterns may be stimulus-specific and may not generalize to other movie-excerpts. Until now, movie excerpts were mainly analyzed with data driven methods such as ISC and independent-component analysis (ICA), instead of a model-based approach. Therefore, additional studies using model-based analyses need to determine stimulus-independence and generalizability of the here presented activation patterns.
Peirce’s pragmatic approach to communication processes makes his theory well suited for systematic analyses (Mittelberg, 2008, 2018; Bateman et al., 2017). Our content-coding based design is a theory-driven approach to iteratively obtain a meaningful typology of non-verbal social interactions (Weber et al., 2009). The coding results show a good reliability. Validity is, on the one hand, established by the theoretical foundation and, on the other hand, confirmed by the meaningful neural contributions (Pajula et al., 2012). The approach based on semiotic categories facilitates neurocognitive analysis; however, additional non-categorical, continuous measures may reflect interaction-related features in more detail. Further refinements of the operationalization can target not only the validity of the coding but may employ the UCs to also describe other processes that underlie the perception and comprehension of various kinds of signs.
Conclusion
We operationalized Peirce’s semiotic typology to describe basic social cognitive categories of non-verbal interactions in a movie narrative. Functional imaging revealed specific and meaningful responses in the brain to the observed events. Firstness: During the observation of unresolved interactions, the ambiguous visual information enhanced neural involvement in bilateral STG even in the absence of speech – conceivably as a cognitive mechanism to attend to additional resolving cues in another modality. Secondness: In response to non-habitual interactions that contrasted contextual expectations, the visual and prefrontal cortices as well as the TPJ supported the interpretation of intentions and the re-evaluation of social rules. Thirdness: The interpretation of habitual interactions recruited neural correlates for object recognition and associative memory. Semiotic approaches may help to elucidate mechanisms of social communication beyond confined linguistic theories.
Author Contributions
DW have designed, tested and applied the content coding scheme, analyzed and interpreted the data, and wrote the manuscript. IM have applied the semiotic theory on content coding scheme and edited the manuscript. L-MR have applied the semiotic theory on content coding scheme and edited the manuscript. SB have planned the study and collected the data. MZ have implemented the fMRI measurement details and assisted in data collection. AH have designed, tested, and applied the content coding scheme. MK have assisted in designing the content coding scheme and edited the manuscript. FC have counselled and assisted in data analysis. KM have planned the study, assisted in data analysis and interpretation, and edited the manuscript.
Funding
This study was funded by the German Research Foundation (DFG IRTG 2150, MA 2631/6-1); the German Ministry for Education and Research (BMBF; APIC: 01EE1405A, 01EE1405B, and 01EE1405C); and the Interdisciplinary Center for Clinical Research (IZKF) of the University Hospital Aachen.
Conflict of Interest Statement
The authors declare that the research was conducted in the absence of any commercial or financial relationships that could be construed as a potential conflict of interest.
Acknowledgments
The authors would like to thank Dr. Maxim Zaitsev for providing the single-shot multi-echo EPI sequence (Multi-Echo EPI C2P package, version 4.2), Cordula Kemper for assistance with measurements, and the Brain Imaging Facility of the IZKF, RWTH Aachen University, for technical support.
Supplementary Material
The Supplementary Material for this article can be found online at: https://www.frontiersin.org/articles/10.3389/fnhum.2018.00296/full#supplementary-material
Footnotes
References
Adolphs, R. (2009). The social brain: neural basis of social knowledge. Annu. Rev. Psychol. 60, 693–716. doi: 10.1146/annurev.psych.60.110707.163514
Aminoff, E. M., Kveraga, K., and Bar, M. (2013). The role of the parahippocampal cortex in cognition. Trends Cogn. Sci. 17, 379–390. doi: 10.1016/j.tics.2013.06.009
Andric, M., and Small, S. L. (2012). Gesture’s neural language. Front. Psychol. 3:99. doi: 10.3389/fpsyg.2012.00099
Andric, M., Solodkin, A., Buccino, G., Goldin-Meadow, S., Rizzolatti, G., and Small, S. L. (2013). Brain function overlaps when people observe emblems, speech, and grasping. Neuropsychologia 51, 1619–1629. doi: 10.1016/j.neuropsychologia.2013.03.022
Augoustinos, M., and Innes, J. M. (1990). Towards an integration of social representations and social schema theory. Br. J. Soc. Psychol. 29, 213–231. doi: 10.1111/j.2044-8309.1990.tb00901.x
Badre, D., Kayser, A. S., and D’Esposito, M. (2010). Frontal cortex and the discovery of abstract action rules. Neuron 66, 315–326. doi: 10.1016/j.neuron.2010.03.025
Bar, M. (2009). The proactive brain: memory for predictions. Philos. Trans. R. Soc. B Biol. Sci. 364, 1235–1243. doi: 10.1098/rstb.2008.0310
Bartlett, F. C. (1932). Remembering: A Study in Experimental and Social Psychology. Cambridge: Cambridge University Press.
Bateman, J., Wildfeuer, J., and Hiippala, T. (2017). Multimodality: Foundations, Research and Analysis - A Problem-Oriented Introduction. Berlin: de Gruyter. doi: 10.1515/9783110479898
Becchio, C., Manera, V., Sartori, L., Cavallo, A., and Castiello, U. (2012). Grasping intentions: from thought experiments to empirical evidence. Front. Hum. Neurosci. 6:117. doi: 10.3389/fnhum.2012.00117
Bernardis, P., and Gentilucci, M. (2006). Speech and gesture share the same communication system. Neuropsychologia 44, 178–190. doi: 10.1016/j.neuropsychologia.2005.05.007
Bhavsar, S., Zvyagintsev, M., and Mathiak, K. (2014). BOLD sensitivity and SNR characteristics of parallel imaging-accelerated single-shot multi-echo EPI for fMRI. Neuroimage 84, 65–75. doi: 10.1016/j.neuroimage.2013.08.007
Biau, E., Fernandez, L. M., Holle, H., Avila, C., and Soto-Faraco, S. (2016). Hand gestures as visual prosody: BOLD responses to audio-visual alignment are modulated by the communicative nature of the stimuli. Neuroimage 132, 129–137. doi: 10.1016/j.neuroimage.2016.02.018
Bolling, D. Z., Pitskel, N. B., Deen, B., Crowley, M. J., McPartland, J. C., Mayes, L. C., et al. (2011). Dissociable brain mechanisms for processing social exclusion and rule violation. Neuroimage 54, 2462–2471. doi: 10.1016/j.neuroimage.2010.10.049
Bunge, S. A. (2004). How we use rules to select actions: a review of evidence from cognitive neuroscience. Cogn. Affect. Behav. Neurosci. 4, 564–579. doi: 10.3758/CABN.4.4.564
Bunge, S. A., Kahn, I., Wallis, J., Miller, E. K., and Wagner, A. D. (2003). Neural circuits subserving the retrieval and maintenance of abstract rules. J. Neurophysiol. 90, 3419–3428. doi: 10.1152/jn.00910.2002
Burgess, P. W., Veitch, E., De Lacy Costello, A., and Shallice, T. (2000). The cognitive and neuroanatomical correlates of multitasking. Neuropsychologia 38, 848–863. doi: 10.1016/S0028-3932(99)00134-7
Daddesio, T. C. (1994). On Minds and Symbols. The Relevance of Cognitive Science for Semiotics. Berlin: Mouton de Gruyter.
Deacon, T. W. (1997). The Symbolic Species: The Co-Evolution of Language and the Brain. New York, NY: W.W. Norton & Company.
Decety, J., and Sommerville, J. A. (2003). Shared representations between self and other: a social cognitive neuroscience view. Trends Cogn. Sci. 7, 527–533. doi: 10.1016/j.tics.2003.10.004
Deleuze, G. (1986). Cinema1: The Movement-Image, 1st Edn. Minneapolis, MN: University of Minnesota Press.
den Ouden, H. E. M., Kok, P., and de Lange, F. P. (2012). How prediction errors shape perception, attention, and motivation. Front. Psychol. 3:548. doi: 10.3389/fpsyg.2012.00548
Deuse, L., Rademacher, L. M., Winkler, L., Schultz, R. T., Gründer, G., and Lammertz, S. E. (2016). Neural correlates of naturalistic social cognition: brain-behavior relationships in healthy adults. Soc. Cogn. Affect. Neurosci. 11, 1741–1751. doi: 10.1093/scan/nsw094
Diederich, A., and Colonius, H. (2004). Bimodal and trimodal multisensory enhancement: effects of stimulus onset and intensity on reaction time. Percept. Psychophys. 66, 1388–1404. doi: 10.3758/BF03195006
Donohue, S. E., Wendelken, C., Crone, E. A., and Bunge, S. A. (2005). Retrieving rules for behavior from long-term memory. Neuroimage 26, 1140–1149. doi: 10.1016/j.neuroimage.2005.03.019
Evans, A. C., Collins, D. L., Mills, S. R., Brown, E. D., Kelly, R. L., and Peters, T. M. (1993). “3D statistical neuroanatomical models from 305 MRI volumes,” in Proceedings of the 1993 IEEE Conference Record Nuclear Science Symposium and Medical Imaging Conference (IEEE), (San Francisco, CA: IEEE), 1813–1817. doi: 10.1109/NSSMIC.1993.373602
Fletcher, P. C., Anderson, J. M., Shanks, D. R., Honey, R., Carpenter, T. A., Donovan, T., et al. (2001). Responses of human frontal cortex to surprising events are predicted by formal associative learning theory. Nat. Neurosci. 4, 1043–1048. doi: 10.1038/nn733
Fricke, E. (2007). Origo, Geste und Raum. Lokaldeixis im Deutschen. Berlin: de Gruyter: Mouton. doi: 10.1515/9783110897746
Friston, K. J., Worsley, K. J., Frackowiak, R. S., Mazziotta, J. C., and Evans, A. C. (1994). Assessing the significance of focal activations using their spatial extent. Hum. Brain Mapp. 1, 210–220. doi: 10.1002/hbm.460010306
Galantucci, B., Garrod, S., and Roberts, G. (2012). Experimental semiotics. Lang. Linguist. Compass 6, 477–493. doi: 10.1002/lnc3.351
Geng, J. J., and Vossel, S. (2013). Re-evaluating the role of TPJ in attentional control: Contextual updating? Neurosci. Biobehav. Rev. 37, 2608–2620. doi: 10.1016/j.neubiorev.2013.08.010
Goel, V., Shuren, J., Sheesley, L., and Grafman, J. (2004). Asymmetrical involvement of frontal lobes in social reasoning. Brain 127, 783–790. doi: 10.1093/brain/awh086
Guynes, S. A. (2013). Four-color sound: a Peircean semiotics of comic book onomatopoeia. Public J. Semiot. 6, 58–72.
Hari, R., and Kujala, M. V. (2009). Brain basis of human social interaction: from concepts to brain imaging. Physiol. Rev. 89, 453–479. doi: 10.1152/physrev.00041.2007
Hasson, U. (2004). Intersubject synchronization of cortical activity during natural vision. Science 303, 1634–1640. doi: 10.1126/science.1089506
Hasson, U., and Honey, C. J. (2012). Future trends in neuroimaging: neural processes as expressed within real-life contexts. Neuroimage 62, 1272–1278. doi: 10.1016/j.neuroimage.2012.02.004
Hein, G., Doehrmann, O., Muller, N. G., Kaiser, J., Muckli, L., and Naumer, M. J. (2007). Object familiarity and semantic congruency modulate responses in cortical audiovisual integration areas. J. Neurosci. 27, 7881–7887. doi: 10.1523/JNEUROSCI.1740-07.2007
Hertrich, I., Mathiak, K., Lutzenberger, W., Menning, H., and Ackermann, H. (2007). Sequential audiovisual interactions during speech perception: a whole-head MEG study. Neuropsychologia 45, 1342–1354. doi: 10.1016/j.neuropsychologia.2006.09.019
Holenstein, E. (2008). Semiotics as a cognitive science. Cogn. Semiot. 2008, 6–19. doi: 10.3726/81606_6
Huth, A. G., Lee, T., Nishimoto, S., Bilenko, N. Y., Vu, A. T., and Gallant, J. L. (2016). Decoding the semantic content of natural movies from human brain activity. Front. Syst. Neurosci. 10:81. doi: 10.3389/fnsys.2016.00081
Iacoboni, M., Lieberman, M. D., Knowlton, B. J., Molnar-Szakacs, I., Moritz, M., Throop, C. J., et al. (2004). Watching social interactions produces dorsomedial prefrontal and medial parietal BOLD fMRI signal increases compared to a resting baseline. Neuroimage 21, 1167–1173. doi: 10.1016/j.neuroimage.2003.11.013
Jääskeläinen, I. P., Halme, H., Agam, Y., Glerean, E., Lahnakoski, J. M., Sams, M., et al. (2016). Neural mechanisms supporting evaluation of others’ errors in real-life like conditions. Sci. Rep. 6:18714. doi: 10.1038/srep18714
Jääskeläinen, I. P., Koskentalo, K., Balk, M. H., Autti, T., Kauramäki, J., Pomren, C., et al. (2008). Inter-subject synchronization of prefrontal cortex hemodynamic activity during natural viewing. Open Neuroimag. J. 2, 14–19. doi: 10.2174/1874440000802010014
Jakobson, R. (1966). “Quest for the essence of langauge,” in Roman Jakobson. On Language, eds L. Waugh and M. Monville-Burston (Cambridge: MA: Harvard University Press), 407–421.
Kable, J. W., and Chatterjee, A. (2006). Specificity of action representations in the lateral occipitotemporal cortex. J. Cogn. Neurosci. 18, 1498–1517. doi: 10.1162/jocn.2006.18.9.1498
Kauppi, J. P., Pajula, J., Niemi, J., Hari, R., and Tohka, J. (2017). Functional brain segmentation using inter-subject correlation in fMRI. Hum. Brain Mapp. 38, 2643–2665. doi: 10.1002/hbm.23549
Klasen, M., Chen, Y.-H., and Mathiak, K. (2012a). Multisensory emotions: perception, combination and underlying neural processes. Rev. Neurosci. 23, 381–392. doi: 10.1515/revneuro-2012-0040
Klasen, M., Weber, R., Kircher, T. T. J., Mathiak, K. A., and Mathiak, K. (2012b). Neural contributions to flow experience during video game playing. Soc. Cogn. Affect. Neurosci. 7, 485–495. doi: 10.1093/scan/nsr021
Koski, L., Wohlschläger, A., Bekkering, H., Woods, R. P., Dubeau, M.-C., Mazziotta, J. C., et al. (2002). Modulation of motor and premotor activity during imitation of target-directed actions. Cereb. Cortex 12, 847–855. doi: 10.1093/cercor/12.8.847
Krall, S. C., Rottschy, C., Oberwelland, E., Bzdok, D., Fox, P. T., Eickhoff, S. B., et al. (2015). The role of the right temporoparietal junction in attention and social interaction as revealed by ALE meta-analysis. Brain Struct. Funct. 220, 587–604. doi: 10.1007/s00429-014-0803-z
Lahnakoski, J. M., Glerean, E., Jääskeläinen, I. P., Hyönä, J., Hari, R., Sams, M., et al. (2014). Synchronous brain activity across individuals underlies shared psychological perspectives. Neuroimage 100, 316–324. doi: 10.1016/j.neuroimage.2014.06.022
Lahnakoski, J. M., Glerean, E., Salmi, J., Jääskeläinen, I. P., Sams, M., Hari, R., et al. (2012). Naturalistic FMRI mapping reveals superior temporal sulcus as the hub for the distributed brain network for social perception. Front. Hum. Neurosci. 6:233. doi: 10.3389/fnhum.2012.00233
Lee, S. M., and McCarthy, G. (2015). Neural regions discriminating contextual information as conveyed through the learned preferences of others. Front. Hum. Neurosci. 9:492. doi: 10.3389/fnhum.2015.00492
Lee, Y. (2012). “Narrative communication: a Peircean approach,” in Proceedings of the 10th World Congress of the International Association for Semiotic Studies (IASS/AIS), (A Coruña: Universidade da Coruna), 1383–1394.
Lenartowicz, A., Escobedo-quiroz, R., and Cohen, J. D. (2010). Updating of context in working memory: an event related potential study. Cogn. Affect. Behav. Neurosci 10, 298–315. doi: 10.3758/CABN.10.2.298.Updating
Lin, G. G.-H., and Scott, J. G. (2011). Investigations of the constitutive overexpression of CYP6D1 in the permethrin resistant LPR strain of house fly (Musca domestica). Pestic. Biochem. Physiol. 100, 130–134. doi: 10.1016/j.pestbp.2011.02.012
Lingnau, A., and Downing, P. E. (2015). The lateral occipitotemporal cortex in action. Trends Cogn. Sci. 19, 268–277. doi: 10.1016/j.tics.2015.03.006
Lotze, M., Heymans, U., Birbaumer, N., Veit, R., Erb, M., Flor, H., et al. (2006). Differential cerebral activation during observation of expressive gestures and motor acts. Neuropsychologia 44, 1787–1795. doi: 10.1016/j.neuropsychologia.2006.03.016
Magnussen, A. (2000). “The semiotics of C.S. Peirce as a theoretical framework for the understanding of comics,” in Comics and Culture: Analytical and Theoretical Approaches to Comics, eds A. Magnussen and H.-C. Christiansen (Copenhagen: Museum of Tusculanum Press), 193–207.
Malinen, S., Hlushchuk, Y., and Hari, R. (2007). Towards natural stimulation in fMRI–issues of data analysis. Neuroimage 35, 131–139. doi: 10.1016/j.neuroimage.2006.11.015
Massaro, D. W., and Egan, P. B. (1996). Perceiving affect from the voice and the face. Psychon. Bull. Rev. 3, 215–221. doi: 10.3758/BF03212421
Mathiak, K., Hertrich, I., Grodd, W., and Ackermann, H. (2004). Discrimination of temporal information at the cerebellum: functional magnetic resonance imaging of nonverbal auditory memory. Neuroimage 21, 154–162. doi: 10.1016/j.neuroimage.2003.09.036
Mathiak, K., and Weber, R. (2006). Toward brain correlates of natural behavior: fMRI during violent video games. Hum. Brain Mapp. 27, 948–956. doi: 10.1002/hbm.20234
Mathiak, K. A., Klasen, M., Weber, R., Ackermann, H., Shergill, S. S., and Mathiak, K. (2011). Reward system and temporal pole contributions to affective evaluation during a first person shooter video game. BMC Neurosci. 12:66. doi: 10.1186/1471-2202-12-66
Mathiak, K. A., Klasen, M., Zvyagintsev, M., Weber, R., and Mathiak, K. (2013). Neural networks underlying affective states in a multimodal virtual environment: contributions to boredom. Front. Hum. Neurosci. 7:820. doi: 10.3389/fnhum.2013.00820
McNeill, D. (2005). Gesture and Thought. Chicago, IL: Chicago University Press. doi: 10.7208/chicago/9780226514642.001.0001
Mittelberg, I. (2008). “Peircean semiotics meets conceptual metaphor: iconic modes in gestural representations of grammar,” in Metaphor and Gesture, eds A. Cienki and C. Müller (Amsterdam: John Benjamins), 115–154.
Mittelberg, I. (2013). “The exbodied mind: cognitive-semiotic principles as motivating forces in gesture,” in Body – Language – Communication (HSK 38.2) An International Handbook on Multimodality in Human Interaction (38.1), eds C. Müller, A. Cienki, E. Fricke, S. H. Ladewig, D. McNeill, and S. Teßendorf (Berlin: De Gruyter Mouton), 755–784.
Mittelberg, I. (2018). Peirce’s Universal Categories: On Their Potential for Gesture Theory and Multimodal Analysis. Berlin: De Gruyter.
Mittelberg, I., and Waugh, L. R. (2014). “Gestures and metonymy,” in Body - Language Communication. An International Handbook on Multimodality in Human Interaction, Vol. 2, eds C. Müller, A. Cienki, E. Fricke, S. H. Ladewig, D. McNeill, and J. Bressem (Berlin: De Gruyter Mouton), 1747–1766.
Nardo, D., Console, P., Reverberi, C., and Macaluso, E. (2016). Competition between visual events modulates the influence of salience during free-viewing of naturalistic videos. Front. Hum. Neurosci. 10:320. doi: 10.3389/fnhum.2016.00320
Nöth, W. (2001). “Semiotic foundations of iconicity in language and literature,” in The Motivated Sign: Iconicity in Language and Literature, eds O. Fischer and M. Nänny (Amsterdam: John Benjamins), 17–28.
Nummenmaa, L., and Calder, A. J. (2009). Neural mechanisms of social attention. Trends Cogn. Sci. 13, 135–143. doi: 10.1016/j.tics.2008.12.006
Ochsner, K. N., and Lieberman, M. D. (2001). The emergence of social cognitive neuroscience. Am. Psychol. 56, 717–734. doi: 10.1037/0003-066X.56.9.717
Özyürek, A. (2014). Hearing and seeing meaning in speech and gesture: insights from brain and behaviour. Philos. Trans. R. Soc. Lond. B Biol. Sci. 369:20130296. doi: 10.1098/rstb.2013.0296
Pajula, J., Kauppi, J. P., and Tohka, J. (2012). Inter-subject correlation in fMRI: method validation against stimulus-model based analysis. PLoS One 7:e41196. doi: 10.1371/journal.pone.0041196
Paolucci, C. (2011). Semiotics, pragmatism, extended mind and distributed cognition. Versus 2, 69–96.
Park, S., Brady, T. F., Greene, M. R., and Oliva, A. (2011). Disentangling scene content from spatial boundary: complementary roles for the parahippocampal place area and lateral occipital complex in representing real-world scenes. J. Neurosci. 31, 1333–1340. doi: 10.1523/JNEUROSCI.3885-10.2011
Peelen, M. V., and Kastner, S. (2014). Attention in the real world: Toward understanding its neural basis. Trends Cogn. Sci. 18, 242–250. doi: 10.1016/j.tics.2014.02.004
Peirce, C. S. (1955). “Logic as semiotic: the theory of signs,” in Philosophical Writings of Peirce, ed. J. Buchler (New York, NY: Dover), 98–119.
Peirce, C. S. (1960). Collected Papers of Charles Sanders Peirce, Vol. 1 2, eds C. Hartshorne and P. Weiss (Cambridge: The Belknap of Harvard University Press).
Pelphrey, K. A., Morris, J. P., and McCarthy, G. (2004). Grasping the intentions of others: the perceived intentionality of an action influences activity in the superior temporal sulcus during social perception. J. Cogn. Neurosci. 16, 1706–1716. doi: 10.1162/0898929042947900
Poser, B. A., Versluis, M. J., Hoogduin, J. M., and Norris, D. G. (2006). BOLD contrast sensitivity enhancement and artifact reduction with multiecho EPI: parallel-acquired inhomogeneity-desensitized fMRI. Magn. Reson. Med. 55, 1227–1235. doi: 10.1002/mrm.20900
Potter, V. G. (1967). Charles S. Peirce on Norms and Ideals. Amherst, MA: The University of Massachusetts Press.
Pulvermüller, F. (2013). How neurons make meaning: brain mechanisms for embodied and abstract-symbolic semantics. Trends Cogn. Sci. 17, 458–470. doi: 10.1016/j.tics.2013.06.004
Rach, S., Diederich, A., and Colonius, H. (2011). On quantifying multisensory interaction effects in reaction time and detection rate. Psychol. Res. 75, 77–94. doi: 10.1007/s00426-010-0289-0
Redcay, E., Dodell-Feder, D., and Pearrow, M. (2010). Live face-to-face interaction during fMRI: a new tool for social cognitive neuroscience. Neuroimage 50, 1639–1647. doi: 10.1016/j.neuroimage.2010.01.052.Live
Saggar, M., Shelly, E. W., Lepage, J.-F., Hoeft, F., and Reiss, A. L. (2014). Revealing the neural networks associated with processing of natural social interaction and the related effects of actor-orientation and face-visibility. Neuroimage 84, 648–656. doi: 10.1016/j.neuroimage.2013.09.046
Sato, W., Kochiyama, T., Uono, S., and Yoshikawa, S. (2009). Commonalities in the neural mechanisms underlying automatic attentional shifts by gaze, gestures, and symbols. Neuroimage 45, 984–992. doi: 10.1016/j.neuroimage.2008.12.052
Saxe, R., Xiao, D.-K., Kovacs, G., Perrett, D. I., and Kanwisher, N. (2004). A region of right posterior superior temporal sulcus responds to observed intentional actions. Neuropsychologia 42, 1435–1446. doi: 10.1016/j.neuropsychologia.2004.04.015
Schilbach, L., Wohlschlaeger, A. M., Kraemer, N. C., Newen, A., Shah, N. J., Fink, G. R., et al. (2006). Being with virtual others: Neural correlates of social interaction. Neuropsychologia 44, 718–730. doi: 10.1016/j.neuropsychologia.2005.07.017
Skipper, J. I. (2014). Echoes of the spoken past: how auditory cortex hears context during speech perception. Philos. Trans. R. Soc. B Biol. Sci. 369:20130297. doi: 10.1098/rstb.2013.0297
Sonesson, G. (2005). Lecture 4/: From the Linguistic Model to Semiotic Ecology?: Structure and Indexicality in Pictures and in the Perceptual World. Available at: http://semioticon.com/sio/courses/pictorial-semiotics/ [accessed May 20, 2017]
Sonesson, G. (2014). Still do not block the line of inquiry: on the Peircean way to cognitive semiotics. Cogn. Semiot. 7, 281–296. doi: 10.1515/cogsem-2014-0090
Spalding, K. N., Jones, S. H., Duff, M. C., Tranel, D., and Warren, D. E. (2015). Investigating the neural correlates of schemas: ventromedial prefrontal cortex is necessary for normal schematic influence on memory. J. Neurosci. 35, 15746–15751. doi: 10.1523/JNEUROSCI.2767-15.2015
Spiers, H. J., and Maguire, E. A. (2007). Decoding human brain activity during real-world experiences. Trends Cogn. Sci. 11, 356–365. doi: 10.1016/j.tics.2007.06.002
Stjernfelt, F. (ed.) (2007). Diagrammatology: An Investigation on the Borderlines of Phenomenology, Ontology, and Semiotics (Dordrecht: Springer). doi: 10.1007/978-1-4020-5652-9
Summerfield, C., and Egner, T. (2009). Expectation (and attention) in visual cognition. Trends Cogn. Sci. 13, 403–409. doi: 10.1016/j.tics.2009.06.003
Sykes, G. (2009). The images of film and the categories of signs: Peirce and Deleuze on media. Semiotica 2009, 65–81. doi: 10.1515/semi.2009.061
Turner, D. C. (2004). The role of the lateral frontal cortex in causal associative learning: exploring preventative and super-learning. Cereb. Cortex 14, 872–880. doi: 10.1093/cercor/bhh046
Tylén, K., Philipsen, J. S., Roepstorff, A., and Fusaroli, R. (2016). Trails of meaning construction: symbolic artifacts engage the social brain. Neuroimage 134, 105–112. doi: 10.1016/j.neuroimage.2016.03.056
Tylén, K., Wallentin, M., and Roepstorff, A. (2009). Say it with flowers! An fMRI study of object mediated communication. Brain Lang. 108, 159–166. doi: 10.1016/j.bandl.2008.07.002
Tzourio-Mazoyer, N., Landeau, B., Papathanassiou, D., Crivello, F., Etard, O., Delcroix, N., et al. (2002). Automated anatomical labeling of activations in SPM using a macroscopic anatomical parcellation of the MNI MRI single-subject brain. Neuroimage 15, 273–289. doi: 10.1006/nimg.2001.0978
van Koningsbruggen, M. G., Peelen, M. V., and Downing, P. E. (2013). A causal role for the extrastriate body area in detecting people in real-world scenes. J. Neurosci. 33, 7003–7010. doi: 10.1523/JNEUROSCI.2853-12.2013
Villarreal, M. F., Fridman, E. A., and Leiguarda, R. C. (2012). The effect of the visual context in the recognition of symbolic gestures. PLoS One 7:e29644. doi: 10.1371/journal.pone.0029644
Vossel, S., Geng, J. J., and Fink, G. R. (2014). Dorsal and ventral attention systems. Neurosci. 20, 150–159. doi: 10.1177/1073858413494269
Wagner, D. D., Kelley, W. M., Haxby, J. V., and Heatherton, T. F. (2016). The dorsal medial prefrontal cortex responds preferentially to social interactions during natural viewing. J. Neurosci. 36, 6917–6925. doi: 10.1523/JNEUROSCI.4220-15.2016
Wagner, P., Malisz, Z., and Kopp, S. (2014). Gesture and speech in interaction: an overview. Speech Commun. 57, 209–232. doi: 10.1016/j.specom.2013.09.008
Waskom, M. L., Kumaran, D., Gordon, A. M., Rissman, J., and Wagner, A. D. (2014). Frontoparietal representations of task context support the flexible control of goal-directed cognition. J. Neurosci. 34, 10743–10755. doi: 10.1523/JNEUROSCI.5282-13.2014
Weber, R., Behr, K. M., Tamborini, R., Ritterfeld, U., and Mathiak, K. (2009). What do we really know about first-person-shooter games? An event-related, high-resolution content analysis. J. Comput. Commun. 14, 1016–1037. doi: 10.1111/j.1083-6101.2009.01479.x
Willems, R. M. (2015). “Cognitive neuroscience of natural language use: introduction,” in Cognitive Neuroscience of Natural Language Use, ed. R. M. Willems (Cambridge: Cambridge University Press), 1–7. doi: 10.1017/CBO9781107323667
Wilson, S. M., Molnar-Szakacs, I., and Iacoboni, M. (2008). Beyond superior temporal cortex: intersubject correlations in narrative speech comprehension. Cereb. Cortex 18, 230–242. doi: 10.1093/cercor/bhm049
Wolf, D., Klasen, M., Eisner, P., Zepf, F. D., Zvyagintsev, M., Palomero-Gallagher, N., et al. (2018). Central serotonin modulates neural responses to virtual violent actions in emotion regulation networks. Brain Struct. Funct. doi: 10.1007/s00429-018-1693-2 [Epub ahead of print].
Wolf, D., Schock, L., Bhavsar, S., Demenescu, L. R., Sturm, W., and Mathiak, K. (2014). Emotional valence and spatial congruency differentially modulate crossmodal processing: an fMRI study. Front. Hum. Neurosci. 8:659. doi: 10.3389/fnhum.2014.00659
Wolf, I., Dziobek, I., and Heekeren, H. R. (2010). Neural correlates of social cognition in naturalistic settings: a model-free analysis approach. Neuroimage 49, 894–904. doi: 10.1016/j.neuroimage.2009.08.060
Xu, J., Gannon, P. J., Emmorey, K., Smith, J. F., and Braun, A. R. (2009). Symbolic gestures and spoken language are processed by a common neural system. Proc. Natl. Acad. Sci. U.S.A. 106, 20664–20669. doi: 10.1073/pnas.0909197106
Yang, J., Andric, M., and Mathew, M. M. (2015). The neural basis of hand gesture comprehension: a meta-analysis of functional magnetic resonance imaging studies. Neurosci. Biobehav. Rev. 57, 88–104. doi: 10.1016/j.neubiorev.2015.08.006
Zlatev, J. (2012). Cognitive semiotics: an emerging field for the transdisciplinary study of meaning. Public J. Semiot. 4, 2–24.
Keywords: social cognitive neuroscience, natural film viewing, functional imaging, semiotics, social interaction
Citation: Wolf D, Mittelberg I, Rekittke L-M, Bhavsar S, Zvyagintsev M, Haeck A, Cong F, Klasen M and Mathiak K (2018) Interpretation of Social Interactions: Functional Imaging of Cognitive-Semiotic Categories During Naturalistic Viewing. Front. Hum. Neurosci. 12:296. doi: 10.3389/fnhum.2018.00296
Received: 08 March 2018; Accepted: 06 July 2018;
Published: 14 August 2018.
Edited by:
Michael Hanke, Universitätsklinikum Magdeburg, GermanyReviewed by:
Enrico Glerean, Aalto University, FinlandDylan D. Wagner, The Ohio State University, United States
Copyright © 2018 Wolf, Mittelberg, Rekittke, Bhavsar, Zvyagintsev, Haeck, Cong, Klasen and Mathiak. This is an open-access article distributed under the terms of the Creative Commons Attribution License (CC BY). The use, distribution or reproduction in other forums is permitted, provided the original author(s) and the copyright owner(s) are credited and that the original publication in this journal is cited, in accordance with accepted academic practice. No use, distribution or reproduction is permitted which does not comply with these terms.
*Correspondence: Dhana Wolf, ZGh3b2xmQHVrYWFjaGVuLmRl