Corrigendum: Diffusion Tensor Imaging in Parkinson's Disease and Parkinsonian Syndrome: A Systematic Review
- 1Department of Psychiatry, War Related Illness and Injury Study Center, Veterans Affairs Palo Alto Health Care System, Palo Alto, CA, United States
- 2Department of Psychiatry, Mainline Health, Bryn Mawr Hospital, Bryn Mawr, PA, United States
Diffusion tensor imaging (DTI) allows measuring fractional anisotropy and similar microstructural indices of the brain white matter. Lower than normal fractional anisotropy as well as higher than normal diffusivity is associated with loss of microstructural integrity and neurodegeneration. Previous DTI studies in Parkinson's disease (PD) have demonstrated abnormal fractional anisotropy in multiple white matter regions, particularly in the dopaminergic nuclei and dopaminergic pathways. However, DTI is not considered a diagnostic marker for the earliest Parkinson's disease since anisotropic alterations present a temporally divergent pattern during the earliest Parkinson's course. This article reviews a majority of clinically employed DTI studies in PD, and it aims to prove the utilities of DTI as a marker of diagnosing PD, correlating clinical symptomatology, tracking disease progression, and treatment effects. To address the challenge of DTI being a diagnostic marker for early PD, this article also provides a comparison of the results from a longitudinal, early stage, multicenter clinical cohort of Parkinson's research with previous publications. This review provides evidences of DTI as a promising marker for monitoring PD progression and classifying atypical PD types, and it also interprets the possible pathophysiologic processes under the complex pattern of fractional anisotropic changes in the first few years of PD. Recent technical advantages, limitations, and further research strategies of clinical DTI in PD are additionally discussed.
Introduction
Parkinson's disease (PD) is the second most common neurodegenerative disorder after Alzheimer disease. PD generally begins with motor symptoms, including bradykinesia, rigidity, resting tremor, and postural instability. PD also includes numerous non-motor symptoms (such as cognitive impairment, depression, sleep behavioral problems, and olfactory dysfunction) (1). The classical neuropathologic hallmark of PD involves the loss of dopaminergic neurons in the substantia nigra (SN), resulting in a decreased dopaminergic output through the cortico-basal ganglia-thalamocortical motor circuit, which causes dysregulation of motor functions (2). Another neuropathologic characteristic of PD is the presence of Lewy bodies (3) and neurites in both neuron bodies and axons, including aggregates of the α-synuclein protein (4). When PD begins, the complex features of motor and non-motor symptoms reflect the progression of underlying pathologies, including α-synuclein immunoreactive inclusions in Lewy bodies; loss of dopaminergic, cholinergic, serotonergic, and noradrenergic projections from the brainstem to the midbrain and basal forebrain; and, finally, to the neocortex (5). Over the course of PD, patients commonly experienced comorbid neuropsychiatric disturbances, including depression, behavioral and cognitive deficits, and dementia, which need to be differentiated from other neurodegenerative causes such as dementia with Lewy bodies (DLB) and Alzheimer's disease. On the other hand, the term “parkinsonism” refers to motor syndromes that also present in PD, including bradykinesia, cogwheel rigidity, resting tremor, a slow shuffling gait, and imbalance. The atypical causes of parkinsonism that mimic idiopathic PD include multiple system atrophy (MSA), progressive supranuclear palsy (PSP), or corticobasal syndrome (CBS), as well as drug-induced parkinsonism and others. The most common motor and non-motor syndromes of PD, and other causes of parkinsonism that are involved in PD differential diagnosis, are listed in Table 1.
In PD, the loss of dopaminergic neurons and the accumulation of Lewy bodies are typically accompanied by damage of neuroglial cells and demyelination of axons with increasing microglia concentration in extracellular spaces. It is therefore plausible that the detection of extracellular microstructural abnormalities in brain regions with dopaminergic neurons and along dopaminergic pathways might be a biomarker of incipient PD.
Diffusion Tensor Imaging (DTI), one of the magnetic resonance imaging (MRI) sequences, has been employed to measure white matter microstructural integrity in neurodegenerative diseases, as well as to visualize brain fiber connections via tractography (6). Many quantitative DTI indices (as summarized in Table 2) could be derived from a clinical DTI sequence. Fractional anisotropy (FA), radial (RD), axial (AD), and mean diffusivity (MD) have been most commonly used to describe the degree of random motion of water molecules on a microscopic scale. Specifically, FA, which measures the directionality of random water motion, has been used to probe nerve fiber arrangements, axonal integrity, and the degree of axonal myelination (64). FA is clinically feasible to deduce the microstructural integrity of brain tissues, especially preferred to oriented tissues, such as white matter and fiber-tract architectures. MD measures the magnitude of water diffusion. A high MD is thought to indicate broad cellular damages including edema and necrosis. MD is used clinically to capture these microstructural alterations in both gray and white matter tissues (65). AD measures the magnitude of diffusion along the main axis, and RD measures the magnitude of transverse diffusion. In animal studies, increased RD appears to describe myelin pathology-induced myelin thinning (66), while decreased AD indicates acute axonal injury but does not correlate with chronic axonal damage (67). However, the clinical utilities of these DTI metrics have to consider several limitations: (1) All these DTI metrics lack validations with specific neuropathology in postmortem brain. (2) The interpretation of RD/AD might be difficult in voxels containing isotropic structure. Wheeler-Kingshott and Cercignani (68) reported that fictitious changes between RD and AD could occur in areas of low anisotropy, severe pathology, partial volume, and crossing-fibers. They advised that interpretations of RD/AD should be strongly discouraged under such circumstances. (3) There is no appropriate neurobiological implication for AD increases. Linking the increased AD to axonal recovery (as a reverse response of axonal injury) remains questioned, because many studies found AD increase is associated with neurodegenerative disorders such as dementia (69). Despite these limitations, the decreased FA and increased MD have been found correlated with neuronal degeneration (70) and degeneration caused by dopamine loss (71). Further, previous studies (72, 73) reported that abnormal FA values are detected in PD prior to atrophy, suggesting the usefulness of DTI measures as a biomarker of PD in clinical studies.
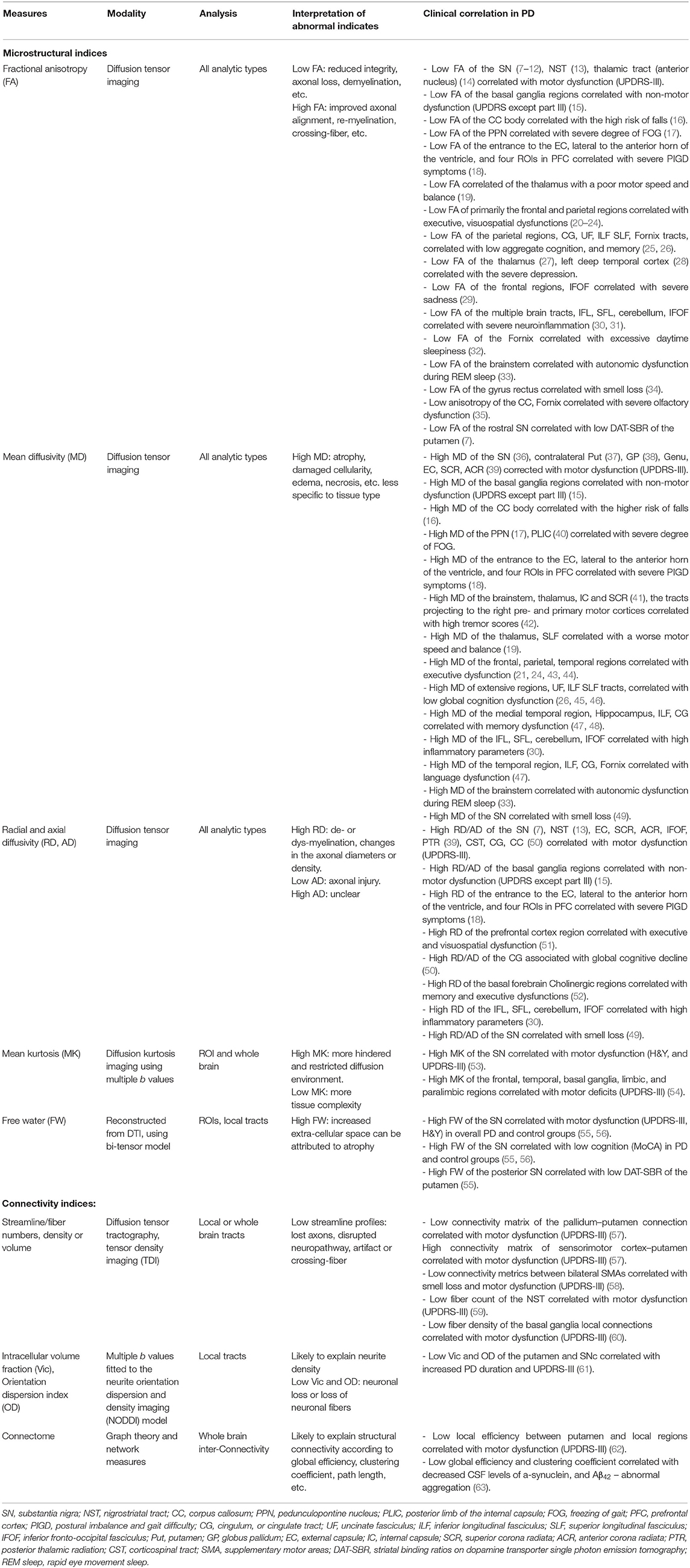
Table 2. Summary 1of common microstructural and connectivity indices derived from DTI, as well as their utilities, interpretations, and clinical correlations in PD.
This study aims to review a majority of clinically employed DTI studies in investigations of PD, together with a comparison of the results from a longitudinal, early stage, multicenter clinical cohort. It is sought to prove the utilities of DTI as a biomarker of diagnosing PD, correlating clinical symptomatology, and tracking disease progression and treatment effects on PD. Advantages and challenges of clinical application of DTI in PD will be discussed further.
DTI Assessment of PD Diagnosis
Non-hypothesis Driven, Voxel-Wise DTI Analyses in PD
The voxel-based whole brain analysis is a computational approach to identify to what extent in brain anatomy there are significant group differences in DTI indices, via a voxel-by-voxel comparison throughout the entire brain. This analysis automatically registers individual DTI maps to a template, which reduces the significant differences in brain anatomy between people. Registration algorithms can be intensity-based, for example, voxel-based analyses (VBA) (74), or tract-based, for example, tract-based spatial statistics (TBSS) (75). The advantages of the whole brain analyses are fully automatic, unbiased, unsupervised, without the need of prior hypotheses. However, the disadvantages of the whole brain approaches could be sensitive to various artifacts and technical issues, which include mis-registration of brain tissues, mis-classification of tissue types, etc. All these matters may confound the statistical analysis and either decrease the sensitivity to true DTI effects or increase the chance of false positives. Multiple studies have utilized non-hypothesis driven DTI analyses (Table 3) in comparing PD with healthy controls (HC). By analyzing datasets with a large variety of sample ages, disease severity, medication status, and non-motor symptoms, these studies reported a heterogeneous, multifocal pattern of abnormal DTI changes. The complex distribution of abnormal DTI changes is consistent with the notion that PD is a multisystem disorder involving several neurotransmitters beyond the loss of only dopamine. A recent review (102) summarized the various anatomical regions where abnormal DTI values correlated with a variety of PD symptomatology, including motor and motor phenotypes as well as non-motor features such as cognitive, mood, olfactory dysfunction, hallucinations/psychosis, and sleep disturbance, such as rapid eye movement sleep behavior disorder (RBD). It is therefore conceivable that the inconsistent findings of DTI abnormality across previous studies reflect the clinical heterogeneity of the study population. In this context, it will be indeed necessary to replicate these findings in a large population, detect imaging-clinical correlations under unique types of dysfunction, and track how these findings may alter during the early course of PD.
The Parkinson's Progression Markers Initiative (PPMI) Findings
Previous studies have highlighted a very complex picture of DTI as a PD-specific biomarker. For example, some studies reported that PD exhibits significantly lower FA, but some others showed PD has higher FA than HC subjects. To address if an FA increase or decrease is a characteristic feature of PD, we investigated the temporal changes of FA along the early disease course, by analyzing data from a multicenter, longitudinal PPMI (103) cohort (from now on termed “this study”). The methodological details of data selection, MRI acquisition, image processing, and analyses of this study are described in the Supplementary Material S1–S3. Overall, the PPMI data is characterized as early diagnosed cases (diagnosed within 2 years), drug-naïve (de-novo) including 22% patients with onset at young adulthood (i.e., age ≤ 50 years). Figures below depict the findings resulted from this study, as a comparison with the numerous results published previously.
Figure 1 shows statistical T-maps of FA differences between PD groups within year-1 (Y0) or more than 3 years (Y3), and demographically matched HC groups. Considering the impact of age at PD onset, the figure illustrated results of group differences separately for young-onset group (YPD, age of onset ≤ 50 years) and typical-onset group (OPD, age of onset > 50 years). In comparison between YPD(Y0) and YHC, significantly higher FA was observed in patients in multiple brain regions, including the bilateral corticospinal tract (CST), internal capsule, striatum (the putamen, pallidum, and caudate nuclei), anterior and posterior and superior corona radiata, as well as in white matter areas that lie next to the motor and supplementary motor cortices. Further, a comparison between YPD(Y3) and YHC showed that higher FA in patients remained significant in the internal capsule, striatum, corona radiata, and motor and supplementary motor white matter areas, but with a lesser extent of distribution. In the comparison between YPD and YHC groups, no region was found with lower-than-normal FA. On the other hand, the comparison between OPD(Y0) and OHC showed significantly higher FA in patients only in the bilateral CST. The comparison between OPD(Y3) and OHC showed prominently lower FA in patients in the SN, midbrain, thalamus, and multifocal non-motor areas of the white matter across all major lobes, while no area was found with higher-than-normal FA.
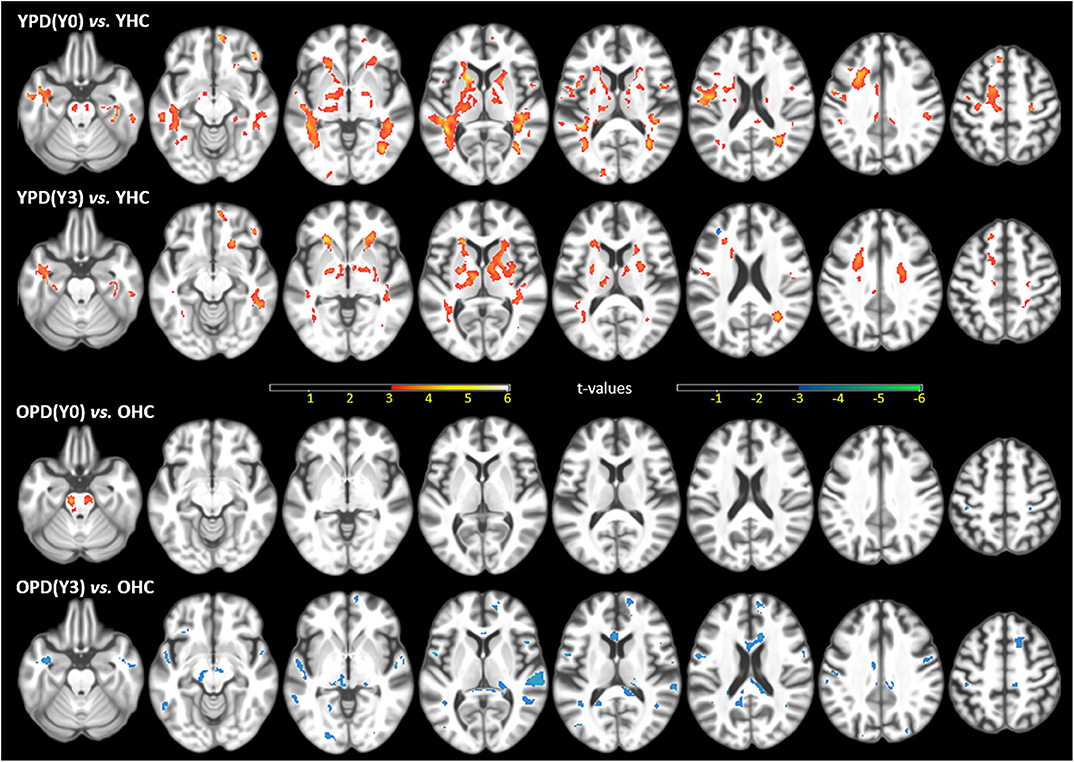
Figure 1. Statistical t-maps of fractional anisotropy (FA) maps, superimposed on a T1WI, of the significant differences between the first-year PD(Y0) or after the 3rd-year PD(Y3) patients and demographically matched HC (ANCOVA p ≤ 0.001, uncorrected for multiple comparison), separated for young onset (YPD) and old onset (OPD) groups, and demographically matched young (YHC) and old (OHC) controls. Clusters in warm colors indicate regions of higher FA values in PD than HC; clusters in cool colors indicate the opposite. This figure is illustrative and is created by the author.
Figure 2 depicts temporal pattern of FA changes during the early course of PD (from 0 to 79 months after the clinical diagnosis was made) in the SN and CST. For comparison, FA of the HC subjects at baseline are presented. The scatter plots are separately illustrated for the YPD vs. YHC groups and OPD vs. OHC groups. In CST, FA was significantly increased from the first year through the third year for both YPD and OPD, and later decreased to a normal level after the third year of clinical PD. In the SN, FA reduced steadily for OPD throughout the PD course.
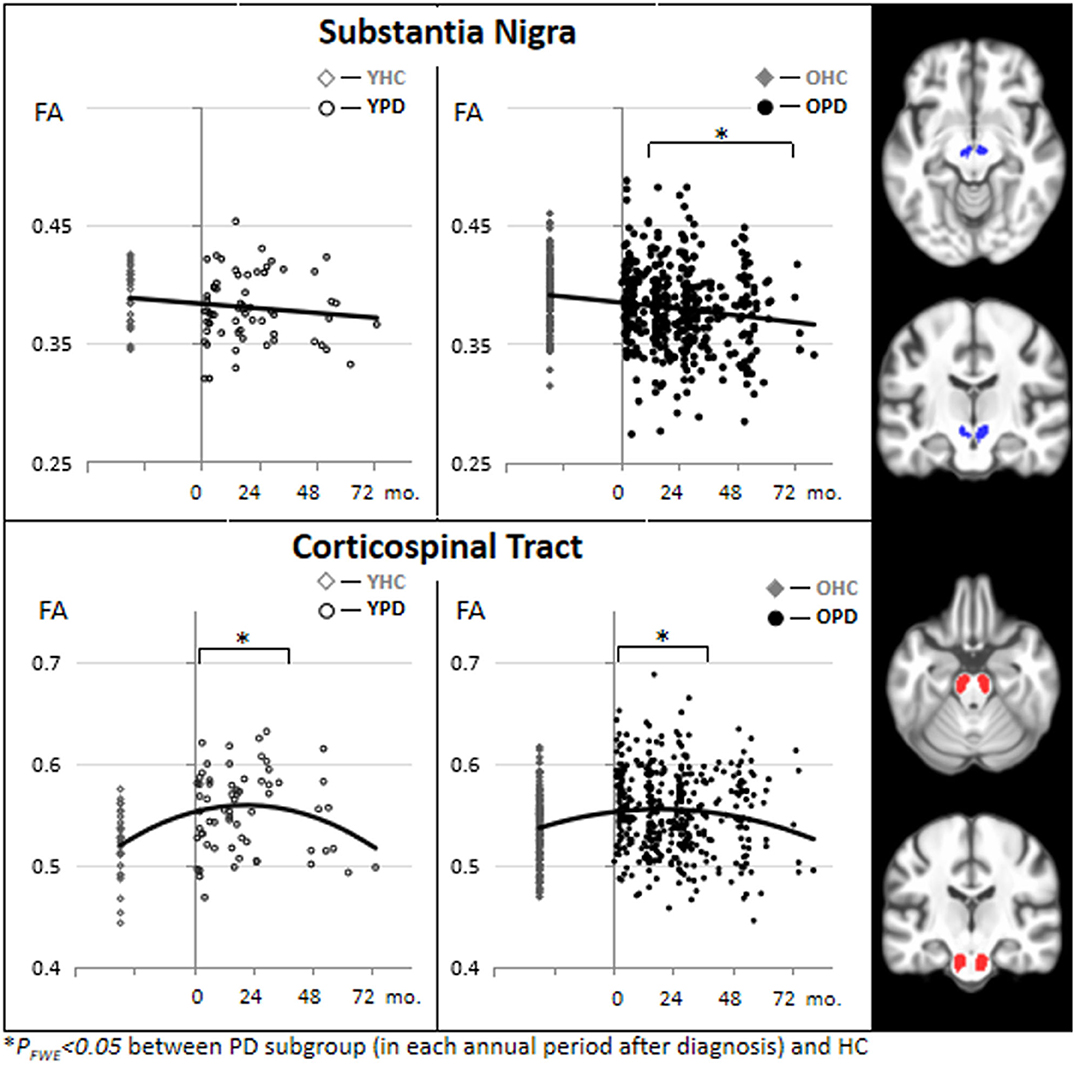
Figure 2. Scatter plots and binominal trend lines of FA changes during the course of PD (from 0 to 72 months after PD diagnosis) in the substantia nigra and the corticospinal tract, separated for young onset (YPD) and old onset (OPD) groups. FA values of the HC subjects are also separated into demographically comparable groups (OHC and YHC). This figure is illustrative and is created by the author.
Despite a multifocal regional pattern of FA reduction, which has been consistent with those commonly reported in literature, this study presented a robust FA increase in extensive motor areas in a large sample of the earliest and young-onset PD. The FA increase in the CST and other motor areas is not surprising because it has been also reported in previous studies using PPMI data (89, 97, 98) or other pilot data (80, 85, 92). Reduced FA is often found in neurodegenerative conditions, such as aging, Alzheimer's disease, and Parkinsonism, and is believed to be attributed to demyelination, loss of axons, and interrupted connection. It is unlikely that an FA increase is interpreted as its opposite physiological meaning (e.g., axonal regeneration, remyelination, redundant white matter networks) in neurodegenerative disorders. A meta-analysis (104) revealed that previous studies usually considered the FA increase to be due to methodological confounding factors. For example, different MRI scanners, field strength, and the number of diffusion directions could result in variability of the FA measurement and make group comparisons very difficult based on a multi-center population. Another issue is that voxels composed of fiber populations with different spatial orientations (so called “crossing-fibers”) may result in an average increase in FA. There are about 90% white matter voxels containing crossing-fibers (105). Crossing-fibers may affect an anisotropy analysis and might lead to difficulties in interpreting FA increases (106). In addition, a concomitant FA increase may also be due to the variability in ROI sizes, with less or more inclusion of voxels containing surrounding isotropic neurons with low FA. However, the coexistence of FA increase and FA reduction in this study is consistent with the previous meta-analysis (107), which by accounting for heterogeneities of 39 published articles revealed FA increase in the CST and caudate nuclei, and FA decrease in the SN, corpus callosum, cingulate, and temporal areas. With an increasing number of consistent findings, an elevated FA in early PD cannot merely be attributed to methodological confounding factors but could instead indicate some pathophysiological correspondences of the disease. Possible interpretations of the FA increase include compensatory responses or excitatory reactions, which have been proposed by many fMRI (108), perfusion (109), and glucose metabolic (110) studies as a PD-related anatomical pattern of motor dysfunction (PDRD). Compensatory increased structural connectivity could be parallel to hyper-functional activation in similar motor areas (111) and are particularly activated in young aged PD (112). A previous study (98), also using PPMI data, found an FA increase in the tremor dominant (TD) phenotype, but not in the postural instability and gait difficulty (PIGD) phenotype of PD, suggesting that the compensatory white matter reorganization is more specific to the TD type in early PD. Moreover, an earlier study (73) using pilot data reported a whole-brain FA increase only in the akinetic-rigid (AR) phenotype of PD. It is therefore highly encouraged to have more investigations to validate whether the pattern of FA increase is selectively associated with a specific phenotype of motor dysfunction (i.e., TD vs. PIGD or AR subtypes), in addition to several existing pilot cohorts showing conflicting results (18, 19, 41, 113). Although there is a lack of research to date, the pattern of coexisting FA divergency in early PD is likely also explained by the pathophysiology of inhibition (reduced white matter integrity) through direct pathways and disinhibition (increased white matter integrity) through indirect pathways in the basal ganglia (114).
Hypothesis Driven DTI Analyses in PD
DTI Changes in SN and SN Subdivisions
In contrast to the voxel-based whole-brain analysis, the hypothesis-driven region-of-interest (ROI) analysis is often used to identify DTI abnormalities in patients with PD in regions that are known to be specific to the characteristic PD pathologies. SN is one of the main sites of selective loss of dopaminergic neurons in PD, because there is already a loss of 60–80% dopaminergic neurons in the SN before PD motor symptoms emerge (115, 116). Table 4 lists 32 independent studies that analyzed DTI in the total SN area and SN sub-regions in order to identify substantial DTI alterations in patients with PD. These studies were included in four previous meta-analyses and reviews (104, 131, 135, 136). Except for two studies that reported elevated FA values in the SN of PD, 11 studies found no FA abnormalities. The other 19 studies reported significantly reduced FA values in the entire SN. Aside from FA, only a few studies found an MD increase in the SN, while most others found no significant MD abnormalities, suggesting FA is a better contributive index in identifying nigral abnormalities in PD. However, it remains unclear whether or not FA alterations in the entire SN (Type A ROI subdivision as illustrated in Table 4) is sensitive to capture the dopamine loss in PD.
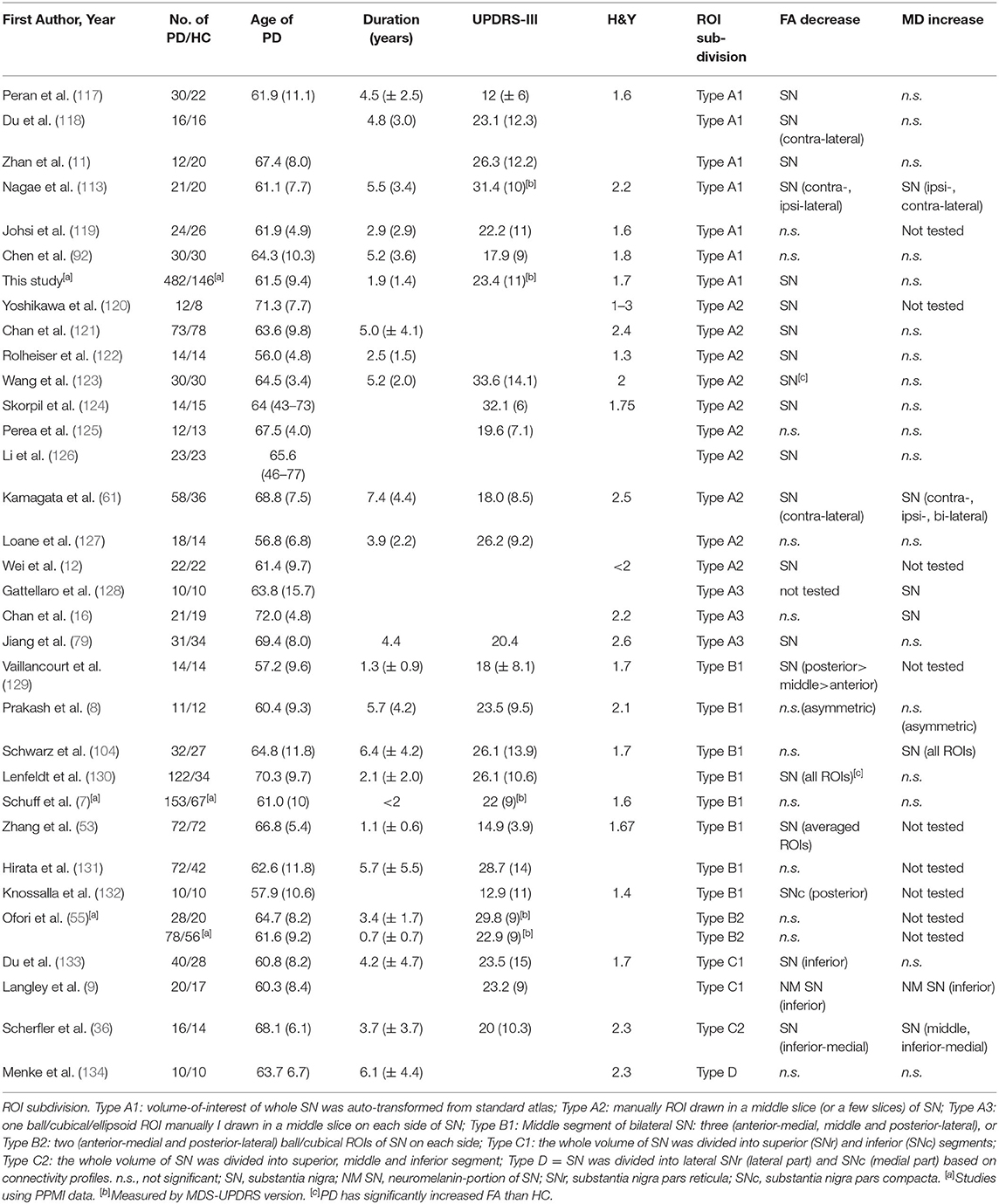
Table 4. Overview of studies in comparison of FA/MD differences in the SN and SN subregions between PD and HC.
Molecular and neuropathological studies (137) of PD have highlighted that progressive loss of dopaminergic neurons is primarily involved in the pars compacta (SNc), which contains rich pigment neuromelanin formed by dopaminergic neurons, and lies in the inferior and posterior part of the SN, in contrast to the pars reticulata (SNr), which lies lateral to the SNc. Therefore, focusing the microstructural alterations on SN subregions, such as the SNc, would appear to be ideal and vital in identifying dopamine loss-related abnormality. In general practice, it is challenging to delineate the SNc on DTI image because there are no well-defined borders of this substructure. An earlier study (129) reported that reduced FA in the caudal and lateral sub-regions of the SN could completely separate patients with PD from HC with 100% diagnostic accuracy. The authors proposed that FA measurements in the caudal and lateral sub-regions of the SN, presumably coinciding with the histological described SNc location (ROI subdivision Type B1), have the best diagnostic value for PD. However, eight subsequent studies with the same SN subdivision (subdivision Type B) reported discrepant findings: two studies (13, 132) likely replicated the findings of FA reduction in the dorsal lateral sub-region of the SN in PD; five other studies (7, 8, 55, 104, 131) found no significant FA differences between PD and HC in any SN sub-region; and one (130) reported elevated FA in all the SN sub-regions in PD. Technical difficulties outlining the sub-regions may explain these discrepant findings. To combat these issues, several studies (9, 36, 133) have divided the SN into two or three segments from the anterior top position through the posterior bottom position (ROI subdivision Type C). All these studies reported significantly decreased FA in the caudal (or inferior) segment of SN in patients with PD as compared with HC. Among these studies, Langley 2016 (9) used a mask of caudal zone in the SN that was previously established in a brain atlas based on magnetization transfer MRI that is sensitive to neuromelanin. This mask (i.e., the inferior portion of SN) supposedly coincides with the neuromelanin accumulation site. Another study (134) localized SN subfields using DTI tractography to segment the SN into an internal and an external part (ROI subdivision Type D), claiming that each part represents the SNc (the internal SN) and SNr (the external SN), respectively. However, no significant differences in FA or MD values between patients with PD and HC were identified in any of these subdivisions. It is likely that differences in delineating and partitioning the SN sub-regions across DTI studies are largely responsible for the inconsistent findings. Further methodological improvements are needed to ultimately demonstrate that microstructural alterations in SNc are a sensitive marker for diagnosing PD.
DTI Changes in Dopaminergic Tracts
The classical pathophysiological model of PD and recent updates have been established based on experimental and clinical studies (138–141), which consists of functional disabilities of two main pathways: an “indirect circuit,” which interconnects neocortex-putamen-external pallidum/subthalamic nucleus-SN, and a “direct circuit,” which interconnects neocortex-putamen-internal pallidum-SN-thalamus-neocortex. Under the conditions of PD, the reduced dopamine (results from neuronal loss in the SN) inhibits the indirect pathway, resulting in slow movement, and excites the direct pathway that may lead to unwanted movement such as resting tremor. Figure 3 (left panel) illustrates an example of these basial ganglia pathways on DTI imaging. Neuroanatomically, the dopaminergic pathway, meaning the structural connection between SN and putamen, has been assumed to contain dual effects of activities onto the indirect and direct pathways. Detecting white matter damage to these neuromodulator pathways could add to the understanding of PD pathophysiology.
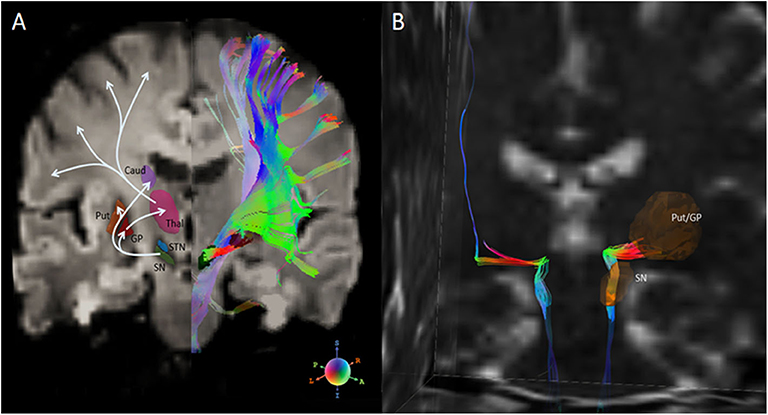
Figure 3. (A) Left half-panel: basal ganglia nuclei and connections of the direct and indirect pathways for movement. Right half-panel: diffusion tensor tractography of all fiber streamlines that path through the SN. (B) Isolation of the nigrostriatal tract (NST) between SN and inferior Put/GP in a healthy subject. Diffusion tensor tractographic streamlines on the right hemisphere shows the nigrostriatal tract anatomically connects through brainstem, SN and Put/GP. A part of the streamlines further connects through the Thal, and finally projects into the premotor cortex. Fiber orientation is R-G-B coded. Note, DTI tractography does not separate the afferent and efferent directions. SN, substantia nigra; Put, putamen; GP, globus pallidus; Caud, caudate nucleus; Thal, thalamus; STN, subthalamic nuclei. This figure is illustrative and is created by the author.
Diffusion tensor tractography, as an alternative DTI-based approach, offers a possibility to detect the disrupted connections of the dopaminergic pathway and may aid the early diagnosis of PD. White matter tracts that connect the SN and striatum, namely the nigrostriatal tract (NST), are considered one of the major dopaminergic pathways, and have been shown clinically applicable in viewing anatomy of the healthy human brain (142), as well as targeting deep brain stimulation (DBS) (143, 144). Although diffusion tensor tractography could not reach much detailed pathophysiologic meanings due to the limited resolution and directional information, it might still indirectly reflect a functional dysregulation of the dopaminergic circuits on a neuroanatomical basis. Figure 3 (right panel) shows an example of the nigrostriatal tractography in a healthy PPMI subject. An early DTI study (120) measured DTI indices in 11 oval ROIs that were arrayed along a line between the SN and caudate/putamen complex, and reported significantly lower than normal FA values in patients with parkinsonism at all disease stages. With the advances of DTI tractography, recent studies (13, 59, 89, 145, 146) have assessed the FA and tract connectivity profiles of the NST in identifying differences of nigrostriatal connection between patients with PD and healthy subjects. Most of these studies reported either an FA decrease (along with an increase in RD) (13, 59) in PD or reduced tract connectivity (59, 145, 146). Some studies (13, 59, 60) also found that reductions in FA or tract profiles are associated with the severity of motor dysfunctions in PD patients. These consistent findings provide a possible explanation of PD pathophysiological mechanism, that is, the main motor manifestations of PD are related to the diminished connectivity of this dopaminergic circuit. Although based on a small sample, Menke et al. (147) demonstrated that the combination of volumes of the SN and the NST could achieve 100% sensitivity and 80% specificity for PD classification. Taken together, these studies suggest that DTI tractography is a promising, complementary marker of PD diagnosis. On the other hand, a tractographic analysis of the NST also benefits new PD treatment strategies that target the nigrostriatal pathway, such as deep brain stimulation, dopamine graft implantation, and infusion of glial-cell-line–derived neurotrophic factor.
Other white matter circuits that may be involved in PD pathology are of research interests. Braak et al. (3) suggested that Lewy bodies are mainly confined to the medulla oblongata/pontine tegmentum and olfactory bulb/anterior olfactory nucleus in the earliest stages of PD. Using voxel-based analysis, two studies (90, 148) found PD is associated with abnormal diffusivity in white matter tracts adjacent to the olfactory sulcus. Although tractography of the olfactory tract is practically difficult in this area, using ROI drawing, some studies (92, 119, 122) reported low FA of the olfactory tract in PD. Another interesting approach is the tractographic analysis in corticothalamic connections of PD. The reduction of dopamine in SN results in abnormal activation of connections between the thalamus and the motor cortices. This may subsequently lead to inhibition of basal ganglia output and dysfunction in the cortical-subcortical circuits. Several studies have focused on the DTI connection of the corticothalamic tracts (14, 60, 85). However, diverse FA changes were reported in these studies.
DTI Assessment of Clinical Symptomatology and Dopamine Transporter
PD is characterized as a wide variety of motor and non-motor symptoms, while the neuropathological processes underlying these heterogeneous symptoms are not fully understood. Assessing imaging-clinical correlations in PD has been considered useful for adding further insights into neuropathological underpinnings of PD symptomatology. Table 2 summarizes the findings of correlations between available DTI metrics and clinical assessments.
DTI Correlates of Motor Dysfunction
A previous article (102) provided a thorough review of the correlations between diffusivity abnormalities and PD motor symptoms, but it lacked information about FA. Many studies, including the analyses with PPMI data, have reported significant correlations between decreased FA in the SN and increased severity of the motor symptoms, which are assessed by the motor exams (part-III) of the Unified Parkinson's Disease Rating Scale (UPDRS) (7–12, 61, 92) or H&Y scales (113, 121). However, some other studies failed to observe significant correlations. One reason for the discrepant reports of previous studies is the variation of “on-medication” or “off-medication” conditions in real-time examination of the PD patients. Dopaminergic treatments have substantial effects on the clinical measurement of motor symptoms such as UPDRS, whereas they have less effects on structural imaging such as DTI. Another complication is that, unlike quantitative imaging measurement, UPDRS is a subject measurement and depends on observers' experiences (149). Nevertheless, the consistent correlation findings from many previous studies imply that nigral FA values could offer an objective assessment of severity of the PD motor dysfunction, when clinical motor measurement is not affected by real-time treatment effects.
Investigations of the correlations between DTI and subtypes of motor dysfunctions remain rudimentary. A few studies revealed significant correlations between DTI changes and severities in terms of freezing of gait (17, 40), risk of falling (16), severity of postural instability and gait disturbance (PIGD) symptoms (18), motor speed and balance (19), and the degree of tremor (41, 42). Some studies, using DTI for classifying PD motor subtypes (73, 95), have found differentiable group effects only, but no substantial clinical correlations were observed in patients. The abnormal DTI changes in specific regions are considered useful biomarkers that characterize PD symptomology or differentiate PD phenotypes, and thus may provide an enormous potential for managing treatment directions of PD. However, it should be cautioned that the diversity of the subtype definition and the substantial instability in motor scoring might hamper the actual imaging-clinical correlations and interpretations. More reliable, objective measures of motor subtypes are necessary to validate these subtype-associated DTI changes conclusively.
DTI Correlates of Non-motor Dysfunction
Cognitive impairment is one of the major non-motor syndromes in PD. In patients with non-demented PD, the relationships between white matter integrity and global cognition, functions of various cognitive domains have been investigated in multiple studies (as illustrated in Table 2). For example, global cognitive function measured by Mini-Mental State Examination (MMSE) or Montreal Cognitive Assessment (MoCA) has been found to be associated with abnormal DTI variables in the bilateral frontal, parietal, and temporal regions, long association tracts connecting these cortices, and the hippocampus (25, 26, 43, 45, 95). Executive dysfunctions are associated with abnormal DTI variables widely distributed in the parietal and frontal regions (20–23, 43–45, 51). Declined verbal and semantic fluency and visuospatial memory are associated with abnormal diffusion variables in the parietal and frontal regions (23, 24, 44, 51). Worsened performance of language and attention is associated with abnormal diffusivities in frontal and temporal regions and the fornix (46, 47). Memory impairments are correlated with the frontal and hippocampal diffusivity abnormalities (45, 48, 52). Overall, the characteristic white matter abnormalities associated with cognitive impairment in PD can be summarized as follows: multiple regions are involved with a heterogeneous pattern; abnormal diffusion variables are widely distributed in white matter adjacent to cortices and limbic subcortices; the diffusion abnormalities are predominantly shown as the diffusivity changes but not the FA.
DTI correlates with other non-motor manifestations in PD have been comprehensively reviewed in a previous article (102). Briefly, DTI alterations in the thalamus (27), damaged long association tracts connecting to the frontal cortices, are associated with depression (28) and sadness (29). Diffusion changes of the hippocampus are found to be related to the impaired visuospatial memory, leading to visual hallucinations (150) in the advanced stages of PD. Diffusivity changes in the brainstem, midbrain, and pons are associated with autonomic dysfunction during a rapid eye movement (REM) sleep (33), and diffusion alterations of the limbic fornix are associated with excessive daytime sleepiness (32). However, most of these studies have been limited to small sample sizes, resulting in a low statistical power, and conflictions in terms of findings and interpretations. Further replication studies are needed to elucidate these abnormalities in more details and to truly aid the unique insights into PD symptomology.
DTI Correlates of Striatal DAT-SPECT
Dopamine transporter single photon emission tomography (DAT-SPECT) provides a meaningful measurement of striatal dopaminergic deafferentation. The best quantitative assessment on DAT-SPECT is to measure the striatal dopamine transporters binding ratio (SBR) in the left and right putamen and caudate nucleus. Studies have demonstrated substantial SBR signal loss in early PD patient relative to controls (151, 152). Recent machine learning techniques (such as using the support vector machine, SVM) have shown that the SBR measures achieve high accuracies (97–100%) in predicting early PD (153, 154). Additionally, the SBR has been shown strongly correlated with the number of dopaminergic neurons in substantia nigra (155). Pathological data also confirmed that quantitative measures of SBR enhance the accuracy of detecting dopaminergic neuron loss in PD (156), suggesting that SBR might be a reliable diagnostic biomarker of PD. Therefore, identifying a significant relationship between abnormal DTI and diminished SBR would support the idea that DTI alteration in the dopaminergic area is a potential marker of PD pathology. Recent findings showed a strong relationship between abnormal DTI [including decreased free water (FW) (7, 55) and increased FW (157) of the SN] and decreased putaminal SBR in PD patients. Furthermore, the progressive reduction of nigral FA has been shown correlated with the rate of SBR reduction over the first year of the PPMI patients (158). Aside from a few studies (159, 160) that reported controversial findings, the strong inter-modality correlations suggest that the abnormal DTI changes in the SN are, at least indirectly, associated with the loss of dopaminergic neurons in PD. Thus, this supports the usefulness of nigral DTI measures in linking neurodegeneration to the characterized dopaminergic deficiency in PD.
DTI Assessment of PD Progression and Treatment Effect
Although it remains a question using the DTI as a diagnostic marker of early PD, many previous studies have reported stable, region-specific, cross-sectional correlations between DTI alterations in the dopaminergic regions and motor symptom severities, suggesting the usefulness of longitudinal DTI as a reliable biomarker for monitoring PD progression. A recent longitudinal DTI study (127) has found increased rate of DTI abnormalities (i.e., FA decrease and MD increase) in the SN of PD patients over a 19.3-month follow-up. Ofori et al. (161) reported significant FW increases in the posterior SN at 1-year follow-up of PD. Further, the same authors' group (162) confirmed these longitudinal FW increases using PPMI data (103 PD at baseline, the 1st, 2nd, and 4th year) in comparison with unchanged FW in the control group (49 HC at baseline, the 1st year). Another longitudinal study (158) measuring FA and diffusivity variables of the PPMI data (122 PD vs. 50 age matched HC at baseline and 1-year follow-up) reported that PD has the highest annual rate of 3.6 ± 1.4% FA reduction in the SN, followed with a moderate rate of FA reduction in the basal ganglia, in comparison with the non-significant DTI changes in HC. Several longitudinal DTI studies identified other vulnerable regions over the PD progression: a recent study (163) found PD had a greater decrease in FA and increase in MD in the rostral brainstem, compared to controls; a 2-years longitudinal DTI study (164) reported a decrease in FA in the putamen of PD patients; another cohort (94) with 18-month follow-up of PD patients with cognitive impairment revealed greater MD increases in frontal white matter than those patients with normal cognition. These studies have presented a similar topology (mainly in the SN, also involved in the midbrain, thalamus, and to some extent to the frontal white matter) of FA decrease/MD increase over the PD course. This anatomical pattern of longitudinal DTI changes is consistent with the regional spread of Lewy body and the accumulation of Lewy neurites during PD progression (5). According to the generally consistent findings, longitudinal DTI shows a promising PD progression marker and could be valuable for monitoring and evaluating treatment effects.
Outcome measures that are most commonly used for tracking the PD treatment effects are the standard UPDRS scores (165, 166) and, sometimes, the dopamine transporter imaging (167). A PPMI study (168) followed 423 patients from treatment beginning to year-5 and found that dopaminergic therapy provides significant improvements in the Movement Disorder Society revised UPDRS (MDS-UPDRS) scores and the SBR calculated from DAT-SPECT. However, these functional outcome measures may appear significantly different depending on whether they are evaluated at ON or OFF medication status. Tracking the long-term treatment effects using DTI measures could be promising as DTI is used to identify chronic responses of the brain microstructure, and thus is considered to be less affected by the ON or OFF medication status at the imaging time. Figure 4 depicts individual trajectories of nigral FA values extracted from the PPMI data (134 patients and 75 healthy subjects) over a maximum of 36 months (data information is provided in Supplementary Material S1–S3). It shows that the untreated PD patients exhibit on average a steeper decline in FA than HC subjects, while the treated PD patients maintain their respective FA levels to a decline that begins in the 3rd year of medication. This finding supports the fact that early PD is generally responsive to medications. Taken together with recent PPMI findings that nigral FA is associated with disease duration and motor rating scales (97, 169), we suggest that disease modifying effects of levodopa treatment can be measured by longitudinal changes of nigral FA, at least in the first few years of treatment. Further DTI studies of PD clinical trials are needed to validate whether this is indeed true.
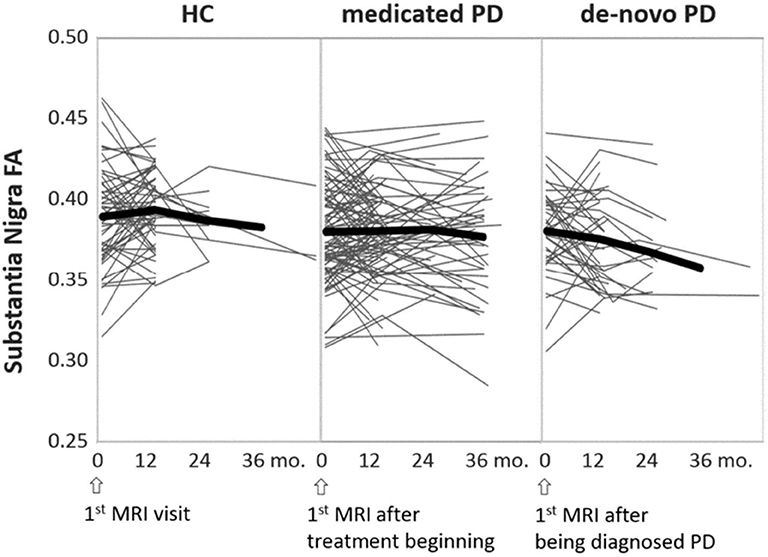
Figure 4. Individual trajectories of the nigral FA changes over time, separately illustrated for HC, treated (medicated), and untreated (de-novo) PD groups. The thick black lines indicate the mean trajectory of FA changes over time at each group level. Time is represented as the MRI intervals, starting from the baseline MRI through the latest follow-up MRI. This figure is illustrative and is created by the author.
DTI Assessment of Differential Diagnosis
Differentiation Between PD and Atypical PD
It is important to diagnostically differentiate between idiopathic PD and atypical parkinsonism (aPD), including the three most common sporadic neurodegenerative syndromes: multiple system atrophy (MSA), progressive supranuclear palsy (PSP), and corticobasal syndrome (CBS), because interventional management and prognosis could be different and difficult for aPDs. DTI shows a substantial value in such differentiation.
Compared to idiopathic PD, various DTI studies have demonstrated reduced FA and/or increased MD in the pons, cerebellum, middle cerebellar peduncles (MCP), and putamen in patients with a Parkinsonian type of MSA (MSA-P) (88, 126, 170–174). Several cross-validation studies (173, 175–178) reported that diffusion measurement has 73–94% sensitivity and 89–94% specificity, and 89–100% area under curve (AUC) in discriminating MSA-P from idiopathic PD.
PSP and CBS are neurodegenerative disorders characterized by abnormal tau pathology in the form of globose neurofibrillary tangles, tufted astrocytes, coiled bodies, and threads, with a predominance of 4-repeat (4R) tau isoforms (179). Both diseases provoke symptoms characteristic of PD. A recent review (180) suggests that quantitative measures and visual assessments of atrophy in the superior cerebellar peduncles (SCP) are substantial for differentiating PSP from PD, suggesting microstructural analysis of this key anatomy may provide supportive information. DTI studies (174, 181–183) showed that patients with PSP present strikingly decreased FA and increased MD in the SCP in comparison to patients with idiopathic PD. Studies (174, 184) examining FA of the SCP yielded 86–94% sensitivity and 88–94% specificity for differentiating PSP from PD. Although there are few related investigations in literature, a previous study reported significantly decreased FA and increased MD in the posterior callosal truncus in CBS patients in comparison to PD. Measuring MD of the truncus yielded a 78% AUC in discriminating between CBS and PD.
In summary, PD and aPD are differentiable according to their unique anatomical distributions of microstructural abnormalities detected by reduced FA and increased MD. For example, abnormal DTI in the cerebellum and MCP may be primarily involved in MSA-P; DTI of the SCP is mostly vulnerable in PSP; and abnormal DTI in the supratentorial white matter regions is primarily involved in CBS. Additional evidence that supports these regionally specific changes in PSP and CBS are the high progressive rate of DTI abnormalities (185) and the progressive atrophy (186, 187) in respective key regions of PSP and CBS observed in previous longitudinal imaging studies. For idiopathic PD, the predominant DTI changes are characterized in the SN and the basal ganglia.
Differentiation Between PD and Associated Cognitive Impairment and Dementia
Cognitive impairment, one of the non-motor symptoms, is particularly problematic in PD. Mild cognitive impairment (MCI) occurs in approximately 30% of PD patients (188). Up to 80% of late-staged PD patients eventually develop an associated dementia (PDD) (189, 190). In general, subtle cognitive deficits occur in early-staged PD patients, and dementia typically occurs in elderly patients or patients in advanced stages. With matched stages and ages, PD patients with MCI (PD-MCI) show a decreased FA and increased MD compared to cognitively normal PD (PD-CN) predominantly in the frontal and interhemispheric white matter (i.e., genu and body of the corpus callosum) (45, 191–193). The PDD group, when compared to the non-demented PD group, showed FA decrease and/or MD increase in the bilateral cingulate tract (194–196), hippocampus (26), prefrontal white matter, and the genu of the corpus callosum (196, 197). In addition, cognitive status, measured by MMSE or MoCA, was associated with abnormal DTI changes in the corpus callosum, anterior cingulate, and several frontal white matter areas (26, 195, 196, 198). Moreover, Shchuz's study (199) suggested that degeneration of the nucleus basalis of Meynert measured by DTI predicts the onset of cognitive impairment, whereas a PPMI study (200) could not confirm DTI as a predictor for further cognitive decline.
The neuropathological basis of dementia in PD is not yet clear. Detectable DTI alterations in the white matter connecting to the neocortex and limbic cortex, including the frontal white matter, the corpus callosum, cingulum, and hippocampus may provide meaningful information in identifying dementia from PD. Consistent with Braak's stages V-VI (3), when α-synuclein spreads to neocortex particularly to the frontal, temporal, and limbic cortices, the abnormal DTI changes in these neuronal fibers play an important role in manifesting dementia processing in PD. On the other hand, the primary mechanisms of cognitive impairment in PD have been manifested by atrophy and reduced glucose hypometabolism in the cerebral cortices (e.g., the temporoparietal cortices) and limbic cortices (e.g., the posterior cingulate gyrus and the hippocampus) (201). White matter burden might occur secondarily or indirectly after the gray matter pathologies such as cortical accumulations of amyloid beta and tau. Further, a study (202) reported that PD with early onset of MCI (onset at PD < 1 year) exhibited lower FA and higher diffusivities in the frontal white matter than PD with late onset of MCI (onset at PD ≥1 year). In this context, whether the cognitive decline and dementia are manifested by age or dopamine deficiency needs further investigations.
A few studies (25, 203) using DTI in differentiation between PDD and Lewy body dementia (LBD) found generally similar regions of reduced FA in both disorders. A few other studies (51, 125) comparing PDD with Alzheimer's disease suggested that PDD may exhibit greater white matter abnormalities than Alzheimer's disease, whereas Novellin et al. (204) suggested the controversial findings. Due to the limited number investigations and the conflicting results, whether DTI can reliably differentiate PDD from LBD or Alzheimer's disease remains unknown.
Developments in DTI Techniques
Advanced Diffusion Imaging Technology
Table 5 lists a comparison of imaging parameters in conventional DTI, PPMI, and advanced diffusion imaging techniques. To date, DTI is not broadly applied in clinically standard MRI examinations. In clinical practice, the conventional DTI sequence, especially for scanning patients, has to be performed within 10 min or so. The limited scan time results in problems such as low resolution, noise, artifacts, distortion and crossing-fibers, and reduces qualities of the DTI image and its quantitative measures. Technical developments and limitations of DTI studies in clinical PD have been well-documented in recent review papers (107, 205). New developments of diffusion imaging techniques, such as high angular resolution diffusion imaging (HARDI) (206) and diffusion spectrum imaging (DSI) (207), have shown improvements including increased signal sensitivity and resolution, gaining details of intravoxel directions and allowing a better differentiation of the crossing fibers or the joining fibers. These novel techniques are primarily used for mapping human brain connectome and brain fiber atlas building. Optimization of these sequences to be a clinically tolerable acquisition will be necessary for their clinical application.
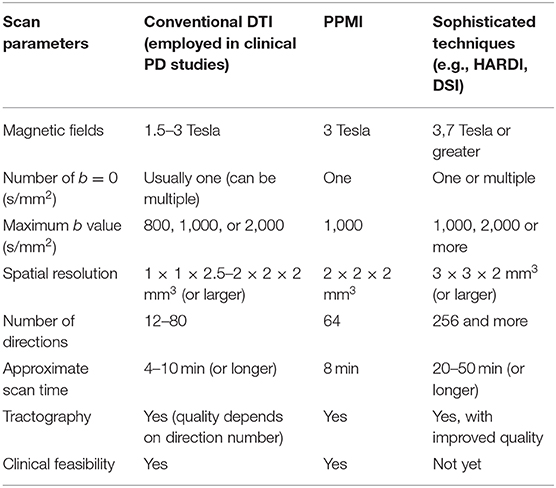
Table 5. Comparison of DTI scan parameters in conventional DTI, PPMI study, and advanced diffusion imaging techniques.
Variables Beyond Traditional DTI Indices
Beyond the commonly employed DTI metrics including FA and diffusivities, other quantitative indices that derived from special diffusion sequence or post-image modeling provide additional information on PD biomarker research. FW is an index generated from bi-tensor diffusion model (208) and estimates fractional volume of free water within a voxel without bias from directional dependence and cellular environmental restrictions. FW expresses atrophy-based microstructural degenerations and is also used to correct partial volume effects on FA and MD measures. FW has been found more sensitive than FA in identifying abnormalities in the SN (55), significantly correlated with UPDRS (157), and persistently increased in 1, 2, and 4 years of PD (161, 209). Diffusion kurtosis (210) is an index modeled from multiple b-values. Mean kurtosis (MK) estimates non-gaussian forms of diffusion and indicates microstructural complexity such as water leakage through cell membranes and myelin breakdown. It resolves the adverse effects of crossing-fibers, which may influence the accuracy the FA assessment, and thus provide better efficiency in diagnosing early PD (53, 211). More recently, there are increasing interests in the use of structural connectome measures to identify abnormal structural connectivity in PD or in PD motor-subtypes through network-based statistical analysis (57, 63, 212–217). The commonly used indices derived from DTI, together with their biological interpretations and clinical correlations in PD, are summarized in Table 2. Notably, none of the aforementioned variables are specific to PD neuropathology. Postmortem studies are compulsory to confirm these imaging-pathological correlations.
DTI in Gray Matter
Voxel-based whole brain analyses provide fully automated processing and become popular in DTI studies in PD. However, these analyses have been predominantly focused on white matter structures, because the approaches highly depend on reliable co-registration and spatial normalization methods, while most image registration algorisms used for DTI analyses are established based on white matter skeleton (such as TBSS) or FA intensity, and accompany a use of FA mask (usually sets at absolute FA > 0.2) to constrain DTI analyses within the white matter. These techniques provide substantial improvements of white matter registration, but the accuracy of gray matter registration is sacrificed. Although the motor dysfunctions in PD could be examined through white matter in the pyramidal and extrapyramidal motor pathways, the non-motor symptoms are primarily driven by abnormalities in the cortical and limbic subcortical gray matter (218). Specifically, cortical deficits can be presented in early PD with mild cognitive impairments (219). Therefore, identifying PD-related gray matter microstructural abnormality would be crucial, because it can alert treatment strategies and potentially prevent patients from developing to dementia. Recent technical advantages in DTI processing, including cortical surface-based (220) registration, such as using FreeSurfer (https://surfer.nmr.mgh.harvard.edu/), as well as gray matter skeleton-based registration, such as using Gray matter-based Spatial Statistics (GBSS) (221, 222), permit highly reliable DTI analyses of the gray matter. On the other hand, diffusivity variables are considered having a better sensitivity than FA in capturing neurodegenerative abnormalities in gray matter (223). Using a FreeSurfer registration, and intracortical MD measures of the PPMI data, two recent studies (224, 225) with cross-sectional and longitudinal (within 1-year follow up) analyses have found that compared to controls, PD patients had significantly increased MD in the frontal and occipital cortices; among PD patients these cortical MD changes correlated with worsened cognitive performance; furthermore, the posterior-cortical (i.e., medial temporal and temporo-occipital) MD significantly correlated with the increases of serum neurofilament light chain. These DTI studies in gray matter benefits the understanding of neuropathologic mechanisms underlying PD, especially those accompanied with cognitive impairments.
Conclusion
This review outlines the clinical utilities of the low-cost, non-invasive diffusion tensor MRI for biomarker of diagnosing PD, correlating PD symptomatology, assessing PD progression, and differentiating atypical types of parkinsonism. The robustness of the findings provides compelling evidences that DTI may be a promising marker for monitoring PD progressing and classifying atypical PD types. Therefore, it provides outcome measures to clinical trials and helps clinicians find better patient management. But the utility of DTI for diagnosing early PD is still challenging. Another merit is that, together with the findings on PPMI data, this review presented a divergent pattern of temporal FA changes in the earliest stage of PD. In particular, FA increase is pronounced in the young onset PD and in the earliest years of PD. These observations help to improve understanding of the pathophysiologic basis (e.g., the compensatory mechanisms, excitations of the inhibitory circuits) during the earliest stages of PD.
Limitations of clinical utilities of DTI shall be mentioned: (1) Because alterations of all DTI variables generally explain the non-specific biological features, they only permit indirect interpretations of the pathogenesis underlying PD. More investigations of imaging-pathological correlations are needed to corroborate a direct implication. Clinical DTI scans are subjected to low resolution, crossing-fibers, noise, and distortions. These factors affect a precise delineation of the key substructures [e.g., SNc, olfactory bulbs and tracts, locus coeruleus, nucleus accumbens, and small fiber connections in the brainstem (226)] that might be specific to PD pathology. Advanced diffusion techniques, such as high-resolution, high filed MRI, improved distortion-corrections, as well as solutions of fiber crossings, will lead to better qualities of DTI analyses, but it is rather important to develop clinically feasible parameters upon these novel techniques. (3) DTI variables are highly dependent on scan parameters, such as MRI field strength, number of encoding directions, and maximum b values. To finally benefit the individual definition and interventions of PD, DTI measures across different MRI centers need to be harmonized, and standardized cutoff points for these DTI measures need to be established.
Further research studies are required to address (1) whether anatomically specific DTI changes are related to the core motor symptom domains (e.g., tremor, rigidity, bradykinesia, gait or postural problems) of PD and how DTI could be able to distinguish these motor subtypes; (2) how DTI change is associated with PD-risks (e.g., prodromal syndromes, risk genes) and whether this DTI change could predict further conversions to PD; (3) whether combining DTI analyses with other imaging modalities (e.g., MRI volumetric and thickness analyses, task-based and resting-state functional MRI, DAT-SPECT and neuroimaging of dopamine or alpha-synuclein, etc.), and clinical evaluations (227) could improve precisions in diagnosing early PD; (4) whether the advanced DTI analyses help the understanding of the brainstem reticular circuits and their roles in the brainstem neurotransmitter systems and how they impact the PD non-motor features (e.g., autonomic syndromes, sleep difficult and sleep behavior problems, pain, fatigue, etc.); and (5) lastly, further validation studies need to take into account of the age of PD onset, disease duration, and whether the non-motor features (especially cognitive deficits) present at the same time as the motor features, whether the PD patients are examined with MRI under an ON or OFF medication status, as well as the history and dosage of medication.
Author Contributions
YZ: conception and research design, literature review, imaging data processing and analyses, results interpretation, and drafting the first manuscript. MB: conception and research design, results interpretation, language-editing, review, and critical revision of the manuscript. All authors contributed to the article and approved the submitted version.
Conflict of Interest
The authors declare that the research was conducted in the absence of any commercial or financial relationships that could be construed as a potential conflict of interest.
Acknowledgments
Data used in the preparation of this article were obtained from the Parkinson's Progression Markers Initiative (PPMI) database (www.ppmi-info.org/data). For up-to-date information on the study, visit www.ppmi-info.org. PPMI—a public-private partnership—is funded by the Michael J. Fox Foundation for Parkinson's Research and funding partners, including AbbVie, Avid Radiopharmaceuticals, Biogen, Bristol-Myers Squibb, Covance, GE Healthcare, Genentech, GlaxoSmithKline (GSK), Eli Lilly and Company, Lundbeck, Merck, Meso Scale Discovery (MSD), Pfizer, Piramal Imaging, Roche, Sanofi Genzyme, Servier, Teva, and UCB (www.ppmi-info.org/fundingpartners). We want to thank all patients, volunteers, and clinical staffs who participated the PPMI study. We thank Dr. Norbert Schuff for his previous leadership of the PPMI diffusion tensor imaging core. We thank Ms. I-Wei Wu for her previous work on collecting and coordinating the PPMI data.
Supplementary Material
The Supplementary Material for this article can be found online at: https://www.frontiersin.org/articles/10.3389/fneur.2020.531993/full#supplementary-material
References
1. de Lau LM, Breteler MM. Epidemiology of Parkinson's disease. Lancet Neurol. (2006) 5:525–35. doi: 10.1016/S1474-4422(06)70471-9
2. DeLong MR. Primate models of movement disorders of basal ganglia origin. Trends Neurosci. (1990) 13:281–5. doi: 10.1016/0166-2236(90)90110-V
3. Braak H, Ghebremedhin E, Rub U, Bratzke H, Del Tredici K. Stages in the development of Parkinson's disease-related pathology. Cell Tissue Res. (2004) 318:121–34. doi: 10.1007/s00441-004-0956-9
4. Saha AR, Hill J, Utton MA, Asuni AA, Ackerley S, Grierson AJ, et al. Parkinson's disease alpha-synuclein mutations exhibit defective axonal transport in cultured neurons. J Cell Sci. (2004) 117:1017–24. doi: 10.1242/jcs.00967
5. Braak H, Del Tredici K, Rub U, de Vos RA, Jansen Steur EN, Braak E. Staging of brain pathology related to sporadic Parkinson's disease. Neurobiol Aging. (2003) 24:197–211. doi: 10.1016/S0197-4580(02)00065-9
6. Moseley ME, Kucharczyk J, Asgari HS, Norman D. Anisotropy in diffusion-weighted MRI. Magn Reson Med. (1991) 19:321–6. doi: 10.1002/mrm.1910190222
7. Schuff N, Wu IW, Buckley S, Foster ED, Coffey CS, Gitelman DR, et al. Diffusion imaging of nigral alterations in early Parkinson's disease with dopaminergic deficits. Mov Disord. (2015) 30:1885–92. doi: 10.1002/mds.26325
8. Prakash BD, Sitoh YY, Tan LC, Au WL. Asymmetrical diffusion tensor imaging indices of the rostral substantia nigra in Parkinson's disease. Parkinsonism Relat Disord. (2012) 18:1029–33. doi: 10.1016/j.parkreldis.2012.05.021
9. Langley J, Huddleston DE, Merritt M, Chen X, McMurray R, Silver M, et al. Diffusion tensor imaging of the substantia nigra in Parkinson's disease revisited. Hum Brain Mapp. (2016) 37:2547–56. doi: 10.1002/hbm.23192
10. Modrego PJ, Fayed N, Artal J, Olmos S. Correlation of findings in advanced MRI techniques with global severity scales in patients with Parkinson disease. Acad Radiol. (2011) 18:235–41. doi: 10.1016/j.acra.2010.09.022
11. Zhan W, Kang GA, Glass GA, Zhang Y, Shirley C, Millin R, et al. Regional alterations of brain microstructure in Parkinson's disease using diffusion tensor imaging. Mov Disord. (2012) 27:90–7. doi: 10.1002/mds.23917
12. Wei X, Yan R, Chen Z, Weng R, Liu X, Gao H, et al. Combined diffusion tensor imaging and arterial spin labeling as markers of early parkinson's disease. Sci Rep. (2016) 6:33762. doi: 10.1038/srep33762
13. Zhang Y, Wu IW, Buckley S, Coffey CS, Foster E, Mendick S, et al. Diffusion tensor imaging of the nigrostriatal fibers in Parkinson's disease. Mov Disord. (2015) 30:1229–36. doi: 10.1002/mds.26251
14. Planetta PJ, Schulze ET, Geary EK, Corcos DM, Goldman JG, Little DM, et al. Thalamic projection fiber integrity in de novo Parkinson disease. AJNR Am J Neuroradiol. (2013) 34:74–9. doi: 10.3174/ajnr.A3178
15. Lenfeldt N, Hansson W, Larsson A, Nyberg L, Birgander R, Forsgren L. Diffusion tensor imaging and correlations to Parkinson rating scales. J Neurol. (2013) 260:2823–30. doi: 10.1007/s00415-013-7080-2
16. Chan LL, Ng KM, Rumpel H, Fook-Chong S, Li HH, Tan EK. Transcallosal diffusion tensor abnormalities in predominant gait disorder parkinsonism. Parkinsonism Relat Disord. (2014) 20:53–9. doi: 10.1016/j.parkreldis.2013.09.017
17. Youn J, Lee JM, Kwon H, Kim JS, Son TO, Cho JW. Alterations of mean diffusivity of pedunculopontine nucleus pathway in Parkinson's disease patients with freezing of gait. Parkinsonism Relat Disord. (2015) 21:12–7. doi: 10.1016/j.parkreldis.2014.10.003
18. Lenfeldt N, Holmlund H, Larsson A, Birgander R, Forsgren L. Frontal white matter injuries predestine gait difficulties in Parkinson's disease. Acta Neurol Scand. (2016) 134:210–8. doi: 10.1111/ane.12532
19. Surova Y, Lampinen B, Nilsson M, Latt J, Hall S, Widner H, et al. Alterations of diffusion kurtosis and neurite density measures in deep grey matter and white matter in parkinson's disease. PLoS ONE. (2016) 11:e0157755. doi: 10.1371/journal.pone.0157755
20. Matsui H, Nishinaka K, Oda M, Niikawa H, Komatsu K, Kubori T, et al. Wisconsin card sorting test in Parkinson's disease: diffusion tensor imaging. Acta Neurol Scand. (2007) 116:108–12. doi: 10.1111/j.1600-0404.2006.00795.x
21. Rae CL, Correia MM, Altena E, Hughes LE, Barker RA, Rowe JB. White matter pathology in Parkinson's disease: the effect of imaging protocol differences and relevance to executive function. Neuroimage. (2012) 62:1675–84. doi: 10.1016/j.neuroimage.2012.06.012
22. Gallagher C, Bell B, Bendlin B, Palotti M, Okonkwo O, Sodhi A, et al. White matter microstructural integrity and executive function in Parkinson's disease. J Int Neuropsychol Soc. (2013) 19:349–54. doi: 10.1017/S1355617712001373
23. Theilmann RJ, Reed JD, Song DD, Huang MX, Lee RR, Litvan I, et al. White-matter changes correlate with cognitive functioning in Parkinson's disease. Front Neurol. (2013) 4:37. doi: 10.3389/fneur.2013.00037
24. Chen YS, Chen MH, Lu CH, Chen PC, Chen HL, Yang IH, et al. Associations among cognitive functions, plasma DNA, and white matter integrity in patients with early-onset Parkinson's disease. Front Neurosci. (2017) 11:9. doi: 10.3389/fnins.2017.00009
25. Hattori T, Orimo S, Aoki S, Ito K, Abe O, Amano A, et al. Cognitive status correlates with white matter alteration in Parkinson's disease. Hum Brain Mapp. (2012) 33:727–39. doi: 10.1002/hbm.21245
26. Chen B, Fan GG, Liu H, Wang S. Changes in anatomical and functional connectivity of Parkinson's disease patients according to cognitive status. Eur J Radiol. (2015) 84:1318–24. doi: 10.1016/j.ejrad.2015.04.014
27. Li W, Liu J, Skidmore F, Liu Y, Tian J, Li K. White matter microstructure changes in the thalamus in Parkinson disease with depression: a diffusion tensor MR imaging study. AJNR Am J Neuroradiol. (2010) 31:1861–6. doi: 10.3174/ajnr.A2195
28. Huang P, Xu X, Gu Q, Xuan M, Yu X, Luo W, et al. Disrupted white matter integrity in depressed versus non-depressed Parkinson's disease patients: a tract-based spatial statistics study. J Neurol Sci. (2014) 346:145–8. doi: 10.1016/j.jns.2014.08.011
29. Baggio HC, Segura B, Ibarretxe-Bilbao N, Valldeoriola F, Marti MJ, Compta Y, et al. Structural correlates of facial emotion recognition deficits in Parkinson's disease patients. Neuropsychologia. (2012) 50:2121–8. doi: 10.1016/j.neuropsychologia.2012.05.020
30. Chiang PL, Chen HL, Lu CH, Chen PC, Chen MH, Yang IH, et al. White matter damage and systemic inflammation in Parkinson's disease. BMC Neurosci. (2017) 18:48. doi: 10.1186/s12868-017-0367-y
31. Haghshomar M, Rahmani F, Hadi Aarabi M, Shahjouei S, Sobhani S, Rahmani M. White matter changes correlates of peripheral neuroinflammation in patients with Parkinson's disease. Neuroscience. (2019) 403:70–8. doi: 10.1016/j.neuroscience.2017.10.050
32. Matsui H, Nishinaka K, Oda M, Niikawa H, Komatsu K, Kubori T, et al. Disruptions of the fornix fiber in Parkinsonian patients with excessive daytime sleepiness. Parkinsonism Relat Disord. (2006) 12:319–22. doi: 10.1016/j.parkreldis.2006.01.007
33. Pyatigorskaya N, Mongin M, Valabregue R, Yahia-Cherif L, Ewenczyk C, Poupon C, et al. Medulla oblongata damage and cardiac autonomic dysfunction in Parkinson disease. Neurology. (2016) 87:2540–5. doi: 10.1212/WNL.0000000000003426
34. Ibarretxe-Bilbao N, Junque C, Marti MJ, Valldeoriola F, Vendrell P, Bargallo N, et al. Olfactory impairment in Parkinson's disease and white matter abnormalities in central olfactory areas: a voxel-based diffusion tensor imaging study. Mov Disord. (2010) 25:1888–94. doi: 10.1002/mds.23208
35. Sobhani S, Rahmani F, Aarabi MH, Sadr AV. Exploring white matter microstructure and olfaction dysfunction in early parkinson disease: diffusion MRI reveals new insight. Brain Imaging Behav. (2019) 13:210–9. doi: 10.1007/s11682-017-9781-0
36. Scherfler C, Esterhammer R, Nocker M, Mahlknecht P, Stockner H, Warwitz B, et al. Correlation of dopaminergic terminal dysfunction and microstructural abnormalities of the basal ganglia and the olfactory tract in Parkinson's disease. Brain. (2013) 136:3028–37. doi: 10.1093/brain/awt234
37. Wang J, Yang QX, Sun X, Vesek J, Mosher Z, Vasavada M, et al. MRI evaluation of asymmetry of nigrostriatal damage in the early stage of early-onset Parkinson's disease. Parkinsonism Relat Disord. (2015) 21:590–6. doi: 10.1016/j.parkreldis.2015.03.012
38. Esterhammer R, Seppi K, Reiter E, Pinter B, Mueller C, Kremser C, et al. Potential of diffusion tensor imaging and relaxometry for the detection of specific pathological alterations in Parkinson's Disease (PD). PLoS ONE. (2015) 10:e0145493. doi: 10.1371/journal.pone.0145493
39. Wen MC, Heng HS, Ng SY, Tan LC, Chan LL, Tan EK. White matter microstructural characteristics in newly diagnosed Parkinson's disease: an unbiased whole-brain study. Sci Rep. (2016) 6:35601. doi: 10.1038/srep35601
40. Iseki K, Fukuyama H, Oishi N, Tomimoto H, Otsuka Y, Nankaku M, et al. Freezing of gait and white matter changes: a tract-based spatial statistics study. J Clin Mov Disord. (2015) 2:1. doi: 10.1186/s40734-014-0011-2
41. Luo C, Song W, Chen Q, Yang J, Gong Q, Shang HF. White matter microstructure damage in tremor-dominant Parkinson's disease patients. Neuroradiology. (2017) 59:691–8. doi: 10.1007/s00234-017-1846-7
42. Vervoort G, Leunissen I, Firbank M, Heremans E, Nackaerts E, Vandenberghe W, et al. Structural brain alterations in motor subtypes of Parkinson's disease: evidence from probabilistic tractography and shape analysis. PLoS ONE. (2016) 11:e0157743. doi: 10.1371/journal.pone.0157743
43. Koshimori Y, Segura B, Christopher L, Lobaugh N, Duff-Canning S, Mizrahi R, et al. Imaging changes associated with cognitive abnormalities in Parkinson's disease. Brain Struct Funct. (2015) 220:2249–61. doi: 10.1007/s00429-014-0785-x
44. Duncan GW, Firbank MJ, Yarnall AJ, Khoo TK, Brooks DJ, Barker RA, et al. Gray and white matter imaging: a biomarker for cognitive impairment in early Parkinson's disease? Mov Disord. (2016) 31:103–110. doi: 10.1002/mds.26312
45. Melzer TR, Watts R, MacAskill MR, Pitcher TL, Livingston L, Keenan RJ, et al. White matter microstructure deteriorates across cognitive stages in Parkinson disease. Neurology. (2013) 80:1841–9. doi: 10.1212/WNL.0b013e3182929f62
46. Zheng Z, Shemmassian S, Wijekoon C, Kim W, Bookheimer SY, Pouratian N. DTI correlates of distinct cognitive impairments in Parkinson's disease. Hum Brain Mapp. (2014) 35:1325–33. doi: 10.1002/hbm.22256
47. Kantarci K, Senjem ML, Avula R, Zhang B, Samikoglu AR, Weigand SD, et al. Diffusion tensor imaging and cognitive function in older adults with no dementia. Neurology. (2011) 77:26–34. doi: 10.1212/WNL.0b013e31822313dc
48. Carlesimo GA, Piras F, Assogna F, Pontieri FE, Caltagirone C, Spalletta G. Hippocampal abnormalities and memory deficits in Parkinson disease: a multimodal imaging study. Neurology. (2012) 78:1939–45. doi: 10.1212/WNL.0b013e318259e1c5
49. Haehner A, Schopf V, Loureiro A, Linn J, Reichmann H, Hummel T, et al. Substantia nigra fractional anisotropy changes confirm the PD at-risk status of patients with idiopathic smell loss. Parkinsonism Relat Disord. (2018) 50:113–6. doi: 10.1016/j.parkreldis.2018.02.026
50. Guimaraes RP, Campos BM, de Rezende TJ, Piovesana L, Azevedo PC, Amato-Filho AC, et al. Is diffusion tensor imaging a good biomarker for early Parkinson's disease? Front Neurol. (2018) 9:626. doi: 10.3389/fneur.2018.00626
51. Auning E, Kjaervik VK, Selnes P, Aarsland D, Haram A, Bjornerud A, et al. White matter integrity and cognition in Parkinson's disease: a cross-sectional study. BMJ Open. (2014) 4:e003976. doi: 10.1136/bmjopen-2013-003976
52. Gargouri F, Gallea C, Mongin M, Pyatigorskaya N, Valabregue R, Ewenczyk C, et al. Multimodal magnetic resonance imaging investigation of basal forebrain damage and cognitive deficits in Parkinson's disease. Mov Disord. (2019) 34:516–25. doi: 10.1002/mds.27561
53. Zhang G, Zhang Y, Zhang C, Wang Y, Ma G, Nie K, et al. Diffusion kurtosis imaging of substantia nigra is a sensitive method for early diagnosis and disease evaluation in Parkinson's disease. Parkinsons Dis. (2015) 2015:207624. doi: 10.1155/2015/207624
54. Kamagata K, Zalesky A, Hatano T, Ueda R, Di Biase MA, Okuzumi A, et al. Gray matter abnormalities in idiopathic Parkinson's disease: evaluation by diffusional kurtosis imaging and neurite orientation dispersion and density imaging. Hum Brain Mapp. (2017) 38:3704–22. doi: 10.1002/hbm.23628
55. Ofori E, Pasternak O, Planetta PJ, Burciu R, Snyder A, Febo M, et al. Increased free water in the substantia nigra of Parkinson's disease: a single-site and multi-site study. Neurobiol Aging. (2015) 36:1097–104. doi: 10.1016/j.neurobiolaging.2014.10.029
56. Planetta PJ, Ofori E, Pasternak O, Burciu RG, Shukla P, DeSimone JC, et al. Free-water imaging in Parkinson's disease and atypical parkinsonism. Brain. (2016) 139:495–508. doi: 10.1093/brain/awv361
57. Kim M, Park H. Structural connectivity profile of scans without evidence of dopaminergic deficit (SWEDD) patients compared to normal controls and Parkinson's disease patients. Springerplus. (2016) 5:1421. doi: 10.1186/s40064-016-3110-8
58. Wen MC, Xu Z, Lu Z, Chan LL, Tan EK, Tan LCS. Microstructural network alterations of olfactory dysfunction in newly diagnosed Parkinson's disease. Sci Rep. (2017) 7:12559. doi: 10.1038/s41598-017-12947-7
59. Tan WQ, Yeoh CS, Rumpel H, Nadkarni N, Lye WK, Tan EK, et al. Deterministic tractography of the nigrostriatal-nigropallidal pathway in parkinson's disease. Sci Rep. (2015) 5:17283. doi: 10.1038/srep17283
60. Son SJ, Kim M, Park H. Imaging analysis of Parkinson's disease patients using SPECT and tractography. Sci Rep. (2016) 6:38070. doi: 10.1038/srep38070
61. Kamagata K, Hatano T, Okuzumi A, Motoi Y, Abe O, Shimoji K, et al. Neurite orientation dispersion and density imaging in the substantia nigra in idiopathic Parkinson disease. Eur Radiol. (2016) 26:2567–77. doi: 10.1007/s00330-015-4066-8
62. Kamagata K, Zalesky A, Hatano T, Di Biase MA, El Samad O, Saiki S, et al. Connectome analysis with diffusion MRI in idiopathic Parkinson's disease: evaluation using multi-shell, multi-tissue, constrained spherical deconvolution. Neuroimage Clin. (2018) 17:518–29. doi: 10.1016/j.nicl.2017.11.007
63. Abbasi N, Mohajer B, Abbasi S, Hasanabadi P, Abdolalizadeh A, Rajimehr R. Relationship between cerebrospinal fluid biomarkers and structural brain network properties in Parkinson's disease. Mov Disord. (2018) 33:431–9. doi: 10.1002/mds.27284
64. Basser PJ, Jones DK. Diffusion-tensor MRI. Theory, experimental design and data analysis - a technical review. NMR Biomed. (2002) 15:456–67. doi: 10.1002/nbm.783
65. Pierpaoli C, Jezzard P, Basser PJ, Barnett A, Di Chiro G. Diffusion tensor MR imaging of the human brain. Radiology. (1996) 201:637–48. doi: 10.1148/radiology.201.3.8939209
66. Song SK, Sun SW, Ramsbottom MJ, Chang C, Russell J, Cross AH. Dysmyelination revealed through MRI as increased radial (but unchanged axial) diffusion of water. Neuroimage. (2002) 17:1429–36. doi: 10.1006/nimg.2002.1267
67. Kim JH, Budde MD, Liang HF, Klein RS, Russell JH, Cross AH, et al. Detecting axon damage in spinal cord from a mouse model of multiple sclerosis. Neurobiol Dis. (2006) 21:626–32. doi: 10.1016/j.nbd.2005.09.009
68. Wheeler-Kingshott CA, Cercignani M. About “axial” and “radial” diffusivities. Magn Reson Med. (2009) 61:1255–60. doi: 10.1002/mrm.21965
69. Acosta-Cabronero J, Williams GB, Pengas G, Nestor PJ. Absolute diffusivities define the landscape of white matter degeneration in Alzheimer's disease. Brain. (2010) 133:529–39. doi: 10.1093/brain/awp257
70. Thomalla G, Glauche V, Koch MA, Beaulieu C, Weiller C, Rother J. Diffusion tensor imaging detects early Wallerian degeneration of the pyramidal tract after ischemic stroke. Neuroimage. (2004) 22:1767–74. doi: 10.1016/j.neuroimage.2004.03.041
71. Boska MD, Hasan KM, Kibuule D, Banerjee R, McIntyre E, Nelson JA, et al. Quantitative diffusion tensor imaging detects dopaminergic neuronal degeneration in a murine model of Parkinson's disease. Neurobiol Dis. (2007) 26:590–6. doi: 10.1016/j.nbd.2007.02.010
72. Karagulle Kendi AT, Lehericy S, Luciana M, Ugurbil K, Tuite P. Altered diffusion in the frontal lobe in Parkinson disease. AJNR Am J Neuroradiol. (2008) 29:501–5. doi: 10.3174/ajnr.A0850
73. Tessa C, Giannelli M, Della Nave R, Lucetti C, Berti C, Ginestroni A, et al. A whole-brain analysis in de novo Parkinson disease. AJNR Am J Neuroradiol. (2008) 29:674–80. doi: 10.3174/ajnr.A0900
74. Soares JM, Marques P, Alves V, Sousa N. A hitchhiker's guide to diffusion tensor imaging. Front Neurosci. (2013) 7:31. doi: 10.3389/fnins.2013.00031
75. Smith SM, Jenkinson M, Johansen-Berg H, Rueckert D, Nichols TE, Mackay CE, et al. Tract-based spatial statistics: voxelwise analysis of multi-subject diffusion data. Neuroimage. (2006) 31:1487–505. doi: 10.1016/j.neuroimage.2006.02.024
76. Zhang K, Yu C, Zhang Y, Wu X, Zhu C, Chan P, et al. Voxel-based analysis of diffusion tensor indices in the brain in patients with Parkinson's disease. Eur J Radiol. (2011) 77:269–73. doi: 10.1016/j.ejrad.2009.07.032
77. Kim HJ, Kim SJ, Kim HS, Choi CG, Kim N, Han S, et al. Alterations of mean diffusivity in brain white matter and deep gray matter in Parkinson's disease. Neurosci Lett. (2013) 550:64–8. doi: 10.1016/j.neulet.2013.06.050
78. Diez-Cirarda M, Ojeda N, Pena J, Cabrera-Zubizarreta A, Gomez-Beldarrain MA, Gomez-Esteban JC, et al. Neuroanatomical correlates of theory of mind deficit in Parkinson's disease: a multimodal imaging study. PLoS ONE. (2015) 10:e0142234. doi: 10.1371/journal.pone.0142234
79. Jiang MF, Shi F, Niu GM, Xie SH, Yu SY. A novel method for evaluating brain function and microstructural changes in Parkinson's disease. Neural Regen Res. (2015) 10:2025–2032. doi: 10.4103/1673-5374.172322
80. Skidmore FM, Spetsieris PG, Anthony T, Cutter GR, von Deneen KM, Liu Y, et al. A full-brain, bootstrapped analysis of diffusion tensor imaging robustly differentiates Parkinson disease from healthy controls. Neuroinformatics. (2015) 13:7–18. doi: 10.1007/s12021-014-9222-9
81. Vercruysse S, Leunissen I, Vervoort G, Vandenberghe W, Swinnen S, Nieuwboer A. Microstructural changes in white matter associated with freezing of gait in Parkinson's disease. Mov Disord. (2015) 30:567–76. doi: 10.1002/mds.26130
82. Yoo HB, Lee JY, Lee JS, Kang H, Kim YK, Song IC, et al. Whole-brain diffusion-tensor changes in parkinsonian patients with impulse control disorders. J Clin Neurol. (2015) 11:42–7. doi: 10.3988/jcn.2015.11.1.42
83. Price CC, Tanner J, Nguyen PT, Schwab NA, Mitchell S, Slonena E, et al. Gray and white matter contributions to cognitive frontostriatal deficits in non-demented Parkinson's disease. PLoS ONE. (2016) 11:e0147332. doi: 10.1371/journal.pone.0147332
84. Lim JS, Shin SA, Lee JY, Nam H, Lee JY, Kim YK. Neural substrates of rapid eye movement sleep behavior disorder in Parkinson's disease. Parkinsonism Relat Disord. (2016) 23:31–36. doi: 10.1016/j.parkreldis.2015.11.027
85. Mole JP, Subramanian L, Bracht T, Morris H, Metzler-Baddeley C, Linden DE. Increased fractional anisotropy in the motor tracts of Parkinson's disease suggests compensatory neuroplasticity or selective neurodegeneration. Eur Radiol. (2016) 26:3327–35. doi: 10.1007/s00330-015-4178-1
86. Wang M, Jiang S, Yuan Y, Zhang L, Ding J, Wang J, et al. Alterations of functional and structural connectivity of freezing of gait in Parkinson's disease. J Neurol. (2016) 263:1583–92. doi: 10.1007/s00415-016-8174-4
87. Canu E, Agosta F, Markovic V, Petrovic I, Stankovic I, Imperiale F, et al. White matter tract alterations in Parkinson's disease patients with punding. Parkinsonism Relat Disord. (2017) 43:85–91. doi: 10.1016/j.parkreldis.2017.07.025
88. Chen B, Fan G, Sun W, Shang X, Shi S, Wang S, et al. Usefulness of diffusion-tensor MRI in the diagnosis of Parkinson variant of multiple system atrophy and Parkinson's disease: a valuable tool to differentiate between them? Clin Radiol. (2017) 72:610 e619–e15. doi: 10.1016/j.crad.2017.02.005
89. Cousineau M, Jodoin PM, Morency FC, Rozanski V, Grand'Maison M, Bedell BJ, et al. A test-retest study on Parkinson's PPMI dataset yields statistically significant white matter fascicles. Neuroimage Clin. (2017) 16:222–33. doi: 10.1016/j.nicl.2017.07.020
90. Georgiopoulos C, Warntjes M, Dizdar N, Zachrisson H, Engstrom M, Haller S, et al. Olfactory impairment in parkinson's disease studied with diffusion tensor and magnetization transfer imaging. J Parkinsons Dis. (2017) 7:301–11. doi: 10.3233/JPD-161060
91. Lee WW, Yoon EJ, Lee JY, Park SW, Kim YK. Visual hallucination and pattern of brain degeneration in Parkinson's disease. Neurodegener Dis. (2017) 17:63–72. doi: 10.1159/000448517
92. Chen NK, Chou YH, Sundman M, Hickey P, Kasoff WS, Bernstein A, et al. Alteration of diffusion-tensor magnetic resonance imaging measures in brain regions involved in early stages of Parkinson's disease. Brain Connect. (2018) 8:343–9. doi: 10.1089/brain.2017.0558
93. Li XR, Ren YD, Cao B, Huang XL. Analysis of white matter characteristics with tract-based spatial statistics according to diffusion tensor imaging in early Parkinson's disease. Neurosci Lett. (2018) 675:127–32. doi: 10.1016/j.neulet.2017.11.064
94. Minett T, Su L, Mak E, Williams G, Firbank M, Lawson RA, et al. Longitudinal diffusion tensor imaging changes in early Parkinson's disease: ICICLE-PD study. J Neurol. (2018) 265:1528–39. doi: 10.1007/s00415-018-8873-0
95. Pietracupa S, Suppa A, Upadhyay N, Gianni C, Grillea G, Leodori G, et al. Freezing of gait in Parkinson's disease: gray and white matter abnormalities. J Neurol. (2018) 265:52–62. doi: 10.1007/s00415-017-8654-1
96. Rektor I, Svatkova A, Vojtisek L, Zikmundova I, Vanicek J, Kiraly A, et al. White matter alterations in Parkinson's disease with normal cognition precede grey matter atrophy. PLoS ONE. (2018) 13:e0187939. doi: 10.1371/journal.pone.0187939
97. Taylor KI, Sambataro F, Boess F, Bertolino A, Dukart J. Progressive decline in gray and white matter integrity in de novo parkinson's disease: an analysis of longitudinal parkinson progression markers initiative diffusion tensor imaging data. Front Aging Neurosci. (2018) 10:318. doi: 10.3389/fnagi.2018.00318
98. Wen MC, Heng HSE, Lu Z, Xu Z, Chan LL, Tan EK, et al. Differential white matter regional alterations in motor subtypes of early drug-naive parkinson's disease patients. Neurorehabil Neural Repair. (2018) 32:129–41. doi: 10.1177/1545968317753075
99. Guan X, Huang P, Zeng Q, Liu C, Wei H, Xuan M, et al. Quantitative susceptibility mapping as a biomarker for evaluating white matter alterations in Parkinson's disease. Brain Imaging Behav. (2019) 13:220–31. doi: 10.1007/s11682-018-9842-z
100. Movement Disorder Society Task Force on Rating Scales for Parkinson's D. The Unified Parkinson's Disease Rating Scale (UPDRS): status and recommendations. Mov Disord. (2003) 18:738–50. doi: 10.1002/mds.10473
101. Goetz CG, Fahn S, Martinez-Martin P, Poewe W, Sampaio C, Stebbins GT, et al. Movement Disorder Society-sponsored revision of the Unified Parkinson's Disease Rating Scale (MDS-UPDRS): Process, format, and clinimetric testing plan. Mov Disord. (2007) 22:41–7. doi: 10.1002/mds.21198
102. Hall JM, Ehgoetz Martens KA, Walton CC, O'Callaghan C, Keller PE, Lewis SJ, et al. Diffusion alterations associated with Parkinson's disease symptomatology: a review of the literature. Parkinsonism Relat Disord. (2016) 33:12–26. doi: 10.1016/j.parkreldis.2016.09.026
103. Marek K. The Parkinson Progression Marker Initiative (PPMI). Prog Neurobiol. (2011) 95:629–35. doi: 10.1016/j.pneurobio.2011.09.005
104. Schwarz ST, Abaei M, Gontu V, Morgan PS, Bajaj N, Auer DP. Diffusion tensor imaging of nigral degeneration in Parkinson's disease: a region-of-interest and voxel-based study at 3 T and systematic review with meta-analysis. Neuroimage Clin. (2013) 3:481–8. doi: 10.1016/j.nicl.2013.10.006
105. Jeurissen B, Leemans A, Tournier JD, Jones DK, Sijbers J. Investigating the prevalence of complex fiber configurations in white matter tissue with diffusion magnetic resonance imaging. Hum Brain Mapp. (2013) 34:2747–66. doi: 10.1002/hbm.22099
106. Mori S. Introduction to Diffusion Tensor Imaging. 1st Edn. Elsevier Science (2007). doi: 10.1016/b978-0-444-52828-5.x5014-5
107. Atkinson-Clement C, Pinto S, Eusebio A, Coulon O. Diffusion tensor imaging in Parkinson's disease: review and meta-analysis. Neuroimage Clin. (2017) 16:98–110. doi: 10.1016/j.nicl.2017.07.011
108. Baudrexel S, Witte T, Seifried C, von Wegner F, Beissner F, Klein JC, et al. Resting state fMRI reveals increased subthalamic nucleus-motor cortex connectivity in Parkinson's disease. Neuroimage. (2011) 55:1728–38. doi: 10.1016/j.neuroimage.2011.01.017
109. Melzer TR, Watts R, MacAskill MR, Pearson JF, Rueger S, Pitcher TL, et al. Arterial spin labelling reveals an abnormal cerebral perfusion pattern in Parkinson's disease. Brain. (2011) 134:845–55. doi: 10.1093/brain/awq377
110. Ma Y, Tang C, Spetsieris PG, Dhawan V, Eidelberg D. Abnormal metabolic network activity in Parkinson's disease: test-retest reproducibility. J Cereb Blood Flow Metab. (2007) 27:597–605. doi: 10.1038/sj.jcbfm.9600358
111. Collier TJ, Lipton J, Daley BF, Palfi S, Chu Y, Sortwell C, et al. Aging-related changes in the nigrostriatal dopamine system and the response to MPTP in nonhuman primates: diminished compensatory mechanisms as a prelude to parkinsonism. Neurobiol Dis. (2007) 26:56–65. doi: 10.1016/j.nbd.2006.11.013
112. Hindle JV. Ageing, neurodegeneration and Parkinson's disease. Age Ageing. (2010) 39:156–61. doi: 10.1093/ageing/afp223
113. Nagae LM, Honce JM, Tanabe J, Shelton E, Sillau SH, Berman BD. Microstructural changes within the basal ganglia differ between Parkinson disease subtypes. Front Neuroanat. (2016) 10:17. doi: 10.3389/fnana.2016.00017
114. Alexander GE. Biology of Parkinson's disease: pathogenesis and pathophysiology of a multisystem neurodegenerative disorder. Dialogues Clin Neurosci. (2004) 6:259–80.
116. Cheng HC, Ulane CM, Burke RE. Clinical progression in Parkinson disease and the neurobiology of axons. Ann Neurol. (2010) 67:715–25. doi: 10.1002/ana.21995
117. Peran P, Cherubini A, Assogna F, Piras F, Quattrocchi C, Peppe A, et al. Magnetic resonance imaging markers of Parkinson's disease nigrostriatal signature. Brain. (2010) 133:3423–33. doi: 10.1093/brain/awq212
118. Du G, Lewis MM, Styner M, Shaffer ML, Sen S, Yang QX, et al. Combined R2* and diffusion tensor imaging changes in the substantia nigra in Parkinson's disease. Mov Disord. (2011) 26:1627–32. doi: 10.1002/mds.23643
119. Joshi N, Rolheiser TM, Fisk JD, McKelvey JR, Schoffer K, Phillips G, et al. Lateralized microstructural changes in early-stage Parkinson's disease in anterior olfactory structures, but not in substantia nigra. J Neurol. (2017) 264:1497–505. doi: 10.1007/s00415-017-8555-3
120. Yoshikawa K, Nakata Y, Yamada K, Nakagawa M. Early pathological changes in the parkinsonian brain demonstrated by diffusion tensor MRI. J Neurol Neurosurg Psychiatr. (2004) 75:481–4. doi: 10.1136/jnnp.2003.021873
121. Chan LL, Rumpel H, Yap K, Lee E, Loo HV, Ho GL, et al. Case control study of diffusion tensor imaging in Parkinson's disease. J Neurol Neurosurg Psychiatr. (2007) 78:1383–6. doi: 10.1136/jnnp.2007.121525
122. Rolheiser TM, Fulton HG, Good KP, Fisk JD, McKelvey JR, Scherfler C, et al. Diffusion tensor imaging and olfactory identification testing in early-stage Parkinson's disease. J Neurol. (2011) 258:1254–60. doi: 10.1007/s00415-011-5915-2
123. Wang JJ, Lin WY, Lu CS, Weng YH, Ng SH, Wang CH, et al. Parkinson disease: diagnostic utility of diffusion kurtosis imaging. Radiology. (2011) 261:210–7. doi: 10.1148/radiol.11102277
124. Skorpil M, Soderlund V, Sundin A, Svenningsson P. MRI diffusion in Parkinson's disease: using the technique's inherent directional information to study the olfactory bulb and substantia nigra. J Parkinsons Dis. (2012) 2:171–80. doi: 10.3233/JPD-2012-12091
125. Perea RD, Rada RC, Wilson J, Vidoni ED, Morris JK, Lyons KE, et al. A comparative white matter study with Parkinson's disease, Parkinson's disease with dementia and Alzheimer's disease. J Alzheimers Dis Parkinsonism. (2013) 3:123. doi: 10.4172/2161-0460.1000123
126. Ji L, Wang Y, Zhu D, Liu W, Shi J. White matter differences between multiple system atrophy (parkinsonian type) and Parkinson's disease: a diffusion tensor image study. Neuroscience. (2015) 305:109–16. doi: 10.1016/j.neuroscience.2015.07.060
127. Loane C, Politis M, Kefalopoulou Z, Valle-Guzman N, Paul G, Widner H, et al. Aberrant nigral diffusion in Parkinson's disease: a longitudinal diffusion tensor imaging study. Mov Disord. (2016) 31:1020–6. doi: 10.1002/mds.26606
128. Gattellaro G, Minati L, Grisoli M, Mariani C, Carella F, Osio M, et al. White matter involvement in idiopathic Parkinson disease: a diffusion tensor imaging study. AJNR Am J Neuroradiol. (2009) 30:1222–6. doi: 10.3174/ajnr.A1556
129. Vaillancourt DE, Spraker MB, Prodoehl J, Abraham I, Corcos DM, Zhou XJ, et al. High-resolution diffusion tensor imaging in the substantia nigra of de novo Parkinson disease. Neurology. (2009) 72:1378–84. doi: 10.1212/01.wnl.0000340982.01727.6e
130. Lenfeldt N, Larsson A, Nyberg L, Birgander R, Forsgren L. Fractional anisotropy in the substantia nigra in Parkinson's disease: a complex picture. Eur J Neurol. (2015) 22:1408–14. doi: 10.1111/ene.12760
131. Hirata FCC, Sato JR, Vieira G, Lucato LT, Leite CC, Bor-Seng-Shu E, et al. Substantia nigra fractional anisotropy is not a diagnostic biomarker of Parkinson's disease: a diagnostic performance study and meta-analysis. Eur Radiol. (2017) 27:2640–8. doi: 10.1007/s00330-016-4611-0
132. Knossalla F, Kohl Z, Winkler J, Schwab S, Schenk T, Engelhorn T, et al. High-resolution diffusion tensor-imaging indicates asymmetric microstructural disorganization within substantia nigra in early Parkinson's disease. J Clin Neurosci. (2018) 50:199–202. doi: 10.1016/j.jocn.2018.01.023
133. Du G, Lewis MM, Sen S, Wang J, Shaffer ML, Styner M, et al. Imaging nigral pathology and clinical progression in Parkinson's disease. Mov Disord. (2012) 27:1636–43. doi: 10.1002/mds.25182
134. Menke RA, Jbabdi S, Miller KL, Matthews PM, Zarei M. Connectivity-based segmentation of the substantia nigra in human and its implications in Parkinson's disease. Neuroimage. (2010) 52:1175–80. doi: 10.1016/j.neuroimage.2010.05.086
135. Deng XY, Wang L, Yang TT, Li R, Yu G. A meta-analysis of diffusion tensor imaging of substantia nigra in patients with Parkinson's disease. Sci Rep. (2018) 8:2941. doi: 10.1038/s41598-018-20076-y
136. Cochrane CJ, Ebmeier KP. Diffusion tensor imaging in parkinsonian syndromes: a systematic review and meta-analysis. Neurology. (2013) 80:857–64. doi: 10.1212/WNL.0b013e318284070c
137. Fearnley JM, Lees AJ. Ageing and Parkinson's disease: substantia nigra regional selectivity. Brain. (1991) 114:2283–301. doi: 10.1093/brain/114.5.2283
138. Rodriguez-Oroz MC, Jahanshahi M, Krack P, Litvan I, Macias R, Bezard E, et al. Initial clinical manifestations of Parkinson's disease: features and pathophysiological mechanisms. Lancet Neurol. (2009) 8:1128–39. doi: 10.1016/S1474-4422(09)70293-5
139. Lau A, So RWL, Lau HHC, Sang JC, Ruiz-Riquelme A, Fleck SC, et al. alpha-Synuclein strains target distinct brain regions and cell types. Nat Neurosci. (2020) 23:21–31. doi: 10.1038/s41593-019-0541-x
140. McColgan P, Joubert J, Tabrizi SJ, Rees G. The human motor cortex microcircuit: insights for neurodegenerative disease. Nat Rev Neurosci. (2020) 21:401–5. doi: 10.1038/s41583-020-0315-1
141. Westbrook A, van den Bosch R, Maatta JI, Hofmans L, Papadopetraki D, Cools R, et al. Dopamine promotes cognitive effort by biasing the benefits versus costs of cognitive work. Science. (2020) 367:1362–6. doi: 10.1126/science.aaz5891
142. Lehericy S, Ducros M, Van de Moortele PF, Francois C, Thivard L, Poupon C, et al. Diffusion tensor fiber tracking shows distinct corticostriatal circuits in humans. Ann Neurol. (2004) 55:522–9. doi: 10.1002/ana.20030
143. Sedrak M, Gorgulho A, Bari A, Behnke E, Frew A, Gevorkyan I, et al. Diffusion tensor imaging (DTI) and colored fractional anisotropy (FA) mapping of the subthalamic nucleus (STN) and the globus pallidus interna (GPi). Acta Neurochir. (2010) 152:2079–84. doi: 10.1007/s00701-010-0813-4
144. Hauptman JS, DeSalles AA, Espinoza R, Sedrak M, Ishida W. Potential surgical targets for deep brain stimulation in treatment-resistant depression. Neurosurg Focus. (2008) 25:E3. doi: 10.3171/FOC/2008/25/7/E3
145. Andica C, Kamagata K, Hatano T, Okuzumi A, Saito A, Nakazawa M, et al. Neurite orientation dispersion and density imaging of the nigrostriatal pathway in Parkinson's disease: retrograde degeneration observed by tract-profile analysis. Parkinsonism Relat Disord. (2018) 51:55–60. doi: 10.1016/j.parkreldis.2018.02.046
146. Theisen F, Leda R, Pozorski V, Oh JM, Adluru N, Wong R, et al. Evaluation of striatonigral connectivity using probabilistic tractography in Parkinson's disease. Neuroimage Clin. (2017) 16:557–63. doi: 10.1016/j.nicl.2017.09.009
147. Menke RA, Scholz J, Miller KL, Deoni S, Jbabdi S, Matthews PM, et al. MRI characteristics of the substantia nigra in Parkinson's disease: a combined quantitative T1 and DTI study. Neuroimage. (2009) 47:435–41. doi: 10.1016/j.neuroimage.2009.05.017
148. Scherfler C, Schocke MF, Seppi K, Esterhammer R, Brenneis C, Jaschke W, et al. Voxel-wise analysis of diffusion weighted imaging reveals disruption of the olfactory tract in Parkinson's disease. Brain. (2006) 129:538–42. doi: 10.1093/brain/awh674
149. Mentzel TQ, Lieverse R, Levens A, Mentzel CL, Tenback DE, Bakker PR, et al. Reliability and validity of an instrument for the assessment of bradykinesia. Psychiatr Res. (2016) 238:189–95. doi: 10.1016/j.psychres.2016.02.011
150. Yao N, Cheung C, Pang S, Shek-kwan Chang R, Lau KK, Suckling J, et al. Multimodal MRI of the hippocampus in Parkinson's disease with visual hallucinations. Brain Struct Funct. (2016) 221:287–300. doi: 10.1007/s00429-014-0907-5
151. Buchert R, Lange C, Spehl TS, Apostolova I, Frings L, Jonsson C, et al. Diagnostic performance of the specific uptake size index for semi-quantitative analysis of I-123-FP-CIT SPECT. Harmonized multi-center research setting versus typical clinical single-camera setting. EJNMMI Res. (2019) 9:37. doi: 10.1186/s13550-019-0506-9
152. Jakobson Mo S, Axelsson J, Jonasson L, Larsson A, Ogren MJ, Ogren M, et al. Dopamine transporter imaging with [(18)F]FE-PE2I PET and [(123)I]FP-CIT SPECT-a clinical comparison. EJNMMI Res. (2018) 8:100. doi: 10.1186/s13550-018-0450-0
153. Prashanth R, Roy SD, Mandal PK, Ghosh S. High-accuracy classification of Parkinson's disease through shape analysis and surface fitting in 123I-Ioflupane SPECT imaging. IEEE J Biomed Health Inform. (2017) 21:794–802. doi: 10.1109/JBHI.2016.2547901
154. Augimeri A, Cherubini A, Cascini GL, Galea D, Caligiuri ME, Barbagallo G, et al. CADA-computer-aided DaTSCAN analysis. EJNMMI Phys. (2016) 3:4. doi: 10.1186/s40658-016-0140-9
155. Kraemmer J, Kovacs GG, Perju-Dumbrava L, Pirker S, Traub-Weidinger T, Pirker W. Correlation of striatal dopamine transporter imaging with post mortem substantia nigra cell counts. Mov Disord. (2014) 29:1767–73. doi: 10.1002/mds.25975
156. Walker Z, Jaros E, Walker RW, Lee L, Costa DC, Livingston G, et al. Dementia with Lewy bodies: a comparison of clinical diagnosis, FP-CIT single photon emission computed tomography imaging and autopsy. J Neurol Neurosurg Psychiatr. (2007) 78:1176–81. doi: 10.1136/jnnp.2006.110122
157. Yang J, Archer DB, Burciu RG, Muller M, Roy A, Ofori E, et al. Multimodal dopaminergic and free-water imaging in Parkinson's disease. Parkinsonism Relat Disord. (2019) 62:10–5. doi: 10.1016/j.parkreldis.2019.01.007
158. Zhang Y, Wu IW, Tosun D, Foster E, Schuff N, Parkinson's Progression Markers I. Progression of regional microstructural degeneration in Parkinson's disease: a multicenter diffusion tensor imaging study. PLoS ONE. (2016) 11:e0165540. doi: 10.1371/journal.pone.0165540
159. Lenfeldt N, Eriksson J, Astrom B, Forsgren L, Mo SJ. Fractional anisotropy and mean diffusion as measures of dopaminergic function in parkinson's disease: challenging results. J Parkinsons Dis. (2017) 7:129–42. doi: 10.3233/JPD-161011
160. Lorio S, Sambataro F, Bertolino A, Draganski B, Dukart J. The combination of DAT-SPECT, structural and diffusion MRI predicts clinical progression in Parkinson's disease. Front Aging Neurosci. (2019) 11:57. doi: 10.3389/fnagi.2019.00057
161. Ofori E, Pasternak O, Planetta PJ, Li H, Burciu RG, Snyder AF, et al. Longitudinal changes in free-water within the substantia nigra of Parkinson's disease. Brain. (2015) 138:2322–31. doi: 10.1093/brain/awv136
162. Burciu RG, Ofori E, Archer DB, Wu SS, Pasternak O, McFarland NR, et al. Progression marker of Parkinson's disease: a 4-year multi-site imaging study. Brain. (2017) 140:2183–92. doi: 10.1093/brain/awx146
163. Pozorski V, Oh JM, Adluru N, Merluzzi AP, Theisen F, Okonkwo O, et al. Longitudinal white matter microstructural change in Parkinson's disease. Hum Brain Mapp. (2018) 39:4150–61. doi: 10.1002/hbm.24239
164. Surova Y, Nilsson M, Lampinen B, Latt J, Hall S, Widner H, et al. Alteration of putaminal fractional anisotropy in Parkinson's disease: a longitudinal diffusion kurtosis imaging study. Neuroradiology. (2018) 60:247–54. doi: 10.1007/s00234-017-1971-3
165. Miyasaki JM, Martin W, Suchowersky O, Weiner WJ, Lang AE. Practice parameter: initiation of treatment for Parkinson's disease: an evidence-based review: report of the quality standards subcommittee of the American academy of neurology. Neurology. (2002) 58:11–7. doi: 10.1212/WNL.58.1.11
166. Elm JJ, Goetz CG, Ravina B, Shannon K, Wooten GF, Tanner CM, et al. A responsive outcome for Parkinson's disease neuroprotection futility studies. Ann Neurol. (2005) 57:197–203. doi: 10.1002/ana.20361
167. Ravina B, Marek K, Eberly S, Oakes D, Kurlan R, Ascherio A, et al. Dopamine transporter imaging is associated with long-term outcomes in Parkinson's disease. Mov Disord. (2012) 27:1392–7. doi: 10.1002/mds.25157
168. Simuni T, Siderowf A, Lasch S, Coffey CS, Caspell-Garcia C, Jennings D, et al. longitudinal change of clinical and biological measures in early Parkinson's disease: Parkinson's progression markers initiative cohort. Mov Disord. (2018) 33:771–82. doi: 10.1002/mds.27361
169. Mishra VR, Sreenivasan KR, Zhuang X, Yang Z, Cordes D, Walsh RR. Influence of analytic techniques on comparing DTI-derived measurements in early stage Parkinson's disease. Heliyon. (2019) 5:e01481. doi: 10.1016/j.heliyon.2019.e01481
170. Ito M, Watanabe H, Kawai Y, Atsuta N, Tanaka F, Naganawa S, et al. Usefulness of combined fractional anisotropy and apparent diffusion coefficient values for detection of involvement in multiple system atrophy. J Neurol Neurosurg Psychiatr. (2007) 78:722–8. doi: 10.1136/jnnp.2006.104075
171. Wang PS, Wu HM, Lin CP, Soong BW. Use of diffusion tensor imaging to identify similarities and differences between cerebellar and Parkinsonism forms of multiple system atrophy. Neuroradiology. (2011) 53:471–81. doi: 10.1007/s00234-010-0757-7
172. Ota M, Nakata Y, Ito K, Kamiya K, Ogawa M, Murata M, et al. Differential diagnosis tool for parkinsonian syndrome using multiple structural brain measures. Comput Math Methods Med. (2013) 2013:571289. doi: 10.1155/2013/571289
173. Peran P, Barbagallo G, Nemmi F, Sierra M, Galitzky M, Traon AP, et al. MRI supervised and unsupervised classification of Parkinson's disease and multiple system atrophy. Mov Disord. (2018) 33:600–8. doi: 10.1002/mds.27307
174. Du G, Lewis MM, Kanekar S, Sterling NW, He L, Kong L, et al. Combined diffusion tensor imaging and apparent transverse relaxation rate differentiate Parkinson disease and atypical Parkinsonism. AJNR Am J Neuroradiol. (2017) 38:966–72. doi: 10.3174/ajnr.A5136
175. Umemura A, Oeda T, Hayashi R, Tomita S, Kohsaka M, Yamamoto K, et al. Diagnostic accuracy of apparent diffusion coefficient and 123I-metaiodobenzylguanidine for differentiation of multiple system atrophy and Parkinson's disease. PLoS ONE. (2013) 8:e61066. doi: 10.1371/journal.pone.0061066
176. Prodoehl J, Li H, Planetta PJ, Goetz CG, Shannon KM, Tangonan R, et al. Diffusion tensor imaging of Parkinson's disease, atypical parkinsonism, and essential tremor. Mov Disord. (2013) 28:1816–22. doi: 10.1002/mds.25491
177. Baudrexel S, Seifried C, Penndorf B, Klein JC, Middendorp M, Steinmetz H, et al. The value of putaminal diffusion imaging versus 18-fluorodeoxyglucose positron emission tomography for the differential diagnosis of the Parkinson variant of multiple system atrophy. Mov Disord. (2014) 29:380–7. doi: 10.1002/mds.25749
178. Sako W, Abe T, Murakami N, Miyazaki Y, Izumi Y, Harada M, et al. Imaging-based differential diagnosis between multiple system atrophy and Parkinson's disease. J Neurol Sci. (2016) 368:104–8. doi: 10.1016/j.jns.2016.06.061
179. Dickson DW, Hauw JJ, Agid Y, Litvan I. Progressive Supranuclear Palsy and Corticobasal Degeneration. 2nd ed. Chichester: Wiley-Blackwell. (2011). doi: 10.1002/9781444341256.ch15
180. Whitwell JL, Hoglinger GU, Antonini A, Bordelon Y, Boxer AL, Colosimo C, et al. Radiological biomarkers for diagnosis in PSP. Where are we and where do we need to be? Mov Disord. (2017) 32:955–71. doi: 10.1002/mds.27038
181. Blain CR, Barker GJ, Jarosz JM, Coyle NA, Landau S, Brown RG, et al. Measuring brain stem and cerebellar damage in parkinsonian syndromes using diffusion tensor MRI. Neurology. (2006) 67:2199–205. doi: 10.1212/01.wnl.0000249307.59950.f8
182. Worker A, Blain C, Jarosz J, Chaudhuri KR, Barker GJ, Williams SC, et al. Diffusion tensor imaging of Parkinson's disease, multiple system atrophy and progressive supranuclear palsy: a tract-based spatial statistics study. PLoS ONE. (2014) 9:e112638. doi: 10.1371/journal.pone.0112638
183. Seki M, Seppi K, Mueller C, Potrusil T, Goebel G, Reiter E, et al. Diagnostic potential of dentatorubrothalamic tract analysis in progressive supranuclear palsy. Parkins Relat Disord. (2018) 49:81–7. doi: 10.1016/j.parkreldis.2018.02.004
184. Cherubini A, Morelli M, Nistico R, Salsone M, Arabia G, Vasta R, et al. Magnetic resonance support vector machine discriminates between Parkinson disease and progressive supranuclear palsy. Mov Disord. (2014) 29:266–9. doi: 10.1002/mds.25737
185. Zhang Y, Walter R, Ng P, Luong PN, Dutt S, Heuer H, et al. Progression of microstructural degeneration in progressive supranuclear palsy and corticobasal syndrome: a longitudinal diffusion tensor imaging study. PLoS ONE. (2016) 11:e0157218. doi: 10.1371/journal.pone.0157218
186. Paviour DC, Price SL, Jahanshahi M, Lees AJ, Fox NC. Longitudinal MRI in progressive supranuclear palsy and multiple system atrophy: rates and regions of atrophy. Brain. (2006) 129(Pt 4):1040–9. doi: 10.1093/brain/awl021
187. Dutt S, Binney RJ, Heuer HW, Luong P, Attygalle S, Bhatt P, et al. Progression of brain atrophy in PSP and CBS over 6 months and 1 year. Neurology. (2016) 87:2016–25. doi: 10.1212/WNL.0000000000003305
188. Litvan I, Goldman JG, Troster AI, Schmand BA, Weintraub D, Petersen RC, et al. Diagnostic criteria for mild cognitive impairment in Parkinson's disease: movement disorder society task force guidelines. Mov Disord. (2012) 27:349–56. doi: 10.1002/mds.24893
189. Aarsland D, Andersen K, Larsen JP, Lolk A, Kragh-Sorensen P. Prevalence and characteristics of dementia in Parkinson disease: an 8-year prospective study. Arch Neurol. (2003) 60:387–92. doi: 10.1001/archneur.60.3.387
190. Hely MA, Reid WG, Adena MA, Halliday GM, Morris JG. The sydney multicenter study of Parkinson's disease: the inevitability of dementia at 20 years. Mov Disord. (2008) 23:837–44. doi: 10.1002/mds.21956
191. Bertrand JA, Bedetti C, Postuma RB, Monchi O, Genier Marchand D, Jubault T, et al. Color discrimination deficits in Parkinson's disease are related to cognitive impairment and white-matter alterations. Mov Disord. (2012) 27:1781–8. doi: 10.1002/mds.25272
192. Deng B, Zhang Y, Wang L, Peng K, Han L, Nie K, et al. Diffusion tensor imaging reveals white matter changes associated with cognitive status in patients with Parkinson's disease. Am J Alzheimers Dis Other Demen. (2013) 28:154–64. doi: 10.1177/1533317512470207
193. Agosta F, Canu E, Stefanova E, Sarro L, Tomic A, Spica V, et al. Mild cognitive impairment in Parkinson's disease is associated with a distributed pattern of brain white matter damage. Hum Brain Mapp. (2014) 35:1921–9. doi: 10.1002/hbm.22302
194. Matsui H, Nishinaka K, Oda M, Niikawa H, Kubori T, Udaka F. Dementia in Parkinson's disease: diffusion tensor imaging. Acta Neurol Scand. (2007) 116:177–81. doi: 10.1111/j.1600-0404.2007.00838.x
195. Kamagata K, Motoi Y, Abe O, Shimoji K, Hori M, Nakanishi A, et al. White matter alteration of the cingulum in Parkinson disease with and without dementia: evaluation by diffusion tensor tract-specific analysis. AJNR Am J Neuroradiol. (2012) 33:890–5. doi: 10.3174/ajnr.A2860
196. Chondrogiorgi M, Astrakas LG, Zikou AK, Weis L, Xydis VG, Antonini A, et al. Multifocal alterations of white matter accompany the transition from normal cognition to dementia in Parkinson's disease patients. Brain Imaging Behav. (2019) 13:232–40. doi: 10.1007/s11682-018-9863-7
197. Kamagata K, Motoi Y, Tomiyama H, Abe O, Ito K, Shimoji K, et al. Relationship between cognitive impairment and white-matter alteration in Parkinson's disease with dementia: tract-based spatial statistics and tract-specific analysis. Eur Radiol. (2013) 23:1946–55. doi: 10.1007/s00330-013-2775-4
198. Wiltshire K, Concha L, Gee M, Bouchard T, Beaulieu C, Camicioli R. Corpus callosum and cingulum tractography in Parkinson's disease. Can J Neurol Sci. (2010) 37:595–600. doi: 10.1017/S0317167100010751
199. Schulz J, Pagano G, Fernandez Bonfante JA, Wilson H, Politis M. Nucleus basalis of Meynert degeneration precedes and predicts cognitive impairment in Parkinson's disease. Brain. (2018) 141:1501–16. doi: 10.1093/brain/awy072
200. Caspell-Garcia C, Simuni T, Tosun-Turgut D, Wu IW, Zhang Y, Nalls M, et al. Multiple modality biomarker prediction of cognitive impairment in prospectively followed de novo Parkinson disease. PLoS ONE. (2017) 12:e0175674. doi: 10.1371/journal.pone.0175674
201. Silbert LC, Kaye J. Neuroimaging and cognition in Parkinson's disease dementia. Brain Pathol. (2010) 20:646–53. doi: 10.1111/j.1750-3639.2009.00368.x
202. Shin NY, Shin YS, Lee PH, Yoon U, Han S, Kim DJ, et al. Different functional and microstructural changes depending on duration of mild cognitive impairment in Parkinson disease. AJNR Am J Neuroradiol. (2016) 37:897–903. doi: 10.3174/ajnr.A4626
203. Lee JE, Park HJ, Park B, Song SK, Sohn YH, Lee JD, et al. A comparative analysis of cognitive profiles and white-matter alterations using voxel-based diffusion tensor imaging between patients with Parkinson's disease dementia and dementia with Lewy bodies. J Neurol Neurosurg Psychiatr. (2010) 81:320–6. doi: 10.1136/jnnp.2009.184747
204. Novellino F, Vasta R, Sarica A, Chiriaco C, Salsone M, Morelli M, et al. Relationship between hippocampal subfields and category cued recall in AD and PDD. A multimodal MRI study. Neuroscience. (2018) 371:506–17. doi: 10.1016/j.neuroscience.2017.12.028
205. Bergamino M, Keeling EG, Mishra VR, Stokes AM, Walsh RR. Assessing white matter pathology in early-stage Parkinson disease using diffusion MRI. A systematic review. Front Neurol. (2020) 11:314. doi: 10.3389/fneur.2020.00314
206. Tuch DS, Reese TG, Wiegell MR, Makris N, Belliveau JW, Wedeen VJ. High angular resolution diffusion imaging reveals intravoxel white matter fiber heterogeneity. Magn Reson Med. (2002) 48:577–82. doi: 10.1002/mrm.10268
207. Wedeen VJ, Wang RP, Schmahmann JD, Benner T, Tseng WY, Dai G, et al. Diffusion spectrum magnetic resonance imaging (DSI) tractography of crossing fibers. Neuroimage. (2008) 41:1267–77. doi: 10.1016/j.neuroimage.2008.03.036
208. Pasternak O, Sochen N, Gur Y, Intrator N, Assaf Y. Free water elimination mapping from diffusion MRI. Magn Reson Med. (2009) 62:717–30. doi: 10.1002/mrm.22055
209. Guttuso T Jr., Bergsland N, Hagemeier J, Lichter DG, Pasternak O, et al. Substantia nigra free water increases longitudinally in Parkinson disease. AJNR Am J Neuroradiol. (2018) 39:479–84. doi: 10.3174/ajnr.A5545
210. Jensen JH, Helpern JA, Ramani A, Lu H, Kaczynski K. Diffusional kurtosis imaging: the quantification of non-gaussian water diffusion by means of magnetic resonance imaging. Magn Reson Med. (2005) 53:1432–40. doi: 10.1002/mrm.20508
211. Kamagata K, Tomiyama H, Hatano T, Motoi Y, Abe O, Shimoji K, et al. A preliminary diffusional kurtosis imaging study of Parkinson disease: comparison with conventional diffusion tensor imaging. Neuroradiology. (2014) 56:251–8. doi: 10.1007/s00234-014-1327-1
212. Vriend C, van den Heuvel OA, Berendse HW, van der Werf YD, Douw L. Global and subnetwork changes of the structural connectome in de novo Parkinson's disease. Neuroscience. (2018) 386:295–308. doi: 10.1016/j.neuroscience.2018.06.050
213. Hall JM, Shine JM, Ehgoetz Martens KA, Gilat M, Broadhouse KM, Szeto JYY, et al. Alterations in white matter network topology contribute to freezing of gait in Parkinson's disease. J Neurol. (2018) 265:1353–64. doi: 10.1007/s00415-018-8846-3
214. Tinaz S, Lauro PM, Ghosh P, Lungu C, Horovitz SG. Changes in functional organization and white matter integrity in the connectome in Parkinson's disease. Neuroimage Clin. (2017) 13:395–404. doi: 10.1016/j.nicl.2016.12.019
215. Galantucci S, Agosta F, Stefanova E, Basaia S, van den Heuvel MP, Stojkovic T, et al. Structural brain connectome and cognitive impairment in Parkinson disease. Radiology. (2017) 283:515–25. doi: 10.1148/radiol.2016160274
216. Nigro S, Riccelli R, Passamonti L, Arabia G, Morelli M, Nistico R, et al. Characterizing structural neural networks in de novo Parkinson disease patients using diffusion tensor imaging. Hum Brain Mapp. (2016) 37:4500–10. doi: 10.1002/hbm.23324
217. Sharman M, Valabregue R, Perlbarg V, Marrakchi-Kacem L, Vidailhet M, Benali H, et al. Parkinson's disease patients show reduced cortical-subcortical sensorimotor connectivity. Mov Disord. (2013) 28:447–54. doi: 10.1002/mds.25255
218. Sterling NW, Du G, Lewis MM, Swavely S, Kong L, Styner M, et al. Cortical gray and subcortical white matter associations in Parkinson's disease. Neurobiol Aging. (2017) 49:100–8. doi: 10.1016/j.neurobiolaging.2016.09.015
219. Pagonabarraga J, Kulisevsky J. Cognitive impairment and dementia in Parkinson's disease. Neurobiol Dis. (2012) 46:590–6. doi: 10.1016/j.nbd.2012.03.029
220. Illan-Gala I, Montal V, Borrego-Ecija S, Vilaplana E, Pegueroles J, Alcolea D, et al. Cortical microstructure in the behavioural variant of frontotemporal dementia: looking beyond atrophy. Brain. (2019) 142:1121–33. doi: 10.1093/brain/awz031
221. Ball G, Srinivasan L, Aljabar P, Counsell SJ, Durighel G, Hajnal JV, et al. Development of cortical microstructure in the preterm human brain. Proc Natl Acad Sci USA. (2013) 110:9541–6. doi: 10.1073/pnas.1301652110
222. Andica C, Kamagata K, Hatano T, Saito A, Uchida W, Ogawa T, et al. Free-water imaging in white and gray matter in Parkinson's disease. Cells. (2019) 8:839. doi: 10.3390/cells8080839
223. Weston PS, Simpson IJ, Ryan NS, Ourselin S, Fox NC. Diffusion imaging changes in grey matter in Alzheimer's disease: a potential marker of early neurodegeneration. Alzheimers Res Ther. (2015) 7:47. doi: 10.1186/s13195-015-0132-3
224. Sampedro F, Perez-Gonzalez R, Martinez-Horta S, Marin-Lahoz J, Pagonabarraga J, Kulisevsky J. Serum neurofilament light chain levels reflect cortical neurodegeneration in de novo Parkinson's disease. Parkinsonism Relat Disord. (2020) 74:43–9. doi: 10.1016/j.parkreldis.2020.04.009
225. Sampedro F, Martinez-Horta S, Marin-Lahoz J, Pagonabarraga J, Kulisevsky J. Longitudinal intracortical diffusivity changes in de-novo Parkinson's disease: a promising imaging biomarker. Parkinsonism Relat Disord. (2019) 68:22–5. doi: 10.1016/j.parkreldis.2019.09.031
226. Zhang Y, Vakhtin AV, Jennings JS, Massaband P, Wintermark M, Craig PL, et al. Diffusion tensor tractography of brainstem fibers and its application in pain. PLoS ONE. (2019) 15:e0213952. doi: 10.1371/journal.pone.0213952
Keywords: diffusion tensor imaging (DTI), fractional anisotropy (FA), Parkinson's progression marker initiative (PPMI), diffusion tensor tractography (DTT), dopaminergic pathway, substantia nigra (SN), Parkinsion's disease (PD)
Citation: Zhang Y and Burock MA (2020) Diffusion Tensor Imaging in Parkinson's Disease and Parkinsonian Syndrome: A Systematic Review. Front. Neurol. 11:531993. doi: 10.3389/fneur.2020.531993
Received: 20 April 2020; Accepted: 18 August 2020;
Published: 25 September 2020.
Edited by:
Emily Keshner, Temple University, United StatesReviewed by:
Fred Sampedro, Sant Pau Institute for Biomedical Research, SpainShantanu Ghosh, Akal University, India
Copyright © 2020 Zhang and Burock. This is an open-access article distributed under the terms of the Creative Commons Attribution License (CC BY). The use, distribution or reproduction in other forums is permitted, provided the original author(s) and the copyright owner(s) are credited and that the original publication in this journal is cited, in accordance with accepted academic practice. No use, distribution or reproduction is permitted which does not comply with these terms.
*Correspondence: Yu Zhang, eXV6aGFuZ3Vjc2ZlZHUmI3gwMDA0MDtnbWFpbC5jb20=; WXUuWmhhbmcmI3gwMDA0MDt2YS5nb3Y=