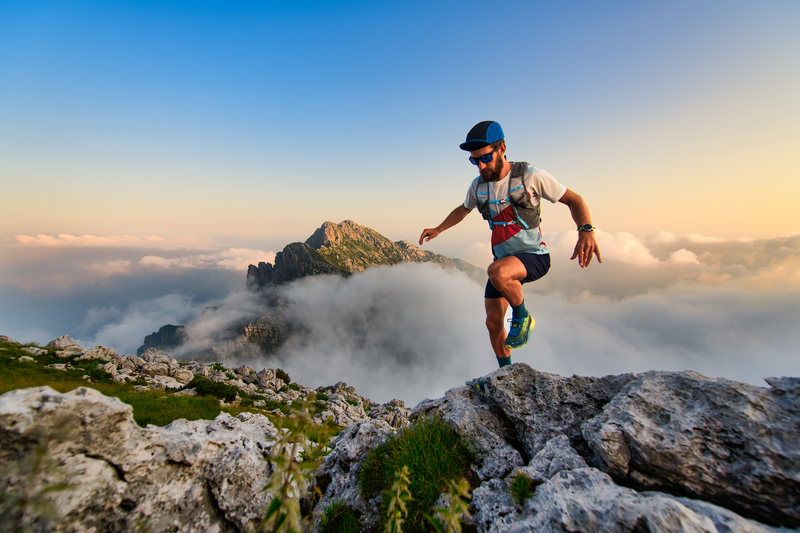
94% of researchers rate our articles as excellent or good
Learn more about the work of our research integrity team to safeguard the quality of each article we publish.
Find out more
ORIGINAL RESEARCH article
Front. Neurol. , 06 October 2020
Sec. Applied Neuroimaging
Volume 11 - 2020 | https://doi.org/10.3389/fneur.2020.529930
This article is part of the Research Topic Applied Neuroimaging - Editor's Pick 2022 View all 10 articles
Non-linear relations of brain amyloid beta (Aβ) with task- based functional connectivity (tbFC) measured with functional magnetic resonance imaging (fMRI) have been reported in late middle age. Our objective was to examine the association between brain Aβ and resting-state functional connectivity (rsFC) in late middle-aged adults. Global brain Aβ burden was ascertained with 18F-Florbetaben Positron Emission Tomography (PET); rsFC was ascertained on 3T Magnetic Resonance Imaging (MRI) among 333 late middle-aged Hispanics adults without dementia in four major brain functional connectivity networks: default mode network (DMN), fronto-parietal control network (FPC), salience network (SAL) and dorsal attention network (DAN). We examined the relationship of global brain Aβ with rsFC using multivariable linear regression adjusted for age, sex, education, and APOE-ε4 genotype. We quantified the non-linear associations both with quadratic terms and by categorizing Aβ into three groups: low Aβ, intermediate Aβ, and positive Aβ. We found no significant linear or non-linear associations between Aβ, measured either continuously or categorically, with rsFC in the examined networks. Our null findings may be explained by the younger age of our participants in whom amyloid burden is relatively low. It is also possible that the recently reported non-linear relationship is exclusive to task fMRI and not rsfMRI.
The deposition of amyloid beta (Aβ) in the brain starts decades before it impacts cognition (1). The effects of Aβ on brain function using resting-state functional connectivity (rsFC) magnetic reasoning imaging (fMRI) have been largely studied in cognitively normal older adults and have yielded conflicting findings with both positive and negative linear relationships reported for Aβ levels and functional connectivity (2–6). Similar results of hyperactivation and hypoactivation in association with brain Aβ are also observed with task activation (7–10). To address these mixed results, a recent study of 62 middle-aged and older adults (mean age: 67.73 years) reported a non-linear quadratic association between levels of Aβ and task-based functional connectivity (tbFC), such that intermediate levels of Aβ are associated with higher activation in regions of the default mode network (DMN), but high levels of Aβ are associated with lower activation (11). Another study from the same group (n = 68, mean age: 68.59) replicated these findings using a different functional task paradigm, suggesting that this non-linear relationship between Aβ and FC is task-independent (12). If replicated in rsFC, such a finding would provide evidence for the hypothesis that the brain dose-dependent response to levels of Aβ may also be apparent at rest when no task is performed (12).
Our primary goal in this study was to determine if the non-linear association of Aβ with functional connectivity, recently observed in tbFC, is also present at rest using fMRI in late middle-age, a critical period for the deposition of Aβ (13). Thus, we examined the association of global brain Aβ burden with rsFC among 333 late middle-aged Hispanic adults without dementia in four major cognitive networks: default mode network (DMN), fronto-parietal control network (FPC), dorsal attention network (DAN), and salience network (SAL).
This was a cross-sectional analysis of a community-based cohort of 333 late middle-aged Hispanic adults without dementia and with functional connectivity data out of 350 recruited between 03/01/2016 and 07/31/2019 in New York City as part of a study of Alzheimer's disease biomarkers (14). We targeted Hispanics because they are the most common ethnic group in the community surrounding CUIMC (15) and because there is a paucity of AD biomarkers studies in Non-Whites (13). Inclusion criteria included ages between 55 and 69 years, men and women, able to undergo phlebotomy, clinical and neuropsychological assessments, 3T brain magnetic resonance imaging (MRI), and Positron Emission Tomography (PET) with the Aβ radioligand 18F-Florbetaben. Exclusion criteria included dementia diagnosis, cancer other than non-melanoma skin cancer, and MRI contraindications. We screened 659 potential participants; 114 (17.30%) declined to participate, 178 (27.01%) were ineligible, 16 (2.43%) did not complete study procedures; 17 participants (2.58%) were excluded due to incomplete fMRI data and one additional participant (0.15%) was excluded from analyses due to incomplete data on APOE genotype, the most important predictor of in-vivo brain amyloid burden (16) (Figure 1). The interval between amyloid PET and MRI was 15.79 ± 33.41 days. The Institutional Review Board and the Joint Radiation Safety Commission at CUIMC approved this study. Participants provided written informed consent. Funding sources had no role in study design, data collection, data analyses or interpretation.
Our main exposure of interest was global brain Aβ ascertained with 18F-Florbetaben positron emission tomography (PET). The dose of 18F-Florbetaben was 300 MBq (8.1 mCi), maximum 30 mcg mass dose, administered as a single slow intravenous bolus. Images were acquired over 20 min starting 90 min after injection. Dynamic PET frames (4 scans) were aligned to the first frame using rigid-body registration and a static PET image was obtained by averaging the four registered frames. Additional information on the Aβ PET scan processing protocol has been previously published (17). The standardized uptake value (SUV), defined as the decay-corrected brain radioactivity concentration normalized for injected dose and body weight, was calculated in all regions and each voxel, which subsequently normalized to the SUV in cerebellar gray matter to derive the regional and voxel-wise SUVR. We used a standard approach to calculating global Aβ burden using Alzheimer's Disease Neuroimaging Initiative (ADNI) defined regions of interests (ROIs) to generate four lobar SUVRs (frontal, temporal, cingulate and parietal cortex) (18). We then averaged them to compute an overall mean value of global cortical Aβ. Aβ measures were examined both continuously and categorically. We examined the non-linear association of Aβ measured continuously by incorporating quadratic terms in the model. The threshold for dichotomization into Aβ positive and negative groups was 1.342 and was derived using the K-means clustering method. To classify two groups, the K-means clustering algorithm starts by identifying two Aβ SUVR values from our data at random and subsequently assigns all other observations to the group with the closest mean Aβ SUVR. Once this process has been completed for all observations, it continues to reiterate, checking if observations should be reclassified to the other cluster, until all group assignments are stable. The final classifications should minimize each within-cluster variance, equivalent to the sum of the squared Euclidean distance between each Aβ SUVR value and its within-cluster mean (19). Values under this resulting threshold (SUVR = 1.342) were further dichotomized as intermediate and low using a median split (SUVR = 1.126).
Resting-state scans were collected on a 3T GE scanner during 10 min of resting state protocol where participants were instructed to lie still, keep their eyes open, and to not think about anything in particular. T1-weighted images of the whole brain were acquired for each participant with a magnetization prepared rapid gradient-echo sequence (Time of Inversion (TI) = 450 ms; Flip angle (FA) =12 degree; Field of view (FOV) = 25.6 × 25.6 cm; Matrix size = 256 × 256). Ten minutes of rsfMRI scans were collected with GRE-EPI sequence using the following parameters: TR/TE = 2,000/23 ms; FOV =19.2 × 19.2 cm; FA = 77 degree; matrix size = 96 × 96; slice thickness/gap = 3/0 mm; number of slices = 40.
Images were preprocessed using an in-house developed native space method (20). Briefly, “slice timing correction was done using the FSL slice timing tool (21). We then used mcflirt (a motion correction tool available in the FSL package) to register all volumes to a reference image (21, 22). The reference image was generated by registering (6 df, 256 bins mutual information, and Sinc interpolation) all volumes to the middle volume and averaging them. We then used the method described in Power et al. (23) to calculate frame-wise displacement (FWD) from the six motion parameters and root mean square difference (RMSD) of the realigned fMRI signal in the consecutive volumes for each subject. To be conservative, we lowered the threshold of our RMSD to 0.3% (it was originally suggested to be 0.5%). RMSD was computed on the motion-corrected volumes before temporal filtering. The contaminated volumes were detected by the criteria FWD > 0.5 mm or RMSD > 0.3%. Identified contaminated volumes were replaced with new volumes generated by linear interpolation of adjacent volumes. Volume replacement was done before band-pass filtering (24). The motion-corrected signals were passed through a band-pass filter with the cutoff frequencies of 0.01 and 0.09 Hz. We used flsmaths– bptf to do the filtering in this study (22). Finally, we residualized the motion-corrected, scrubbed, and temporally filtered volumes by regressing out the FWD, RMSD, left and right hemisphere white matter, and lateral ventricular signals (25).”
T1-weighted image segmentation was performed with FreeSurfer (http://surfer.nmr.mgh.harvard.edu/) (26–28) and inspected visually for any inaccuracies. Using the state of the art non-linear registration method, advanced normalization tools (ANTs), we transferred the 264 putative functional coordinates derived from a brainwide graph described by Power et al. (29) from MNI space to subjects' native space. A conjunction mask was obtained as an intersection of a 10 mm radius spherical mask, centered at each coordinate and the native space gray-matter mask. This conjunction mask was used to compute the time-series for each coordinate by averaging the time-series of all voxels that fall within the conjunction mask. To prevent inflation of within-network functional connectivity (correlation), we also averaged all coordinates that fell within each node of the networks. For instance, all the DMN coordinates that were closely located in the left/right angular gyrus and inferior parietal regions were averaged to obtain a single time-series for left/right angular gyrus node of the DMN. This resulted in 53 separate nodes representing all the networks reported in Power et al. (29). The averaged functional time-series of each of the 53 regions were used to compute a correlation matrix for each participant preprocessed rsfMRI scan. Then, the correlations were Fisher z-transformed to generate normalized correlation matrices for each subject. In order to remove correlations between an area and itself, the diagonal of each correlation matrix was set to zero. Regions of interest (ROIs) were labeled based on their network assignment according to Power et al. (29) and within network functional connectivity was computed by averaging the inter-nodal correlation within each network. Four networks were preselected for statistical analysis based on their role on cognitive processes: default mode network (DMN; 9 nodes), salience network (SAL; 9 nodes), fronto-parietal control network (FPC; 9 nodes), and dorsal attention network (DAN; 6 nodes). For details on the location of each node within each network, see Supplemental Table 1.
All participants were administered a neuropsychological assessment that included tests of memory, language, visuospatial abilities, and processing speed. A Spanish version of the neuropsychological test battery was used for Spanish-speaking participants (30). The following tests were included: the Buschke Selective reminding Tests (SRT) and its components, including total recall, delayed recall, and delayed recognition (31); the Boston Naming-15 item Test (32); letter fluency; category fluency; the similarities subtest from the Wechsler Adult Intelligence Scale-Revised (33); the repetition and comprehension subtests of the Boston Diagnostic Aphasia Evaluation (34); the recognition and matching components of the Benton Visual Retention Test (35); the Rosen Drawing Test (36); the identities and oddities subtest from the Mattis Dementia Rating Scale (37); Color Trails 1 and 2 (38). To aggregate the neuropsychological test data, we performed a Principal Component Analysis (PCA). PCA is a statistical method most commonly used for data reduction on a set of collinear variables, where each principle component is a linear combination of the centered loading variables (in this case, our neuropsychological test scores) such that the variance of these variables is maximized (39). Each principle component is independent and captures a proportion of the variance in the neuropsychological tests, meaning that 15 principle components would completely capture the variance in our 15 tests. We chose to only retain the first principle component for our analysis as it captured much more variance in our tests than any other component. Additionally, the difference in the variance captured by all other principle components was not appreciable by visual inspection of the scree plot of our components (Supplemental Figure 1).
Characteristics considered as potential covariates included age, sex, education, and APOE-ε4 genotype. We controlled for age, sex and education due to their known effects on functional connectivity (40, 41). We controlled for APOE-ε4 genotyping because it is a strong determinant of Aβ burden (42). Participants were classified as APOE-ε4 carriers if they were homozygous or heterozygous for APOE-ε4. APOE-ε4 genotyping was conducted by LGC genomics (Beverly, MA) using single nucleotide polymorphisms rs429358 and rs7412.
RsFC values in the four networks examined were right-skewed and required logarithmic transformation to approximate a normal distribution. Bivariate comparisons across Aβ categories were made using analysis of variance (ANOVA) for continuous variables. Differences in categorical variables were evaluated using chi-squared tests. The relationship between global brain Aβ SUVR and rsFC was evaluated with a multivariable linear regression model including continuous Aβ SUVR and squared terms for Aβ SUVR, adjusted for age, sex, education, and APOE-ε4 carrier status. The relationship of Aβ categories (low, intermediate, and positive) with rsFC was examined with linear regression models adjusted for age, sex, education, and APOE-ε4 carrier status. The statistical significance was defined as p < 0.05. All statistical analyses were performed using the Statistical Package for the Social Sciences (SPSS) 26 (SPSS, Chicago, IL) and R version 3.6.0.
As a sensitivity analysis, we examined the association of Aβ with rsFC among participants who were cognitively normal only. Cognitive impairment was defined according to cutoffs of 1.0 or 1.5 standard deviations below the first principal component of the neuropsychological battery of our sample (43). The rationale for conducting sensitivity analyses restricted to cognitively normal participants was to replicate prior studies, which exclusively examined these associations among cognitively normal participants (11, 12). We validated our assumption that the first principle component of the neuropsychological battery is an appropriate approximation of global cognition by verifying that better performance on all neuropsychological evaluations was significantly positively correlated with increases in this principle component.
Table 1 shows the characteristics of the total sample and across Aβ categories (low, intermediate, positive). The sample consisted of 237 women and 96 men, who had a mean age of 64.14 ± 3.37 years and 10.51 ± 3.95 years of education. There were no significant differences across Aβ groups with respect to age and education. There was a higher proportion of women among the intermediate and positive Aβ categories. As expected, compared to participants without the APOE-ε4 allele, APOE-ε4 carriers had higher odds of being in the intermediate or positive category as compared to the low Aβ category (Table 1, Supplemental Figure 2). Figure 2 shows the distribution of global Aβ SUVR in the sample. As expected, Aβ SUVR had a bimodal distribution and the first peak resembled a normal distribution.
Figure 2. Distribution of global brain amyloid SUVR. The solid line overlaid onto the histogram represents a non-parametric kernel density estimation. The right vertical dashed line marks the threshold for amyloid positivity using the k-means clustering method (1.342) while the left vertical line marks the threshold for intermediate amyloid (1.126).
Figure 3 represents scatter plots for the association between rsFC in the four networks and global Aβ SUVR. We observed no linear or quadratic association between Aβ and rsFC in the four networks, including the DMN. Results for adjusted and unadjusted associations with continuous Aβ (SUVR) and rsFC are reported in Table 2. In Table 3, we report associations between dichotomized Aβ categories and rsFC. Results were similar in sensitivity analyses restricted to participants who were cognitively normal only based either on 1.5 (n = 318) or 1.0 SD (n = 291) below the first PCA of the sample neuropsychological performance.
Figure 3. Scatterplots for the association between rsFC and global Aβ SUVRs in four cognitive networks: DMN (A), FPC (B), SAL (C) and DAN (D). RsFC for each network has been log-transformed. The two dashed lines represent Aβ thresholds between low and intermediate (SUVR = 1.126) and between intermediate and positive (SUVR = 1.342) categories.?Abbreviations: Ln, natural logarithm; Aβ, amyloid beta; SUVR, Standardized Uptake Value Ratio; rsFC, resting state functional connectivity; DMN, default mode network; FPC, fronto-parietal control; DAN, dorsal attention network; SAL, salience.
Table 2. Coefficients and p-values from linear regression for linear and quadratic term of Aβ (SUVR) on rsFC.
Table 3. Association between Aβ categories (low, intermediate and positive) and rsFC using linear regressions.
Our goal in the current study was to test if the recent evidence of a dose-dependent relationship between Aβ and tbFC in regions of the DMN (11, 12) are also present at rest. We found that there is no association between Aβ burden and rsFC.
In a study of 62 middle-aged and older adults (mean age=67.73 years), Foster et al. (11) reported that the association between Aβ SUVR and tbFC is quadratic with both hyperactivation and hypoactivation phases in regions of the DMN. These findings were further replicated by the same group using a different functional task paradigm (n = 68, mean age: 68.59), suggesting that this non-linear relationship is task independent (12). However, the question on whether this observed association between Aβ and functional connectivity is actually present at rest remains unanswered. The results from our large sample of 333 found no linear or non-linear association between global brain Aβ and functional connectivity in major cognitive networks, including DMN, measured at rest.
There are several possible explanations of our findings. First, it is possible that the reported non-linear association by Foster et al. with task fMRI (11, 12), although independent of the type of task performed, may be dependent on the action of performing a task in general and therefore not an intrinsic effect apparent at rest. The fact that this was observed using different task paradigms does not entirely confirm its task independency. Alternatively, it is possible that the relatively younger age group of our sample is at its initial stages of Aβ accumulation which has not yet affected rsFC. Most of the reported studies on Aβ and rsFC were done in older adults and still yielded different results between a positive and negative associations (2–6). A null association with static rsFC, as was observed in our study, was also reported in a community-based cohort of 133 adults with a mean age of 72 years (44). A follow-up of our cohort will allow for more time for Aβ accumulation, at which point an effect on rsFC may be detected. Finally both Aβ and tau accumulation may be required for the non-linear effects when it comes to the resting brain which has been recently reported in cognitively normal older adults (45). We cannot address the effects of tau in our data. However, we are currently collecting tau PET data in this sample, which will allow us to investigate the interactive roles of tau and Aβ on functional connectivity in future analyses.
Strengths of our study include the relatively large sample of late middle-aged Hispanic adults within a relatively narrow age range, which addresses a gap in studies in late middle age and in ethnic minorities. The main limitation of our study is its cross-sectional design, which limits our ability to infer causality from our findings. It is also possible that our findings are not generalizable to other ethnic groups. However, we could also argue that our findings may be generalized to other ethnics groups since APOE-ε4 in our sample was associated with higher amyloid burden as reported in most Non-Hispanic White samples (46).
In conclusion, we found that no association between brain Aβ burden and rsFC in our cohort of late middle-aged Hispanics without dementia. Continued follow-up of the same cohort is needed to examine future effects of Aβ accumulation on brain functional networks.
The datasets generated for this study are available on request to the corresponding author.
The studies involving human participants were reviewed and approved by the Institutional Review Board at Columbia University Irving Medical Center. The patients/participants provided their written informed consent to participate in this study.
MT conducted statistical analyses, and drafted the initial manuscript. BR performed data curation, advised on data interpretation, and manuscript review. PP advised on data interpretation and manuscript review. LS, FC, MP, GS, GH, and RA assisted with data collection. HH conducted the PET data processing. AS conducted the fMRI data processing. SA helped with fMRI data processing. JT, HM, and AB advised on data interpretation and manuscript review. QR advised on fMRI/PET data analyses and manuscript review. JL advised on data interpretation and manuscript review and supervised the project. All authors contributed to the article and approved the submitted version.
Support for the reported work was provided by United States National Institutes of Health Grant Nos. R01AG050440, RF1AG051556, and RF1AG051556-01S2. Partial support was also provided by Grant Nos. K24AG045334, P30AG059303, and ULT1TR001873. PP is supported by Grant No. R00 AG052830 from the National Institute of Aging. The views expressed in this manuscript are those of the authors and do not necessarily represent the views of the National Institute of Aging; the National Institutes of Health; or the U.S. Department of Health and Human Services.
JL receives a stipend from Wolters Kluwer, N.V. as Editor in Chief of The Journal Alzheimer's Disease and Associated Disorders, and has served as a paid consultant to vTv, In review therapeutics, Inc. and Recruitment Partners. AB is a paid consultant for Regeneron Pharmaceuticals and Cognition Therapeutics, Inc and owns equity in Mars Holding Limited.
The remaining authors declare that the research was conducted in the absence of any commercial or financial relationships that could be construed as a potential conflict of interest.
The Supplementary Material for this article can be found online at: https://www.frontiersin.org/articles/10.3389/fneur.2020.529930/full#supplementary-material
1. Gonneaud J, Arenaza-Urquijo EM, Mezenge F, Landeau B, Gaubert M, Bejanin A, et al. Increased florbetapir binding in the temporal neocortex from age 20 to 60 years. Neurology. (2017) 89:2438–46. doi: 10.1212/WNL.0000000000004733
2. Hedden T, Van Dijk KR, Becker JA, Mehta A, Sperling RA, Johnson KA, et al. Disruption of functional connectivity in clinically normal older adults harboring amyloid burden. J Neurosci. (2009) 29:12686–94. doi: 10.1523/JNEUROSCI.3189-09.2009
3. Sheline YI, Raichle ME, Snyder AZ, Morris JC, Head D, Wang S, et al. Amyloid plaques disrupt resting state default mode network connectivity in cognitively normal elderly. Biol Psychiatry. (2010) 67:584–7. doi: 10.1016/j.biopsych.2009.08.024
4. Wang L, Brier MR, Snyder AZ, Thomas JB, Fagan AM, Xiong C, et al. Cerebrospinal fluid Abeta42, phosphorylated Tau181, and resting-state functional connectivity. JAMA Neurol. (2013) 70:1242–8. doi: 10.1001/jamaneurol.2013.3253
5. Lim HK, Nebes R, Snitz B, Cohen A, Mathis C, Price J, et al. Regional amyloid burden and intrinsic connectivity networks in cognitively normal elderly subjects. Brain. (2014) 137:3327–38. doi: 10.1093/brain/awu271
6. Mormino EC, Smiljic A, Hayenga AO, Onami SH, Greicius MD, Rabinovici GD, et al. Relationships between beta-amyloid and functional connectivity in different components of the default mode network in aging. Cereb Cortex. (2011) 21:2399–407. doi: 10.1093/cercor/bhr025
7. Sperling RA, Dickerson BC, Pihlajamaki M, Vannini P, LaViolette PS, Vitolo OV, et al. Functional alterations in memory networks in early alzheimer's disease. Neuromolecular Med. (2010) 12:27–43. doi: 10.1007/s12017-009-8109-7
8. Sperling R. Potential of functional MRI as a biomarker in early alzheimer's disease. Neurobiol Aging. (2011) 32 Suppl 1:S37–43. doi: 10.1016/j.neurobiolaging.2011.09.009
9. Kennedy KM, Rodrigue KM, Devous MD Jr, Hebrank AC, Bischof GN, et al. Effects of beta-amyloid accumulation on neural function during encoding across the adult lifespan. Neuroimage. (2012) 62:1–8. doi: 10.1016/j.neuroimage.2012.03.077
10. Oh H, Steffener J, Razlighi QR, Habeck C, Stern Y. beta-amyloid deposition is associated with decreased right prefrontal activation during task switching among cognitively normal elderly. J Neurosci. (2016) 36:1962–70. doi: 10.1523/JNEUROSCI.3266-15.2016
11. Foster CM, Kennedy KM, Horn MM, Hoagey DA, Rodrigue KM. Both hyper- and hypo-activation to cognitive challenge are associated with increased beta-amyloid deposition in healthy aging: a nonlinear effect. Neuroimage. (2018) 166:285–92. doi: 10.1016/j.neuroimage.2017.10.068
12. Kennedy KM, Foster CM, Rodrigue KM. Increasing beta-amyloid deposition in cognitively healthy aging predicts nonlinear change in bold modulation to difficulty. Neuroimage. (2018) 183:142–9. doi: 10.1016/j.neuroimage.2018.08.017
13. Jack CR Jr, Knopman DS, Jagust WJ, Petersen RC, Weiner MW, et al. Tracking pathophysiological processes in alzheimer's disease: an updated hypothetical model of dynamic biomarkers. Lancet Neurol. (2013) 12:207–16. doi: 10.1016/S1474-4422(12)70291-0
14. Luchsinger JA, Palta P, Rippon B, Sherwood G, Soto L, Ceballos F, et al. Pre-Diabetes, but not type 2 diabetes, is related to brain amyloid in late middle-age. J Alzheimers Dis. (2020) 75:1241–52. doi: 10.3233/JAD-200232
15. Luchsinger JA, Cabral R, Eimicke JP, Manly JJ, Teresi J. glycemia, diabetes status, and cognition in hispanic adults aged 55-64 years. Psychosom Med. (2015) 77:653–63. doi: 10.1097/PSY.0000000000000208
16. Ossenkoppele R, Jansen WJ, Rabinovici GD, Knol DL, van der Flier WM, van Berckel BNM, et al. Prevalence of amyloid PET positivity in dementia syndromes: a meta-analysis. JAMA. (2015) 313:1939–49. doi: 10.1001/jama.2015.4669
17. Tahmi M, Bou-Zeid W, Razlighi QR. A fully automatic technique for precise localization and quantification of amyloid-beta PET scans. J Nucl Med. (2019) 60:1771–9. doi: 10.2967/jnumed.119.228510
18. Landau SM, Horng A, Fero A, Jagust WJ, Alzheimer's Disease Neuroimaging I. Amyloid negativity in patients with clinically diagnosed alzheimer disease and MCI. Neurology. (2016) 86:1377–85. doi: 10.1212/WNL.0000000000002576
19. MacQueen J editor. Some methods for classification and analysis of multivariate observations. In: Proceedings of the Fifth Berkeley Symposium on Mathematical Statistics and Probability, Vol. 1: Statistics (1967). Berkeley, CA: University of California Press.
20. Razlighi QR, Habeck C, Steffener J, Gazes Y, Zahodne LB, Mackay-Brandt A, et al. Unilateral disruptions in the default network with aging in native space. Brain Behav. (2014) 4:143–57. doi: 10.1002/brb3.202
21. Jenkinson M, Beckmann CF, Behrens TE, Woolrich MW, Smith SM. Fsl. Neuroimage. (2012) 62:782–90. doi: 10.1016/j.neuroimage.2011.09.015
22. Jenkinson M, Bannister P, Brady M, Smith S. Improved optimization for the robust and accurate linear registration and motion correction of brain images. Neuroimage. (2002) 17:825–41. doi: 10.1006/nimg.2002.1132
23. Power JD, Barnes KA, Snyder AZ, Schlaggar BL, Petersen SE. Spurious but systematic correlations in functional connectivity MRI networks arise from subject motion. Neuroimage. (2012) 59:2142–54. doi: 10.1016/j.neuroimage.2011.10.018
24. Carp J. Optimizing the order of operations for movement scrubbing: comment on power et al. Neuroimage. (2013) 76:436–8. doi: 10.1016/j.neuroimage.2011.12.061
25. Birn RM, Diamond JB, Smith MA, Bandettini PA. Separating respiratory-variation-related fluctuations from neuronal-activity-related fluctuations in fMRI. Neuroimage. (2006) 31:1536–48. doi: 10.1016/j.neuroimage.2006.02.048
26. Dale AM, Fischl B, Sereno MI. Cortical surface-based analysis. I. Segmentation and surface reconstruction. Neuroimage. (1999) 9:179–94. doi: 10.1006/nimg.1998.0395
27. Fischl B, Salat DH, Busa E, Albert M, Dieterich M, Haselgrove C, et al. Whole brain segmentation: automated labeling of neuroanatomical structures in the human brain. Neuron. (2002) 33:341–55. doi: 10.1016/S0896-6273(02)00569-X
28. Fischl B, van der Kouwe A, Destrieux C, Halgren E, Segonne F, Salat DH, et al. Automatically parcellating the human cerebral cortex. Cereb Cortex. (2004) 14:11–22. doi: 10.1093/cercor/bhg087
29. Power JD, Cohen AL, Nelson SM, Wig GS, Barnes KA, Church JA, et al. Functional network organization of the human brain. Neuron. (2011) 72:665–78. doi: 10.1016/j.neuron.2011.09.006
30. Siedlecki KL, Manly JJ, Brickman AM, Schupf N, Tang MX, Stern Y. Do neuropsychological tests have the same meaning in Spanish speakers as they do in English speakers? Neuropsychology. (2010) 24:402–11. doi: 10.1037/a0017515
31. Buschke H, Fuld PA. Evaluating storage, retention, and retrieval in disordered memory and learning. Neurology. (1974) 24:1019–25. doi: 10.1212/WNL.24.11.1019
32. Roth C. Boston naming test. In: Kreutzer JS, DeLuca J, Caplan B, editors. Encyclopedia of Clinical Neuropsychology. New York, NY: Springer New York (2011). p. 430–3.
33. Wechsler D, De Lemos MM. Wechsler Adult Intelligence Scale-Revised New York, NY: Harcourt Brace Jovanovich (1981).
34. Goodglass H, Kaplan E. The Assessment of Aphasia and Related Disorders Philadelphia, PA: Lea & Febiger (1972).
37. Mattis S. Mental status examination for organic mental syndrome in the elderly patient. In: Bellack L, Karusu TB, editors. Geriatric Psychiatry. New York, NY: Grune & Stratton (1976). p. 77–121.
38. L.F D'Elia PS, C.L Uchiyama TW. Color Trails Test. Odessa, FL: Psychological Assessment Resources (1996).
39. Jolliffe IT, Cadima J. Principal component analysis: a review and recent developments. Philos Trans A Math Phys Eng Sci. (2016) 374:20150202. doi: 10.1098/rsta.2015.0202
40. Scheinost D, Finn ES, Tokoglu F, Shen X, Papademetris X, Hampson M, et al. Sex differences in normal age trajectories of functional brain networks. Hum Brain Mapp. (2015) 36:1524–35. doi: 10.1002/hbm.22720
41. Shen X, Cox SR, Adams MJ, Howard DM, Lawrie SM, Ritchie SJ, et al. Resting-State connectivity and its association with cognitive performance, educational attainment, and household income in the UK biobank. Biol Psychiatry Cogn Neurosci Neuroimaging. (2018) 3:878–86. doi: 10.1016/j.bpsc.2018.06.007
42. Sanchez-Juan P, Seshadri S. Dynamic measurements of beta-amyloid accumulation: the early effect of APOE. Neurology. (2017) 89:986–7. doi: 10.1212/WNL.0000000000004344
43. Albert MS, DeKosky ST, Dickson D, Dubois B, Feldman HH, Fox NC, et al. The diagnosis of mild cognitive impairment due to alzheimer's disease: recommendations from the national institute on aging-alzheimer's association workgroups on diagnostic guidelines for alzheimer's disease. Alzheimers Dement. (2011) 7:270–9. doi: 10.1016/j.jalz.2011.03.008
44. Hahn A, Strandberg TO, Stomrud E, Nilsson M, van Westen D, Palmqvist S, et al. association between earliest amyloid uptake and functional connectivity in cognitively unimpaired elderly. Cereb Cortex. (2019) 29:2173–82. doi: 10.1093/cercor/bhz020
45. Schultz AP, Chhatwal JP, Hedden T, Mormino EC, Hanseeuw BJ, Sepulcre J, et al. phases of hyperconnectivity and hypoconnectivity in the default mode and salience networks track with amyloid and tau in clinically normal individuals. J Neurosci. (2017) 37:4323–31. doi: 10.1523/JNEUROSCI.3263-16.2017
Keywords: Alzheimer's disease, amyloid beta, RsfMRI, fMRI, functional connectivity, middle-age, hispanic
Citation: Tahmi M, Rippon B, Palta P, Soto L, Ceballos F, Pardo M, Sherwood G, Hernandez G, Arevalo R, He H, Sedaghat A, Arabshahi S, Teresi J, Moreno H, Brickman AM, Razlighi QR and Luchsinger JA (2020) Brain Amyloid Burden and Resting-State Functional Connectivity in Late Middle-Aged Hispanics. Front. Neurol. 11:529930. doi: 10.3389/fneur.2020.529930
Received: 27 January 2020; Accepted: 06 October 2020;
Published: 06 October 2020.
Edited by:
Peter Sörös, University of Oldenburg, GermanyReviewed by:
Silvia Tommasin, Sapienza University of Rome, ItalyCopyright © 2020 Tahmi, Rippon, Palta, Soto, Ceballos, Pardo, Sherwood, Hernandez, Arevalo, He, Sedaghat, Arabshahi, Teresi, Moreno, Brickman, Razlighi and Luchsinger. This is an open-access article distributed under the terms of the Creative Commons Attribution License (CC BY). The use, distribution or reproduction in other forums is permitted, provided the original author(s) and the copyright owner(s) are credited and that the original publication in this journal is cited, in accordance with accepted academic practice. No use, distribution or reproduction is permitted which does not comply with these terms.
*Correspondence: José A. Luchsinger, amFsOTRAY3VtYy5jb2x1bWJpYS5lZHU=
Disclaimer: All claims expressed in this article are solely those of the authors and do not necessarily represent those of their affiliated organizations, or those of the publisher, the editors and the reviewers. Any product that may be evaluated in this article or claim that may be made by its manufacturer is not guaranteed or endorsed by the publisher.
Research integrity at Frontiers
Learn more about the work of our research integrity team to safeguard the quality of each article we publish.