- 1College of Life Science and Bioengineering, Beijing University of Technology, Beijing, China
- 2Beijing Tiantan Hospital, Capital Medical University, Beijing, China
Background: Although functional brain connectivity in anxiety-related disorders has been studied, brain connectivity in non-clinical populations with high trait anxiety has been rarely reported. Whether structural brain connectivity changes in young healthy individuals with high trait anxiety remains unknown.
Methods: Thirty-eight young healthy individuals with high anxiety levels and 34 healthy subjects with low anxiety levels who were matched by age, gender, and educational level were recruited. Diffusion tensor images were acquired to analyze white matter connectivity. A two-sample t-test was used for group comparison of weighted networks and graph properties.
Results: Different connections were detected in fractional anisotropy- and fiber number-weighted networks. These connections were widely distributed in various regions, where relative significance was located in the inter-hemispheric frontal lobe, the frontal-limbic lobe in the right intra-hemisphere, and the frontal-temporal lobe in the ipsilateral hemisphere. However, no significant difference was found in fiber length-weighted network and in graph properties among the three networks.
Conclusions: The structural connectivity of white matter may be a vulnerability marker. Hence, healthy individuals with high trait anxiety levels are susceptible to anxiety-related psychopathology. The findings may help elucidate the pathological mechanism of anxiety and establish interventions for populations susceptible to anxiety disorders.
Introduction
An increasing number of young people feel large pressure caused by the fast-paced life in our modern society. The morbidity of anxiety-related disorders has increased in recent years. Trait anxiety refers to the general traits or personality and is manifested as persistent worry and instability. Anxiety is disruptive to daily life, and long-term anxiety significantly increases the risks of developing anxiety-related disorders. We supposed that young healthy individuals with high trait anxiety level may be susceptible to anxiety-related disorders (1, 2).
The analysis of brain connectivity through graph theory and magnetic resonance imaging (MRI) has become popular in research of nervous system diseases. Previous studies demonstrated abnormalities in brain connectivity among anxious patients. Zhu et al. found that changes in “small-world” properties in resting-state functional MRI (fMRI) are prominent among people with social anxiety disorders (3). Pacheco-Unguetti et al. found that trait anxiety is related to deficiencies in the executive control network, and attention-executive control function is impaired in adults with generalized anxiety disorder (4). Liao et al. found increased functional connectivity of the right posterior inferior temporal gyrus to the left inferior occipital gyrus, the right parahippocampal/hippocampal gyrus to the left middle temporal gyrus, and enhanced structural connectivity located in the genu of the corpus callosum among people with social anxiety disorders (5). Baur et al. found that the anterior insula and basolateral amygdala constitute a network markedly linked to anxiety (6).
In addition to an analysis using fMRI, electroencephalography (EEG) is used to detect abnormal connections in patients with anxiety disorders even at a rest state (7). However, T1-weighted imaging and diffusion tensor imaging (DTI) have been rarely used to detect structural brain connectivity. For the non-clinical population with high trait anxiety level, few neuroimaging studies have focused on brain connectivity. Our previous study used tract-based spatial statistics method to compare white matter differences among young healthy individuals with low and high trait anxiety levels (8). Alterations in the thalamus–cortical circuit and some emotion-related areas are commonly reported in anxiety-related disorders (8). In the present study, three weighted networks featured by fractional anisotropy (FA), fiber number (FN), and fiber length (FL) were analyzed; and group comparisons were performed using two-sample t-test to explore anatomical brain connectivity changes in a healthy population with high trait anxiety levels.
We expected to find important white matter structural connections related to trait anxiety in young healthy populations with high trait anxiety levels. These findings may provide supports for establishing a neuroimaging biomarker of susceptibility to anxiety disorders to help prevent and treat the disease.
Materials and Methods
Subjects
Seventy-two healthy right-handed undergraduate or postgraduate students were recruited from the Southwest University Longitudinal Imaging Multimodal, Brain Data Repository (China) (http://fcon_1000.projects.nitrc.org/indi/retro/southwestuni_qiu_index.html) (9). Self-rating scores including State-Trait Anxiety Inventory (10) and Combined Raven's matrix test (CRT) (11) were measured. Subjects with trait anxiety scores exceeding 50 were classified into the high trait anxiety (HTA) group (19 males and 19 females, trait score 54.5 ± 2.628), and those with scores below 30 were classified into the low trait anxiety (LTA) group (14 males and 20 females, trait score 26.177 ± 2.516) (12). The participants were matched by age (t = −0.567, p = 0.572) and gender (χ2 = 0.563, p = 0.453). None of the subjects presented a history of neurological or psychiatric disorders nor underwent mental health treatment and medications. All subjects conformed to the standards of MRI scanning and provided informed written consent prior to the study. The procedures of consent and experiments were approved by the Research Ethics Committee of the Brain Imaging Center of Southwest University and agreed with the standards of the Declaration of Helsinki (1989).
Procedures
MRI Data Acquisition
All participants were scanned using a 3.0-T Siemens Trio MRI scanner (Siemens Medical, Erlangen, Germany). Diffusion-weighted imaging (DWI) for each subject was acquired with a single-shot, spin-echo, echo-planar imaging (EPI) sequence [TR/TE = 11,000/98 ms, matrix = 128 × 128, field of view (FOV) = 256 × 256 mm2, voxel size = 2.0 × 2.0 × 2.0 mm3, 60 axial slices, slice thickness = 2.0 mm, b value 1 = 0 s/mm2, b value 2 = 1,000 s/mm2] in 30 directions. The subjects were repeatedly scanned three times to increase the signal-to-noise ratio (SNR) in the DWI sequence. For each time, one b0 = 0 volume and 30 b0 = 1,000 volumes were acquired.
In addition, a magnetization-prepared rapid gradient echo (MPRAGE) sequence was used to acquire high-resolution T1-weighted anatomical images. T1-weighted structural images would be used as the templates of brain regions in the following data analysis procedure. The main parameters are as follows: repetition time = 1,900 ms, echo time = 2.52 ms, inversion time = 900 ms, flip angle = 9°, resolution matrix = 256 × 256, slices = 176, thickness = 1.0 mm, and voxel size = 1 × 1 × 1 mm3. In this study, T1 images were non-linearly transformed into the Montreal Neurological Institute (MNI) space, using the ICBM152 T1 template as a reference.
Data Analysis
All imaging data were processed by PANDA software (13), a MATLAB toolbox that integrates MRIcron (https://www.nitrc.org/projects/mricron), FSL (https://fsl.fmrib.ox.ac.uk/fsl/fslwiki), and Diffusion Toolkit (http://www.trackvis.org/dtk/). The flowchart of data analysis is shown in Figure 1. The procedure using PANDA mainly comprises data preprocessing and construction of white matter structural network.
Image Preprocessing
In detail, the following data preprocessing steps were performed: (1) checking of image quality and converting of DICOM files into NIFTI images; (2) brain extraction and brain mask estimation; (3) cropping of images and correcting of eddy current/motion; and (4) calculation of DTI metrics (FA).
Construction of White Matter Structural Networks
After preprocessing, white matter structural networks were constructed. (1) Affine transformation was used to match FA map and its corresponding T1 image in individual space. (2) The transformed T1 map was non-linearly registered into MNI space by T1 template. (3) Based on the above two steps, non-linear transformation from DTI individual space to MNI standard space and its inverse transformation were obtained. The Automated Anatomical Labeling (AAL) template (14) including 90 brain subregions in MNI space was registered into individual space by inverse transformation. Ninety subregions in the individual space were then segmented and labeled. (4) White matter fibers were tracked with deterministic tracking algorithm. When FA <0.2 or direction change > 45°, the white matter fiber tracking was terminated to acquire the whole brain tractography. (5) Based on the whole brain tractography, white matter structural networks could be constructed by defining FA, FN, and FL as edge weight and 90 subregions as nodes.
Network Analysis
Graph theoretical network analysis was performed to investigate potential differences in structural network topological characteristics between HTA and LTA groups. Edges with FN value <3 and FA <0.2 were excluded to eliminate the pseudoconnection or noise of the network (15). Accordingly, FA-weighted, FN-weighted, and FL-weighted matrix for the following analysis were obtained. The metrics of these networks such as global efficiency (Eg), local efficiency (Eloc), clustering coefficient (Cp), characteristic path length (Lp), and small-world property (Sigma) were calculated by GRETNA software (16). Global efficiency refers to the average inverse shortest path length, which reflects the overall efficiency of information transmission in the network. Clustering coefficient was described as the prevalence of clustered connectivity around individual nodes. The average shortest path length between all pairs of nodes in the network was called the characteristic path length (17). Local efficiency was defined as the average efficiency of the subnetwork, which described the information exchange efficiency of subnetworks. Small-world property indicated a network with high global efficiency and local efficiency (18).
In addition, edges of the three white matter connectivity matrices (FA, FN, and FL) between HTA and LTA groups were compared to identify alterations in fiber connection. The different connections were displayed using Brainnet viewer software (19).
Statistical Analysis
Differences in structural network properties (Sigma, Eg, Eloc, Cp, and Lp) and white matter connectivities between HTA and LTA groups were assessed by two-sample t-test. The false discovery rate (FDR) correction for multiple comparisons was used. In addition, given that depression may influence the results, the Beck Depression Inventory (BDI) score was regarded as a covariate. The above analysis was performed by GRETNA software.
Results
Demographic Characteristics and Behavioral Data
Demographic characteristics and behavioral data of the subjects were compared (Table 1). No significant differences in age, gender, and CRT were observed between HTA and LTA groups.
Alterations in Structural Networks
The topological properties of the three structural networks were not significantly different. However, some altered connections were detected in FA- and FN-weighted networks.
Fractional Anisotropy-Weighted Network
Twenty-five connections featured by FA value decreased significantly in the HTA group compared with the LTA group (Table 2 and Figure 2) (p < 0.01, FDR corrected). The most different connections primarily comprised the connectivity of the left inferior orbitofrontal gyrus to the right inferior frontal gyrus (triangular), the right superior orbitofrontal gyrus to the right hippocampus, the right medial orbitofrontal gyrus to the right lenticular nucleus (putamen), the left hippocampus to the left posterior cingulate gyrus, the left thalamus, the right calcarine, the right superior temporal gyrus to the left posterior cingulate gyrus, and the right middle occipital gyrus to the right precentral gyrus (p < 0.001, FDR corrected).
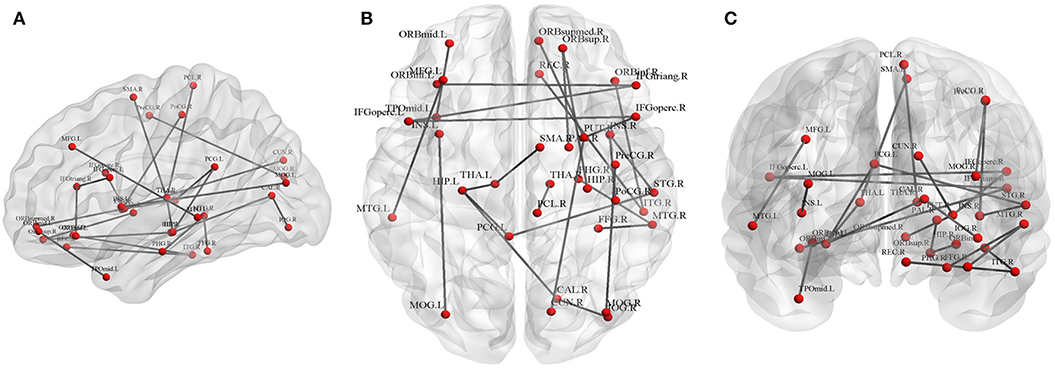
Figure 2. Graphical representation of different connections in fractional anisotropy (FA)-weighted network. (A) Sagittal plane, (B) axial plane, and (C) coronal plane.
Fiber Number-Weighted Network
Twenty connections were detected to have differences (p < 0.01, FDR corrected, Table 3 and Figure 3). The most different connections primarily comprised the connectivity of the left inferior frontal gyrus (opercular) to the right inferior frontal gyrus (opercular), the right inferior frontal gyrus (triangular) to the left inferior orbitofrontal gyrus, the right superior orbitofrontal gyrus to the right hippocampus, the left hippocampus to the right calcarine and the left posterior cingulate gyrus, the right middle occipital gyrus to the right precentral gyrus, and the right superior temporal gyrus to the left posterior cingulate gyrus (p < 0.001, FDR corrected).
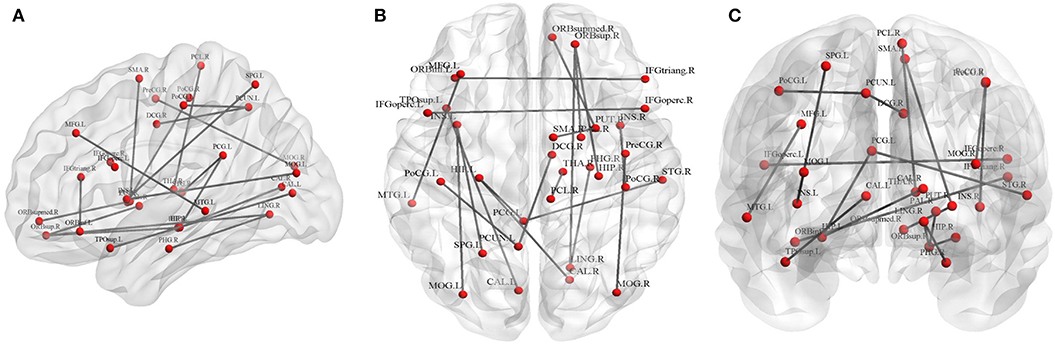
Figure 3. Graphical representation of different connections in fiber number (FN)-weighted network. (A) Sagittal plane, (B) axial plane, and (C) coronal plane.
Discussion
To our knowledge, the investigation on white matter structural connectivity in young healthy people with high trait anxiety is limited. Our group previously used tract-based spatial statistics method to detect the changes of white matter in young healthy populations with high trait anxiety (8). In this study, our results showed widespread abnormal connections in brain regions, but no significant difference in the network property was detected. Alterations in white matter structural connectivity were primarily located in the inter-hemispheric frontal lobe, the frontal-limbic lobe in the right hemisphere, and the frontal-temporal lobe in the ipsilateral hemisphere.
Patients with generalized anxiety disorder exhibited decreased brain signal variability in widespread regions, including the visual network, the sensorimotor network, the fronto-parietal network, the limbic system, and the thalamus (20). It should be noted that in our study, all subjects are young healthy populations. Although the HTA group has a higher trait anxiety score, they are still healthy populations, and their brains have no significant decline yet in ability their deliver global information. It may be the primary reason why the global properties of the structural brain networks did not show alterations. The abnormal connections may only influence the local information transmission a bit or even has no significant influence. Another reason may be that they are young adults aged only 20 years, and their brains will have more plasticity during their development or maturity. At the early stage, the global network properties have no such sensitivity as the biomarker to describe the brain structural change. However, the results showed that some different connections occurred in FA and FN networks. These different connections may support to elucidate our understanding of the pathological mechanism of trait anxiety in young populations.
Thalamus-Related Connections
In our previous voxel-wise study on highly anxious population, alterations in the anterior corona radiata (ACR)–thalamus pathway have been detected, as manifested by decreased FA values in the bilateral corona radiata and anterior thalamic radiation regions. This study also showed abnormal connectivity between the left hippocampus and the thalamus. The cortical–thalamus–limbic pathway is closely associated with emotional behavior and regulation related to posttraumatic stress disorder (21). Giménez et al. found that patients with social anxiety disorder showed significant functional connections between the thalamo-cortical and fronto-striatal circuits with task induction (22). These findings suggest that thalamus-related connections may be a vulnerable marker in young healthy individuals with high anxiety.
Temporal Lobe/Hippocampus
A large number of studies reported the importance of the hippocampus/temporal lobe for negative emotionality. Montag et al. found that four white matter tracts linking the temporal lobe/hippocampus to other brain regions were strongly correlated with trait anxiety in male participants only (23). Similarly, we demonstrated that population with high anxiety manifested significant abnormalities in 11 white matter tracts linking the temporal lobe/hippocampus to several brain regions. This study also demonstrated the abnormal connectivity of the hippocampus regions to the posterior cingulate gyrus in highly anxious populations.
Insula
The main function of the insula is emotional processing. Baur et al. stated that the anterior insula and basolateral amygdala constitute a network that is significantly related to anxiety (6). They also supposed that the resting-state functional connection of the anterior insula to the basolateral amygdala was highly related to anxiety. Hamm et al. found that the connection of the right amygdala to the insula showed significantly increased connectivity among pediatrics with anxiety disorders (24). Yang et al. found the abnormal functional architecture of the supramarginal gyrus network and the superior parietal gyrus network in patients with anxiety disorder (25). Dennis et al. found that the enhanced connection of the left anterior insula to the default network in healthy populations with anxiety, but connections of the parahippocampal and posterior cingulate gyrus to the default network increased in adults but not in youth (26). In addition, correlations were reported between insula activity and Liebowitz Social Anxiety Scale (LSAS) (27) or Social Phobia Inventory (SPIN) (28). We also found that the connections of the insula to the occipital and parietal lobes showed abnormality in the FA- and FN-weighted networks (the left insula to the left middle occipital gyrus, the right insula to the right postcentral gyrus in FA-weighted network, the left insula to the left superior parietal gyrus, the right insula to the right postcentral gyrus, and the left insula to left middle occipital gyrus in the FN-weighted network).
Frontal Cortex and Limbic Areas
The orbitofrontal cortex and the prefrontal cortex located in the anterior–inferior frontal lobe play a crucial role in modulation of fear via the amygdala (29). Baur et al. found that the volume of the left uncinate fasciculus decreased in individuals with social anxiety disorder; this finding suggests deficient structural connectivity from high-level control areas in the orbitofrontal cortex to more basal limbic areas, such as the amygdala (30). A study on functional and structural connectivity demonstrated the relationship between trait anxiety and axial diffusivity and reported a direct pathway from the anterior insula to the basolateral amygdala (6). In our study, the observed abnormalities of several frontal lobe connections comprising connectivity linking the orbitofrontal to the limbic regions may be a trait marker in white matter structural network for young healthy individuals. In the FA- and FN-weighted network, individuals with high anxiety manifested abnormalities in the three similar pathways, including the connections of the right superior frontal gyrus (orbital part) to the limbic regions (right hippocampus, pallidum, and putamen).
Basten et al. found that some regions (inferior frontal junction areas, dorsal anterior cingulate gyrus, and left fusiform gyrus) showed significantly weaker task-specific coupling for highly anxious subjects than for those with low anxiety level (31). Kim et al. found a negative functional connectivity of amygdala–dorsal to medial prefrontal cortex in subjects with high anxiety and a positive correlation with activity at rest in subjects with low anxiety (32). Hamm et al. found that anxiety disorders showed a high connection between the left amygdala and the ventromedial prefrontal cortex and posterior cingulate cortex (24). A meta-analysis of fMRI studies investigating emotional processing in individuals who excessively worry demonstrated the convergent abnormalities at the middle frontal gyrus, the inferior frontal gyrus, and the anterior insula compared with normal controls (33). During anticipation of uncertain threat, individuals with high trait anxiety level showed significantly abnormal functional activation in the thalamus, middle temporal gyrus, dorsomedial prefrontal cortex, and precuneus (34). Du et al. found that functional connectivity changed in the frontal–limbic–striatal and default-mode networks (35). In predicting uncertain threats for individuals with high trait anxiety level, it was found that the activation of the thalamus, the medial temporal gyrus, and the dorsolateral prefrontal cortex increased significantly, whereas that of the precuneus decreased (34). We also found aberrant connections in precuneus regions in high anxiety populations through an analysis of the FN-weighted network.
Amygdala
The amygdala is a key center for processing threats and plays an important role in generation, recognition, and regulation of emotions. The amygdala is located on the medial dorsal side of the anterior temporal lobe and is a part of the limbic system. Functional brain imaging studies showed that the level of amygdala activation was higher in anxious patients than in healthy controls; moreover, increased amygdala response in clinical and healthy individuals was associated with trait anxiety (36). Makovac et al. found reduced connections of the amygdala to the prefrontal cortex in people with generalized anxiety disorders (36). Similarly, Xue et al. found that high state anxiety level was associated with decreased connectivity of the amygdala to the precuneus/posterior cingulate cortex in adults with anxiety disorders (37).
In their fMRI study, Bishop et al. found that threat-related distractors activated the rostral anterior cingulate cortex in patients with anxiety. In addition, the rostral anterior cingulate cortex and the functions of lateral–prefrontal cortex activities decreased in participants with high anxiety level (38). Similarly, the medial prefrontal cortex and anterior cingulate cortex play important roles in coping with negative emotional stimuli (emotional processing in anterior cingulate and medial prefrontal cortex). Klumpp et al. found that the bilateral anterior insula of patients with generalized social anxiety disorder exhibited strong responses to fear (39). Yang et al. found that functional connectivity among the dorsolateral prefrontal cortex, the ventral medial prefrontal cortex, and the limbic regions was enhanced in the frontal marginal circuit of emotional regulation in patients with social anxiety disorder (40). Jacob et al. found that in people with social anxiety disorders, emotion was associated with decreased connectivity between the amygdala and the prefrontal cortex (41). A task-based fMRI study concerning social anxiety disorders reported abnormal emotion processing areas (e.g., amygdala and insula) (42). However, other scholars believed that the nature of trait anxiety is related to attitudes and strategies and not to situational incentives (4). On the contrary, our results showed no abnormalities of the connections associated with the amygdala. Although the amygdala was an important brain region for anxiety disorders, it has not been structurally altered in healthy people with trait anxiety.
This study has several limitations. First, we only evaluated Chinese undergraduate students who were right-handed and had no history of mental illness. The other similar young healthy populations like those with a relatively low educational level may have results that contrast the current results. Second, the sample size of the current study is still not large enough, which may influence the precision of the results. Third, our study did not report the correlation of functional connection with our structural connection results. Nevertheless, we believe that our data provide strong support for white matter structural connectivity in young healthy individuals with high anxiety level. Results may be helpful to develop interventions before the manifestation of morbidity in young individuals with anxious disorders and to establish a microstructural biomarker of anxiety-related diseases.
Data Availability Statement
Publicly available datasets were analyzed in this study. This data can be found here: The Southwest University Longitudinal Imaging Multimodal, Brain Data Repository (http://fcon_1000.projects.nitrc.org/indi/retro/southwestuni_qiu_index.html).
Ethics Statement
This study was carried out in accordance with the recommendations of the Declaration of Helsinki (1989) and the Research Ethics Committee of the Brain Imaging Center of Southwest University. The protocol was approved by the Research Ethics Committee of the Brain Imaging Center of Southwest University. All subjects gave written informed consent in accordance with the Declaration of Helsinki.
Author Contributions
CY and YZ made contributions to the conception, design, and analysis of DTI data and drafted the manuscript. ML interpreted and discussed the DTI data. JR and ZL made contributions to the revision of the final manuscript. All authors read and approved the final manuscript.
Funding
This work was supported by Beijing Nova Program (CN) (no. Z161100004916157); the National Natural Science Foundation of China (nos. 31640035, 71661167001, and 81601126); the Beijing Municipal Commission of Education (no. PXM2017_014204_500012); the Natural Science Foundation of Beijing (no. 4162008); and research fund for CAAE-UCB program, no. 2017010.
Conflict of Interest
The authors declare that the research was conducted in the absence of any commercial or financial relationships that could be construed as a potential conflict of interest.
Acknowledgments
Thanks to the Intelligent Physiological Measurement and Clinical Translation, Beijing International Base, for their scientific and technological cooperation. Similarly, thanks also to the Southwest University Longitudinal Imaging Multimodal, Brain Data Repository (China).
Abbreviations
AAL, Automated Anatomical Labeling; ACR, anterior corona radiata; BDI, Beck Depression Inventory; CRT, combined Raven's matrix test; DTI, diffusion tensor imaging; EEG, electroencephalography; FA, fractional anisotropy; FDR, false discovery rate; FL, fiber length; fMRI, functional magnetic resonance imaging; FN, fiber number; HTA, high trait anxiety; LSAS, Liebowitz Social Anxiety Scale; LTA, low trait anxiety; MNI, Montreal Neurological Institute; MRI, magnetic resonance imaging; SPIN, Social Phobia Inventory.
References
1. Hsiao CY, Tsai HC, Chi MH, Chen KC, Chen PS, Lee IH, et al. The Association between baseline subjective anxiety rating and changes in cardiac autonomic nervous activity in response to tryptophan depletion in healthy volunteers. Medicine. (2016) 95:e3498. doi: 10.1097/MD.0000000000003498
2. Dodd HF, Vogt J, Turkileri N, Notebaert L. Task relevance of emotional information affects anxiety-linked attention bias in visual search. Biol Psychol. (2017) 122:13–20. doi: 10.1016/j.biopsycho.2016.01.017
3. Zhu H, Qiu C, Meng Y, Yuan M, Zhang Y, Ren Z, et al. Altered topological properties of brain networks in social anxiety disorder: a resting-state functional MRI study. Sci Rep. (2017) 7:43089. doi: 10.1038/srep43089
4. Pacheco-Unguetti AP, Acosta A, Callejas A, Lupianez J. Attention and anxiety: different attentional functioning under state and trait anxiety. Psychol Sci. (2010) 21:298–304. doi: 10.1177/0956797609359624
5. Liao W, Xu Q, Mantini D, Ding J, Machado-de-Sousa JP, Hallak JE, et al. Altered gray matter morphometry and resting-state functional and structural connectivity in social anxiety disorder. Brain Res. (2011) 1388:167–77. doi: 10.1016/j.brainres.2011.03.018
6. Baur V, Hänggi J, Langer N, Jäncke L. Resting-state functional and structural connectivity within an insula–amygdala route specifically index state and trait anxiety. Biol Psychiatr. (2013) 73:85–92. doi: 10.1016/j.biopsych.2012.06.003
7. Xing M, Tadayonnejad R, MacNamara A, Ajilore O, Phan KL, Klumpp H, Leow A. EEG based functional connectivity reflects cognitive load during emotion regulation. In: IEEE 13th International Symposium on Biomedical Imaging (ISBI). Chicago, IL (2016). doi: 10.1109/isbi.2016.7493380
8. Lu M, Yang C, Chu T, Wu S. Cerebral white matter changes in young healthy individuals with high trait anxiety: a tract-based spatial statistics study. Front Neurol. (2018) 9:704. doi: 10.3389/fneur.2018.00704
9. Liu W, Wei D, Chen Q, Yang W, Meng J, Wu G, et al. Longitudinal test-retest neuroimaging data from healthy young adults in southwest China. Sci Data. (2017) 4:170017. doi: 10.1038/sdata.2017.17
10. Spielberger CD, Gorsuch RL. Manual for the State-Trait Anxiety Inventory (form Y): (“Self-Evaluation Questionnaire”). Palo Alto, CA: Consulting Psychologists Press, Incorporated (1983).
11. Li D, Hu KD, Chen GP, Jin Y, Li M. The testing results report on the combined Raven's test in Shanghai. Psychol Sci. (1988) 4:27–31.
12. Eden AS, Schreiber J, Anwander A, Keuper K, Laeger I, Zwanzger P, et al. Emotion regulation and trait anxiety are predicted by the microstructure of fibers between amygdala and prefrontal cortex. J Neurosci. (2015) 35:6020–7. doi: 10.1523/JNEUROSCI.3659-14.2015
13. Cui Z, Zhong S, Xu P, Gong G, He Y. PANDA: a pipeline toolbox for analyzing brain diffusion images. Front Hum Neurosci. (2013) 7:42. doi: 10.3389/fnhum.2013.00042
14. Tzourio-Mazoyer N, Landeau B, Papathanassiou D, Crivello F, Etard O, Delcroix N, et al. Automated anatomical labeling of activations in spm using a macroscopic anatomical parcellation of the MNI MRI single-subject brain. NeuroImage. (2002) 15:273–89. doi: 10.1006/nimg.2001.0978
15. Li Y, Liu Y, Li J, Qin W, Li K, Yu C, et al. Brain anatomical network and intelligence. NeuroImage. (2009) 5:e1000395. doi: 10.1371/journal.pcbi.1000395
16. Wang J, Wang X, Xia M, Liao X, Evans A, He Y. GRETNA: a graph theoretical network analysis toolbox for imaging connectomics. Front Hum Neurosci. (2015) 9:386. doi: 10.3389/fnhum.2015.00386
17. Rubinov M, Sporns O. Complex network measures of brain connectivity: uses and interpretations. NeuroImage. (2010) 52:1059–69. doi: 10.1016/j.neuroimage.2009.10.003
18. Latora V, Marchiori M. Efficient behavior of small-world networks. Phys Rev Lett. (2001) 87:198701. doi: 10.1103/PhysRevLett.87.198701
19. Xia M, Wang J, He Y. BrainNet viewer: a network visualization tool for human brain connectomics. PLoS ONE. (2013) 8:e68910. doi: 10.1371/journal.pone.0068910
20. Li L, Wang Y, Ye L, Chen W, Huang X, Cui Q, et al. Altered brain signal variability in patients with generalized anxiety disorder. Front Psychiatr. (2019) 10:84. doi: 10.3389/fpsyt.2019.00084
21. Schuff N, Zhang Y, Zhan W, Lenoci M, Ching C, Boreta L, et al. Patterns of altered cortical perfusion and diminished subcortical integrity in posttraumatic stress disorder: an MRI study. NeuroImage. (2011) 54:S62–8. doi: 10.1016/j.neuroimage.2010.05.024
22. Giménez M, Pujol J, Ortiz H, Soriano-Mas C, López-Solà M, Farré M, et al. Altered brain functional connectivity in relation to perception of scrutiny in social anxiety disorder. Psychiatr Res Neuroimaging. (2012) 202:214–23. doi: 10.1016/j.pscychresns.2011.10.008
23. Montag C, Reuter M, Weber B, Markett S, Schoene-Bake JC. Individual differences in trait anxiety are associated with white matter tract integrity in the left temporal lobe in healthy males but not females. Neuroscience. (2012) 217:77–83. doi: 10.1016/j.neuroscience.2012.05.017
24. Hamm LL, Jacobs RH, Johnson MW, Fitzgerald DA, Fitzgerald KD, Langenecker SA, et al. Aberrant amygdala functional connectivity at rest in pediatric anxiety disorders. Biol Mood Anxiety Disord. (2014) 4:15. doi: 10.1186/s13587-014-0015-4
25. Yang F, Fan L, Zhai T, Lin Y, Wang Y, Ma J, et al. Decreased intrinsic functional connectivity in first-episode, drug-naive adolescents with generalized anxiety disorder. Front Hum Neurosci. (2019) 12:539. doi: 10.3389/fnhum.2018.00539
26. Dennis EL, Gotlib IH, Thompson PM, Thomason ME. Anxiety modulates insula recruitment in resting-state functional magnetic resonance imaging in youth and adults. Brain Connectivity. (2011) 1:245–54. doi: 10.1089/brain.2011.0030
27. Liebowitz MR. Social phobia. Modern problems of pharmacopsychiatry. (1987) 22:141–73. doi: 10.1159/000414022
28. Connor KM, Davidson JR, Churchill LE, Sherwood A, Foa E, Weisler RH. Psychometric properties of the Social Phobia Inventory (SPIN). Br J Psychiatr. (2000) 176:379–86. doi: 10.1192/bjp.176.4.379
29. Quirk GJ, Likhtik E, Pelletier JG, Paré D. Stimulation of medial prefrontal cortex decreases the responsiveness of central amygdala output neurons. J Neurosci. (2003) 23:8800–7. doi: 10.1523/JNEUROSCI.23-25-08800.2003
30. Baur V, Brühl AB, Herwig U, Eberle T, Rufer M, Delsignore A, et al. Evidence of frontotemporal structural hypoconnectivity in social anxiety disorder: a quantitative fiber tractography study. Hum Brain Mapp. (2011) 34:437–46. doi: 10.1002/hbm.21447
31. Basten U, Stelzel C, Fiebach CJ. Trait anxiety modulates the neural efficiency of inhibitory control. J Cogn Neurosci. (2011) 23:3132–45. doi: 10.1162/jocn_a_00003
32. Kim MJ, Gee DG, Loucks RA, Davis FC, Whalen PJ. Anxiety dissociates dorsal and ventral medial prefrontal cortex functional connectivity with the amygdala at rest. Cereb Cortex. (2010) 21:1667–73. doi: 10.1093/cercor/bhq237
33. Weber-Goericke F, Muehlhan M. A quantitative meta-analysis of fMRI studies investigating emotional processing in excessive worriers: application of activation likelihood estimation analysis. J Affect Disord. (2018) 243:348–59. doi: 10.1016/j.jad.2018.09.049
34. Geng H, Wang Y, Gu R, Luo YJ, Xu P, Huang Y, et al. Altered brain activation and connectivity during anticipation of uncertain threat in trait anxiety. Hum Brain Mapp. (2018) 39:3898–914. doi: 10.1002/hbm.24219
35. Du MY, Liao W, Lui S, Huang XQ, Li F, Kuang WH, Gong QY. Altered functional connectivity in the brain default-mode network of earthquake survivors persists after 2 years despite recovery from anxiety symptoms. Soc Cogn Affect Neurosci. (2015) 10:1497–505. doi: 10.1093/scan/nsv040
36. Makovac E, Mancini M, Fagioli S, Watson DR, Meeten F, Rae CL, et al. Network abnormalities in generalized anxiety pervade beyond the amygdala-pre-frontal cortex circuit: Insights from graph theory. Psychiatr Res Neuroimaging. (2018) 281:107–16. doi: 10.1016/j.pscychresns.2018.09.006
37. Xue S, Lee T, Guo Y. Spontaneous activity in medial orbitofrontal cortex correlates with trait anxiety in healthy male adults. J Zhejiang Univ Sci B. (2018) 19:643–53. doi: 10.1631/jzus.B1700481
38. Bishop S, Duncan J, Brett M, Lawrence AD. Prefrontal cortical function and anxiety: controlling attention to threat-related stimuli. Nat Neurosci. (2004) 7:184–8. doi: 10.1038/nn1173
39. Klumpp H, Angstadt M, Phan KL. Insula reactivity and connectivity to anterior cingulate cortex when processing threat in generalized social anxiety disorder. Biol Psychol. (2012) 89:273–6. doi: 10.1016/j.biopsycho.2011.10.010
40. Yang X, Liu J, Meng Y, Xia M, Cui Z, Wu X, et al. Network analysis reveals disrupted functional brain circuitry in drug-naive social anxiety disorder. NeuroImage. (2017) 190:213–23. doi: 10.1016/j.neuroimage.2017.12.011.
41. Jacob Y, Shany O, Goldin PR, Gross JJ, Hendler T. Reappraisal of interpersonal criticism in social anxiety disorder: a brain network hierarchy perspective. Cereb Cortex. (2018) 29:3154–67. doi: 10.1093/cercor/bhy181
Keywords: high anxiety populations, diffusion tensor imaging, white matter, structural network, connectivity, young healthy individuals
Citation: Yang C, Zhang Y, Lu M, Ren J and Li Z (2020) White Matter Structural Brain Connectivity of Young Healthy Individuals With High Trait Anxiety. Front. Neurol. 10:1421. doi: 10.3389/fneur.2019.01421
Received: 16 September 2019; Accepted: 30 December 2019;
Published: 13 February 2020.
Edited by:
Jan Kassubek, University of Ulm, GermanyReviewed by:
Costanza Giannì, Sapienza University of Rome, ItalySalem Hannoun, American University of Beirut, Lebanon
Copyright © 2020 Yang, Zhang, Lu, Ren and Li. This is an open-access article distributed under the terms of the Creative Commons Attribution License (CC BY). The use, distribution or reproduction in other forums is permitted, provided the original author(s) and the copyright owner(s) are credited and that the original publication in this journal is cited, in accordance with accepted academic practice. No use, distribution or reproduction is permitted which does not comply with these terms.
*Correspondence: Min Lu, bWlubHUxOTk1JiN4MDAwNDA7MTYzLmNvbQ==