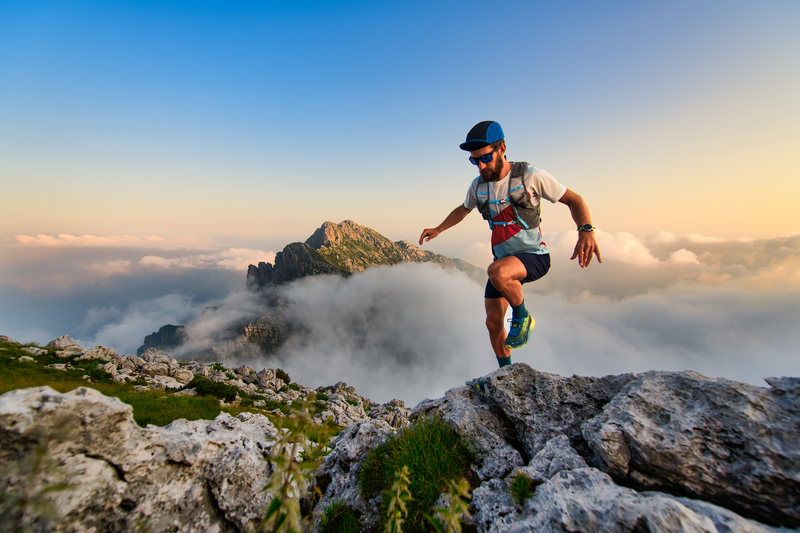
95% of researchers rate our articles as excellent or good
Learn more about the work of our research integrity team to safeguard the quality of each article we publish.
Find out more
REVIEW article
Front. Neurol. , 12 November 2019
Sec. Stroke
Volume 10 - 2019 | https://doi.org/10.3389/fneur.2019.01106
Introduction: Recurrent stroke has a higher rate of death and disability. A number of risk scores have been developed to predict short-term and long-term risk of stroke following an initial episode of stroke or transient ischemic attack (TIA) with limited clinical utilities. In this paper, we review different risk score models and discuss their validity and clinical utilities.
Methods: The PubMed bibliographic database was searched for original research articles on the various risk scores for risk of stroke following an initial episode of stroke or TIA. The validation of the models was evaluated by examining the internal and external validation process as well as statistical methodology, the study power, as well as the accuracy and metrics such as sensitivity and specificity.
Results: Different risk score models have been derived from different study populations. Validation studies for these risk scores have produced conflicting results. Currently, ABCD2 score with diffusion weighted imaging (DWI) and Recurrence Risk Estimator at 90 days (RRE-90) are the two acceptable models for short-term risk prediction whereas Essen Stroke Risk Score (ESRS) and Stroke Prognosis Instrument-II (SPI-II) can be useful for prediction of long-term risk.
Conclusion: The clinical risk scores that currently exist for predicting short-term and long-term risk of recurrent cerebral ischemia are limited in their performance and clinical utilities. There is a need for a better predictive tool which can overcome the limitations of current predictive models. Application of machine learning methods in combination with electronic health records may provide platform for development of new-generation predictive tools.
Personalized medicine is dependent on data science and predictive analytics. This allows care providers to receive important alerts about potential events before they happen, and therefore make an informed decision. Predictive modeling of diseases that have a long-term effect can be crucial due to high individual and societal impact. Therefore, stroke as a leading cause of long-term disability is an essential target (1). It is vital and might be more practical to identify patients who are at a higher risk of recurrent cerebral ischemia. Recurrent stroke has a higher rate of death and disability and the long-term risk of recurrent ischemic stroke cannot be reliably assessed.
To date, a number of risk scores have been developed to predict short-term and long-term stroke recurrence after an initial episode of stroke or risk of stroke after transient ischemic attack (TIA). In this paper, we review different risk score models and discuss their validity and clinical utilities in the current clinical environment.
The PubMed bibliographic database was searched for articles published on the various risk scores for recurrence of stroke. Search terms included: predictive scores stroke, predictive scores TIA, risk score stroke recurrence. We also explored the reference lists of the identified articles. All the relevant risk score models [e.g., ABCD, Essen Stroke Risk Score (ESRS)] were searched in the PubMed database. Studies were eligible for inclusion if they were original research articles in a peer-reviewed journal and were clinically applicable. Validation studies were required to have a follow-up of the reported cohort. The validation of the models was evaluated by examining the internal and external validation process. We also examined the statistical methodology, sample size, and the study power; as well as assessed the accuracy, and metrics such as sensitivity, specificity, and the receiver-operating-characteristic curve (ROC) (2). We paid attention to study population (general population- vs. hospital-based cohort and specialized center cohorts), study inclusion and exclusion criteria, and the outcome definition and measurement.
We identified 71 original articles describing, validating, or studying 16 risk scores to predict short-term or long-term recurrent cerebral ischemia (Tables 1, 5). Each study was performed in different study populations and employed different methodology. The included studies had different inclusion and exclusion criteria. Many of the studies considered death as an outcome.
SPI-I (Stroke Prognosis Instrument-I) was developed in 1991 as a prognostic system for patients with a carotid transient ischemic attack (TIA) or minor stroke to estimate the risk of stroke or death within 2 years (3).
The initial prognostic system consisted of three factors- age > 65 years (3 points), diabetes mellitus (3 points), and hypertension (2 points); and three risk groups- risk group I (0 points), II (1–5 points), and III (6–8 points). The development of the system was done in a cohort of 142 patients (Yale cohort) (3) in which the rate of stroke or death was found to be 2, 31, and 54% in the three risk groups, respectively (P < 0.0001). The initial system was testeds in another independent cohort of 330 patients (Canadian cohort) (3) in which the outcome rates were found to be 12, 21, and 31% in the three groups, respectively (P = 0.04). It was concluded that the initial system did not work in the second cohort as well as it did in the initial cohort. The high outcome rate in the first risk group (group I) in the Canadian cohort was of concern. Also, the outcome rates in the risk groups II and III were comparatively less in the Canadian cohort than in the initial cohort. To overcome these differences, the addition of coronary heart disease (1 point) and the distinction between TIA and stroke at baseline event (2 points) to the initial system led to the final SPI-I as given in Table 2.
This final SPI-I did well in both Yale cohorts (outcome rates of 3, 27, and 48% P < 0.001) and Canadian cohorts (outcome rates of 10, 21, and 59%, P < 0.001) in the three groups, although the outcome rate in risk group I of Canadian cohort remained high. The difference in performance in the two cohorts was attributed to the probable inaccuracy in classifying coronary heart disease in the Canadian cohort (3).
SPI-I provided a convenient prognostic scoring system using clinical parameters which were readily available. The outcome rates among the three risk groups were large, and it was reproduced in an independent cohort (3). Despite this, the developers of SPI-I suggested that more studies were needed to confirm that the weights assigned to the risk factors of SPI-I were accurate. This eventually led to the creation of SPI-II. Also, stroke alone was not considered in SPI-I because excluding early mortality could lead to false stroke risk outcome. It also did not distinguish between ischemic stroke and hemorrhagic stroke in the stroke outcome. Further validation of SPI-I came in 4 independent cohorts during the development of SPI-II. SPI-I performed fairly in these validation cohorts, but SPI-II outperformed SPI-I (4).
SPI-II is a revised version of SPI-I that was introduced and internally validated by the same investigators in 2000. SPI-II was intended to predict the risk of stroke or death within 2 years of TIA or ischemic stroke. This clinical score included two more risk factors—congestive heart failure and prior stroke. The points assigned to different risk factors were also modified in SPI-II. The risk factors of SPI-II and allocated points are detailed in Table 2. This modified risk score is the result of the work done to validate and improve SPI-I in 4 test cohorts. These cohorts were from Women's Estrogen for Stroke Trial (WEST), United Kingdom Transient Ischemic Attack (UK-TIA) Aspirin Trial, the Clopidogrel vs. Aspirin in Patients at Risk of Ischemic Events (CAPRIE) Trial and the Northern Manhattan Stroke Study (NoMaSS). Data from the WEST cohort was used to recognize new variables to develop SPI-II, and the other three cohorts were used to validate the score (4).
The pooled rates of stroke or death within 2 years (in the 3 cohorts excluding the cohort used to develop SPI-II) for SPI-I were 9, 17, and 23% in the three risk groups (Risk group I: 2,430 patients, II: 5,411 patients, III: 1,379 patients), respectively. For SPI-II, the pooled rates were 10, 19, and 31% in the three risk groups (Risk group I: 4,725 patients, II: 3,314 patients, III: 1,181 patients), respectively. In receiver operator characteristic (ROC) analysis, AUC for SPI-II was 0.63 (95% CI, 0.62–0.65) as compared to SPI-I whose AUC was 0.59 (95% CI, 0.57–0.60) verifying that SPI-II performed better than SPI-I. Nevertheless, the area under the curve of ROC analysis was only marginally better. In addition, it does not seem that SPI-II is performing better among patients in the lowest-risk group. Both SPI-I and II do not take stroke type and aortic plaque into account—two factors which might affect prognosis. Both SPI-I and II were developed and validated in research cohorts retrospectively, and their application in a non-research setting might differ. It should also be emphasized that the application of SPI scores are restricted to patients with carotid territory TIA or minor stroke.
In a large, prospective, community-based cohort of 5,575 patients, the C-statistic for SPI-II was 0.62 (95% CI, 0.61–0.64) (13). In another cohort of 1,897 patients with acute non-disabling ischemic stroke or TIA from German stroke centers with a median follow up of 1 year (14), SPI-II performed marginally better (recurrent stroke AUC 0.65 [95%CI, 0.6–0.7]; recurrent stroke or cardiovascular death AUC 0.66 [95% CI, 0.61–0.70]) than Essen Stroke Risk Score (ESRS), Hankey and Life-Long after Cerebral Ischemia (LiLAC) scores.
On the other hand, in a validation cohort of 592 patients with TIA or minor stroke, SPI-II overestimated the risk of outcome in low-risk patients and underestimated the risk in the high-risk group (discrimination C-statistics 0.64 [95% CI, 056–0.72) (15). In a population-based study in Oxfordshire, UK, SPI-II performed poorly in predicting the short-term risk of recurrent stroke (16), which is unsurprising because SPI-II was intended to predict long-term risk of stroke or death. Overall, SPI-II has fair AUROC/c-statistics (0.6–0.72) in these studies (13–15) for long-term risk prediction. But the SPI-II score is a poor model for predicting the short-term risk of stroke.
Essen Stroke Risk Score (ESRS) (5) was developed from the data of subgroup of stroke patients from the CAPRIE trial (17) to predict 1 year risk of recurrent stroke. The parameters of ESRS and points assigned to these parameters and the separation of patients into low- and high-risk groups are detailed in Table 3.
In a validation cohort of 852 patients with acute ischemic stroke or TIA in German stroke units, patients with an ESRS >= 3 (compared to patients with ESRS <3) had a significantly higher risk of recurrent stroke or cardiovascular death (9.7% vs. 5.1%; OR 2.00, 95% CI, 1.08–3.70) (18). Further validation came from a large cohort of 15,605 outpatients with previous TIA or stroke from the Reduction of Atherosclerosis for Continued Health (REACH) registry (19), in which ESRS accurately stratified the risk of recurrent stroke or major vascular events. On the other hand, ESRS (and SPI-II) performed poorly in predicting short-term recurrent stroke risk in a population-based study in Oxfordshire, United Kingdom (16).
Performance of SPI-II (recurrent stroke AUC 0.65 [95% CI, 0.60–0.70]; combined recurrent stroke or cardiovascular death AUC 0.66 [95% CI, 0.61–0.70]) was marginally superior to ESRS (recurrent stroke AUC 0.62 [95% CI, 0.57–0.67]; combined recurrent stroke or cardiovascular death AUC 0.65 [95% CI, 0.60–0.69] as shown in a prospective study of German stroke center cohort (14) but ESRS has been validated in a stable outpatient population with cerebrovascular disease. In another study (20) of 730 patients with TIA or ischemic stroke, the AUC for ESRS was 0.59. In a Chinese cohort of 11,384 patients with TIA or ischemic stroke, ESRS and SPI-II had similar predictive value (21). However one study found ESRS had a superior AUC compared to SPI-II (0.677 vs. 0.553) and even concluded that ESRS might be suitable for short-term prediction of stroke despite a very small sample size (n = 167) (22). In a prospective Chinese cohort of 3,316 outpatients with ischemic stroke, ESRS had c-statistics of 0.63 (0.57–0.69) for recurrent stroke and 0.63 (0.58–0.68) for combined vascular events (23).
A modified ESRS was generated by adding scores for waist circumference, stroke subtype categorized by etiology, gender to the ESRS and it was validated using a large prospective cohort of 3,588 patients (EVEREST cohort) with ischemic stroke in Japan (24). The C-statistics for recurrent ischemic stroke was 0.632 (95% CI, 0.579–0.684) in the modified ESRS and was 0.604 (95% CI, 0.554–0.654) for the original ESRS. For cardiovascular events, c-statistics were 0.64 (95% CI, 0.590–0.661) in the modified ESRS and 0.613 (95% CI, 0.564–0.689) for ESRS. While modified ESRS showed better c-statistics, there were limitations to this study. The incidence of recurrent ischemic stroke was low (121 events in 3,292 patients) and it was not confirmed in a validation cohort although the EVEREST data was externally validated using a cross-validation method.
Recurrence Risk Estimator at 90 days (RRE-90) is a web-based prognostic scoring system designed to predict 90 day recurrent stroke risk in patients presenting with ischemic stroke. RRE-90 actually consists of 2 models- model A is a clinical-based model, whereas model B takes both clinical and imaging findings into account (6). The parameters included in RRE-90 were a history of TIA or stroke within the month preceding index stroke, admission stroke subtype according to Causative Classification of Stroke System (CCS), and MRI imaging findings—isolated cortical infarcts, multiple acute infarcts, simultaneous infarcts in different vascular territories, and multiple infarcts of different ages. Some of the vascular risk factors such as hypertension and diabetes, which have been associated with stroke and included in many other scoring systems have not been included in RRE-90.
The primary derivation cohort of RRE-90 consisted of 1,458 patients with ischemic stroke identified retrospectively. RRE-90 demonstrated good discrimination not only in the initial study (AUC: 0.7–0.8) but also in another cohort of 433 patients (AUC: 0.7–0.76) (6). Nevertheless, the retrospective design, incomplete follow-up (half of the patients completed the follow-up), and single hospital setting were some of the limitations of this study.
The validity of RRE-90 was tested in 1,468 patients with MRI-confirmed acute ischemic stroke from 3 hospital-based cohorts (the United States, Brazil, and South Korea) (25) and AUC ROC curve for discrimination was found to be 0.76 (95% CI, 0.70–0.82). In a retrospective study of 257 consecutive patients with DWI-positive TIA, the sensitivity and specificity of an RRE score of ≥2 for predicting 7 day stroke risk were 87 and 73%, respectively (AUC: 0.85, 95% CI, 0.78–0.92) (26). In a prospective study, c-statistic of RRE-90 for 90 days recurrence was 0.681 (95% CI, 0.592–0.771) (27). Both of these studies showed better discrimination for RRE-90 compared to the ABCD2 score. However, the study population was limited to patients with DWI-positive TIA or minor stroke. Compared to some other scoring systems including ABCD2 score, it seems that RRE-90 has a better predictive value for early recurrence risk after cerebral ischemia; however, it requires both accurate subtyping and neuroradiological assessment.
In the Dutch TIA Trial, 997 patients with TIA and 2,130 patients with minor stroke were enrolled for 2 year risk analysis for outcomes of fatal or non-fatal stroke in addition to myocardial infarction, stroke, or vascular death (7). It identified the following independent risk factors for stroke, myocardial infarction or vascular death: age > 65 years; male sex; dysarthria; multiple attacks; diabetes; angina pectoris; intermittent claudication; computed tomographic evidence of any cerebral infarct especially a border zone infarct or white matter hypodensity; and electrocardiographic evidence of anteroseptal infarct, ST depression, left ventricular hypertrophy, or left atrial conduction delay. Prognostic models derived from DTT showed poor calibration and discriminative value (28). In a validation cohort consisting of 592 patients with TIA or minor stroke, Dutch TIA model was found to overestimate the risk and discrimination was poor (c-statistic 0.64, 95% CI, 0.56–0.72) (15).
The Dutch TIA cohort was followed-up for a mean period of 10 years, and the data was reanalyzed in LiLAC (8). Three models were designed to predict the outcome of all-cause mortality and the composite of the event of death from all vascular causes, non-fatal stroke, and non-fatal myocardial infarction. The AUC-ROC for all the three models reached >0.8, but this could not be replicated in a validation cohort (14).
Hankey Score was derived from a cohort of 469 TIA patients without prior stroke and evaluated prospectively over an average period of 4.1 years (range 1–10 years). The major outcome events were a stroke, coronary event, stroke, MI, or vascular death. It used 8 prognostic factors to determine a 5 year risk percentage. These factors included age, gender, affected region (amaurosis fugax, carotid as well as vertebrobasilar TIAs) frequency of TIA, peripheral vascular disease, left ventricular hypertrophy, and residual neurological signs (9).
In two independent cohorts (1,653 TIA patients in UK-TIA aspirin trial and 107 TIA patients in Oxfordshire Community Stroke Project), the reliability of Hankey score was good for lower-risk patients, but it overestimated risk in the higher risk group (29). In a validation cohort of 592 patients with recent TIA or minor stroke (15), Hankey score overestimated the 2 year risk, and its discrimination c-statistics was found to be 0.63 (95% CI, 0.54–0.72). In the 2,381 patients with TIA or non-disabling ischemic stroke from German stroke centers (14), Hankey score had AUC 0.62 (95% CI, 0.57–0.67) for recurrent stroke and AUC of 0.64 (95% CI, 0.60–0.69) for the combined endpoint of stroke or cardiovascular death. Nevertheless, the clinical usefulness of this score is questionable because it involves a sophisticated equation to determine the 1 or 5 year stroke-free survival after TIA.
California risk score was derived from a retrospective cohort of 1,707 patients identified by ED physicians as having TIA to predict the risk of stroke in the 90 days following TIA (10). This simple score was found to be useful in estimating the risk which varied from 0% in patients with score 0 to 34% in patients with score 5. The risk factors included in this score are detailed in Table 4.
ABCD score (Table 4) was derived from a population-based cohort of patients (n = 209, Oxfordshire Community Stroke Project) with a probable or definite TIA to estimate the 7 day risk of stroke and it was validated in a similar cohort of 190 patients (Oxford Vascular Study; OXVASC cohort) (11).
There have been several studies into ABCD score—many of these studies (30–35) have validated the predictive value of ABCD in predicting short-term stroke risk; however, many of these studies have several limitations including retrospective design and small sample size. Several validation studies have concluded that ABCD failed to identify all the high-risk patients (36, 37) or some patients assigned to the low-risk group also had a high risk of cerebral ischemia or radiographic evidence of infarction despite transient symptoms (38).
The investigators who derived California risk score and ABCD score joined to validate the two scores in four validation cohorts (12) for stroke risks at 2, 7, and 90 days (c statistics 0.60–0.81). One of the aims of this study was to derive and validate a unified score from these scores. The resultant score ABCD2 had improved c-statistics in both the derivation cohorts and validated well (c-statistics 0.62–0.83).
In a study to compare ABCD, ABCD2 and California score and to evaluate their relationship to acute ischemic lesions (positive DWI), it was found that elevated California, ABCD or ABCD2 scores were not associated with positive DWI (39). In another study comparing the performance of these three scores, the c-statistics for high-stroke risk was not significantly different between the three scores (40).
ABCD2 is the unified score derived from original California and ABCD score to predict short-term (2, 7, and 90 days) risk of stroke among patients with TIA. The risk factors included in the ABCD2 score are detailed in Table 4.
ABCD2 score has been widely replicated and studied in different cohorts with conflicting results. While many studies (15, 16, 33, 35, 37, 40–53) have validated ABCD2 with c-statistics/area under the ROC curve ranging from 0.57 to 0.88 (not all of these studies provided c-statistics/AUC), there were a few studies which have raised doubts about the predictive value of ABCD2 (54–67). In particular, high rates of recurrence was observed in low-risk groups in studies with large sample size (54, 56, 57). In a study to assess the performance of ABCD2 score in TIA patients subcategorized as tissue-positive or tissue-negative on DWI or CT imaging, it was found that positive tissue patients with low ABCD2 and tissue negative patients with high ABCD2 had similar stroke risks. Another study found that 1 in 5 patients with low ABCD2 score had symptomatic vascular stenotic lesions (68). Use of ABCD2 by non-stroke specialists was not reliable to risk-stratify patients according to some studies (58, 69, 70).
Apart from validating ABCD2, many of these studies were done in an attempt to improve the performance of ABCD2 by adding additional variables such as C-reactive protein (CRP), diffusion-weighted imaging (DWI), CT or carotid imaging (Table 5). For example, one study (72) showed that including CRP measurements in the acute phase to ABCD2 improved the AUC significantly, but the TIA patient sample size was small and lacked validation. Other studies have also shown that adding parameters like DWI findings alone (44), brain infarction on DWI or CT (46), carotid imaging (at least 50% stenosis) and abnormal DWI (48), etiology and DWI (43, 71, 73), CT or transcranial Doppler (74), or carotid artery intima-media thickness (75) to ABCD2 increase the c-statistics/AUC of ABCD2 significantly. But many of these predictive models lack validation in large size cohorts. ABCD2 with DWI seems to be the most predictive out of these models.
ABCD2 + MRI was created by adding diffusion-weighted lesion and vessel occlusion status to the ABCD2 score. In a prospective cohort of 180 patients with TIA or minor stroke (41) to predict recurrent stroke and functional impairment at 90 days, the area under the curve was higher for ABCD2+MRI (0.88 vs. 0.78, P = 0.01).
Clinical- and Imaging-based prediction of stroke risk after TIA (CIP Model) was created to improve the prediction for 7 day stroke risk after TIA by DWI imaging to ABCD2. In a cohort of 601 patients with TIA, adding imaging to ABCD2 increased the area under the curve to 0.81 (95% CI, 0.74–0.88) from 0.66 (95% CI, 0.57–0.76) for ABCD2 alone (42).
ABCD2-I was the result of an international multicenter collaborative study in which unpublished data of 4,574 patients with TIA was used to determine the optimal weighting of brain imaging in the ABCD2 score (46). The resultant score ABCD2-I showed improved predictive power with the weighting of 3 points which was given for infarction on CT or DWI. The area under the curve increased from 0.66 (0.53–0.78) for ABCD2 to 0.78 (0.72–0.85) for ABCD2-I. The shortcomings of this study include the data quality which was obtained from 12 different cohorts over an 11 year period in different countries and using different imaging modalities. Nevertheless, ABCD2-I performed better than ABCD2 in all the individual cohorts except one.
In a cohort (76) of 410 patients with TIA, ABCD2-I showed the improved area under the curve of 0.77 for ABCD2-I from 0.59 for ABCD2 but this study compared the 1 year risk of stroke, unlike the derivation study.
ABCD3 and ABCD3-I scores were developed by the same investigators with aim to improve ABCD2 based on preclinical information (ABCD3) and after initial investigations were completed (ABCD3-I). ABCD3 was derived from ABCD2 by assigning 2 points for dual TIA and ABCD3-I by assigning 2 points for at least 50% stenosis on carotid imaging and another 2 points for abnormal DWI (48). A total of 2,654 TIA patients were included in the derivation cohort. C-statistics for ABCD3 and ABCD3-I at 2, 7, 28, and 90 days were improved compared to ABCD2. In a validation cohort of 1,232 patients, ABCD3 and ABCD3-I predicted early stroke at 7, 28, and 90 days but the performance of ABCD3 was similar to ABCD2 in this validation cohort.
In a cohort of 693 patients with TIA, ABCD3 and ABCD3-I had improved c-statistics of 0.61 and 0.66, respectively, for short-term prediction of stroke compared with ABCD2 (77). In another cohort of 239 patients with TIA, c-statistics of ABCD3-I (0.825, 95% CI, 0.752–0.898) was superior than that of ABCD2 score (0.694, 95% CI, 0.601–0.786; P < 0.001) (78). Other studies (79–81) have provided further validation to ABCD3-I.
ABCDE + was derived from ABCD-score by adding variables etiology and DWI positivity to predict stroke or recurrent TIA within 90 days. In a cohort of 248 patients with TIA (71), ABCDE+ had superior predictive power as it had better area under the curve (0.67 95% CI, 0.55–0.75) compared to ABCD2 (0.48, 95% CI, 0.37–0.58) and ABCD score (0.50, 95% CI, 0.40–0.61; P = 0.07). ABCDE+ was validated in a cohort of 150 patients with TIA with the superior area under the curve for ABCDE+ compared with ABCD2 for predicting stroke (0.64 vs. 0.60) and for predicting death (0.62 vs. 0.56) (73).
When comparing the AUC/C-statistic for the different scores (Table 6), there is considerable overlap with no clear superiority shown by any of the scores. Scores developed to predict long-term risk of stroke have also been studied for short-term prediction of stroke risk and vice-versa as is evident in Table 6. ESRS and SPI-II did not perform well when studied for short-term prediction of stroke recurrence. This is not surprising as they were developed for long-term prediction of stroke recurrence.
Table 6. Range of C-statistic/AUC-ROC for risk of stroke recurrence of different scores in different studies.
Several risk score models have been developed to predict stroke recurrence. However, differences in the methodologies used in the derivation cohorts, population, and outcome make it difficult to compare these risk predictive models. For instance, some of these models have been developed solely from either TIA cohorts (like ABCD2 and Hankey score), or from stroke cohorts (ESRS, RRE-90) while others were developed from a combined cohort of TIA and stroke patients (SPI-I and -II, Dutch TIA score and LiLAC score). Most of the derivation/validation studies used time-based definition of TIA. In a study where the performance of ABCD3-I was assessed in both time and tissue-based definition of TIA, the performance measures were similar (81). A different study indicated that patients with transient symptoms and cerebral infarction are at higher risk of recurrence (26). In addition, different scoring systems have distinct application or utility. For example, RRE-90, ABCD2, and its derivatives were developed to predict short-term recurrence while scores like SPI-I and II, Hankey, ESRS, Dutch TIA, and LiLAC scores were developed to predict the long-term recurrence. The take home message is that discrimination is poor for the currently available risk prediction models. ABCD2 was derived from TIA cohort to predict short-term risk, making the comparison with scores like SPI-II and ESRS challenging. Furthermore, the number of patients in the derivation cohorts of some of these predictive models were limited (e.g., SPI-I was derived from sample size of 142 patients) and models like SPI-II and ESRS were derived from research cohorts and validations were also carried out in research cohorts.
In addition to these differences, some models (like Dutch TIA and LiLAC) attempted to improve the precision of the models by a) increasing the number of variables, or b) including imaging parameters (i.e., RRE-90). However, there is a tradeoff between improvement in precision and the ease of use in clinical settings by non-stroke specialists and general adoption and clinical value; for instance, complex models (such as Hankey score) might not be easily used and integrated into clinical practice. All the above issues highlight why the predictive value of existing models might differ significantly in non-research population.
Ischemic stroke and its recurrence are multifactorial conditions with a combination of genetic, environmental, and vascular factors at play. While a number of risk factors are associated with increased risk of recurrent stroke, there are recurrences which cannot be explained by conventional risk factors (82, 83). In addition, early stroke risk factors might be different from long-term risk factors. Therefore, one prediction model will unlikely be able to be optimized for both short and long-term recurrence for TIA and stroke patients. All the stroke recurrence prediction models reviewed in this work have their own strengths, scopes and limits. It should be noted that recent advances in the management of TIA and minor stroke might also have influenced stroke recurrence rate and the diagnostic performance of current predictive models. Currently, The ABCD2 (especially with DWI) and RRE-90 are the two optimal models for the prediction of short-term risk of recurrent stroke whereas ESRS and SPI-II are the better options for the prediction of long-term risk.
Finally, to address some of the challenges of existing models, it might be valuable to consider addition of insight from biomarkers such as copeptin (84–86) and serum asymmetric dimethylarginine (ADMA) (87), cystatin C (88), Lipoprotein (a) (89), all of which have shown some level of association with recurrent stroke.
Alternatively, application of machine learning methods in combination with rich longitudinal data at various level of resolution could drive the next generation of prediction models that are better tailored to individual patients (90). While machine learning methods are relatively new for healthcare applications, there is already evidence to suggest these methods can outperform currently available tools. These have been used for prediction of outcomes in acute stroke (91) with better area under the curve for deep neural network model. Deep neural networks have been studied for ischemic stroke risk assessment with promising results (92). Artificial neural network have been used to differentiate stroke from stroke mimics (93) and to identify patients at high risk for TIA or minor stroke (94). The use of machine learning in prediction modeling is encouraging but require further studies.
Currently, electronic health record data can capture clinical data from patients and can be mapped with geocoded information from CENSUS and other databases with information about food and environment and, be enriched with genetic data whenever available. Such wealth of information can be used to extract insight and identify potential risk factors and be used in building more stable and generalizable predictive models that can seamlessly work in the background as part of the electronic health record system and provide decision support system on-demand. Advances in machine learning and artificial intelligence will be an important component of the healthcare system, but their integration and adoption still require an ideological shift among healthcare providers.
DC and RZ conducted the literature search, reviewed the included studies, and evaluated the validation of the models. DC wrote the first draft of the manuscript. VA and RZ wrote different sections of the manuscripts. VA, JL, CS, CG, and RZ contributed to significant editing and revisions of the manuscript. All authors read and approved the final version.
This study was partially funded by Geisinger Health Plan Quality awarded to RZ. VA was partly supported by the National Institute of Health (NIH) Grant No. R56HL116832 to Sutter Health and sub-awarded to VA (Sub-PI, Geisinger) as well as funds from the Defense Threat Reduction Agency (DTRA) Grant No. HDTRA1-18-1-0008 to Virginia Tech and sub-awarded to VA (Sub-PI, Geisinger, sub-award No. 450557-19D03). The funders had no role in study design, data collection, and interpretation, or the decision to submit the work for publication.
The authors declare that the research was conducted in the absence of any commercial or financial relationships that could be construed as a potential conflict of interest.
1. Benjamin EJ, Blaha MJ, Chiuve SE, Cushman M, Das SR, Deo R, et al. Heart disease and stroke statistics-2017 update: a report from the American Heart Association. Circulation. (2017) 135:e146–603. doi: 10.1161/CIR.0000000000000491
2. Zou KH, O'Malley AJ, Mauri L. Receiver-operating characteristic analysis for evaluating diagnostic tests and predictive models. Circulation. (2007) 115:654–7. doi: 10.1161/CIRCULATIONAHA.105.594929
3. Kernan WN, Horwitz RI, Brass LM, Viscoli CM, Taylor KJ. A prognostic system for transient ischemia or minor stroke. Ann Intern Med. (1991) 114:552–7. doi: 10.7326/0003-4819-114-7-552
4. Kernan WN, Viscoli CM, Brass LM, Makuch RW, Sarrel PM, Roberts RS, et al. The Stroke Prognosis Instrument II (SPI-II) : a clinical prediction instrument for patients with transient ischemia and nondisabling ischemic stroke. Stroke. (2000) 31:456–62. doi: 10.1161/01.STR.31.2.456
5. Diener HC, Ringleb PA, Savi P. Clopidogrel for the secondary prevention of stroke. Expert Opin Pharmacother. (2005) 6:755–64. doi: 10.1517/14656566.6.5.755
6. Ay H, Gungor L, Arsava EM, Rosand J, Vangel M, Benner T, et al. A score to predict early risk of recurrence after ischemic stroke. Neurology. (2010) 74:128–35. doi: 10.1212/WNL.0b013e3181ca9cff
7. The Dutch TIA Trial Study Group. Predictors of major vascular events in patients with a transient ischemic attack or nondisabling stroke. Stroke. (1993) 24:527–31. doi: 10.1161/01.STR.24.4.527
8. van Wijk I, Kappelle LJ, van Gijn J, Koudstaal PJ, Franke CL, Vermeulen M, et al. Long-term survival and vascular event risk after transient ischaemic attack or minor ischaemic stroke: a cohort study. Lancet. (2005) 365:2098–104. doi: 10.1016/S0140-6736(05)66734-7
9. Hankey GJ, Slattery JM, Warlow CP. Transient ischaemic attacks: which patients are at high (and low) risk of serious vascular events? J Neurol Neurosurg Psychiatry. (1992) 55:640–52. doi: 10.1136/jnnp.55.8.640
10. Johnston SC, Gress DR, Browner WS, Sidney S. Short-term prognosis after emergency department diagnosis of TIA. JAMA. (2000) 284:2901–6. doi: 10.1001/jama.284.22.2901
11. Rothwell PM, Giles MF, Flossmann E, Lovelock CE, Redgrave JN, Warlow CP, et al. A simple score (ABCD) to identify individuals at high early risk of stroke after transient ischaemic attack. Lancet. (2005) 366:29–36. doi: 10.1016/S0140-6736(05)66702-5
12. Johnston SC, Rothwell PM, Nguyen-Huynh MN, Giles MF, Elkins JS, Bernstein AL, et al. Validation and refinement of scores to predict very early stroke risk after transient ischaemic attack. Lancet. (2007) 369:283–92. doi: 10.1016/S0140-6736(07)60150-0
13. Navi BB, Kamel H, Sidney S, Klingman JG, Nguyen-Huynh MN, Johnston SC. Validation of the stroke prognostic instrument-II in a large, modern, community-based cohort of ischemic stroke survivors. Stroke. (2011) 42:3392–6. doi: 10.1161/STROKEAHA.111.620336
14. Weimar C, Benemann J, Michalski D, Muller M, Luckner K, Katsarava Z, et al. Prediction of recurrent stroke and vascular death in patients with transient ischemic attack or nondisabling stroke: a prospective comparison of validated prognostic scores. Stroke. (2010) 41:487–93. doi: 10.1161/STROKEAHA.109.562157
15. Wijnhoud AD, Maasland L, Lingsma HF, Steyerberg EW, Koudstaal PJ, Dippel DW. Prediction of major vascular events in patients with transient ischemic attack or ischemic stroke: a comparison of 7 models. Stroke. (2010) 41:2178–85. doi: 10.1161/STROKEAHA.110.580985
16. Chandratheva A, Geraghty OC, Rothwell PM. Poor performance of current prognostic scores for early risk of recurrence after minor stroke. Stroke. (2011) 42:632–7. doi: 10.1161/STROKEAHA.110.593301
17. CAPRIE Steering Committee. A randomised, blinded, trial of clopidogrel versus aspirin in patients at risk of ischaemic events (CAPRIE). Lancet. (1996) 348:1329–39. doi: 10.1016/S0140-6736(96)09457-3
18. Weimar C, Goertler M, Rother J, Ringelstein EB, Darius H, Nabavi DG, et al. Predictive value of the Essen Stroke Risk Score and Ankle Brachial Index in acute ischaemic stroke patients from 85 German stroke units. J Neurol Neurosurg Psychiatry. (2008) 79:1339–43. doi: 10.1136/jnnp.2008.146092
19. Weimar C, Diener HC, Alberts MJ, Steg PG, Bhatt DL, Wilson PW, et al. The Essen stroke risk score predicts recurrent cardiovascular events: a validation within the REduction of Atherothrombosis for Continued Health (REACH) registry. Stroke. (2009) 40:350–4. doi: 10.1161/STROKEAHA.108.521419
20. Fitzek S, Leistritz L, Witte OW, Heuschmann PU, Fitzek C. The Essen Stroke Risk Score in one-year follow-up acute ischemic stroke patients. Cerebrovasc Dis. (2011) 31:400–7. doi: 10.1159/000323226
21. Meng X, Wang Y, Zhao X, Wang C, Li H, Liu L, et al. Validation of the Essen Stroke Risk Score and the Stroke Prognosis Instrument II in Chinese patients. Stroke. (2011) 42:3619–20. doi: 10.1161/STROKEAHA.111.624148
22. Liu J, Li M, Liu J. Evaluation of the ESRS and SPI-II scales for short-term prognosis of minor stroke and transient ischemic attack. Neurol Res. (2013) 35:568–72. doi: 10.1179/1743132813Y.0000000172
23. Chen P, Liu Y, Wang Y, Wang A, Zheng H, Zhao X, et al. A validation of the Essen Stroke Risk Score in outpatients with ischemic stroke. J Stroke Cerebrovasc Dis. (2016) 25:2189–95. doi: 10.1016/j.jstrokecerebrovasdis.2016.02.001
24. Sumi S, Origasa H, Houkin K, Terayama Y, Uchiyama S, Daida H, et al. A modified Essen stroke risk score for predicting recurrent cardiovascular events: development and validation. Int J Stroke. (2013) 8:251–7. doi: 10.1111/j.1747-4949.2012.00841.x
25. Arsava EM, Kim GM, Oliveira-Filho J, Gungor L, Noh HJ, Lordelo Mde J, et al. Prediction of early recurrence after acute ischemic stroke. JAMA Neurol. (2016) 73:396–401. doi: 10.1001/jamaneurol.2015.4949
26. Arsava EM, Furie KL, Schwamm LH, Sorensen AG, Ay H. Prediction of early stroke risk in transient symptoms with infarction: relevance to the new tissue-based definition. Stroke. (2011) 42:2186–90. doi: 10.1161/STROKEAHA.110.604280
27. Song B, Pei L, Fang H, Zhao L, Gao Y, Wang Y, et al. Validation of the RRE-90 scale to predict stroke risk after transient symptoms with infarction: a prospective cohort study. PLoS ONE. (2015) 10:e0137425. doi: 10.1371/journal.pone.0137425
28. Dippel DW, Koudstaal PJ. We need stronger predictors of major vascular events in patients with a recent transient ischemic attack or nondisabling stroke. Dutch TIA Trial Study Group. Stroke. (1997) 28:774–6. doi: 10.1161/01.STR.28.4.774
29. Hankey GJ, Slattery JM, Warlow CP. Can the long term outcome of individual patients with transient ischaemic attacks be predicted accurately? J Neurol Neurosurg Psychiatry. (1993) 56:752–9. doi: 10.1136/jnnp.56.7.752
30. Tsivgoulis G, Spengos K, Manta P, Karandreas N, Zambelis T, Zakopoulos N, et al. Validation of the ABCD score in identifying individuals at high early risk of stroke after a transient ischemic attack: a hospital-based case series study. Stroke. (2006) 37:2892–7. doi: 10.1161/01.STR.0000249007.12256.4a
31. Bray JE, Coughlan K, Bladin C. Can the ABCD Score be dichotomised to identify high-risk patients with transient ischaemic attack in the emergency department? Emerg Med J. (2007) 24:92–5. doi: 10.1136/emj.2006.041624
32. Calvet D, Lamy C, Touze E, Oppenheim C, Meder JF, Mas JL. Management and outcome of patients with transient ischemic attack admitted to a stroke unit. Cerebrovasc Dis. (2007) 24:80–5. doi: 10.1159/000103120
33. Koton S, Rothwell PM. Performance of the ABCD and ABCD2 scores in TIA patients with carotid stenosis and atrial fibrillation. Cerebrovasc Dis. (2007) 24:231–5. doi: 10.1159/000104483
34. Sciolla R, Melis F, Group S. Rapid identification of high-risk transient ischemic attacks: prospective validation of the ABCD score. Stroke. (2008) 39:297–302. doi: 10.1161/STROKEAHA.107.496612
35. Harrison JK, Sloan B, Dawson J, Lees KR, Morrison DS. The ABCD and ABCD2 as predictors of stroke in transient ischemic attack clinic outpatients: a retrospective cohort study over 14 years. QJM. (2010) 103:679–85. doi: 10.1093/qjmed/hcq108
36. Purroy Garcia F, Molina Cateriano CA, Montaner Villalonga J, Delgado Martinez P, Santmarina Perez E, Toledo M, et al. [Lack of usefulness of ABCD score in the early risk of recurrent stroke in transient ischemic attack patients]. Med Clin. (2007) 128:201–3. doi: 10.1157/13098716
37. Fothergill A, Christianson TJ, Brown RD Jr, Rabinstein AA. Validation and refinement of the ABCD2 score: a population-based analysis. Stroke. (2009) 40:2669–73. doi: 10.1161/STROKEAHA.109.553446
38. Cucchiara BL, Messe SR, Taylor RA, Pacelli J, Maus D, Shah Q, et al. Is the ABCD score useful for risk stratification of patients with acute transient ischemic attack? Stroke. (2006) 37:1710–4. doi: 10.1161/01.STR.0000227195.46336.93
39. Purroy F, Begue R, Quilez A, Pinol-Ripoll G, Sanahuja J, Brieva L, et al. The California, ABCD, and unified ABCD2 risk scores and the presence of acute ischemic lesions on diffusion-weighted imaging in TIA patients. Stroke. (2009) 40:2229–32. doi: 10.1161/STROKEAHA.108.537969
40. Nguyen H, Kerr D, Kelly AM. Comparison of prognostic performance of scores to predict risk of stroke in ED patients with transient ischaemic attack. Eur J Emerg Med. (2010) 17:346–8. doi: 10.1097/MEJ.0b013e328337b1c6
41. Coutts SB, Eliasziw M, Hill MD, Scott JN, Subramaniam S, Buchan AM, et al. An improved scoring system for identifying patients at high early risk of stroke and functional impairment after an acute transient ischemic attack or minor stroke. Int J Stroke. (2008) 3:3–10. doi: 10.1111/j.1747-4949.2008.00182.x
42. Ay H, Arsava EM, Johnston SC, Vangel M, Schwamm LH, Furie KL, et al. Clinical- and imaging-based prediction of stroke risk after transient ischemic attack: the CIP model. Stroke. (2009) 40:181–6. doi: 10.1161/STROKEAHA.108.521476
43. Calvet D, Touze E, Oppenheim C, Turc G, Meder JF, Mas JL. DWI lesions and TIA etiology improve the prediction of stroke after TIA. Stroke. (2009) 40:187–92. doi: 10.1161/STROKEAHA.108.515817
44. Cucchiara BL, Messe SR, Sansing L, MacKenzie L, Taylor RA, Pacelli J, et al. D-dimer, magnetic resonance imaging diffusion-weighted imaging, and ABCD2 score for transient ischemic attack risk stratification. J Stroke Cerebrovasc Dis. (2009) 18:367–73. doi: 10.1016/j.jstrokecerebrovasdis.2009.01.006
45. Asimos AW, Johnson AM, Rosamond WD, Price MF, Rose KM, Catellier D, et al. A multicenter evaluation of the ABCD2 score's accuracy for predicting early ischemic stroke in admitted patients with transient ischemic attack. Ann Emerg Med. (2010) 55:201–10 e5. doi: 10.1016/j.annemergmed.2009.05.002
46. Giles MF, Albers GW, Amarenco P, Arsava MM, Asimos A, Ay H, et al. Addition of brain infarction to the ABCD2 Score (ABCD2I): a collaborative analysis of unpublished data on 4574 patients. Stroke. (2010) 41:1907–13. doi: 10.1161/STROKEAHA.110.578971
47. Holzer K, Feurer R, Sadikovic S, Esposito L, Bockelbrink A, Sander D, et al. Prognostic value of the ABCD2 score beyond short-term follow-up after transient ischemic attack (TIA)–a cohort study. BMC Neurol. (2010) 10:50. doi: 10.1186/1471-2377-10-50
48. Merwick A, Albers GW, Amarenco P, Arsava EM, Ay H, Calvet D, et al. Addition of brain and carotid imaging to the ABCD(2) score to identify patients at early risk of stroke after transient ischaemic attack: a multicentre observational study. Lancet Neurol. (2010) 9:1060–9. doi: 10.1016/S1474-4422(10)70240-4
49. Ong ME, Chan YH, Lin WP, Chung WL. Validating the ABCD(2) score for predicting stroke risk after transient ischemic attack in the ED. Am J Emerg Med. (2010) 28:44–8. doi: 10.1016/j.ajem.2008.09.027
50. Tsivgoulis G, Stamboulis E, Sharma VK, Heliopoulos I, Voumvourakis K, Teoh HL, et al. Multicenter external validation of the ABCD2 score in triaging TIA patients. Neurology. (2010) 74:1351–7. doi: 10.1212/WNL.0b013e3181dad63e
51. Yang J, Fu JH, Chen XY, Chen YK, Leung TW, Mok V, et al. Validation of the ABCD2 score to identify the patients with high risk of late stroke after a transient ischemic attack or minor ischemic stroke. Stroke. (2010) 41:1298–300. doi: 10.1161/STROKEAHA.110.578757
52. Cancelli I, Janes F, Gigli GL, Perelli A, Zanchettin B, Canal G, et al. Incidence of transient ischemic attack and early stroke risk: validation of the ABCD2 score in an Italian population-based study. Stroke. (2011) 42:2751–7. doi: 10.1161/STROKEAHA.110.612705
53. Galvin R, Atanassova PA, Motterlini N, Fahey T, Dimitrov BD. Long-term risk of stroke after transient ischaemic attack: a hospital-based validation of the ABCD(2) rule. BMC Res Notes. (2014) 7:281. doi: 10.1186/1756-0500-7-281
54. Weimar C, Benemann J, Huber R, Mieck T, Kaendler S, Grieshammer S, et al. Long-term mortality and risk of stroke after transient ischemic attack: a hospital-based cohort study. J Neurol. (2009) 256:639–44. doi: 10.1007/s00415-009-0150-9
55. Chandratheva A, Geraghty OC, Luengo-Fernandez R, Rothwell PM, Oxford Vascular Study. ABCD2 score predicts severity rather than risk of early recurrent events after transient ischemic attack. Stroke. (2010) 41:851–6. doi: 10.1161/STROKEAHA.109.570010
56. Sheehan OC, Kyne L, Kelly LA, Hannon N, Marnane M, Merwick A, et al. Population-based study of ABCD2 score, carotid stenosis, and atrial fibrillation for early stroke prediction after transient ischemic attack: the North Dublin TIA study. Stroke. (2010) 41:844–50. doi: 10.1161/STROKEAHA.109.571844
57. Stead LG, Suravaram S, Bellolio MF, Enduri S, Rabinstein A, Gilmore RM, et al. An assessment of the incremental value of the ABCD2 score in the emergency department evaluation of transient ischemic attack. Ann Emerg Med. (2011) 57:46–51. doi: 10.1016/j.annemergmed.2010.07.001
58. Dutta D, Bailey SJ. Validation of ABCD2 scores ascertained by referring clinicians: a retrospective transient ischaemic attack clinic cohort study. Emerg Med J. (2016) 33:543–7. doi: 10.1136/emermed-2015-205519
59. Amarenco P, Labreuche J, Lavallee PC. Patients with transient ischemic attack with ABCD2 <4 can have similar 90-day stroke risk as patients with transient ischemic attack with ABCD2 >/ = 4. Stroke. (2012) 43:863–5. doi: 10.1161/STROKEAHA.111.636506
60. Perry JJ, Sharma M, Sivilotti ML, Sutherland J, Symington C, Worster A, et al. Prospective validation of the ABCD2 score for patients in the emergency department with transient ischemic attack. CMAJ. (2011) 183:1137–45. doi: 10.1503/cmaj.101668
61. Ghandehari K, Ahmadi F, Ebrahimzadeh S, Shariatinezhad K, Ghandehari K. Assessment of ABCD(2) scale in patients with transient ischaemic attack or stroke. Neurol Neurochir Pol. (2012) 46:421–7. doi: 10.5114/ninp.2012.31351
62. Ghia D, Thomas P, Cordato D, Epstein D, Beran RG, Cappelen-Smith C, et al. Low positive predictive value of the ABCD2 score in emergency department transient ischaemic attack diagnoses: the South Western Sydney transient ischaemic attack study. Intern Med J. (2012) 42:913–8. doi: 10.1111/j.1445-5994.2011.02564.x
63. Walker J, Isherwood J, Eveson D, Naylor AR. Triaging TIA/minor stroke patients using the ABCD2 score does not predict those with significant carotid disease. Eur J Vasc Endovasc Surg. (2012) 43:495–8. doi: 10.1016/j.ejvs.2012.02.002
64. Chardoli M, Khajavi A, Nouri M, Rahimi-Movaghar V. Value of ABCD2 in predicting early ischemic stroke in patients diagnosed with transient ischemic attack. Acta Med Iran. (2013) 51:611–4. Available online at: http://acta.tums.ac.ir/index.php/acta/article/view/4366
65. Ildstad F, Ellekjaer H, Wethal T, Lydersen S, Sund JK, Fjaertoft H, et al. Stroke risk after transient ischemic attack in a Norwegian prospective cohort. BMC Neurol. (2019) 19:2. doi: 10.1186/s12883-018-1225-y
66. Sadighi A, Stanciu A, Banciu M, Abedi V, Andary NE, Holland N, et al. Rate and associated factors of transient ischemic attack misdiagnosis. eNeurologicalSci. (2019) 15:100193. doi: 10.1016/j.ensci.2019.100193
67. Sadighi A, Abedi V, Stanciu A, El Andary N, Banciu M, Holland N, et al. Six-month outcome of transient ischemic attack and its mimics. Front Neurol. (2019) 10:294. doi: 10.3389/fneur.2019.00294
68. Bhatt A, Farooq MU, Safdar A, Hejabian S, Razak A, Hussain SI, et al. ABCD2 score and large-artery atherosclerosis. Neurohospitalist. (2012) 2:12–7. doi: 10.1177/1941874411418239
69. Bradley D, Cronin S, Kinsella JA, Tobin WO, Mahon C, O'Brien M, et al. Frequent inaccuracies in ABCD2 scoring in non-stroke specialists' referrals to a daily Rapid Access Stroke Prevention service. J Neurol Sci. (2013) 332:30–4. doi: 10.1016/j.jns.2013.05.030
70. Wardlaw J, Brazzelli M, Miranda H, Chappell F, McNamee P, Scotland G, et al. An assessment of the cost-effectiveness of magnetic resonance, including diffusion-weighted imaging, in patients with transient ischaemic attack and minor stroke: a systematic review, meta-analysis and economic evaluation. Health Technol Assess. (2014) 18:1–368, v–vi. doi: 10.3310/hta18270
71. Engelter ST, Amort M, Jax F, Weisskopf F, Katan M, Burow A, et al. Optimizing the risk estimation after a transient ischaemic attack - the ABCDE plus sign in circle score. Eur J Neurol. (2012) 19:55–61. doi: 10.1111/j.1468-1331.2011.03428.x
72. Corso G, Bottacchi E, Brusa A, Lia C, Cordera S, Giardini G, et al. Blood C-reactive protein concentration with ABCD(2) is a better prognostic tool than ABCD(2) alone. Cerebrovasc Dis. (2011) 32:97–105. doi: 10.1159/000328230
73. Arhami Dolatabadi A, Meisami A, Hatamabadi H, Mansori B, Shahrami A, Amini A, et al. Improving the prediction of stroke or death after transient ischemic attack (TIA) by adding diffusion-weighted imaging lesions and TIA etiology to the ABCD2 score. J Stroke Cerebrovasc Dis. (2013) 22:e25–30. doi: 10.1016/j.jstrokecerebrovasdis.2012.03.007
74. Fu J, Tang J, Yang J, Chen X, Chen Y, Leung TW, et al. Adding computed tomography and transcranial Doppler findings to the ABCD2 score to predict long-term risk of stroke after transient ischaemic attack or minor stroke. Eur J Neurol. (2015) 22:520–6. doi: 10.1111/ene.12606
75. Khorvash F, Hemasian H, Shahabi S, Shahzamani A, Sheikhbahaei E, Chitsaz A. Predicting long-term cardiovascular events after transient ischemic attacks: carotid artery intima-media thickness or ABCD2 score or both? Int J Prev Med. (2018) 9:102. doi: 10.4103/ijpvm.IJPVM_415_17
76. Meng X, Wang Y, Liu L, Pu Y, Zhao X, Wang C, et al. Validation of the ABCD(2)-I score to predict stroke risk after transient ischemic attack. Neurol Res. (2011) 33:482–6. doi: 10.1179/016164111X13007856084043
77. Kiyohara T, Kamouchi M, Kumai Y, Ninomiya T, Hata J, Yoshimura S, et al. ABCD3 and ABCD3-I scores are superior to ABCD2 score in the prediction of short- and long-term risks of stroke after transient ischemic attack. Stroke. (2014) 45:418–25. doi: 10.1161/STROKEAHA.113.003077
78. Song B, Fang H, Zhao L, Gao Y, Tan S, Lu J, et al. Validation of the ABCD3-I score to predict stroke risk after transient ischemic attack. Stroke. (2013) 44:1244–8. doi: 10.1161/STROKEAHA.113.000969
79. Chatzikonstantinou A, Wolf ME, Schaefer A, Hennerici MG. Risk prediction of subsequent early stroke in patients with transient ischemic attacks. Cerebrovasc Dis. (2013) 36:106–9. doi: 10.1159/000352060
80. Knoflach M, Lang W, Seyfang L, Fertl E, Oberndorfer S, Daniel G, et al. Predictive value of ABCD2 and ABCD3-I scores in TIA and minor stroke in the stroke unit setting. Neurology. (2016) 87:861–9. doi: 10.1212/WNL.0000000000003033
81. Mayer L, Ferrari J, Krebs S, Boehme C, Toell T, Matosevic B, et al. ABCD3-I score and the risk of early or 3-month stroke recurrence in tissue- and time-based definitions of TIA and minor stroke. J Neurol. (2018) 265:530–4. doi: 10.1007/s00415-017-8720-8
82. Yamamoto H, Bogousslavsky J. Mechanisms of second and further strokes. J Neurol Neurosurg Psychiatry. (1998) 64:771–6. doi: 10.1136/jnnp.64.6.771
83. Hillen T, Coshall C, Tilling K, Rudd AG, McGovern R, Wolfe CD, et al. Cause of stroke recurrence is multifactorial: patterns, risk factors, and outcomes of stroke recurrence in the South London Stroke Register. Stroke. (2003) 34:1457–63. doi: 10.1161/01.STR.0000072985.24967.7F
84. De Marchis GM, Weck A, Audebert H, Benik S, Foerch C, Buhl D, et al. Copeptin for the prediction of recurrent cerebrovascular events after transient ischemic attack: results from the CoRisk study. Stroke. (2014) 45:2918–23. doi: 10.1161/STROKEAHA.114.005584
85. Greisenegger S, Segal HC, Burgess AI, Poole DL, Mehta Z, Rothwell PM. Copeptin and long-term risk of recurrent vascular events after transient ischemic attack and ischemic stroke: population-based study. Stroke. (2015) 46:3117–23. doi: 10.1161/STROKEAHA.115.011021
86. Xu Q, Tian Y, Peng H, Li H. Copeptin as a biomarker for prediction of prognosis of acute ischemic stroke and transient ischemic attack: a meta-analysis. Hypertens Res. (2017) 40:465–71. doi: 10.1038/hr.2016.165
87. Zhang Y, Fan D, Zhang N. [The relationship between serum asymmetric dimethylarginine and ABCD2 score in transient ischemic attack patients]. Zhonghua Nei Ke Za Zhi. (2014) 53:876–9.
88. Zeng Q, Lin K, Yao M, Wei L. Significant correlation between cystatin C, cerebral infarction, and potential biomarker for increased risk of stroke. Curr Neurovasc Res. (2015) 12:40–6. doi: 10.2174/1567202612666150102150941
89. Hong XW, Wu DM, Lu J, Zheng YL, Tu WJ, Yan J. Lipoprotein (a) as a predictor of early stroke recurrence in acute ischemic stroke. Mol Neurobiol. (2018) 55:718–26. doi: 10.1007/s12035-016-0346-9
90. Noorbakhsh-Sabet N, Zand R, Zhang Y, Abedi V. Artificial intelligence transforms the future of health care. Am J Med. (2019) 132:795–801. doi: 10.1016/j.amjmed.2019.01.017
91. Heo J, Yoon JG, Park H, Kim YD, Nam HS, Heo JH. Machine learning-based model for prediction of outcomes in acute stroke. Stroke. (2019) 50:1263–5. doi: 10.1161/STROKEAHA.118.024293
92. Hung CY, Lin CH, Lan TH, Peng GS, Lee CC. Development of an intelligent decision support system for ischemic stroke risk assessment in a population-based electronic health record database. PLoS ONE. (2019) 14:e0213007. doi: 10.1371/journal.pone.0213007
93. Abedi V, Goyal N, Tsivgoulis G, Hosseinichimeh N, Hontecillas R, Bassaganya-Riera J, et al. Novel screening tool for stroke using artificial neural network. Stroke. (2017) 48:1678–81. doi: 10.1161/STROKEAHA.117.017033
Keywords: clinical risk scores, recurrent stroke risk, predictive modeling, ischemic stroke, predicting recurrence
Citation: Chaudhary D, Abedi V, Li J, Schirmer CM, Griessenauer CJ and Zand R (2019) Clinical Risk Score for Predicting Recurrence Following a Cerebral Ischemic Event. Front. Neurol. 10:1106. doi: 10.3389/fneur.2019.01106
Received: 07 August 2019; Accepted: 02 October 2019;
Published: 12 November 2019.
Edited by:
Magdy Selim, Beth Israel Deaconess Medical Center, Harvard Medical School, United StatesReviewed by:
Andrea Morotti, Neurological Institute Foundation Casimiro Mondino (IRCCS), ItalyCopyright © 2019 Chaudhary, Abedi, Li, Schirmer, Griessenauer and Zand. This is an open-access article distributed under the terms of the Creative Commons Attribution License (CC BY). The use, distribution or reproduction in other forums is permitted, provided the original author(s) and the copyright owner(s) are credited and that the original publication in this journal is cited, in accordance with accepted academic practice. No use, distribution or reproduction is permitted which does not comply with these terms.
*Correspondence: Ramin Zand, cnphbmRAZ2Vpc2luZ2VyLmVkdQ==
Disclaimer: All claims expressed in this article are solely those of the authors and do not necessarily represent those of their affiliated organizations, or those of the publisher, the editors and the reviewers. Any product that may be evaluated in this article or claim that may be made by its manufacturer is not guaranteed or endorsed by the publisher.
Research integrity at Frontiers
Learn more about the work of our research integrity team to safeguard the quality of each article we publish.