- 1School of Acupuncture and Tuina, Chengdu University of Traditional Chinese Medicine, Chengdu, China
- 2Department of Rehabilitation, Shuangliu Maternal and Child Health Care Hospital, Chengdu, China
- 3Key Laboratory for NeuroInformation of Ministry of Education, School of Life Science and Technology, University of Electronic Science and Technology of China, Chengdu, China
- 4School of Medicine, Chengdu University, Chengdu, China
- 5School of Rehabilitation and Health Preservation, Chengdu University of Traditional Chinese Medicine, Chengdu, China
- 6Department of Rehabilitation, Zigong Fifth People's Hospital, Zigong, China
Internet addiction (IA) has become a global mental and social problem, which may lead to a series of psychiatric symptoms including uncontrolled use of internet, and lack of concentration. However, the exact pathophysiology of IA remains unclear. Most of functional connectivity studies were based on pre-selected regions of interest (ROI), which could not provide a comprehensive picture of the communication abnormalities in IA, and might lead to limited or bias observations. Using local functional connectivity density (lFCD), this study aimed to explore the whole-brain abnormalities of functional connectivity in IA. We evaluated the whole-brain lFCD resulting from resting-state fMRI data in 28 IA individuals and 30 demographically matched healthy control subjects (HCs). The correlations between clinical characteristics and aberrant lFCD were also assessed. Compared with HCs, subjects with IA exhibited heightened lFCD values in the right dorsolateral prefrontal cortex (DLPFC), left parahippocampal gyrus (PHG), and cerebellum, and the bilateral middle cingulate cortex (MCC) and superior temporal pole (STP), as well as decreased lFCD values in the right inferior parietal lobe (IPL), and bilateral calcarine and lingual gyrus. Voxel-based correlation analysis revealed the significant correlations between the Young's Internet Addiction Test (IAT) score and altered lFCD values in the left PHG and bilateral STP. These findings revealed the hyper-connectivity in cognitive control network and default mode network as well as the hypo-connectivity in visual attention network, verifying the common mechanism in IA and substance addiction, and the underlying association between IA, and attention deficit/hyperactivity disorder in terms of neurobiology.
Introduction
Internet addiction (IA), as a behavioral addiction, was conceptualized as the poorly controlled use of internet with impairment of interpersonal relationships, and psychological and social functioning (1). With the popularity of the internet and the improvement of information technology in the last 2 decades, IA has become a serious public health problem due to its high prevalence around the world (2, 3). In Hong Kong, the prevalence rate of IA has dramatic increased from 3.0 to 26.8% in the last 10 years, with the proportion of teenagers who spend more than 20 h online per week increasing from 25.4 to 42.0% (4). The potential risk of IA has attracted growing concerns from worldwide researchers, and mounting studies, and papers have been published. Besides uncontrolled use of internet, multiple comorbid psychiatric symptoms of IA have been revealed by clinical studies, including anxiety, depression, and lack of concentration (5, 6). Epidemiological research further demonstrated that attention deficit/hyperactivity disorder (ADHD), unsociable personality, and bad relationship with parents were high risk factors of IA (6–8). Additionally, ADHD was thought to be the main complication of IA (9). However, the exact pathophysiology of IA has not been fully understood yet, and the underlying mechanism of the coexistence of IA, and comorbid symptoms remains unclear.
Although the pathogenesis has not been well-elucidated, resting-state fMRI provides a useful platform for understanding the neuropathological changes in IA. Resting state functional connectivity (FC), which reflects the temporal coherence of inter-regional spontaneous blood oxygen level dependent (BOLD) fluctuations in cerebral activity (10), was extensively used to investigate the functional interactions between brain areas in psychiatric research, including IA. In previous fMRI studies, IA was associated with the altered FC of cognitive control network (CCN) (11), which was considered as the main neurobiological characteristic of substance addiction. For instance, enhanced FC between the dorsolateral prefrontal cortex (DLPFC), and the temporoparietal junction (TPJ) was observed in IA individuals (12), which was associated with many other cognitive disorders (13, 14). Furthermore, altered FC within the CCN was also demonstrated. Compared with healthy subjects, adolescents with IA exhibited heightened FC between the DLPFC, and anterior cingulate cortex (ACC), correlating with the symptom severity of IA (15). According to Volkow's addiction model, abnormal CCN was proposed as the key determinant in development, and maintenance of substance addiction (16). The altered FC of CCN found in IA individuals, giving a pathophysiological explanation for the uncontrolled use of internet, suggested that IA might share similar neurobiological mechanism with substance addiction (17). However, most previous FC studies in IA were based on a priori selection of region of interest (ROI). This seed-based analysis cannot provide a whole-brain picture of FC alterations in IA, and may lead to limited or biased observations due to the restriction on ROI.
Local functional connectivity density (lFCD) has been developed to analyze the whole-brain FC abnormalities by computing the temporal correlations of every pair of neighboring voxels in the entire brain (18, 19). Voxels with a higher number of functional connections have greater lFCD values, and are considered to be important functional hubs in the information processing. Unlike seed-based FC, lFCD provides an unbiased approach to the study of whole-brain functional connectivity (18). As a result, extra abnormalities have been revealed by lFCD in many disorders, which would not have been detected using traditional seed-based FC analysis methods (20–22). Investigating the alterations of lFCD in IA may provide the full picture of the communication abnormalities of whole-brain functional network, and offer a more reliable, and comprehensive result beyond the FC. This may help to improve our understanding of the neuropathological mechanism of IA and the common neurobiological dysfunction underlying the coexistence of IA, and comorbid symptoms.
In the current study, we aim to investigate the lFCD dysfunction in IA individuals and to detect the potential relationships between the altered lFCD values and the related symptom scores. Local FCD from 28 IA individuals were compared to those of 30 demographically matched healthy controls (HCs). Furthermore, a voxel-based correlation analysis was performed to examine the possible associations between the clinical variables and the lFCD values of the regions with significant differences.
Materials and Methods
Participants
A total of 58 subjects (28 IAs and 30 HCs) were recruited from university students. All participants were right-handed and native Chinese speakers. The inclusion criteria for IA group were as follows: (1) aged between 18 and 30 years; (2) in line with the diagnostic criteria of IA via Young's Diagnostic Questionnaire (YDQ) (1); (3) a score of 60 or higher on Young's Internet Addiction Test (IAT); (4) not under any form of therapeutic interventions; and (5) without any other organic or mental diseases. Thirty HCs, well-matched with IA group in age, gender, and education, were recruited with the following inclusion criteria: (1) aged between 18 and 30 years; (2) inconsistent with the IA diagnostic criteria by Young (1); (3) IAT score <50; and (4) without any other organic, or mental diseases. Participants with a history of substance addiction were excluded in our study. Additionally, pregnant, or lactating women were also excluded.
The protocol of this study was evaluated and approved by the Sichuan Regional Ethics Review Committee on traditional Chinese medicine (ethical approval number 2016KL-005), in accordance with the Declaration of Helsinki (2000). All participants signed informed consent forms before inclusion and were compensated for their participation.
Questionnaire
Besides IAT, the Yale-Brown Obsessive Compulsive Scale (YBOCS) (23) and the Barratt Impulsiveness Scale-11 (BIS-11) (24) were finished by all subjects, and were used to assess obsessive-compulsive and impulsive behaviors, respectively. All scales were translated into Chinese. We also collected information on sex, age, educational level, and years of internet use via self-designed questionnaire.
Data Acquisition
All fMRI data were collected on a 3.0T MRI scanner (GE Discovery MR 750, USA) with a standard 8-channel head coil. During scanning, participants were instructed to keep their eyes closed and head still, and remain awake without thinking anything in particular. Ear plugs and foam pads were used to restrict noise and displacement of head, respectively. Functional images were acquired using a standard Echo Planar Imaging sequence with the parameters as follows: repetition time = 2000 ms, echo time = 30 ms, flip angle = 90°, field of view = 24 × 24 cm2, image matrix = 64 × 64, no gap, and voxel size = 3.75 × 3.75 × 4.4 mm3. Two hundred and 55 volumes of image were acquired, and each volume included 35 slices.
Date Preprocessing
Functional images were preprocessed using the neuroscience information toolbox (NIT, http://www.neuro.uestc.edu.cn/NIT.html), which is based on Statistical Parametric Mapping (SPM8, http://www.fil.ion.ucl.ac.uk/spm/). Prior to preprocessing, the initial 5 volumes of each subject were removed to minimize the interference of instability in the initial signals. Subsequently, slice timing was conducted to correct the time delay between slices. Spatial realignment was performed to correct the head motion. Participants with more than 2 mm displacement or more than 2° rotation were excluded. The fMRI images were then normalized to standard Montreal Neurological Institute (MNI) template with a resolution of 3 × 3 × 3 mm3. After that, we regressed out 24 head motion parameters and signals from cerebral spinal fluid, and white matter. Finally, band-pass filtering (0.01–0.08 Hz) was performed to reduce the interference of low-frequency drift and high-frequency noise (25, 26).
Local FCD Calculation
Local FCD, which is defined as a sum of the number of efficient neighboring functional connections of a given voxel, reflects the voxel-wise whole-brain functional connectivity strength. In our study, lFCD calculation was conducted using the NIT toolbox, based on the proposal by Tomasi and Volkow (18, 19). First, Pearson correlation coefficients between the time course of a given voxel and those of its neighboring voxels were computed. According to the seminal paper (18), functional connections between two voxels with a correlation coefficient R > 0.6 were considered significant, as thresholds of R < 0.4 might lead to too many false positives, while thresholds of R > 0.7 might reduce the sensitivity of lFCD maps (27). All voxels were calculated to generate the whole brain lFCD map. Then we smoothed the lFCD maps with a Gaussian kernel of 6 mm. A more detailed procedure of lFCD calculation can be found in our previous study (28).
Statistical Analysis
Using SPSS 18.0, we compared demographic data and clinical variables between IA individuals and HCs. Two-sample t-test was performed to compare the intergroup differences in continuous variables (e.g., age, years of internet use, scores on clinical scales) while chi-square test was used for categorical variable (e.g., gender). A P < 0.05 was considered to be statistically significant. Then a two-sample t-test was performed to compare lFCD values between IA individuals and HCs, and a P < 0.01 with cluster size > 23 adjacent voxels was deemed significant according to the random field theory (29), which set the threshold for the spatial extent of clusters is extent−threshold = 638 mm3. In addition, a voxel-based correlation analysis was conducted between the lFCD values and the clinical scores and then the potential association between aberrant lFCD and severity of symptoms was obtained by intersection of lFCD and correlation results. Statistical significance was set at P < 0.05 (FDR corrected).
Results
Demographic Characteristics and Clinical Measures
Subject demographics and clinical scores of 28 IA individuals and 30 HCs are shown in Table 1. No significant intergroup difference was observed in age, sex, or years of education between two groups (P > 0.05). Consistent with the inclusion, IA individuals exhibited higher scores of IAT, YBOCS, BIS-11 significantly (P < 0.001), although the years of internet use showed no difference (P > 0.05).
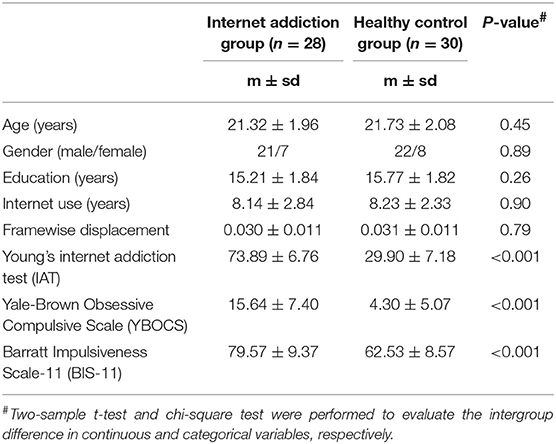
Table 1. Demographics and clinical characteristics of the individuals with internet addiction and the healthy controls.
Local FCD Results
As shown in Table 2 and Figure 1, in comparison to HCs IA individuals exhibited lower lFCD values in the visual attention regions (P < 0.01, with the cluster-level corrected), including the right inferior parietal lobe (IPL, comprising supramarginal gyrus and inferior parietal cortex), and bilateral calcarine and lingual gyrus. Higher lFCD values (P < 0.01, with the cluster-level corrected) were observed in brain regions belonging to the DMN, including the left parahippocampal gyrus (PHG), and bilateral superior temporal pole (STP). In addition, enhanced lFCD values in the CCN were also demonstrated (P < 0.01, with the cluster-level corrected), including the right DLPFC (comprising superior frontal gyrus), left cerebellum, and bilateral middle cingulum cortex (MCC). We further evaluated the influence of framewise displacement (FD), which might partially index physiological noise (30, 31). No significant intergroup difference in FD and no correlation between FD and lFCD values were found (P > 0.05).
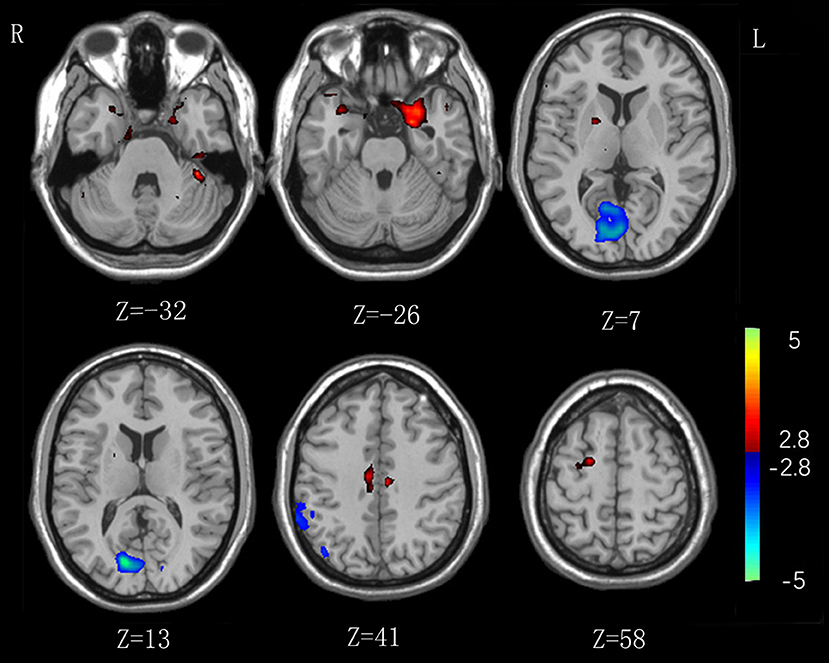
Figure 1. Significant intergroup differences in lFCD between the internet addiction individuals and healthy control subjects. Compared with healthy control subjects, individuals with IA exhibited increased lFCD values in the right superior frontal gyrus, left cerebellum, and parahippocampal gyrus, and bilateral middle cingulum cortex, and superior temporal pole. Decreased lFCD values were exhibited in the right inferior parietal lobe and bilateral calcarine and lingual gyrus.
Relationship Between lFCD Values and Clinical Scores
Voxel-based correlation analysis revealed positive correlations between the IAT score and the heightened lFCD values of left PHG (r = 0.522, P = 0.004) and bilateral STP (Left: r = 0.500, P = 0.007; Right: r = 0.634, P < 0.001) after FDR correction (Figure 2). However, there was no remarkable association between the neural activity changes and BIS-11 or YBOCS after multiple comparison correction, although the IA subjects exhibited higher BIS-11 and YBOCS scores.
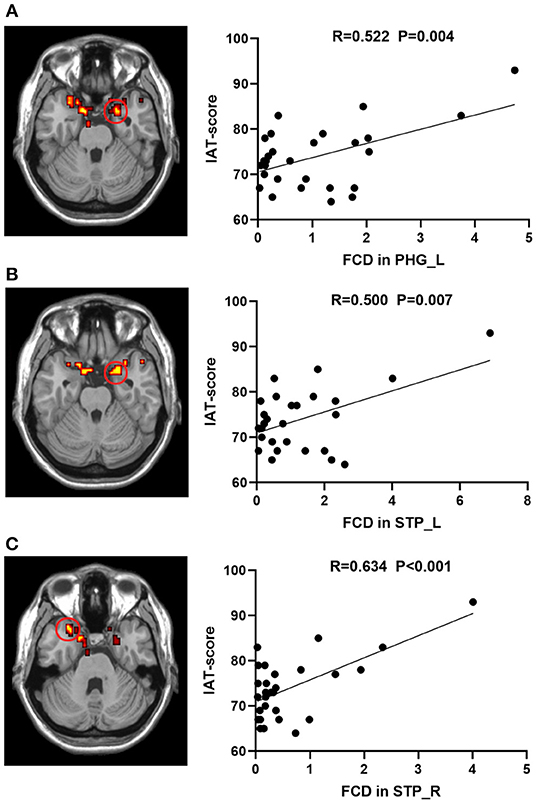
Figure 2. Correlations between lFCD values and IAT score in IA group. The IAT score was positively associated with lFCD values in the (A) STP_L, (B) STP_R, and (C) PHG_L. lFCD, local functional connectivity density; IAT, Young's internet addiction test; L, left; R, right; PHG, parahippocampal gyrus; STP, superior temporal pole.
Discussion
Using a voxel-wise data-driven method, the current study investigated the changes of whole-brain functional connectivity in IA individuals. Similar to substance addiction, IA was manifested with enhanced lFCD in the CCN. In addition, increased lFCD in the DMN and decreased lFCD in the visual attention network were both related to impaired attention, and were also observed in our study. Furthermore, we demonstrated the association between the severity of IA and altered lFCD values in DMN. These findings might provide novel insights into the underlying pathophysiological mechanisms of IA and the underlying association between IA and complications.
Increased lFCD Values in CCN
The CCN, which underlies the ability to allow for appropriate actions through the suppression of inappropriate motor, is thought to be the key determinant in substance dependence model (16). In our study, the IA individuals exhibited heightened lFCD values in the right DLPFC, left cerebellum and bilateral MCC, all of which are main components of the CCN.
The DLPFC is one of the most well-investigated areas in the brain, in particular in the context of cognitive function. As the core node of CCN, the DLPFC is involved in a variety of advanced cognitive functions essential for decision-making and goal-directed behavior, such as self-control (32), action planning (33), and intertemporal choice (34). Dysfunction in DLPFC has been found in many cognitive control disorders (35–37), in particular in substance dependence (38, 39). Evidence from previous neuroimaging studies has demonstrated the pivotal role of DLPFC dysfunction in craving for marijuana (40), cigarette (41), and alcohol (42), and, thus, the altered DLPFC function was presumed as a neuropathological explanation of substance addiction (43). The cingulate cortex, involved in cognition and decision making, is also implicated in various addictions (44, 45). As a vital component of cingulate cortex, the MCC is engaged in decision making for reward/approach and fear/avoidance selection by assessment of potential outcomes (46, 47), and therefore closely related to substance dependence (48).
As to IA, multiple studies attempting to elucidate the pathological mechanism of IA have converged on the abnormalities of the DLPFC and cingulate cortex (49, 50). Higher Regional homogeneity (ReHo) values in the DLPFC and cingulate cortex have been revealed, reflecting the enhanced synchronization between the DLPFC, and cingulate cortex in individuals with IA (51). Additionally, hyperactivity in the DLPFC and cingulate cortex was also found when the IA subjects were exposed to an internet-related cue (52). Furthermore, altered gray matter in the DLPFC and MCC were revealed in IA group by structural MRI (sMRI), suggesting that the functional alterations in the DLPFC, and MCC were based on the structural abnormalities (53, 54). In our study, the heightened lFCD values in the right DLPFC and bilateral MCC observed in IA individuals further demonstrated the dysfunctional CCN in IA, providing new evidence supporting the hypothesis that IA shares similar neurobiological mechanism with substance addiction.
The cerebellum was traditionally considered as a motor center, well-known for its involvement in postural, and motor control. However, in recent studies, cerebellum abnormalities were found in cognitive disorders (55, 56). Conversely, cerebellum resections or lesions caused various types of abnormal social behavior (57, 58). Providing new insights into the cerebellum function, recent studies revealed the role of cerebellum in cognitive control function (59, 60). Furthermore, structural and functional changes in the cerebellum were found in substance-dependent individuals who exhibited uncontrolled craving for substance (61, 62). Similar to those in substance addiction, cerebellum abnormalities were also demonstrated in IA, associated with the severity of addiction (63). Consistent with the previous studies, our results proved the remarkably increased lFCD in cerebellum in IA group, supporting the speculation that there were homological neurobiological changes in the CCN in IA and substance addiction.
Increased lFCD in DMN and Decreased lFCD in Visual Attention Network
The DMN is generally regarded as the most significant component of the resting state network, due to its typical deactivation during attention-demanding cognitive tasks and activation during internal mentation (e.g., retrieval of episodic memory, self-referential, and imagination of future) (64–67). Dysfunctional DMN is implicated in numerous mental disorders, in particular in ADHD. In our study, enhanced lFCD values in the left PHG and bilateral STP, both are integral parts of the DMN, were revealed in IA group.
As an important part of limbic system, the PHG is responding to emotional, sensory, and environmental stimuli (68–70), and involved in contextual associations, which means processing schema (object-context) associations (71). Due to the function of linking specific sensations to contextual predictions, the PHG is thought to play an essential role in attention (72). Similarly, as an integral component of paralimbic region, the temporal pole is involved in spatial navigation and retrieval of memory (73, 74), which are also thought to be important in attentional allocation (72). In previous studies, structural, and functional alterations in the PHG and temporal pole were implicated in the neuropathology of ADHD (75–78). Consistent with those in ADHD, lFCD in the PHG and STP were revealed in our study, implicating the enhanced functional connectivity of DMN in IA individuals.
Contrary to the enhanced DMN, the weaker lFCD values in the right IPL, and bilateral calcarine, and lingual gyrus were observed in our study. All of these regions are components of the visual attention network (79, 80), involved in the bottom-up attentional pathway which is proposed as a circuit-breaker to reorient attention to new and external information (81). Abnormalities in visual attention network were associated with impaired concentration in previous studies (72, 82).
As the primary visual cortex, the calcarine plays a major role in visual information integration and attention processing (83). Structural and functional alterations in the calcarine were revealed in ADHD (84, 85). As the secondary visual cortex, the lingual gyrus has a critical function in spatial memory (86), and visual attention (87). Likewise, abnormalities in the lingual gyrus in ADHD children were identified in the existing literatures (88, 89). Due to its core role in attentional reorienting and visuomotor integration (80), the IPL is regarded as a supramodal core in the ventral attention network. Hypoactivity in ventral attention network, implicating insensitivity with external environment, is proposed as a key determinant in impairment of attention (90). Both structural and functional abnormalities in IPL were associated with ADHD in previous studies (91, 92). As to IA, abnormalities in the IPL and lingual gyrus were also revealed in IA adolescents, implicating the role of visual attention network in the impaired concentration in IA (93, 94).
The association between IA and ADHD has been demonstrated in previous epidemiology studies. ADHD is considered as the most remarkably associated mental disorder of IA (9). Adolescents with IA have obviously higher ADHD symptoms, among which lack of concentration is the most associated one with IA (95). Prior neural evidence has implicated the importance of hyper-connectivity within DMN (96, 97) and hypo-connectivity within the visual attention network (98) in the inattention in patients with ADHD. In our study, the increased lFCD values in the left PHG and bilateral STP and the decreased lFCD values in the right IPL and bilateral calcarine and lingual gyrus were observed, indicating the heightened functional connectivity of DMN and reduced functional connectivity of visual attention network in IA, which were similar with those in ADHD. To our knowledge, this was the first time the STP and calcarine were associated to IA. These findings, providing direct neural explanation for the impaired concentration in IA, demonstrated the underlying association between IA and ADHD in terms of neurobiology. Additionally, we further revealed the positive correlations between the IAT score and the lFCD in left PHG and bilateral STP, implicating the pivotal role of DMN dysfunction in development of IA.
Limitations
Our study has several limitations. First, FCD was restricted to the local functional connectivity in our study. We did not calculate the global FCD due to the misgiving of its high variability (99). And furthermore, according to the seminal paper, the local FCD, and global FCD are proportional to one another (18). Second, due to the small subject size and the sex difference in morbidity, our study fails to analyze the intergender differences. Finally, subjects are restricted to college students, which limits the generalization of this study.
Conclusion
In the present study, a voxel-wise method was performed to analyze the whole-brain FC abnormalities in IA subjects. Our results revealed that individuals with IA exhibited heightened lFCD values in the CCN and DMN as well as decreased lFCD values in the visual attention network. Our observations, providing neural explanation for the lack of concentration and uncontrolled use of internet in IA individuals, verified the similar neurobiological mechanism in IA with that in substance addiction, and implicated the underlying association between IA and ADHD in terms of neurobiology.
Data Availability
The datasets generated for this study are available on request to the corresponding author.
Ethics Statement
This study was carried out in accordance with the recommendations of Ethical Principles for Medical Research Involving Human of Chengdu University of TCM with written informed consent from all subjects. All subjects gave written informed consent in accordance with the Declaration of Helsinki. The protocol was approved by the Sichuan Regional Ethics Review Committee on traditional Chinese medicine.
Author Contributions
TZ, CL, and DY conceptualized the study, designed the protocol, and administered the project. HL supervised the study. YW, YD, CW, LZ, and CZ preformed the experiments. YQ, BS, ZL, and XL conducted the statistical analysis. YW wrote the first draft of the manuscript. All authors read and approved the manuscript.
Funding
This research was supported by the Natural Science Foundation of China (81072852 and 81574047), the Key Research and Development Projects of Sichuan Science and Technology Department (2019YFS0175), the Xinglin Scholars Scientific Research Promotion Program of Chengdu University of Traditional Chinese Medicine (XSGG2019007) and the Training Funds of Academic and Technical Leader in Sichuan Province.
Conflict of Interest Statement
The authors declare that the research was conducted in the absence of any commercial or financial relationships that could be construed as a potential conflict of interest.
Acknowledgments
We are grateful for the participation of all volunteers and the useful suggestion given by Prof. Tang Yong of Chengdu University of Traditional Chinese Medicine.
References
1. Young KS. Internet addiction: the emergence of a new clinical disorder. CyberPsychol Behav. (1998) 1:237–44. doi: 10.1089/cpb.1998.1.237
2. Sevelko K, Bischof G, Bischof A, Besser B, John U, Meyer C, et al. The role of self-esteem in Internet addiction within the context of comorbid mental disorders: findings from a general population-based sample. J Behav Addict. (2018) 7:976–84. doi: 10.1556/2006.7.2018.130
3. Li L, Xu DD, Chai JX, Wang D, Li L, Zhang L, et al. Prevalence of Internet addiction disorder in Chinese university students: a comprehensive meta-analysis of observational studies. J Behav Addict. (2018) 7:610–23. doi: 10.1556/2006.7.2018.53
4. Chung TWH, Sum SMY, Chan MWL. Adolescent internet addiction in hong kong: prevalence, psychosocial correlates, and prevention. J Adoles Health. (2019) 64:S34–43. doi: 10.1016/j.jadohealth.2018.12.016
5. Weinstein A, Lejoyeux M. Internet addiction or excessive internet use. Am J Drug Alcohol Abuse. (2010) 36:277–83. doi: 10.3109/00952990.2010.491880
6. Lam LT. Risk factors of Internet addiction and the health effect of internet addiction on adolescents: a systematic review of longitudinal and prospective studies. Curr Psychiatry Rep. (2014) 16:508. doi: 10.1007/s11920-014-0508-2
7. Trumello C, Babore A, Candelori C, Morelli M, Bianchi D. Relationship with parents, emotion regulation, and callous-unemotional traits in adolescents' internet addiction. Biomed Res Int. (2018) 2018:7914261. doi: 10.1155/2018/7914261
8. Chou WJ, Liu TL, Yang P, Yen CF, Hu HF. Multi-dimensional correlates of Internet addiction symptoms in adolescents with attention-deficit/hyperactivity disorder. Psychiatry Res. (2015) 225:122–8. doi: 10.1016/j.psychres.2014.11.003
9. Ko CH, Yen JY, Chen CS, Chen CC, Yen CF. Psychiatric comorbidity of internet addiction in college students: an interview study. CNS Spectr. (2008) 13:147–53. doi: 10.1017/S1092852900016308
10. Biswal B, Yetkin FZ, Haughton VM, Hyde JS. Functional connectivity in the motor cortex of resting human brain using echo-planar MRI. Magnetic Res Med. (1995) 34:537–41. doi: 10.1002/mrm.1910340409
11. Weinstein AM. An update overview on brain imaging studies of internet gaming disorder. Front Psychiatry. (2017) 8:185. doi: 10.3389/fpsyt.2017.00185
12. Han DH, Kim SM, Bae S, Renshaw PF, Anderson JS. Brain connectivity and psychiatric comorbidity in adolescents with Internet gaming disorder. Addict Biol. (2017) 22:802–12. doi: 10.1111/adb.12347
13. Anderson JS, Nielsen JA, Froehlich AL, DuBray MB, Druzgal TJ, Cariello AN, et al. Functional connectivity magnetic resonance imaging classification of autism. Brain. (2011) 134:3739–51. doi: 10.1093/brain/awr263
14. Anderson JS, Nielsen JA, Ferguson MA, Burback MC, Cox ET, Dai L, et al. Abnormal brain synchrony in down syndrome. Neuroimage Clinical. (2013) 2:703–15. doi: 10.1016/j.nicl.2013.05.006
15. Li W, Li Y, Yang W, Zhang Q, Wei D, Li W, et al. Brain structures and functional connectivity associated with individual differences in Internet tendency in healthy young adults. Neuropsychologia. (2015) 70:134–44. doi: 10.1016/j.neuropsychologia.2015.02.019
16. Volkow ND, Wang GJ, Fowler JS, Tomasi D, Telang F, Baler R. Addiction: decreased reward sensitivity and increased expectation sensitivity conspire to overwhelm the brain's control circuit. Bioessays. (2010) 32:748–55. doi: 10.1002/bies.201000042
17. Kim HS, Hodgins DC. Component model of addiction treatment: a pragmatic transdiagnostic treatment model of behavioral and substance addictions. Front Psychiatry. (2018) 9:406. doi: 10.3389/fpsyt.2018.00406
18. Tomasi D, Volkow ND. Functional connectivity density mapping. Proc Natl Acad Sci USA. (2010) 107:9885–90. doi: 10.1073/pnas.1001414107
19. Tomasi D, Volkow ND. Ultrafast method for mapping local functional connectivity hubs in the human brain. Conf Proc IEEE Eng Med Biol Soc. (2010) 2010:4274–7. doi: 10.1109/IEMBS.2010.5626180
20. Qin W, Xuan Y, Liu Y, Jiang T, Yu C. Functional connectivity density in congenitally and late blind subjects. Cereb Cortex. (2015) 25:2507–16. doi: 10.1093/cercor/bhu051
21. Manza P, Tomasi D, Volkow ND. Subcortical local functional hyperconnectivity in cannabis dependence. Biol Psychiatry. (2018) 3:285–93. doi: 10.1016/j.bpsc.2017.11.004
22. Shokri-Kojori E, Tomasi D, Wiers CE, Wang GJ, Volkow ND. Alcohol affects brain functional connectivity and its coupling with behavior: greater effects in male heavy drinkers. Mol Psychiatry. (2017) 22:1185–95. doi: 10.1038/mp.2016.25
23. Goodman WK, Price LH, Rasmussen SA, Mazure C, Delgado P, Heninger GR, et al. The yale-brown obsessive compulsive scale. II. validity. Arch Gen Psychiatry. (1989) 46:1012–6. doi: 10.1001/archpsyc.1989.01810110054008
24. Patton JH, Stanford MS, Barratt ES. Factor structure of the Barratt impulsiveness scale. J Clin Psychol. (1995) 51:768–74. doi: 10.1002/1097-4679(199511)51:6<768::AID-JCLP2270510607>3.0.CO;2-1
25. Cordes D, Haughton VM, Arfanakis K, Carew JD, Turski PA, Moritz CH, et al. Frequencies contributing to functional connectivity in the cerebral cortex in “resting-state” data. AJNR Am. J. Neuroradiol. (2001) 22:1326–33.
26. Liu T, Li J, Zhang Z, Xu Q, Lu G, Huang S, et al. Altered long- and short-range functional connectivity in patients with betel quid dependence: a resting-state functional MRI study. Cell Physiol Biochem. (2016) 40:1626–36. doi: 10.1159/000453212
27. Cohen AD, Tomasi D, Shokri-Kojori E, Nencka AS, Wang Y. Functional connectivity density mapping: comparing multiband and conventional EPI protocols. Brain Imaging Behav. (2018) 12:848–59. doi: 10.1007/s11682-017-9742-7
28. Luo C, Tu SP, Peng YH, Gao S, Li JF, Dong L, et al. Long-term effects of musical training and functional plasticity in salience system. Neural Plast. (2014) 2014:180138. doi: 10.1155/2014/180138
29. Friston KJ. Testing for anatomically specified regional effects. Hum Brain Mapp. (1997) 5:133–6. doi: 10.1002/(SICI)1097-0193(1997)5:2<133::AID-HBM7>3.0.CO;2-4
30. Power JD. A simple but useful way to assess fMRI scan qualities. NeuroImage. (2017) 154:150–8. doi: 10.1016/j.neuroimage.2016.08.009
31. Power JD, Plitt M, Laumann TO, Martin A. Sources and implications of whole-brain fMRI signals in humans. NeuroImage. (2017) 146:609–25. doi: 10.1016/j.neuroimage.2016.09.038
32. Hare TA, Camerer CF, Rangel A. Self-control in decision-making involves modulation of the vmPFC valuation system. Science. (2009) 324:646–8. doi: 10.1126/science.1168450
33. Mushiake H, Saito N, Sakamoto K, Itoyama Y, Tanji J. Activity in the lateral prefrontal cortex reflects multiple steps of future events in action plans. Neuron. (2006) 50:631–41. doi: 10.1016/j.neuron.2006.03.045
34. Kim S, Hwang J, Lee D. Prefrontal coding of temporally discounted values during intertemporal choice. Neuron. (2008) 59:161–72. doi: 10.1016/j.neuron.2008.05.010
35. Hoppenbrouwers SS, De DR, Stirpe T, Fitzgerald PB, Voineskos AN, Schutter D, et al. Inhibitory deficits in the dorsolateral prefrontal cortex in psychopathic offenders. Cortex. (2013) 49:1377–85. doi: 10.1016/j.cortex.2012.06.003
36. Weygandt M, Mai K, Dommes E, Leupelt V, Hackmack K, Kahnt T, et al. The role of neural impulse control mechanisms for dietary success in obesity. Neuroimage. (2013) 83:669–78. doi: 10.1016/j.neuroimage.2013.07.028
37. Weygandt M, Mai K, Dommes E, Ritter K, Leupelt V, Spranger J, et al. Impulse control in the dorsolateral prefrontal cortex counteracts post-diet weight regain in obesity. Neuroimage. (2015) 109:318–27. doi: 10.1016/j.neuroimage.2014.12.073
38. Ernst LH, Plichta MM, Dresler T, Zesewitz AK, Tupak SV, Haeussinger FB, et al. Prefrontal correlates of approach preferences for alcohol stimuli in alcohol dependence. Addict Biol. (2014) 19:497–508. doi: 10.1111/adb.12005
39. Chang D, Zhang J, Peng W, Shen ZW, Gao X, Du YH, et al. Smoking cessation with 20 Hz repetitive transcranial magnetic stimulation (rTMS) applied to two brain regions: a pilot study. Front Human Neurosci. (2018) 12:344. doi: 10.3389/fnhum.2018.00344
40. Boggio PS, Zaghi S, Villani AB, Fecteau S, Pascual-Leone A, Fregni F. Modulation of risk-taking in marijuana users by transcranial direct current stimulation (tDCS) of the dorsolateral prefrontal cortex (DLPFC). Drug Alcohol Depend. (2010) 112:220–5. doi: 10.1016/j.drugalcdep.2010.06.019
41. Hayashi T, Ko JH, Strafella AP, Dagher A. Dorsolateral prefrontal and orbitofrontal cortex interactions during self-control of cigarette craving. Proc Natl Acad Sci USA. (2013) 110:4422–7. doi: 10.1073/pnas.1212185110
42. Klauss J, Pinheiro LCP, Merlo BLS, Santos GDC, Fregni F, Nitsche MA, et al. A randomized controlled trial of targeted prefrontal cortex modulation with tDCS in patients with alcohol dependence. Int J Neuropsychopharmacol. (2014) 17:1793–803. doi: 10.1017/S1461145714000984
43. Owens MM, Syan SK, Amlung M, Beach SRH, Sweet LH, MacKillop J. Functional and structural neuroimaging studies of delayed reward discounting in addiction: a systematic review. Psychol Bull. (2019) 145:141–64. doi: 10.1037/bul0000181
44. Ersche KD, Barnes A, Jones PS, Morein-Zamir S, Robbins TW, Bullmore ET. Abnormal structure of frontostriatal brain systems is associated with aspects of impulsivity and compulsivity in cocaine dependence. Brain. (2011) 134:2013–24. doi: 10.1093/brain/awr138
45. Schacht JP, Anton RF, Myrick H. Functional neuroimaging studies of alcohol cue reactivity: a quantitative meta-analysis and systematic review. Addict Biol. (2013) 18:121–33. doi: 10.1111/j.1369-1600.2012.00464.x
46. Vogt BA. Submodalities of emotion in the context of cingulate subregions. Cortex. (2014) 59:197–202. doi: 10.1016/j.cortex.2014.04.002
47. Vogt BA. Midcingulate cortex: structure, connections, homologies, functions and diseases. J Chem Neuroanat. (2016) 74:28–46. doi: 10.1016/j.jchemneu.2016.01.010
48. Kuhn J, Grundler TO, Bauer R, Huff W, Fischer AG, Lenartz D, et al. Successful deep brain stimulation of the nucleus accumbens in severe alcohol dependence is associated with changed performance monitoring. Addict Biol. (2011) 16:620–3. doi: 10.1111/j.1369-1600.2011.00337.x
49. Du X, Yang Y, Gao P, Qi X, Du G, Zhang Y, et al. Compensatory increase of functional connectivity density in adolescents with internet gaming disorder. Brain Imaging Behav. (2017) 11:1901–9. doi: 10.1007/s11682-016-9655-x
50. Chun J-W, Choi J, Cho H, Choi M-R, Ahn K-J, Choi J-S, et al. Role of frontostriatal connectivity in adolescents with excessive smartphone use. Front Psychiatry. (2018) 9:437. doi: 10.3389/fpsyt.2018.00437
51. Liu J, Gao XP, Osunde I, Li X, Zhou SK, Zheng HR, et al. Increased regional homogeneity in internet addiction disorder: a resting state functional magnetic resonance imaging study. Chinese Med J. (2010) 123:1904–8. doi: 10.3760/cma.j.issn.0366-6999.2010.14.014
52. Ko CH, Liu GC, Yen JY, Chen CY, Yen CF, Chen CS. Brain correlates of craving for online gaming under cue exposure in subjects with internet gaming addiction and in remitted subjects. Addict Biol. (2013) 18:559–69. doi: 10.1111/j.1369-1600.2011.00405.x
53. Jin CW, Zhang T, Cai CX, Bi YZ, Li YD, Yu DH, et al. Abnormal prefrontal cortex resting state functional connectivity and severity of internet gaming disorder. Brain Imaging Behav. (2016) 10:719–29. doi: 10.1007/s11682-015-9439-8
54. He QH, Turel O, Bechara A. Brain anatomy alterations associated with social networking site (SNS) addiction. Sci Rep. (2017) 7:8. doi: 10.1038/srep45064
55. Zarei M, Mataix-Cols D, Heyman I, Hough M, Doherty J, Burge L, et al. Changes in gray matter volume and white matter microstructure in adolescents with obsessive-compulsive disorder. Biol Psychiatry. (2011) 70:1083–90. doi: 10.1016/j.biopsych.2011.06.032
56. Seidman LJ, Biederman J, Liang L, Valera EM, Monuteaux MC, Brown A, et al. Gray matter alterations in adults with attention-deficit/hyperactivity disorder identified by voxel based morphometry. Biol Psychiatry. (2011) 69:857–66. doi: 10.1016/j.biopsych.2010.09.053
57. Hoche F, Guell X, Sherman JC, Vangel MG, Schmahmann JD. Cerebellar contribution to social cognition. Cerebellum. (2016) 15:732–43. doi: 10.1007/s12311-015-0746-9
58. Schmahmann JD, Sherman JC. The cerebellar cognitive affective syndrome. Brain. (1998) 121(Pt 4):561–79. doi: 10.1093/brain/121.4.561
59. Carta I, Chen CH, Schott AL, Dorizan S, Khodakhah K. Cerebellar modulation of the reward circuitry and social behavior. Science. (2019) 363:eaav0581. doi: 10.1126/science.aav0581
60. Caulfield MD, Zhu DC, McAuley JD, Servatius RJ. Individual differences in resting-state functional connectivity with the executive network: support for a cerebellar role in anxiety vulnerability. Brain Struct Funct. (2016) 221:3081–93. doi: 10.1007/s00429-015-1088-6
61. Hester R, Garavan H. Executive dysfunction in cocaine addiction: evidence for discordant frontal, cingulate, and cerebellar activity. J Neurosci. (2004) 24:11017–22. doi: 10.1523/JNEUROSCI.3321-04.2004
62. Brody AL, Mandelkern MA, Jarvik ME, Lee GS, Smith EC, Huang JC, et al. Differences between smokers and nonsmokers in regional gray matter volumes and densities. Biol Psychiatry. (2004) 55:77–84. doi: 10.1016/S0006-3223(03)00610-3
63. Ding WN, Sun JH, Sun YW, Zhou Y, Li L, Xu JR, et al. Altered default network resting-state functional connectivity in adolescents with Internet gaming addiction. PLoS ONE. (2013) 8:e59902. doi: 10.1371/journal.pone.0059902
64. Schacter DL, Addis DR, Buckner RL. Remembering the past to imagine the future: the prospective brain. Nat Rev Neurosci. (2007) 8:657–61. doi: 10.1038/nrn2213
65. Spreng RN, Mar RA, Kim AS. The common neural basis of autobiographical memory, prospection, navigation, theory of mind, and the default mode: a quantitative meta-analysis. J Cogn Neurosci. (2009) 21:489–510. doi: 10.1162/jocn.2008.21029
66. Amodio DM, Frith CD. Meeting of minds: the medial frontal cortex and social cognition. Nat Rev Neurosci. (2006) 7:268–77. doi: 10.1038/nrn1884
67. Simpson JR Jr, Drevets WC, Snyder AZ, Gusnard DA, Raichle ME. Emotion-induced changes in human medial prefrontal cortex: II. During anticipatory anxiety. Proc Natl Acad Sci USA. (2001) 98:688–93. doi: 10.1073/pnas.98.2.688
68. Van den Stock J, Vandenbulcke M, Sinke CB, de Gelder B. Affective scenes influence fear perception of individual body expressions. Hum Brain Mapp. (2014) 35:492–502. doi: 10.1002/hbm.22195
69. Rajimehr R, Devaney KJ, Bilenko NY, Young JC, Tootell RB. The “parahippocampal place area” responds preferentially to high spatial frequencies in humans and monkeys. PLoS Biol. (2011) 9:e1000608. doi: 10.1371/journal.pbio.1000608
70. Levy I, Hasson U, Avidan G, Hendler T, Malach R. Center-periphery organization of human object areas. Nat Neurosci. (2001) 4:533–9. doi: 10.1038/87490
71. Aminoff EM, Kveraga K, Bar M. The role of the parahippocampal cortex in cognition. Trends Cogn Sci. (2013) 17:379–90. doi: 10.1016/j.tics.2013.06.009
72. Ramzaoui H, Faure S, Spotorno S. Alzheimer's Disease, visual search, and instrumental activities of daily living: a review and a new perspective on attention and eye movements. J Alzheimers Dis. (2018) 66:901–25. doi: 10.3233/JAD-180043
73. Frith U, Frith CD. Development and neurophysiology of mentalizing. Philos Trans R Soc Lond B Biol Sci. (2003) 358:459–73. doi: 10.1098/rstb.2002.1218
74. Shikauchi Y, Ishii S. Robust encoding of scene anticipation during human spatial navigation. Sci Rep. (2016) 6:14. doi: 10.1038/srep37599
75. Fernandez-Jaen A, Lopez-Martin S, Albert J, Fernandez-Mayoralas DM, Fernandez-Perrone AL, Tapia DQ, et al. Cortical thinning of temporal pole and orbitofrontal cortex in medication-naive children and adolescents with ADHD. Psychiatry Res Neuroimaging. (2014) 224:8–13. doi: 10.1016/j.pscychresns.2014.07.004
76. Van Dessel J, Sonuga-Barke E, Moerkerke M, Van der Oord S, Lemiere J, Morsink S, et al. The amygdala in adolescents with attention-deficit/hyperactivity disorder: structural and functional correlates of delay aversion. World J Biol Psychiatry. (2019). doi: 10.1080/15622975.2019.1585946. [Epub ahead of print].
77. Wilbertz G, Delgado MR, Van Elst LT, Maier S, Philipsen A, Blechert J. Neural response during anticipation of monetary loss is elevated in adult attention deficit hyperactivity disorder. World J Biol Psychiatry. (2017) 18:268–78. doi: 10.3109/15622975.2015.1112032
78. Kim JH, Chung YI, Lee JS, Kim IJ, Kim YK, Kim SJ. Voxel-based statistical analysis of regional cerebral glucose metabolism in children with attention-deficit hyperactivity disorder. Neural Regenerat Res. (2011) 6:2850–5. doi: 10.3969/j.issn.1673-5374.2011.36.009
79. Goodale MA, Milner AD. Separate visual pathways for perception and action. Trends Neurosci. (1992) 15:20–5. doi: 10.1016/0166-2236(92)90344-8
80. Igelstrom KM, Graziano MSA. The inferior parietal lobule and temporoparietal junction: a network perspective. Neuropsychologia. (2017) 105:70–83. doi: 10.1016/j.neuropsychologia.2017.01.001
81. Corbetta M, Patel G, Shulman GL. The reorienting system of the human brain: from environment to theory of mind. Neuron. (2008) 58:306–24. doi: 10.1016/j.neuron.2008.04.017
82. Eggebrecht AT, Elison JT, Feczko E, Todorov A, Wolff JJ, Kandala S, et al. Joint attention and brain functional connectivity in infants and toddlers. Cereb Cortex. (2017) 27:1709–20. doi: 10.1093/cercor/bhw403
83. Bezdek MA, Gerrig RJ, Wenzel WG, Shin J, Pirog Revill K, Schumacher EH. Neural evidence that suspense narrows attentional focus. Neuroscience. (2015) 303:338–45. doi: 10.1016/j.neuroscience.2015.06.055
84. Kumar U, Arya A, Agarwal V. Neural alterations in ADHD children as indicated by voxel-based cortical thickness and morphometry analysis. Brain Dev. (2017) 39:403–10. doi: 10.1016/j.braindev.2016.12.002
85. Saad JF, Griffiths KR, Kohn MR, Clarke S, Williams LM, Korgaonkar MS. Regional brain network organization distinguishes the combined and inattentive subtypes of attention deficit hyperactivity disorder. Neuroimage Clin. (2017) 15:383–90. doi: 10.1016/j.nicl.2017.05.016
86. Sulpizio V, Committeri G, Lambrey S, Berthoz A, Galati G. Selective role of lingual/parahippocampal gyrus and retrosplenial complex in spatial memory across viewpoint changes relative to the environmental reference frame. Behav Brain Res. (2013) 242:62–75. doi: 10.1016/j.bbr.2012.12.031
87. Gebodh N, Vanegas MI, Kelly SP. Effects of stimulus size and contrast on the initial primary visual cortical response in humans. Brain Topogr. (2017) 30:450–60. doi: 10.1007/s10548-016-0530-2
88. Wang JB, Zheng LJ, Cao QJ, Wang YF, Sun L, Zang YF, et al. Inconsistency in abnormal brain activity across cohorts of ADHD-200 in children with attention deficit hyperactivity disorder. Front Neurosci. (2017) 11:320. doi: 10.3389/fnins.2017.00320
89. McLaughlin KA, Sheridan MA, Winter W, Fox NA, Zeanah CH, Nelson CA. Widespread reductions in cortical thickness following severe early-life deprivation: a neurodevelopmental pathway to attention-deficit/hyperactivity disorder. Biol Psychiatry. (2014) 76:629–38. doi: 10.1016/j.biopsych.2013.08.016
90. Jiang LW, Qian RB, Fu XM, Zhang D, Peng N, Niu CS, et al. Altered attention networks and DMN in refractory epilepsy: a resting-state functional and causal connectivity study. Epilepsy Behav. (2018) 88:81–6. doi: 10.1016/j.yebeh.2018.06.045
91. Sethi A, Evelyn-Rahr E, Dowell N, Jain S, Voon V, Critchley HD, et al. Magnetization transfer imaging identifies basal ganglia abnormalities in adult ADHD that are invisible to conventional T1 weighted voxel-based morphometry. NeuroImage Clin. (2017) 15:8–14. doi: 10.1016/j.nicl.2017.03.012
92. Zhou M, Yang C, Bu X, Liang Y, Lin H, Hu X, et al. Abnormal functional network centrality in drug-naive boys with attention-deficit/hyperactivity disorder. Euro Child Adoles Psychiatry. (2019). doi: 10.1007/s00787-019-01297-6. [Epub ahead of print].
93. Wang M, Zheng H, Du X, Dong G. Mapping internet gaming disorder using effective connectivity: a spectral dynamic causal modeling study. Addict Behav. (2019) 90:62–70. doi: 10.1016/j.addbeh.2018.10.019
94. Yuan K, Cheng P, Dong T, Bi Y, Xing L, Yu D, et al. Cortical thickness abnormalities in late adolescence with online gaming addiction. PLoS ONE. (2013) 8:e53055. doi: 10.1371/journal.pone.0053055
95. Yen JY, Yen CF, Chen CS, Tang TC, Ko CH. The association between adult ADHD symptoms and internet addiction among college students: the gender difference. Cyberpsychol Behav. (2009) 12:187–91. doi: 10.1089/cpb.2008.0113
96. Barber AD, Jacobson LA, Wexler JL, Nebel MB, Caffo BS, Pekar JJ, et al. Connectivity supporting attention in children with attention deficit hyperactivity disorder. Neuroimage Clin. (2015) 7:68–81. doi: 10.1016/j.nicl.2014.11.011
97. Qian X, Castellanos FX, Uddin LQ, Loo BRY, Liu SW, Koh HL, et al. Large-scale brain functional network topology disruptions underlie symptom heterogeneity in children with attention-deficit/hyperactivity disorder. NeuroImage Clin. (2019) 21:8. doi: 10.1016/j.nicl.2018.11.010
98. Xia SG, Foxe JJ, Sroubek AE, Branch C, Li XB. Topological organization of the “small-world” visual attention network in children with attention deficit/hyperactivity disorder (ADHD). Front Hum Neurosci. (2014) 8:14. doi: 10.3389/fnhum.2014.00162
Keywords: internet addiction, cognitive control network, default mode network, visual attention network, functional connectivity density, substance addiction, attention deficit/hyperactivity disorder
Citation: Wang Y, Qin Y, Li H, Yao D, Sun B, Li Z, Li X, Dai Y, Wen C, Zhang L, Zhang C, Zhu T and Luo C (2019) Abnormal Functional Connectivity in Cognitive Control Network, Default Mode Network, and Visual Attention Network in Internet Addiction: A Resting-State fMRI Study. Front. Neurol. 10:1006. doi: 10.3389/fneur.2019.01006
Received: 28 April 2019; Accepted: 03 September 2019;
Published: 18 September 2019.
Edited by:
Peter Sörös, University of Oldenburg, GermanyReviewed by:
Luke Norman, University of Michigan, United StatesJunjie Wu, Emory University School of Medicine, United States
Copyright © 2019 Wang, Qin, Li, Yao, Sun, Li, Li, Dai, Wen, Zhang, Zhang, Zhu and Luo. This is an open-access article distributed under the terms of the Creative Commons Attribution License (CC BY). The use, distribution or reproduction in other forums is permitted, provided the original author(s) and the copyright owner(s) are credited and that the original publication in this journal is cited, in accordance with accepted academic practice. No use, distribution or reproduction is permitted which does not comply with these terms.
*Correspondence: Tianmin Zhu, dGlhbm1pbnpodSYjeDAwMDQwO2NkdXRjbS5lZHUuY24=; Cheng Luo, Y2hlbmdsdW8mI3gwMDA0MDt1ZXN0Yy5lZHUuY24=